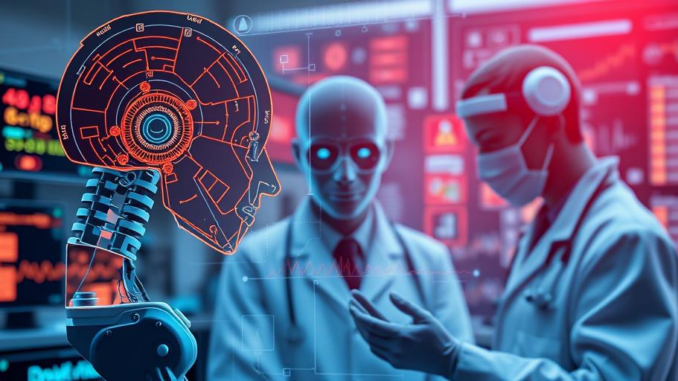
Artificial Intelligence in Personalized Medicine: A Comprehensive Review of Applications, Challenges, and Future Directions
Abstract
Personalized medicine, driven by the integration of individual patient data with advanced analytical tools, represents a paradigm shift in healthcare. Artificial intelligence (AI), with its capacity for complex pattern recognition and predictive modeling, stands at the forefront of this revolution. This research report provides a comprehensive overview of the applications of AI in personalized medicine, examining its impact across various domains including diagnostics, drug discovery, treatment optimization, and predictive health management. We critically evaluate the methodologies employed, highlighting both the significant advancements and the inherent limitations of current AI-driven personalized medicine approaches. Furthermore, we delve into the ethical, regulatory, and data privacy challenges associated with widespread AI implementation, emphasizing the need for robust frameworks to ensure responsible and equitable use. Finally, we discuss future directions, exploring emerging trends and potential breakthroughs that promise to further enhance the precision and efficacy of personalized healthcare through AI.
1. Introduction
The traditional “one-size-fits-all” approach to medicine often falls short in addressing the unique needs and characteristics of individual patients. Factors such as genetic makeup, lifestyle, environmental exposures, and disease progression can significantly influence treatment outcomes. Personalized medicine, also known as precision medicine, aims to tailor medical interventions to the specific attributes of each patient, thereby maximizing therapeutic benefits and minimizing adverse effects. This personalized approach relies heavily on the integration of diverse data types – including genomics, proteomics, imaging data, electronic health records (EHRs), and wearable sensor data – to develop a comprehensive understanding of an individual’s health profile.
Artificial intelligence (AI), particularly machine learning (ML) and deep learning (DL), has emerged as a powerful tool for analyzing these complex datasets and extracting clinically relevant insights. AI algorithms can identify subtle patterns, predict disease risk, optimize treatment strategies, and accelerate drug discovery, all with a level of precision and efficiency that surpasses traditional methods. The convergence of AI and personalized medicine holds immense promise for transforming healthcare delivery, improving patient outcomes, and reducing healthcare costs. However, the implementation of AI in personalized medicine also presents significant challenges, including data privacy concerns, algorithmic bias, regulatory hurdles, and the need for robust validation and clinical integration.
2. AI-Driven Diagnostics
Early and accurate diagnosis is crucial for effective disease management. AI is revolutionizing diagnostic processes by enabling faster, more accurate, and less invasive detection of diseases across various medical specialties.
-
Imaging Diagnostics: AI algorithms excel at analyzing medical images, such as X-rays, CT scans, MRIs, and histopathology slides, to identify anomalies and assist radiologists in making more informed diagnoses. Convolutional neural networks (CNNs), a type of deep learning architecture, have demonstrated remarkable performance in detecting cancers, cardiovascular diseases, and neurological disorders from medical images. For example, AI-powered systems can detect subtle signs of lung cancer on CT scans that might be missed by human radiologists, leading to earlier diagnosis and improved survival rates [1]. Furthermore, AI can quantify disease severity, track disease progression, and predict patient response to treatment based on image analysis. The application of generative adversarial networks (GANs) for image enhancement and synthesis is also improving the quality and interpretability of medical images, further enhancing the diagnostic capabilities of AI.
-
Genomic Diagnostics: AI algorithms can analyze genomic data to identify disease-causing mutations, predict genetic predispositions to diseases, and classify patients into specific risk groups. Machine learning models, such as support vector machines (SVMs) and random forests, are used to analyze large-scale genomic datasets and identify biomarkers that can be used for early disease detection and personalized treatment selection. For instance, AI can identify patients with a high risk of developing Alzheimer’s disease based on their genomic profile, allowing for early intervention and lifestyle modifications [2]. AI-powered tools are also used to interpret complex genomic test results and provide clinicians with actionable insights for personalized treatment decisions. Furthermore, AI is being used to predict the response of patients to specific drugs based on their genomic makeup, enabling pharmacogenomics-guided treatment.
-
Liquid Biopsy Analysis: Liquid biopsies, which involve analyzing circulating tumor cells (CTCs) or circulating tumor DNA (ctDNA) in blood samples, offer a non-invasive way to detect and monitor cancer progression. AI algorithms can analyze liquid biopsy data to identify tumor-specific mutations, track treatment response, and detect early signs of recurrence. Machine learning models are used to differentiate between tumor-derived DNA and normal DNA, quantify the amount of ctDNA in the blood, and identify mutations that may be associated with drug resistance. AI-powered systems can also analyze CTCs to identify tumor heterogeneity and predict the likelihood of metastasis [3]. The combination of liquid biopsy analysis with AI offers a powerful tool for personalized cancer management.
3. AI in Drug Discovery and Development
Traditional drug discovery is a lengthy, expensive, and often inefficient process. AI is accelerating drug discovery by enabling faster identification of drug targets, prediction of drug efficacy, and optimization of drug design.
-
Target Identification: AI algorithms can analyze large-scale biological datasets, such as genomic, proteomic, and metabolomic data, to identify potential drug targets. Machine learning models can identify genes or proteins that are dysregulated in disease and predict the effect of targeting these molecules with drugs. AI can also analyze the structure and function of proteins to identify potential binding sites for drugs. For example, AI has been used to identify novel drug targets for cancer, Alzheimer’s disease, and infectious diseases. Graph neural networks (GNNs) are particularly useful for analyzing complex biological networks and identifying key nodes that are involved in disease pathways [4].
-
Drug Design and Optimization: AI algorithms can predict the efficacy and safety of drug candidates before they are even synthesized. Machine learning models can analyze the chemical structure of drug molecules and predict their binding affinity to drug targets, their pharmacokinetic properties (absorption, distribution, metabolism, and excretion), and their potential toxicity. AI can also optimize the structure of drug molecules to improve their efficacy and reduce their toxicity. Generative models, such as variational autoencoders (VAEs) and GANs, are used to generate novel drug candidates with desired properties. The use of AI in drug design and optimization can significantly reduce the time and cost of drug development.
-
Clinical Trial Optimization: AI can optimize the design and execution of clinical trials to improve their efficiency and increase the likelihood of success. AI algorithms can identify patients who are most likely to respond to a particular drug, stratify patients into different risk groups, and personalize treatment regimens. AI can also monitor patient data during clinical trials to detect early signs of drug efficacy or toxicity. By using AI to optimize clinical trials, pharmaceutical companies can reduce the time and cost of drug development and increase the likelihood of bringing new drugs to market. The use of synthetic control arms, generated using AI from historical patient data, is also gaining traction as a way to reduce the size and cost of clinical trials.
4. AI for Treatment Optimization and Personalized Treatment Plans
AI can analyze patient data to optimize treatment regimens and personalize treatment plans. This includes predicting patient response to different treatments, optimizing drug dosages, and minimizing side effects.
-
Predicting Treatment Response: Machine learning models can analyze patient data, such as genomic data, clinical data, and imaging data, to predict how a patient will respond to a particular treatment. AI can identify biomarkers that are associated with treatment response and use these biomarkers to personalize treatment selection. For example, AI has been used to predict the response of patients with cancer to chemotherapy, immunotherapy, and targeted therapy. The use of AI to predict treatment response can help clinicians make more informed treatment decisions and improve patient outcomes.
-
Dosage Optimization: AI algorithms can optimize drug dosages based on individual patient characteristics, such as age, weight, kidney function, and liver function. AI can also monitor patient data during treatment to detect early signs of drug toxicity and adjust the dosage accordingly. For example, AI has been used to optimize the dosage of anticoagulants, antibiotics, and chemotherapeutic agents. The use of AI to optimize drug dosages can improve treatment efficacy and reduce the risk of side effects.
-
Personalized Treatment Plans: AI can integrate data from multiple sources, including genomic data, clinical data, imaging data, and patient-reported outcomes, to develop personalized treatment plans. AI can identify the most effective treatment strategies for each patient based on their individual characteristics and disease progression. AI can also monitor patient data during treatment to track treatment response and adjust the treatment plan accordingly. The use of AI to develop personalized treatment plans can improve patient outcomes and reduce healthcare costs. This often involves creating digital twins of patients, allowing for in-silico testing of different treatment options.
5. Predictive Health Management and Preventative Care
AI can analyze patient data to predict the risk of developing diseases and recommend preventative interventions. This includes predicting the risk of developing cardiovascular disease, diabetes, cancer, and other chronic diseases.
-
Disease Risk Prediction: Machine learning models can analyze patient data, such as demographic data, lifestyle data, and family history, to predict the risk of developing diseases. AI can identify risk factors that are associated with disease development and use these risk factors to stratify patients into different risk groups. For example, AI has been used to predict the risk of developing cardiovascular disease, diabetes, and cancer. The use of AI to predict disease risk can allow for early intervention and lifestyle modifications to prevent disease development.
-
Early Detection and Screening: AI can analyze patient data to detect early signs of disease and recommend appropriate screening tests. For example, AI can analyze electrocardiograms (ECGs) to detect early signs of heart disease, analyze mammograms to detect early signs of breast cancer, and analyze colonoscopies to detect early signs of colon cancer. The use of AI to improve early detection and screening can lead to earlier diagnosis and improved survival rates.
-
Lifestyle Recommendations: AI can analyze patient data to provide personalized lifestyle recommendations, such as diet, exercise, and sleep recommendations. AI can identify lifestyle factors that are associated with disease development and recommend lifestyle modifications that can reduce the risk of developing diseases. For example, AI can recommend personalized diet plans based on a patient’s genomic profile, lifestyle, and health goals. The use of AI to provide personalized lifestyle recommendations can improve patient health and prevent disease development.
6. Challenges and Limitations
Despite the tremendous potential of AI in personalized medicine, there are several challenges and limitations that need to be addressed before AI can be widely implemented in clinical practice.
-
Data Privacy and Security: The use of sensitive patient data for AI-driven treatment recommendations raises significant concerns about data privacy and security. It is crucial to ensure that patient data is protected from unauthorized access and misuse. Robust data governance frameworks, encryption techniques, and anonymization strategies are needed to protect patient privacy. Compliance with regulations such as HIPAA and GDPR is essential. Furthermore, the use of federated learning, where AI models are trained on decentralized data sources without sharing the data itself, can help to protect data privacy.
-
Algorithmic Bias: AI algorithms can perpetuate and amplify existing biases in the data they are trained on, leading to disparities in healthcare outcomes. It is crucial to ensure that AI algorithms are trained on diverse and representative datasets to mitigate bias. Furthermore, it is important to monitor AI algorithms for bias and implement strategies to correct for bias when it is detected. The development of fair and equitable AI algorithms is essential for ensuring that all patients benefit from personalized medicine.
-
Interpretability and Explainability: Many AI algorithms, particularly deep learning models, are “black boxes,” meaning that it is difficult to understand how they arrive at their predictions. This lack of interpretability can make it difficult for clinicians to trust AI-driven treatment recommendations. It is crucial to develop AI algorithms that are more transparent and explainable. Explainable AI (XAI) techniques can be used to provide insights into the decision-making process of AI algorithms. Furthermore, it is important to involve clinicians in the development and validation of AI algorithms to ensure that they are clinically relevant and understandable.
-
Regulatory Hurdles: The use of AI in personalized medicine is subject to regulatory oversight by agencies such as the FDA. It is important to ensure that AI-driven diagnostic and therapeutic tools are safe and effective before they are widely implemented in clinical practice. Clear regulatory guidelines are needed to facilitate the development and deployment of AI in personalized medicine. The development of validation frameworks for AI algorithms is also essential.
-
Clinical Integration: Integrating AI into clinical workflows can be challenging. It is important to ensure that AI-driven tools are user-friendly and seamlessly integrated into existing EHR systems. Furthermore, it is important to provide clinicians with adequate training on how to use AI-driven tools. The successful integration of AI into clinical practice requires collaboration between AI developers, clinicians, and healthcare administrators.
7. Future Directions
The field of AI in personalized medicine is rapidly evolving, with several promising future directions.
-
Multi-Omics Integration: Integrating data from multiple omics platforms, such as genomics, proteomics, metabolomics, and transcriptomics, can provide a more comprehensive understanding of an individual’s health profile. AI algorithms can analyze multi-omics data to identify complex patterns and predict disease risk and treatment response. The development of advanced computational methods for multi-omics data integration is essential for realizing the full potential of personalized medicine.
-
Real-World Data Integration: Integrating data from electronic health records (EHRs), wearable sensors, and social media can provide a more complete picture of a patient’s health. AI algorithms can analyze real-world data to track disease progression, monitor treatment response, and predict patient outcomes. The use of real-world data can improve the accuracy and generalizability of AI models. However, it is important to address the challenges associated with data quality, data standardization, and data privacy when using real-world data.
-
AI-Driven Patient Engagement: AI can be used to engage patients in their own healthcare and empower them to make more informed decisions. AI-powered chatbots and virtual assistants can provide patients with personalized information, answer their questions, and support them in managing their health. AI can also be used to personalize patient education materials and tailor them to individual patient needs. The use of AI to improve patient engagement can lead to better adherence to treatment plans and improved patient outcomes.
-
Edge Computing: Edge computing, which involves processing data closer to the source, can reduce latency and improve the performance of AI algorithms. This is particularly important for applications that require real-time decision-making, such as remote patient monitoring and autonomous surgical robots. The development of edge-optimized AI algorithms is essential for enabling personalized medicine in resource-constrained settings.
-
Quantum Computing: Quantum computing has the potential to revolutionize AI by enabling the development of more powerful and efficient AI algorithms. Quantum computers can solve complex problems that are intractable for classical computers, such as drug discovery and protein folding. The development of quantum AI algorithms is still in its early stages, but it holds immense promise for the future of personalized medicine.
8. Conclusion
AI is transforming personalized medicine by enabling faster, more accurate, and more efficient diagnosis, drug discovery, treatment optimization, and predictive health management. While significant progress has been made, challenges related to data privacy, algorithmic bias, interpretability, and regulatory hurdles need to be addressed to ensure responsible and equitable implementation of AI in clinical practice. The future of personalized medicine is likely to be driven by the integration of multi-omics data, real-world data, and AI-driven patient engagement, leveraging the power of edge computing and, eventually, quantum computing. By addressing these challenges and capitalizing on emerging opportunities, AI has the potential to revolutionize healthcare delivery and improve the lives of millions of patients worldwide.
References
[1] Ardila, D., Kiraly, A. P., Bharwani, A., Choi, B., Reicher, J. J., Peng, L., … & Corrado, G. S. (2019). End-to-end lung cancer screening with three-dimensional deep learning on low-dose chest computed tomography. Nature medicine, 25(6), 954-961.
[2] Escott-Price, V., Beaumont, R., Sharma, K., Kuyken, W., Khandoker, A. H., Lovestone, S., & Ritchie, C. (2019). Identifying novel markers predictive of transition to Alzheimer’s disease in mild cognitive impairment patients using machine learning. Translational Psychiatry, 9(1), 1-11.
[3] Alix-Panabières, C., & Pantel, K. (2016). Clinical utility of circulating tumor cells for personalized medicine. Clinical Chemistry, 62(3), 383-393.
[4] Zitnik, M., Agarwal, M., & Leskovec, J. (2018). Modeling polypharmacy side effects with graph convolutional networks. Bioinformatics, 34(13), i457-i466.
[5] Jiang, F., Jiang, Y., Zhi, H., Dong, Y., Li, H., Ma, S., … & Wang, Y. (2017). Artificial intelligence in healthcare: past, present and future. Stroke and Vascular Neurology, 2(4), 230-243.
[6] Topol, E. J. (2019). High-performance medicine: the convergence of human and artificial intelligence. Nature Medicine, 25(1), 44-56.
Given the emphasis on data privacy, what level of anonymization is sufficient to enable effective AI analysis while safeguarding patient confidentiality, especially when integrating multi-omics and real-world data?