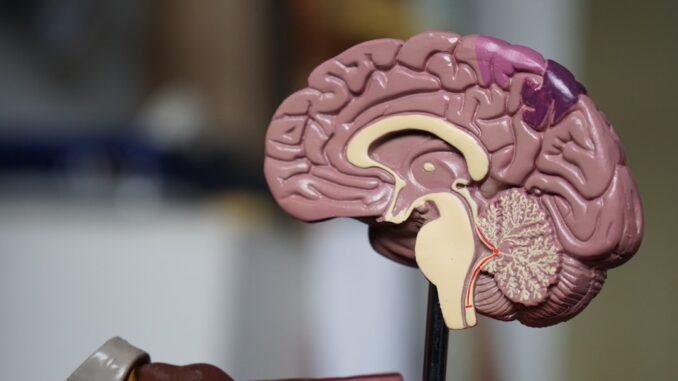
Abstract
Brain-computer interfaces (BCIs) have emerged as a transformative technology with the potential to revolutionize communication, control, and cognitive augmentation for individuals with disabilities and beyond. This research report provides a comprehensive overview of the BCI field, encompassing its historical development, diverse technological approaches (invasive and non-invasive), the current state of research and development, ethical considerations, and potential applications extending beyond assistive communication. The report delves into the challenges and opportunities facing BCI technology, including signal processing limitations, biocompatibility issues, user training requirements, and the complex regulatory landscape. Furthermore, it explores the potential for BCIs to enhance human capabilities, raising critical ethical questions about cognitive enhancement, privacy, and autonomy. Ultimately, this report aims to provide a nuanced understanding of the multifaceted nature of BCI technology and its potential to shape the future of human-machine interaction.
Many thanks to our sponsor Esdebe who helped us prepare this research report.
1. Introduction
Brain-computer interfaces (BCIs) represent a cutting-edge field at the nexus of neuroscience, engineering, and computer science. At its core, a BCI establishes a direct communication pathway between the brain and an external device, bypassing traditional neuromuscular pathways. This paradigm-shifting technology holds immense promise for individuals with severe motor impairments, such as those afflicted by amyotrophic lateral sclerosis (ALS), spinal cord injuries, and stroke. However, the potential applications of BCIs extend far beyond assistive technologies, encompassing areas such as motor rehabilitation, cognitive enhancement, gaming, and even artistic expression.
The initial conception of directly interfacing with the brain can be traced back to the electroencephalography (EEG) experiments of Hans Berger in the 1920s, who first demonstrated the ability to record electrical activity from the human scalp [1]. However, the development of practical BCIs required significant advancements in signal processing, neuroscience, and computer technology. The first implantable BCI was developed in the 1960s by Jose Delgado, who demonstrated the ability to control animal behavior through electrical stimulation of the brain [2]. In the ensuing decades, researchers focused on developing non-invasive BCI systems based on EEG, as well as more invasive approaches involving implanted electrodes. The first successful demonstration of a BCI for communication in humans was achieved in the late 1990s by Philip Kennedy, who implanted electrodes into the motor cortex of a patient with locked-in syndrome, enabling them to control a computer cursor [3].
Today, the field of BCI research is experiencing rapid growth, driven by technological advances and increasing investment from both academic and industry sectors. This report aims to provide a comprehensive overview of the current state of BCI technology, encompassing its history, diverse approaches, ethical implications, potential applications, and regulatory landscape. The report is intended to be accessible to experts in the field while also providing a valuable resource for those seeking a deeper understanding of this rapidly evolving technology.
Many thanks to our sponsor Esdebe who helped us prepare this research report.
2. Types of Brain-Computer Interfaces
BCIs can be broadly categorized based on their invasiveness: invasive, partially invasive, and non-invasive.
2.1 Invasive BCIs
Invasive BCIs involve the implantation of electrodes directly into the brain tissue. This approach offers the highest signal quality and spatial resolution, enabling the decoding of complex neural activity with greater accuracy. However, invasive BCIs also carry significant risks, including infection, tissue damage, and immune response [4].
There are several types of invasive BCIs, including:
- Single-unit recording: This technique involves the implantation of microelectrodes to record the activity of individual neurons. Single-unit recording provides the highest level of detail but is technically challenging and requires precise electrode placement.
- Electrocorticography (ECoG): ECoG involves the placement of electrodes on the surface of the brain, beneath the skull. ECoG offers a compromise between signal quality and invasiveness, providing better signal resolution than EEG while being less invasive than penetrating microelectrode arrays. ECoG is often used in epilepsy monitoring and can be repurposed for BCI applications.
- Local field potential (LFP) recording: LFP recording involves the implantation of electrodes to measure the summed electrical activity of a population of neurons. LFP recording offers a good balance between signal quality and technical complexity.
Invasive BCIs have shown remarkable success in restoring motor function in paralyzed individuals. For example, BrainGate, a neural interface system developed by Cyberkinetics Neurotechnology Systems, has enabled individuals with tetraplegia to control robotic arms and computer cursors with their thoughts [5]. Similarly, researchers at Stanford University have developed a high-performance neural prosthetic system that allows individuals with paralysis to type on a computer at speeds comparable to able-bodied individuals [6].
2.2 Partially Invasive BCIs
Partially invasive BCIs represent a middle ground between invasive and non-invasive approaches. These BCIs typically involve the implantation of devices into the skull, but not directly into the brain tissue. One example of a partially invasive BCI is the electroencephalograph inserted into the epidural space, as opposed to the subdural space of ECoG. The signal quality is lower than direct brain implantation but higher than a non-invasive EEG.
2.3 Non-Invasive BCIs
Non-invasive BCIs utilize sensors placed on the scalp to detect brain activity. The most common non-invasive BCI technique is electroencephalography (EEG), which measures electrical activity through electrodes placed on the scalp [7]. EEG is relatively inexpensive, portable, and non-invasive, making it a widely accessible BCI modality. However, EEG suffers from poor spatial resolution and is susceptible to noise and artifacts, limiting its ability to decode complex neural activity.
Other non-invasive BCI techniques include:
- Magnetoencephalography (MEG): MEG measures magnetic fields produced by electrical activity in the brain. MEG offers better spatial resolution than EEG but is more expensive and requires specialized equipment.
- Functional magnetic resonance imaging (fMRI): fMRI measures brain activity by detecting changes in blood flow. fMRI offers excellent spatial resolution but has poor temporal resolution and is not suitable for real-time BCI applications.
- Near-infrared spectroscopy (NIRS): NIRS measures brain activity by detecting changes in the absorption of near-infrared light. NIRS is relatively inexpensive and portable but has limited spatial resolution.
Non-invasive BCIs have been successfully used for a variety of applications, including assistive communication, motor rehabilitation, and cognitive training. For example, researchers have developed EEG-based BCIs that allow individuals with ALS to control a computer cursor or type on a virtual keyboard [8]. Non-invasive BCIs have also been used to help stroke patients regain motor function by providing feedback based on their brain activity during motor imagery tasks [9].
2.4 Modality Comparison and Future Trends
The choice of BCI modality depends on the specific application and the trade-off between signal quality, invasiveness, and cost. Invasive BCIs offer the highest signal quality but carry significant risks. Non-invasive BCIs are safer and more accessible but suffer from limited signal resolution. Partially invasive methods are trying to achieve a tradeoff.
Future trends in BCI technology include the development of more advanced signal processing algorithms, miniaturization of electrodes, and the integration of BCIs with artificial intelligence (AI). Researchers are also exploring new BCI modalities, such as optogenetics and ultrasound, which could offer improved spatial and temporal resolution [10].
Many thanks to our sponsor Esdebe who helped us prepare this research report.
3. Signal Processing and Machine Learning in BCIs
The core challenge in BCI technology lies in accurately decoding neural signals and translating them into control commands. This requires sophisticated signal processing and machine learning algorithms to extract relevant features from the raw neural data and classify them into distinct intentions or actions [11].
3.1 Preprocessing and Feature Extraction
The raw neural signals acquired by BCI devices are often noisy and contain artifacts, such as muscle activity, eye movements, and electrical interference. Therefore, preprocessing steps are essential to clean the data and remove unwanted noise. Common preprocessing techniques include filtering, artifact removal, and baseline correction.
Once the data has been preprocessed, feature extraction techniques are used to identify relevant characteristics of the neural signals that can be used to discriminate between different intentions or actions. Common feature extraction methods include:
- Time-domain features: These features capture the amplitude and timing of the neural signals, such as mean amplitude, variance, and zero-crossing rate.
- Frequency-domain features: These features capture the frequency content of the neural signals, such as power spectral density (PSD) and band power.
- Time-frequency features: These features capture both the time and frequency characteristics of the neural signals, such as wavelets and spectrograms.
- Spatial features: These features capture the spatial distribution of neural activity across the brain, such as common spatial patterns (CSP) and source localization techniques.
The choice of feature extraction method depends on the specific BCI modality and the type of neural signals being analyzed. For example, EEG-based BCIs often rely on frequency-domain features to detect changes in brain rhythms associated with different cognitive states.
3.2 Machine Learning Algorithms
After feature extraction, machine learning algorithms are used to classify the extracted features into distinct intentions or actions. Common machine learning algorithms used in BCIs include:
- Linear discriminant analysis (LDA): LDA is a simple and efficient linear classifier that is widely used in BCI research.
- Support vector machines (SVMs): SVMs are powerful non-linear classifiers that can handle high-dimensional data and complex decision boundaries.
- Artificial neural networks (ANNs): ANNs are complex non-linear models that can learn intricate patterns from data. Deep learning, a subset of ANNs with multiple layers, has shown promising results in BCI applications.
- Hidden Markov models (HMMs): HMMs are statistical models that can capture the temporal dynamics of neural signals. HMMs are often used in BCIs for continuous control tasks.
The performance of machine learning algorithms in BCIs depends on the quality of the training data, the choice of features, and the algorithm parameters. It is often necessary to optimize these parameters to achieve the best performance. In practice, the performance of the algorithm will be strongly affected by the individual variance of the data. Data taken from a single patient will differ dramatically from patient to patient. Therefore there is an ongoing requirement to create new algorithms that have the ability to work well with relatively small patient datasets, but also generalise over different individuals.
3.3 Adaptive Learning and Transfer Learning
BCI performance can vary significantly over time due to changes in brain activity and electrode impedance. To address this issue, adaptive learning techniques are used to continuously update the BCI model based on new data. Adaptive learning can improve BCI performance and reduce the need for retraining.
Another challenge in BCI research is the inter-subject variability in brain activity. To address this issue, transfer learning techniques are used to transfer knowledge from one subject to another. Transfer learning can reduce the amount of training data required for new subjects and improve BCI performance.
3.4 Challenges and Future Directions
Despite significant progress in signal processing and machine learning, several challenges remain in BCI research. These include:
- Improving signal quality: Reducing noise and artifacts in neural signals is crucial for improving BCI performance.
- Developing more robust and adaptive algorithms: BCI algorithms need to be more robust to variations in brain activity and electrode impedance.
- Reducing the need for training data: Developing algorithms that can learn from limited training data is essential for making BCIs more accessible.
- Addressing inter-subject variability: Developing algorithms that can generalize across different subjects is crucial for translating BCI technology into clinical practice.
Future directions in signal processing and machine learning for BCIs include the development of more advanced deep learning architectures, the integration of multimodal data sources, and the use of personalized models that are tailored to the individual user.
Many thanks to our sponsor Esdebe who helped us prepare this research report.
4. Applications of Brain-Computer Interfaces
The applications of BCIs are diverse and span a wide range of domains, including assistive technology, motor rehabilitation, cognitive enhancement, and entertainment.
4.1 Assistive Technology
The most well-established application of BCIs is in assistive technology for individuals with severe motor impairments. BCIs can provide a communication and control pathway for individuals who have lost the ability to move or speak due to conditions such as ALS, spinal cord injury, and stroke.
BCIs can be used for a variety of assistive tasks, including:
- Communication: BCIs can be used to control a computer cursor or type on a virtual keyboard, allowing individuals to communicate with others. For example, P300-based BCIs have been used to develop spelling devices that enable individuals with locked-in syndrome to communicate by selecting letters on a screen [12].
- Environmental control: BCIs can be used to control home appliances, such as lights, thermostats, and televisions, providing individuals with greater independence.
- Mobility: BCIs can be used to control wheelchairs and robotic arms, allowing individuals to move around and interact with their environment. For example, researchers have developed BCIs that allow individuals with tetraplegia to control a robotic arm to grasp and manipulate objects [13].
4.2 Motor Rehabilitation
BCIs are also being explored as a tool for motor rehabilitation after stroke or spinal cord injury. BCIs can provide feedback based on a patient’s brain activity during motor imagery tasks, helping them to regain motor function. This approach, known as BCI-mediated motor rehabilitation, has shown promising results in clinical trials [14].
BCI-mediated motor rehabilitation typically involves the following steps:
- The patient attempts to perform a motor task, such as moving their hand or foot.
- The BCI detects the patient’s brain activity associated with the motor imagery.
- The BCI provides feedback to the patient, such as visual or auditory cues, indicating whether their brain activity is consistent with the desired motor task.
- The patient adjusts their brain activity based on the feedback, gradually improving their ability to perform the motor task.
4.3 Cognitive Enhancement
Beyond assistive technology and motor rehabilitation, BCIs are also being explored for cognitive enhancement. BCIs can be used to monitor and modulate brain activity, potentially improving cognitive functions such as attention, memory, and learning. This application raises ethical concerns about the potential for unfair advantages and the risks of unintended consequences.
Some potential applications of BCIs for cognitive enhancement include:
- Attention training: BCIs can be used to provide feedback based on a person’s attention level, helping them to improve their focus and concentration.
- Memory enhancement: BCIs can be used to stimulate brain regions involved in memory, potentially improving memory performance.
- Neurofeedback: BCIs can be used to train individuals to self-regulate their brain activity, potentially improving cognitive and emotional well-being [15].
4.4 Entertainment and Gaming
BCIs are also being explored for entertainment and gaming applications. BCIs can be used to control video games, create immersive virtual reality experiences, and even generate music and art. These applications are still in their early stages, but they hold the potential to revolutionize the way we interact with technology and express ourselves creatively.
4.5 Future Applications
The potential applications of BCIs are vast and continue to expand as the technology advances. Some potential future applications include:
- Brain-to-brain communication: BCIs could be used to directly transmit thoughts and emotions between individuals, potentially revolutionizing communication.
- Brain-machine symbiosis: BCIs could be used to create a seamless integration between the human brain and external devices, blurring the lines between human and machine.
- Treatment of neurological and psychiatric disorders: BCIs could be used to treat a wide range of neurological and psychiatric disorders, such as depression, anxiety, and epilepsy.
Many thanks to our sponsor Esdebe who helped us prepare this research report.
5. Ethical Considerations
The development and application of BCIs raise a number of ethical considerations that must be carefully addressed to ensure the responsible and beneficial use of this technology [16].
5.1 Privacy and Security
BCIs have the potential to access and record sensitive information about a person’s thoughts, emotions, and intentions. This raises concerns about the privacy and security of this information and the potential for misuse. Safeguards must be implemented to protect BCI data from unauthorized access and ensure that individuals have control over their own neural data. The concept of neural data being used against someone is particularly chilling and goes far beyond most modern data concerns.
5.2 Autonomy and Agency
BCIs can potentially influence a person’s thoughts and actions, raising concerns about autonomy and agency. It is important to ensure that individuals retain control over their own minds and bodies and that BCIs are not used to manipulate or control them. The implications for BCI control of machinery such as weapons would be extreme. The need for stringent control of access is paramount.
5.3 Cognitive Enhancement and Inequality
The use of BCIs for cognitive enhancement raises concerns about fairness and equality. If BCIs are used to enhance cognitive abilities, it could create a divide between those who have access to this technology and those who do not, leading to social and economic inequality.
5.4 Responsibility and Liability
If a person using a BCI causes harm to themselves or others, it raises questions about responsibility and liability. Who is responsible for the actions of a person using a BCI? Is it the person using the BCI, the BCI manufacturer, or the BCI programmer?
5.5 Informed Consent and Dual Use
It is essential to ensure that individuals provide informed consent before using a BCI. This means that they must be fully aware of the risks and benefits of the technology and have the right to refuse its use. Additionally, there is concern about the potential for BCIs to be used for military or surveillance purposes, raising ethical questions about dual use.
5.6 Neuroethics and Policy
Addressing the ethical challenges posed by BCIs requires a multidisciplinary approach involving neuroscientists, ethicists, policymakers, and the public. Neuroethics is an emerging field that focuses on the ethical implications of neuroscience research and technology. Policy frameworks are needed to regulate the development and use of BCIs and to ensure that they are used in a responsible and ethical manner.
Many thanks to our sponsor Esdebe who helped us prepare this research report.
6. Regulatory Landscape
The regulatory landscape for BCI devices is still evolving, with different countries and regions taking different approaches. In general, BCI devices are classified as medical devices and are subject to regulatory oversight by agencies such as the Food and Drug Administration (FDA) in the United States and the European Medicines Agency (EMA) in Europe [17].
The regulatory requirements for BCI devices vary depending on the level of risk associated with the device. Invasive BCIs, which pose a higher risk to the user, are typically subject to more stringent regulatory requirements than non-invasive BCIs. The regulators are grappling with the complexity and potential risks of these devices, resulting in a cautious approach.
Some of the key regulatory considerations for BCI devices include:
- Safety: BCI devices must be safe for human use and must not pose an unreasonable risk of harm.
- Effectiveness: BCI devices must be effective for their intended purpose and must provide a clinically meaningful benefit to the user.
- Labeling: BCI devices must be properly labeled with clear and accurate information about their use and potential risks.
- Clinical trials: BCI devices may require clinical trials to demonstrate their safety and effectiveness before they can be marketed.
The regulatory landscape for BCIs is likely to become more complex as the technology advances and new applications emerge. It is important for researchers, developers, and policymakers to work together to develop clear and consistent regulatory standards that promote innovation while ensuring the safety and ethical use of BCI technology. A key challenge will be creating agile and responsive regulation that balances innovation with safety.
Many thanks to our sponsor Esdebe who helped us prepare this research report.
7. Future Directions and Conclusion
Brain-computer interfaces hold immense promise for transforming healthcare, human-machine interaction, and our understanding of the brain. While significant progress has been made in recent years, several challenges remain before BCIs can be widely adopted.
Future research directions include:
- Developing more advanced signal processing algorithms: Improving the accuracy and robustness of BCI signal processing is crucial for enhancing performance.
- Creating more biocompatible and durable electrodes: Long-term biocompatibility and durability of implanted electrodes are essential for invasive BCIs.
- Developing closed-loop BCIs: Closed-loop BCIs, which provide feedback to the brain based on its activity, hold the potential to improve learning and adaptation.
- Exploring new BCI modalities: Researching alternative BCI modalities, such as optogenetics and ultrasound, could offer improved spatial and temporal resolution.
- Addressing ethical and societal implications: Open discussions and policy development are needed to address the ethical, legal, and social implications of BCI technology.
In conclusion, brain-computer interfaces represent a rapidly evolving field with the potential to revolutionize numerous aspects of human life. By addressing the technological, ethical, and regulatory challenges, we can harness the power of BCIs to improve the lives of individuals with disabilities, enhance human capabilities, and unlock new frontiers in neuroscience.
Many thanks to our sponsor Esdebe who helped us prepare this research report.
References
[1] Berger, H. (1929). Über das Elektrenkephalogramm des Menschen. Archiv für Psychiatrie und Nervenkrankheiten, 87(1), 527-570.
[2] Delgado, J. M. R. (1969). Physical control of the mind: Toward a psychocivilized society. Harper & Row.
[3] Kennedy, P. R., Bakay, R. A. E., Moore, M. M., Adams, K., & Goldwaithe, J. (2000). Direct control of a computer using human central neurons. IEEE Transactions on Rehabilitation Engineering, 8(2), 198-202.
[4] Kozai, T. D. Y., Langhals, N. B., Patel, P. R., Deng, X., Zhang, H., Smith, K. L., … & Cui, X. T. (2015). Chronic multi-electrode array implantation results in progressive and sustained blood-brain barrier damage, neuronal degeneration and glial scarring. Journal of Neuroscience, 35(46), 14948-14959.
[5] Hochberg, L. R., Serruya, M. D., Friehs, G. M., Mukand, J. A., Saleh, M., Caplan, A. H., … & Donoghue, J. P. (2006). Neuronal ensemble control of prosthetic devices by a human with tetraplegia. Nature, 442(7099), 164-171.
[6] Willett, F. R., Kunz, E. M., Fan, C., Fatherree, J. P., Gilja, V., Druckmann, S., … & Shenoy, K. V. (2021). High-performance brain-to-text communication via imagined handwriting. Nature, 593(7858), 249-254.
[7] Nunez, P. L., & Srinivasan, R. (2006). Electric fields of the brain: The neurophysics of EEG. Oxford University Press.
[8] Kübler, A., Nijboer, F., Mellinger, J., Vaughan, T. M., Pawelzik, H., Schalk, G., & Birbaumer, N. (2005). Patients with ALS can use sensorimotor rhythms to operate a brain-computer interface. Neurology, 64(10), 1775-1777.
[9] Ramos-Murguialday, A., Broetz, D., Rea, M., Läer, L., Diederichsen, G., Calvetti, A., … & Birbaumer, N. (2013). Brain-machine interface in chronic stroke rehabilitation: a controlled study. Annals of Neurology, 74(1), 100-108.
[10] Alivisatos, A. P., Chun, M., Church, G. M., Greenspan, R. J., Roukes, M. L., Yuste, R., & Zimmer, M. (2013). The brain activity map project and the challenge of non-invasive optical imaging of neural activity. Annals of the New York Academy of Sciences, 1291(1), 1-7.
[11] Lotte, F., Bougrain, L., Cichocki, A., Clerc, M., Congedo, A., Rakotomamonjy, A., & Yger, F. (2018). A review of classification algorithms for EEG-based brain-computer interfaces: towards using more complex models. Journal of Neural Engineering, 15(3), 031005.
[12] Farwell, L. A., & Donchin, E. (1988). Talking off the top of your head: toward a mental prosthesis utilizing event-related brain potentials. Electroencephalography and Clinical Neurophysiology, 70(6), 510-523.
[13] Collinger, J. L., Boninger, M. L., Bruns, T. M., Curley, K., Weber, D. J., Wang, W., … & Schwartz, A. B. (2013). High-performance neuroprosthetic control by an individual with tetraplegia. Journal of Neural Engineering, 10(2), 026011.
[14] Biasiucci, A., Leeb, R., Iturrate, I., del R. Grosse-Wentrup, G., Kübler, A., Mattia, D., & Müller, K. R. (2018). An introduction to brain computer interface. European Journal of Translational Myology, 28(4).
[15] Gruzelier, J. H. (2014). EEG-neurofeedback for optimising performance. II: potential clinical applications. Neuroscience & Biobehavioral Reviews, 38, 52-62.
[16] Yuste, R., Genser, J., & Hermann, C. (2017). It’s time for neuro-rights. Nature, 551(7679), 159-161.
[17] Committee on the Ethical and Legal Implications of the Use of Advanced Neural Technologies, National Academies of Sciences, Engineering, and Medicine. (2020). Ethical and societal implications of brain computer interface technology. National Academies Press.
The report mentions the potential for BCIs to access sensitive neural data. Considering the evolving landscape of data privacy regulations like GDPR, how might these frameworks need to adapt to address the unique challenges posed by direct brain data access and potential misuse?
That’s a crucial point! The direct access to neural data certainly presents unique challenges for GDPR and similar frameworks. We might see adaptations focusing on stricter consent protocols, novel anonymization techniques specifically for neural data, and perhaps even new classifications of data sensitivity. Thanks for highlighting this!
Editor: MedTechNews.Uk
Thank you to our Sponsor Esdebe