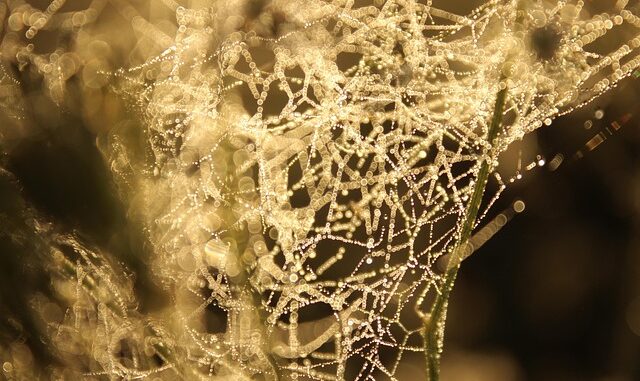
Abstract
Neural interfaces (NIs) represent a rapidly evolving field with the potential to revolutionize our understanding and treatment of neurological disorders, restore lost motor and sensory function, and even augment human capabilities. This review provides a comprehensive overview of NI technologies, encompassing both invasive and non-invasive approaches, signal processing methodologies, biocompatibility challenges, ethical considerations, and emerging applications. We explore the current state-of-the-art, highlighting key advancements in electrode design, decoding algorithms, and closed-loop systems. Furthermore, we critically assess the limitations of existing technologies and discuss promising avenues for future research, including the integration of artificial intelligence, advanced materials, and personalized therapeutic strategies. We argue that a multidisciplinary approach, combining expertise from neuroscience, engineering, materials science, and ethics, is essential to realize the full potential of NIs and ensure their responsible development and deployment.
Many thanks to our sponsor Esdebe who helped us prepare this research report.
1. Introduction
The human nervous system, a complex and intricate network, controls every aspect of our physical and cognitive functions. Disruptions to this network, caused by injury, disease, or congenital conditions, can lead to a wide range of debilitating disorders. Neural interfaces (NIs) offer a direct pathway to interact with the nervous system, holding the promise of restoring lost function, treating neurological diseases, and even enhancing human capabilities. These devices, which can be implanted or applied non-invasively, enable bidirectional communication between the brain and external devices, allowing for the recording and modulation of neural activity.
The field of NIs has witnessed significant progress in recent decades, driven by advances in microfabrication, materials science, signal processing, and machine learning. Early applications focused primarily on motor prosthetics, such as controlling robotic arms with brain signals. However, the scope of NI research has expanded considerably, encompassing a wide range of applications, including sensory restoration (e.g., cochlear implants, retinal prostheses), treatment of neurological disorders (e.g., deep brain stimulation for Parkinson’s disease, epilepsy), and even cognitive enhancement (e.g., memory prostheses). Despite these advancements, significant challenges remain in terms of biocompatibility, long-term stability, signal quality, and ethical considerations. This review aims to provide a comprehensive overview of the current state-of-the-art in NI technology, highlighting key advancements, challenges, and future directions.
Many thanks to our sponsor Esdebe who helped us prepare this research report.
2. Types of Neural Interfaces
NIs can be broadly classified into two categories: invasive and non-invasive, depending on whether they require surgical implantation. The choice of interface type depends on the specific application, the desired signal quality, and the risk-benefit profile for the patient.
2.1 Invasive Neural Interfaces
Invasive NIs involve direct contact with the nervous system, providing high-resolution recordings and precise stimulation. These interfaces typically consist of microelectrode arrays (MEAs) or single electrodes implanted into the brain or peripheral nerves.
- Microelectrode Arrays (MEAs): MEAs are composed of multiple microelectrodes arranged in a grid or other pattern. They can be used to record from and stimulate a large number of neurons simultaneously. Various types of MEAs exist, including silicon-based arrays, flexible polymer arrays, and microwire arrays. Silicon-based arrays, such as the Utah array [1], offer high spatial resolution and are widely used for cortical recordings. Flexible polymer arrays, such as those made from polyimide or parylene, are more biocompatible and can conform to the curvature of the brain, reducing tissue damage. Microwire arrays, composed of bundles of thin wires, are relatively easy to implant but may suffer from lower signal quality.
- Single Electrodes: Single electrodes are used to record from or stimulate individual neurons or small groups of neurons. They are often used for deep brain stimulation (DBS) [2], a therapy for Parkinson’s disease, essential tremor, and other neurological disorders. DBS involves implanting electrodes into specific brain regions, such as the subthalamic nucleus or globus pallidus, and delivering electrical stimulation to modulate neural activity.
Challenges of Invasive NIs: While invasive NIs offer high signal quality, they also pose significant challenges. Biocompatibility is a major concern, as the implanted device can trigger an immune response, leading to inflammation, glial scarring, and eventual degradation of the signal. Long-term stability is also a challenge, as the electrode-tissue interface can change over time, resulting in signal drift or loss. Furthermore, surgical implantation carries risks of infection, bleeding, and neurological damage. Strategies to address these challenges include developing biocompatible materials, using minimally invasive implantation techniques, and optimizing electrode design to minimize tissue damage.
2.2 Non-Invasive Neural Interfaces
Non-invasive NIs do not require surgical implantation, making them a safer and more accessible option for many applications. These interfaces typically rely on measuring electrical or magnetic activity from the scalp using techniques such as electroencephalography (EEG) or magnetoencephalography (MEG).
- Electroencephalography (EEG): EEG measures electrical activity from the scalp using electrodes placed on the head. It is a relatively inexpensive and widely available technique. EEG has been used for a variety of applications, including brain-computer interfaces (BCIs) [3], sleep monitoring, and seizure detection. However, EEG has relatively low spatial resolution due to the blurring effect of the skull and scalp. Furthermore, EEG signals are susceptible to noise from muscle activity and other sources.
- Magnetoencephalography (MEG): MEG measures magnetic fields produced by electrical activity in the brain. It offers better spatial resolution than EEG and is less susceptible to noise from muscle activity. However, MEG is more expensive and less widely available than EEG. MEG has been used for research on cognitive processes, such as language and memory, and for clinical applications, such as epilepsy diagnosis.
- Transcranial Magnetic Stimulation (TMS): TMS is a non-invasive technique that uses magnetic pulses to stimulate or inhibit neural activity in specific brain regions [4]. It can be used for both research and therapeutic purposes. TMS has been used to study cognitive functions, such as motor control and language, and to treat neurological and psychiatric disorders, such as depression and stroke.
- Functional Near-Infrared Spectroscopy (fNIRS): fNIRS measures changes in blood flow in the brain using near-infrared light. It is a non-invasive and relatively inexpensive technique that can be used to monitor brain activity during various tasks. fNIRS has been used for research on cognitive processes, such as attention and memory, and for clinical applications, such as stroke rehabilitation.
Challenges of Non-Invasive NIs: While non-invasive NIs are safer and more accessible than invasive NIs, they also have limitations. The spatial resolution is typically lower than that of invasive NIs, making it difficult to target specific brain regions. The signal quality can also be affected by noise from muscle activity, environmental interference, and individual differences in skull thickness and scalp conductivity. Furthermore, non-invasive stimulation techniques, such as TMS, can only modulate activity in superficial brain regions. Strategies to address these challenges include developing advanced signal processing algorithms, using multi-modal imaging techniques, and optimizing stimulation parameters.
Many thanks to our sponsor Esdebe who helped us prepare this research report.
3. Technologies for Recording and Decoding Neural Signals
The success of NIs relies on the ability to accurately record and decode neural signals. This involves a combination of hardware and software technologies, including signal acquisition systems, signal processing algorithms, and machine learning models.
3.1 Signal Acquisition Systems
Signal acquisition systems are responsible for capturing and amplifying neural signals from the electrodes. These systems typically consist of preamplifiers, amplifiers, filters, and analog-to-digital converters (ADCs). The performance of the signal acquisition system is crucial for obtaining high-quality neural recordings. Key parameters include the noise level, bandwidth, and sampling rate. Low-noise amplifiers are essential for capturing weak neural signals. The bandwidth should be wide enough to capture the relevant frequencies of neural activity. The sampling rate should be high enough to avoid aliasing, which can distort the signal.
3.2 Signal Processing Algorithms
Signal processing algorithms are used to extract relevant information from the raw neural signals. These algorithms can be used to remove noise, filter out unwanted frequencies, and identify specific patterns of neural activity. Common signal processing techniques include filtering, artifact removal, feature extraction, and dimensionality reduction.
- Filtering: Filtering is used to remove noise and unwanted frequencies from the neural signals. Common types of filters include low-pass filters, high-pass filters, and band-pass filters. Low-pass filters attenuate high-frequency noise, such as muscle activity. High-pass filters attenuate low-frequency noise, such as baseline drift. Band-pass filters isolate specific frequency bands, such as the alpha band (8-12 Hz) or the beta band (13-30 Hz).
- Artifact Removal: Artifacts are unwanted signals that can contaminate neural recordings. Common sources of artifacts include muscle activity, eye blinks, and power line interference. Artifact removal techniques include independent component analysis (ICA) and wavelet decomposition. ICA separates the neural signals into independent components, allowing for the identification and removal of artifact components. Wavelet decomposition decomposes the neural signals into different frequency bands, allowing for the removal of artifact components in specific frequency bands.
- Feature Extraction: Feature extraction is used to identify relevant features from the neural signals. These features can be used to classify different brain states or to control external devices. Common features include amplitude, frequency, and phase. Time-frequency analysis techniques, such as the short-time Fourier transform (STFT) and wavelet transform, can be used to extract features from non-stationary neural signals.
- Dimensionality Reduction: Dimensionality reduction techniques are used to reduce the number of features, making the data easier to analyze and classify. Common dimensionality reduction techniques include principal component analysis (PCA) and linear discriminant analysis (LDA). PCA identifies the principal components of the data, which are the directions of maximum variance. LDA finds the linear combination of features that best separates different classes of data.
3.3 Machine Learning Models
Machine learning models are used to decode neural signals and translate them into control signals for external devices. These models can be trained to recognize specific patterns of neural activity associated with different intentions or actions. Common machine learning algorithms include linear classifiers, support vector machines (SVMs), and neural networks.
- Linear Classifiers: Linear classifiers are simple and efficient algorithms that can be used to classify neural signals based on a linear combination of features. Common linear classifiers include linear discriminant analysis (LDA) and logistic regression.
- Support Vector Machines (SVMs): SVMs are powerful algorithms that can be used to classify neural signals based on a non-linear mapping of features into a high-dimensional space. SVMs are particularly well-suited for dealing with high-dimensional data and non-linear relationships.
- Neural Networks: Neural networks are complex algorithms that can learn non-linear relationships between neural signals and desired outputs. Neural networks have been used for a variety of BCI applications, including motor imagery decoding, speech decoding, and emotion recognition. Deep learning, a type of neural network with multiple layers, has shown promising results in recent years for decoding complex neural patterns.
Many thanks to our sponsor Esdebe who helped us prepare this research report.
4. Biocompatibility and Long-Term Stability
Biocompatibility and long-term stability are critical challenges for invasive NIs. The implantation of a foreign object into the brain triggers an immune response, leading to inflammation, glial scarring, and eventual degradation of the signal. Strategies to address these challenges include developing biocompatible materials, using minimally invasive implantation techniques, and optimizing electrode design to minimize tissue damage.
4.1 Biocompatible Materials
The choice of materials for NIs is crucial for minimizing the immune response and promoting long-term stability. Ideal materials should be biocompatible, mechanically flexible, and electrically conductive. Common materials used for NIs include silicon, platinum, gold, and polymers. Silicon is a widely used material for microfabrication, but it is relatively rigid and can cause tissue damage. Platinum and gold are highly conductive and corrosion-resistant, but they are also relatively rigid. Polymers, such as polyimide and parylene, are more biocompatible and flexible than silicon or metals, but they are less conductive. Research is ongoing to develop new materials that combine the advantages of different materials, such as conductive polymers, carbon nanotubes, and graphene.
4.2 Minimally Invasive Implantation Techniques
Minimally invasive implantation techniques can reduce tissue damage and inflammation. These techniques involve using smaller incisions and more precise surgical tools. Stereotactic surgery, a technique that uses a three-dimensional coordinate system to guide the placement of electrodes, is commonly used for DBS. Robotic surgery offers even greater precision and control. Emerging techniques, such as injectable electronics, involve injecting flexible electronic devices into the brain using a syringe. These devices can conform to the curvature of the brain and minimize tissue damage.
4.3 Electrode Design
Electrode design plays a crucial role in minimizing tissue damage and promoting long-term stability. Factors to consider include the size, shape, and spacing of the electrodes. Smaller electrodes cause less tissue damage. Flexible electrodes can conform to the curvature of the brain and reduce stress on the tissue. Optimizing the spacing between electrodes can improve signal quality and reduce interference. Surface modifications, such as coatings with biocompatible materials or growth factors, can promote neuronal adhesion and reduce glial scarring.
Many thanks to our sponsor Esdebe who helped us prepare this research report.
5. Ethical Considerations
The development and application of NIs raise a number of ethical considerations. These include issues related to privacy, security, autonomy, and equity. It is essential to address these ethical concerns proactively to ensure that NIs are used responsibly and for the benefit of society.
5.1 Privacy and Security
NIs can record and transmit sensitive information about a person’s brain activity. This information could be used to infer their thoughts, emotions, and intentions. It is crucial to protect this information from unauthorized access and misuse. Security measures, such as encryption and authentication, should be implemented to prevent hacking and data breaches. Privacy policies should be developed to ensure that individuals have control over their neural data and how it is used.
5.2 Autonomy
NIs can be used to influence a person’s thoughts, emotions, and behavior. This raises concerns about autonomy and the potential for manipulation. It is essential to ensure that individuals have the right to control their own brain activity and to make their own decisions. Informed consent is crucial for any NI application that could affect a person’s autonomy.
5.3 Equity
NIs are currently expensive and not widely available. This raises concerns about equity and access to these technologies. It is important to ensure that NIs are available to all who could benefit from them, regardless of their socioeconomic status. Public funding and research initiatives can help to reduce the cost of NIs and make them more accessible.
5.4 Enhancement
The potential for NIs to enhance human capabilities raises ethical questions about fairness and societal implications. If NIs become available for cognitive or physical enhancement, it could create a divide between those who have access to these technologies and those who do not. This could exacerbate existing inequalities and create new forms of discrimination. It is important to have a public discussion about the ethical implications of enhancement technologies and to develop policies that ensure fairness and equity.
Many thanks to our sponsor Esdebe who helped us prepare this research report.
6. Emerging Applications
Beyond motor prosthetics, NIs are being explored for a wide range of emerging applications, including sensory restoration, treatment of neurological disorders, and cognitive enhancement.
6.1 Sensory Restoration
NIs can be used to restore sensory function in individuals who have lost their sight, hearing, or touch. Cochlear implants are a well-established example of a successful NI for restoring hearing. Retinal prostheses, which stimulate the retina to restore vision, are also under development. Researchers are also exploring the use of NIs to restore touch sensation in individuals with spinal cord injuries or amputations.
6.2 Treatment of Neurological Disorders
DBS is a widely used therapy for Parkinson’s disease, essential tremor, and other neurological disorders. NIs are also being explored for the treatment of epilepsy, depression, and obsessive-compulsive disorder (OCD). Closed-loop systems, which automatically adjust stimulation parameters based on neural activity, are being developed to improve the efficacy of DBS and other NI therapies. Research is also underway to develop NIs that can deliver drugs directly to the brain, bypassing the blood-brain barrier.
6.3 Cognitive Enhancement
NIs are being explored for cognitive enhancement, such as improving memory, attention, and decision-making. Memory prostheses, which stimulate the hippocampus to enhance memory function, are under development. NIs are also being explored for improving attention and focus in individuals with ADHD. The ethical implications of cognitive enhancement technologies are a subject of ongoing debate.
6.4 Brain-Computer Interfaces for Communication
Brain-computer interfaces (BCIs) can provide a communication pathway for individuals with severe paralysis who are unable to speak or move. BCIs can translate brain signals into commands that control a computer cursor, a robotic arm, or a speech synthesizer. Recent advances in BCI technology have enabled individuals with paralysis to communicate more quickly and effectively.
Many thanks to our sponsor Esdebe who helped us prepare this research report.
7. Future Directions
The field of NIs is rapidly evolving, with significant advances expected in the coming years. Promising avenues for future research include the integration of artificial intelligence, advanced materials, and personalized therapeutic strategies.
7.1 Artificial Intelligence
Artificial intelligence (AI) is playing an increasingly important role in NI research. AI algorithms can be used to decode neural signals more accurately and to develop more sophisticated closed-loop systems. Machine learning models can be trained to personalize NI therapies based on individual brain activity patterns. AI can also be used to develop new types of BCIs that are more intuitive and user-friendly.
7.2 Advanced Materials
The development of advanced materials is crucial for improving the biocompatibility and long-term stability of NIs. New materials, such as conductive polymers, carbon nanotubes, and graphene, offer the potential for creating more flexible, durable, and biocompatible electrodes. Nanomaterials can be used to enhance the sensitivity of electrodes and to deliver drugs directly to the brain. Research is also underway to develop biodegradable materials that can be used to create temporary NIs that dissolve after use.
7.3 Personalized Therapeutic Strategies
Personalized therapeutic strategies are essential for maximizing the efficacy of NIs. Individual brain activity patterns vary significantly, and NI therapies need to be tailored to each individual’s specific needs. Closed-loop systems that automatically adjust stimulation parameters based on neural activity can provide personalized therapy in real-time. Genetic information and other biomarkers can also be used to predict an individual’s response to NI therapy and to personalize treatment accordingly.
7.4 Wireless and Minimally Invasive NIs
The development of wireless and minimally invasive NIs is a major goal of NI research. Wireless NIs eliminate the need for external cables, making them more convenient and comfortable for users. Minimally invasive NIs reduce tissue damage and inflammation. Researchers are exploring a variety of techniques for developing wireless and minimally invasive NIs, including injectable electronics, optogenetics, and ultrasound stimulation.
Many thanks to our sponsor Esdebe who helped us prepare this research report.
8. Conclusion
Neural interfaces hold immense promise for restoring lost function, treating neurological disorders, and enhancing human capabilities. Significant progress has been made in recent decades in the development of NI technologies, but significant challenges remain. Biocompatibility, long-term stability, signal quality, and ethical considerations are all critical issues that need to be addressed. A multidisciplinary approach, combining expertise from neuroscience, engineering, materials science, and ethics, is essential to realize the full potential of NIs and ensure their responsible development and deployment. The integration of artificial intelligence, advanced materials, and personalized therapeutic strategies will be crucial for advancing the field and making NIs more effective, safe, and accessible. As NI technology continues to evolve, it is important to have a public dialogue about the ethical implications of these technologies and to develop policies that ensure fairness, equity, and individual autonomy.
Many thanks to our sponsor Esdebe who helped us prepare this research report.
References
[1] Normann, R. A., et al. “A neural interface for a cortical vision prosthesis.” Vision research 39.15 (1999): 2577-2587.
[2] Lozano, A. M., et al. “Deep brain stimulation: current challenges and future directions.” Nature Reviews Neurology 15.3 (2019): 148-160.
[3] Wolpaw, J. R., et al. “Brain-computer interfaces for communication and control.” Clinical neurophysiology 113.6 (2002): 767-791.
[4] Hallett, M. “Transcranial magnetic stimulation: a primer.” Neuron 62.5 (2009): 619-625.
[5] Hochberg, L. R., et al. “Neuronal ensemble control of prosthetic devices by a human with tetraplegia.” Nature 442.7099 (2006): 164-171.
[6] Donoghue, J. P. “Bridging the brain to the world: a perspective on neural interface systems.” Neuron 60.1 (2008): 1-16.
[7] Nicolelis, M. A. L. “Brain-machine interfaces to restore motor function and probe brain plasticity.” Nature Reviews Neuroscience 4.5 (2003): 417-422.
[8] Rao, R. P. N. “Brain-computer interfacing: a survey of principles and applications.” IEEE transactions on neural systems and rehabilitation engineering 11.2 (2003): 13-22.
[9] Lebedev, M. A., and Nicolelis, M. A. L. “Brain-machine interfaces: past, present and future.” Trends in neurosciences 29.9 (2006): 536-546.
[10] Moritz, C. T., et al. “Long-term stability of intracortical microstimulation-mediated motor behavior.” Nature neuroscience 8.12 (2005): 1752-1758.
So, we’re just casually hacking the nervous system now? I wonder if sponsor Esdebe also covers the cost of therapy for the existential dread this tech is bound to induce.
That’s a great point! The psychological impact and access to mental healthcare are definitely crucial aspects we need to consider alongside technological advancements in neural interfaces. How do we ensure responsible development that prioritizes user well-being and addresses potential anxieties related to this tech?
Editor: MedTechNews.Uk
Thank you to our Sponsor Esdebe
The review highlights the potential of AI in personalizing NI therapies. How might we develop robust AI algorithms that account for the inherent variability and complexity of individual brain activity over extended periods to ensure sustained therapeutic efficacy?
That’s a fantastic question! It gets to the heart of the challenges of long-term AI application in neural interfaces. Perhaps incorporating dynamic Bayesian networks or reinforcement learning could allow algorithms to adapt to individual neural drift over time, continuously refining therapeutic approaches. What are your thoughts?
Editor: MedTechNews.Uk
Thank you to our Sponsor Esdebe