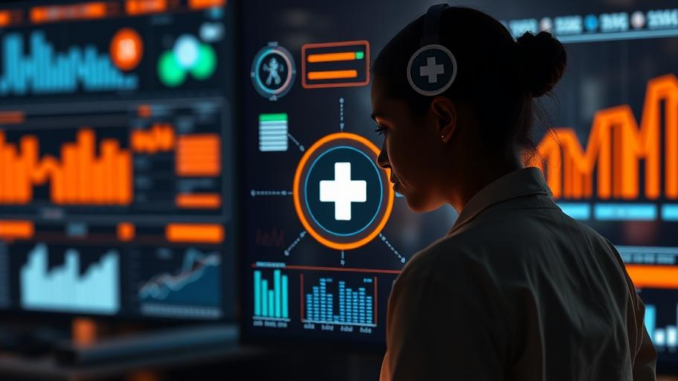
Electronic Health Records: A Critical Review of Data Utility, Challenges, and Future Directions for Predictive Healthcare
Abstract
Electronic Health Records (EHRs) have become ubiquitous in modern healthcare systems, representing a vast repository of patient data. While initially implemented for administrative and clinical documentation purposes, their potential for secondary uses, particularly in predictive analytics and clinical decision support, is increasingly recognized. This report critically examines the evolution of EHRs, their current functionalities, and the multifaceted challenges associated with leveraging EHR data for advanced analytics. We explore data quality issues, interoperability hurdles, data privacy and security concerns, and the potential for bias amplification in algorithmic applications. Furthermore, we analyze strategies for overcoming these challenges, including data standardization efforts, advanced natural language processing techniques, federated learning approaches, and the ethical considerations necessary for responsible AI deployment in healthcare. Finally, the report delves into emerging trends and future directions for EHR development, emphasizing the integration of artificial intelligence, the expansion of patient-generated health data, and the role of EHRs in promoting personalized and preventative medicine. We conclude by arguing that realizing the full potential of EHRs requires a concerted effort from stakeholders across the healthcare ecosystem, prioritizing data governance, technological innovation, and a patient-centric approach.
Many thanks to our sponsor Esdebe who helped us prepare this research report.
1. Introduction
The widespread adoption of Electronic Health Records (EHRs) marks a significant transformation in healthcare delivery and information management. Driven by government incentives, regulatory mandates, and the promise of improved efficiency and patient outcomes, EHR systems have transitioned from primarily paper-based processes to digital platforms. This shift has generated a massive influx of structured and unstructured data, creating opportunities for leveraging this information to improve clinical practice, public health surveillance, and healthcare research [1]. However, the journey towards realizing the full potential of EHRs is fraught with challenges. The heterogeneity of EHR systems, variations in data quality, and concerns surrounding patient privacy and data security pose significant obstacles to effectively utilizing EHR data for advanced analytics and predictive modeling.
This report aims to provide a comprehensive overview of the current landscape of EHRs, focusing on their utility for predictive healthcare, the challenges associated with data utilization, and potential future directions for EHR development. We will delve into the technical, ethical, and policy-related considerations that are crucial for unlocking the transformative power of EHRs while mitigating potential risks. The report is intended to inform researchers, clinicians, policymakers, and industry professionals involved in the design, implementation, and utilization of EHR systems.
Many thanks to our sponsor Esdebe who helped us prepare this research report.
2. Evolution and Current Landscape of EHRs
The evolution of EHRs can be traced back to the early attempts at computerizing medical records in the 1960s and 1970s. However, widespread adoption was slow due to technological limitations and a lack of standardization. The Health Information Technology for Economic and Clinical Health (HITECH) Act of 2009, part of the American Recovery and Reinvestment Act, provided substantial financial incentives for healthcare providers to adopt and meaningfully use certified EHR technology, significantly accelerating EHR adoption rates across the United States [2].
Today, EHR systems encompass a wide range of functionalities, including:
- Clinical Documentation: Capturing patient demographics, medical history, diagnoses, medications, allergies, and treatment plans.
- Order Entry: Electronically ordering medications, laboratory tests, and other medical services.
- Decision Support: Providing alerts, reminders, and evidence-based guidelines to assist clinicians in making informed decisions.
- Results Management: Displaying and managing laboratory results, imaging reports, and other diagnostic information.
- Billing and Coding: Generating claims for reimbursement from insurance companies.
- Reporting and Analytics: Tracking key performance indicators, monitoring patient outcomes, and generating reports for quality improvement initiatives.
Despite the widespread adoption of EHRs, significant variations exist in the functionalities and capabilities of different EHR systems. These variations can be attributed to differences in vendor implementations, user preferences, and organizational needs. Furthermore, the lack of interoperability between different EHR systems remains a major challenge, hindering the seamless exchange of patient information across healthcare settings [3].
Many thanks to our sponsor Esdebe who helped us prepare this research report.
3. EHR Data for Predictive Analytics: Opportunities and Applications
The wealth of data contained within EHRs presents unprecedented opportunities for predictive analytics in healthcare. By applying statistical modeling and machine learning techniques to EHR data, researchers and clinicians can identify patterns and predict future health outcomes, enabling proactive interventions and personalized treatment strategies. Some key applications of EHR data in predictive analytics include:
- Disease Prediction: Identifying individuals at high risk for developing chronic diseases such as diabetes, heart disease, and cancer. Predictive models can incorporate factors such as demographics, medical history, laboratory results, and lifestyle factors to estimate an individual’s risk of developing a specific disease [4].
- Risk Stratification: Categorizing patients based on their risk of adverse events such as hospital readmissions, complications, and mortality. This information can be used to prioritize resources and tailor interventions to high-risk patients [5].
- Treatment Response Prediction: Predicting how patients will respond to different treatments based on their individual characteristics and medical history. This can help clinicians select the most effective treatment options for each patient, minimizing the risk of adverse effects and improving treatment outcomes [6].
- Clinical Decision Support: Integrating predictive models into clinical workflows to provide real-time decision support to clinicians. For example, a predictive model could alert a clinician to a patient’s risk of sepsis based on their vital signs and laboratory results, prompting early intervention [7].
- Public Health Surveillance: Monitoring disease outbreaks, tracking vaccination rates, and identifying trends in healthcare utilization. EHR data can provide valuable insights for public health agencies to inform policy decisions and allocate resources effectively [8].
The successful implementation of predictive analytics in healthcare requires access to high-quality, reliable, and representative data. However, as discussed in the following section, numerous challenges exist in leveraging EHR data for these purposes.
Many thanks to our sponsor Esdebe who helped us prepare this research report.
4. Challenges in Utilizing EHR Data for Predictive Analytics
While the potential benefits of using EHR data for predictive analytics are undeniable, significant challenges must be addressed to ensure the accuracy, reliability, and ethical use of this data. These challenges can be broadly categorized as follows:
4.1 Data Quality Issues
EHR data is often characterized by inconsistencies, inaccuracies, and missing information. These data quality issues can arise from various sources, including:
- Data Entry Errors: Human errors during data entry can lead to inaccurate or incomplete records. Typos, misspellings, and incorrect coding can compromise the integrity of the data [9].
- Lack of Standardization: Different EHR systems may use different coding systems, terminologies, and data formats, making it difficult to integrate data from multiple sources [10].
- Data Fragmentation: Patient data may be scattered across multiple EHR systems, making it challenging to obtain a comprehensive view of a patient’s medical history.
- Temporal Inconsistencies: Data may be recorded at different time points or with varying levels of detail, making it difficult to track changes in patient health over time.
- Data Drift: The data distribution can change over time, which can cause predictive models trained on historical data to become less accurate [11].
4.2 Interoperability Challenges
Interoperability refers to the ability of different EHR systems to exchange and use information seamlessly. Despite efforts to promote interoperability through standards such as HL7 and FHIR, significant challenges remain [12]. These challenges include:
- Technical Barriers: Different EHR systems may use different communication protocols, data formats, and security mechanisms, making it difficult to establish interoperable connections.
- Semantic Barriers: Even if two EHR systems can exchange data, they may interpret the data differently due to variations in coding systems and terminologies.
- Organizational Barriers: Healthcare organizations may be reluctant to share data with competitors or may lack the resources and expertise to implement interoperability solutions.
- Policy and Regulatory Barriers: Data privacy regulations and other legal constraints can limit the ability to share patient information across organizations.
4.3 Data Privacy and Security Concerns
Protecting patient privacy and ensuring data security are paramount when using EHR data for predictive analytics. EHR data contains sensitive information that, if compromised, could lead to identity theft, discrimination, and other harms. Key concerns include:
- Unauthorized Access: Hackers or malicious insiders could gain unauthorized access to EHR systems, compromising patient data.
- Data Breaches: Data breaches can occur due to security vulnerabilities in EHR systems, human error, or social engineering attacks.
- Data Re-identification: Even de-identified data can be re-identified using sophisticated techniques, potentially exposing patient identities [13].
- Privacy Violations: Inappropriate data sharing or use of patient data without informed consent can violate patient privacy rights.
4.4 Bias and Fairness Considerations
EHR data may reflect existing health inequities, leading to biased predictive models that perpetuate disparities in healthcare. These biases can arise from:
- Data Collection Bias: Certain populations may be underrepresented in EHR data due to factors such as lack of access to healthcare or distrust of the healthcare system.
- Measurement Bias: The way data is collected or recorded may differ across different populations, leading to systematic errors.
- Algorithmic Bias: Machine learning algorithms can amplify existing biases in the data, leading to unfair or discriminatory outcomes [14].
Addressing these challenges requires a multifaceted approach that encompasses data standardization, advanced analytical techniques, robust security measures, and ethical guidelines for data use.
Many thanks to our sponsor Esdebe who helped us prepare this research report.
5. Strategies for Overcoming Challenges and Enhancing Data Utility
Several strategies can be employed to address the challenges associated with utilizing EHR data for predictive analytics and to enhance the overall data utility. These strategies include:
5.1 Data Standardization and Harmonization
Implementing standardized coding systems, terminologies, and data formats is crucial for improving data quality and enabling interoperability. Efforts to promote data standardization include:
- Adoption of Standardized Terminologies: Encouraging the use of standardized terminologies such as SNOMED CT, LOINC, and ICD-10 can help ensure that data is consistently coded across different EHR systems [15].
- Data Mapping and Transformation: Developing tools and techniques for mapping data from different EHR systems to a common data model can facilitate data integration and analysis.
- Data Quality Audits: Regularly auditing EHR data to identify and correct errors and inconsistencies can improve data accuracy and reliability.
5.2 Advanced Natural Language Processing (NLP)
NLP techniques can be used to extract structured information from unstructured text in EHRs, such as clinical notes and discharge summaries. This can help to fill gaps in structured data and provide a more comprehensive view of patient health [16]. Specific NLP applications include:
- Entity Extraction: Identifying and extracting key medical concepts such as diagnoses, medications, and procedures from text.
- Sentiment Analysis: Determining the emotional tone or sentiment expressed in clinical notes, which can provide insights into patient experiences.
- Relationship Extraction: Identifying relationships between medical concepts, such as the association between a diagnosis and a medication.
5.3 Federated Learning
Federated learning is a distributed machine learning approach that allows models to be trained on data residing on multiple EHR systems without directly sharing the data. This can help to address data privacy concerns and enable collaborative research across organizations [17].
5.4 Synthetic Data Generation
Synthetic data is artificially generated data that mimics the statistical properties of real data but does not contain any personally identifiable information. Synthetic data can be used to train machine learning models or to test data analytics tools without compromising patient privacy [18].
5.5 Robust Security Measures and Data Governance
Implementing robust security measures and establishing clear data governance policies are essential for protecting patient privacy and ensuring data security. These measures include:
- Access Controls: Restricting access to EHR data based on user roles and responsibilities.
- Encryption: Encrypting data both in transit and at rest to protect it from unauthorized access.
- Auditing: Tracking all access to EHR data to detect and investigate suspicious activity.
- Data Use Agreements: Establishing clear guidelines for data sharing and use, including provisions for data privacy and security.
- Ethical Review Boards: Reviewing and approving research proposals involving EHR data to ensure that they comply with ethical principles and data privacy regulations.
5.6 Addressing Bias and Promoting Fairness
Mitigating bias and promoting fairness in predictive models requires careful attention to data collection, model development, and evaluation. Strategies to address bias include:
- Data Augmentation: Over-sampling underrepresented populations in the data to reduce bias.
- Bias Detection and Mitigation Techniques: Using statistical techniques to identify and mitigate bias in predictive models.
- Fairness-Aware Machine Learning: Developing machine learning algorithms that are explicitly designed to promote fairness and equity.
- Transparency and Explainability: Making predictive models more transparent and explainable to help identify potential sources of bias and ensure accountability [19].
Many thanks to our sponsor Esdebe who helped us prepare this research report.
6. Future Directions and Emerging Trends
The future of EHRs is likely to be shaped by several emerging trends and technological advancements. These include:
6.1 Integration of Artificial Intelligence (AI)
AI is poised to play an increasingly important role in EHRs, automating tasks, improving clinical decision support, and enabling personalized medicine. AI applications in EHRs include:
- Automated Documentation: Using AI to automatically generate clinical notes and summaries from patient encounters.
- Intelligent Alerts and Reminders: Providing personalized alerts and reminders to clinicians based on patient risk factors and clinical guidelines.
- Personalized Treatment Recommendations: Using AI to generate personalized treatment recommendations based on patient characteristics and medical history.
- Predictive Analytics for Population Health Management: Using AI to identify high-risk populations and develop targeted interventions to improve population health outcomes [20].
6.2 Expansion of Patient-Generated Health Data (PGHD)
PGHD, such as data from wearable devices and mobile health apps, is becoming increasingly integrated into EHRs. This data can provide valuable insights into patient behavior and health status outside of the clinical setting, enabling more personalized and proactive care [21].
6.3 Enhanced Interoperability and Data Sharing
Efforts to improve interoperability and data sharing are likely to continue, driven by regulatory mandates and the increasing demand for seamless data exchange across healthcare settings. The widespread adoption of standards such as FHIR is expected to facilitate interoperability and enable the development of innovative healthcare applications.
6.4 Focus on Patient Engagement and Empowerment
EHRs are increasingly being designed to empower patients and engage them in their own healthcare. Patient portals provide patients with access to their medical records, allowing them to view their lab results, schedule appointments, and communicate with their providers. These portals can also be used to collect patient feedback and preferences, enabling more patient-centered care.
6.5 Blockchain Technology
Blockchain technology has the potential to enhance data security, improve data provenance, and facilitate secure data sharing in healthcare. Blockchain can be used to create a secure and immutable record of patient data, enabling patients to control access to their information and share it with trusted parties [22].
Many thanks to our sponsor Esdebe who helped us prepare this research report.
7. Conclusion
Electronic Health Records have revolutionized healthcare delivery, offering unprecedented opportunities for data-driven insights and improved patient outcomes. However, realizing the full potential of EHRs requires addressing significant challenges related to data quality, interoperability, privacy, and bias. By implementing robust data standardization efforts, leveraging advanced analytical techniques, and prioritizing ethical considerations, healthcare organizations can unlock the transformative power of EHR data. The future of EHRs will be shaped by emerging trends such as the integration of AI, the expansion of PGHD, and enhanced interoperability, paving the way for a more personalized, preventative, and equitable healthcare system. A collaborative and concerted effort from stakeholders across the healthcare ecosystem is essential to ensure that EHRs are used responsibly and effectively to improve the health and well-being of individuals and communities.
References
[1] Blumenthal, D., & Tavenner, M. (2010). Meaningful use stage 1. New England Journal of Medicine, 363(6), 501-504.
[2] Adler-Milstein, J., Jha, A. K. (2012). Meaningful use: achieving US healthcare goals. BMJ, 344, e1591.
[3] Vest, J. R., & McGinnis, T. (2011). Health information exchange: persistent challenges and new strategies. Journal of the American Medical Informatics Association, 19(4), 592-598.
[4] Goldstein, B. A., Navar, A. M., Carter, R. E., Pencina, M. J., & Ioannidis, J. P. A. (2017). Development and validation of a parsimonious risk prediction model for incident diabetes in the US: A prospective cohort study. PloS medicine, 14(7), e1002355.
[5] Kansagara, D., Englander, H., Salanitro, A. H., Kagen, D., Theobald, C., Freeman, M., … & Relevo, R. (2011). Risk prediction models for hospital readmission: a systematic review. Jama, 306(15), 1688-1698.
[6] Simon, G. E., Ralston, J. D., Grothaus, L. C., Powers, D., Unützer, J., & Katon, W. J. (2006). Randomized trial of monitoring, feedback, and brief education for improving adherence to antidepressant medication. Journal of the American Medical Association, 295(21), 2526-2533.
[7] Henry, K. E., Hager, D. N., Pronovost, P. J., Saria, S. (2015). A targeted real-time early warning score (TREWScore) for septic shock. Science translational medicine, 7(299), 299ra122.
[8] Buckeridge, D. L., Burkom, H., & Kouzi, A. C. (2004). Population health surveillance. American Journal of Public Health, 94(8), 1266-1270.
[9] Weiskopf, N. G., & Weng, C. (2013). Methods and dimensions of electronic health record data quality assessment: enabling reuse for clinical research. Journal of the American Medical Informatics Association, 20(1), 144-151.
[10] Kahn, M. G., Callahan, T. J., Barnard, J., Bauck, A. E., Brown, S. H., Davidson, B. N., … & Waitman, L. R. (2009). A harmonized data quality assessment terminology and checklist. Journal of the American Medical Informatics Association, 16(5), 575-581.
[11] Gama, J., Žliobaite, I., Pechenizkiy, M., & Bifet, A. (2014). A survey on concept drift adaptation. ACM computing surveys (CSUR), 46(4), 1-37.
[12] De Lusignan, S., & van Weel, C. (2006). The use of routinely collected data for improving primary care. Informatics in Primary Care, 14(1), 55-61.
[13] Narayanan, A., & Shmatikov, V. (2008). Robust de-anonymization of large sparse datasets. In 2008 IEEE Symposium on Security and Privacy (sp 2008) (pp. 111-125). IEEE.
[14] Obermeyer, Z., Powers, B., Vogeli, C., & Mullainathan, S. (2019). Dissecting racial bias in an algorithm used to manage the health of populations. Science, 366(6464), 447-453.
[15] Spackman, K. A., Campbell, K. E., & Cote, R. A. (1997). SNOMED RT: a reference terminology for health care. Advances in patient safety: From research to implementation.
[16] Chapman, W. W., Bridewell, W., Hanbury, P., Cooper, G. F., & Buchanan, B. G. (2001). A simple algorithm for identifying negated findings and diseases in discharge summaries. Journal of biomedical informatics, 34(5), 301-310.
[17] Rieke, N., Hancox, J., Li, W., Milletari, F., Roth, H. R., Albarqouni, S., … & Bakas, S. (2020). The future of digital health with federated learning. NPJ digital medicine, 3(1), 1-7.
[18] Tucker, A., Wang, Z., Rotala, A., Stylianou, A., Beatson, R., Diaz, G., … & Kantarcioglu, M. (2023). Large language models for synthesizing clinically realistic structured electronic health record data. Nature communications, 14(1), 6458.
[19] Doshi-Velez, F., Kortz, M., Budish, R., Bengio, S., Gershman, S. J., Duflo, E., … & Wood, A. (2017). Accountability of AI: Towards systems that value explainability and ethics. arXiv preprint arXiv:1711.01134.
[20] Jiang, F., Jiang, Y., Zhi, H., Dong, Y., Li, H., Ma, S., … & Wang, Y. (2017). Artificial intelligence in healthcare: past, present and future. Stroke and vascular neurology, 2(4), 230-243.
[21] Haluza, D., & Jungwirth, D. (2019). Healthy living supported by mHealth: a systematic review of mobile apps for specific diseases. Wiener klinische Wochenschrift, 131(11-12), 227-237.
[22] Kuo, T. T., Kim, H. E., & Ohno-Machado, L. (2017). Blockchain distributed ledger technologies for biomedical and health care applications. Journal of the American Medical Informatics Association, 24(6), 1211-1220.
The discussion of data quality challenges is critical. How can we proactively identify and rectify data drift in EHRs to maintain the accuracy of predictive models over time, especially given the evolving nature of medical practices and patient demographics?