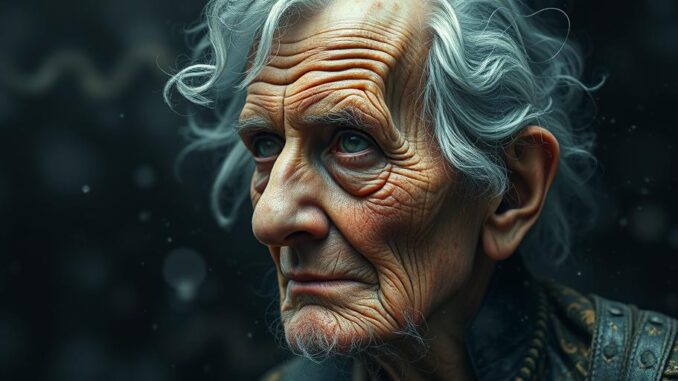
Abstract
Entropy, a fundamental concept in thermodynamics, quantifies the degree of disorder or randomness within a system. While traditionally applied to physical and chemical systems, its relevance to biological processes is increasingly recognized. This report provides a comprehensive overview of entropy in biological systems, delving into its theoretical underpinnings, mechanisms of action, and implications for aging and disease. We examine different approaches to measuring entropy in biological contexts, including Shannon entropy, approximate entropy, and sample entropy, critically evaluating their strengths and limitations. The report explores the role of entropy in driving aging processes at the molecular, cellular, and organismal levels, highlighting its connection to cellular damage, protein misfolding, and declining organ function. Furthermore, we discuss potential interventions aimed at mitigating the effects of entropy, such as caloric restriction, autophagy induction, and antioxidant therapies. Finally, we address the challenges and future directions in entropy research, emphasizing the need for improved measurement techniques and a deeper understanding of the complex interplay between entropy and biological processes.
Many thanks to our sponsor Esdebe who helped us prepare this research report.
1. Introduction
The second law of thermodynamics dictates that the entropy of a closed system tends to increase over time, leading to a state of greater disorder and reduced energy availability. While living organisms are not closed systems, they are subject to entropic forces that degrade their structure and function. Traditionally, biology has focused on the intricate regulatory mechanisms and specific pathways that maintain homeostasis and promote survival. However, an emerging perspective recognizes the importance of entropy as a fundamental constraint on biological systems. This perspective suggests that the accumulation of entropy, often manifested as molecular damage, cellular dysfunction, and loss of organizational complexity, is a major driver of aging and disease.
Unlike simple physical systems, biological systems exhibit remarkable complexity, characterized by hierarchical organization, intricate feedback loops, and dynamic interactions between components. Therefore, quantifying and interpreting entropy in biological contexts presents significant challenges. Nevertheless, advances in information theory, statistical mechanics, and systems biology have provided new tools for studying entropy in living organisms. These tools allow us to analyze the information content of biological signals, assess the degree of order in molecular structures, and model the flow of energy and matter through metabolic networks. By applying these approaches, we can gain a deeper understanding of the role of entropy in shaping biological processes and driving the aging process.
This report aims to provide a comprehensive overview of entropy in biological systems, focusing on its measurement, mechanisms, and implications for aging and disease. We will explore the theoretical foundations of entropy, examine different methods for quantifying entropy in biological contexts, and discuss the role of entropy in driving aging processes at the molecular, cellular, and organismal levels. Furthermore, we will review potential interventions aimed at mitigating the effects of entropy and discuss the challenges and future directions in entropy research.
Many thanks to our sponsor Esdebe who helped us prepare this research report.
2. Theoretical Foundations of Entropy
Entropy, in its most basic definition, is a measure of disorder or randomness within a system. In thermodynamics, it is defined mathematically through the Clausius relation, dS = dQ/T, where dS represents the change in entropy, dQ represents the heat transferred, and T is the temperature. This macroscopic definition, however, lacks the intuitive appeal needed for understanding entropy’s role in biological systems. A more insightful perspective comes from statistical mechanics, where entropy (S) is related to the number of microstates (W) corresponding to a given macrostate through Boltzmann’s equation: S = k * ln(W), where k is Boltzmann’s constant.
This equation highlights the connection between entropy and information. A system with a high number of possible microstates for a given macrostate (e.g., a gas with molecules arranged randomly) has high entropy. Conversely, a system with only a few possible microstates (e.g., a crystal with molecules arranged in a highly ordered lattice) has low entropy. Claude Shannon, in his seminal work on information theory, developed a similar concept of entropy to quantify the uncertainty or information content of a message. Shannon entropy, defined as H = -Σ p(i) * log2(p(i)), where p(i) is the probability of the ith outcome, provides a powerful tool for analyzing the randomness and unpredictability of biological signals.
It is crucial to distinguish between different types of entropy. Thermodynamic entropy focuses on the energy dispersal and disorder in a physical system, whereas information entropy quantifies the uncertainty or information content of a signal. However, these two concepts are deeply related. Landauer’s principle states that erasing information requires a minimum amount of energy dissipation, implying a direct link between information entropy and thermodynamic entropy. This principle has profound implications for biological systems, where information processing is essential for maintaining order and function. Every biological process, from DNA replication to protein synthesis, involves the transfer and processing of information, which inevitably leads to an increase in entropy. Therefore, understanding the theoretical foundations of entropy is crucial for comprehending its role in driving biological aging and disease.
Many thanks to our sponsor Esdebe who helped us prepare this research report.
3. Measuring Entropy in Biological Systems
Quantifying entropy in biological systems poses significant challenges due to their inherent complexity and dynamic nature. Several methods have been developed to address these challenges, each with its own strengths and limitations. These methods can be broadly categorized into information-theoretic approaches, statistical mechanics approaches, and image-based approaches.
3.1. Information-Theoretic Approaches
Information-theoretic approaches, such as Shannon entropy, approximate entropy (ApEn), and sample entropy (SampEn), are widely used to analyze the complexity and regularity of biological time series data. Shannon entropy, as mentioned earlier, quantifies the uncertainty or randomness of a signal based on the probability distribution of its values. However, Shannon entropy is sensitive to the length of the time series and may not accurately capture the underlying dynamics of complex systems. ApEn and SampEn are improved measures of complexity that are less sensitive to the length of the time series and can better distinguish between random and deterministic signals. ApEn quantifies the irregularity of a time series by measuring the probability that similar patterns of data will remain similar on next incremental comparisons. SampEn is similar to ApEn but it is more robust because it does not count self-matches, leading to a more accurate assessment of complexity.
These information-theoretic measures have been applied to a wide range of biological signals, including heart rate variability (HRV), electroencephalogram (EEG) data, and gene expression profiles. For example, studies have shown that HRV entropy decreases with age and disease, indicating a loss of physiological complexity. Similarly, EEG entropy has been used to distinguish between different brain states and to diagnose neurological disorders. However, it is important to note that these measures are sensitive to the parameters used in their calculation and may not always accurately reflect the underlying biological processes.
3.2. Statistical Mechanics Approaches
Statistical mechanics approaches, such as free energy calculations and molecular dynamics simulations, can be used to quantify the entropy of biomolecules and cellular structures. These approaches typically involve constructing a detailed model of the system and then using statistical mechanics to calculate the probability distribution of its possible states. The entropy can then be calculated from this probability distribution using Boltzmann’s equation. For example, molecular dynamics simulations can be used to study the conformational entropy of proteins, which is related to the number of possible conformations that the protein can adopt. Changes in conformational entropy can affect protein folding, stability, and function, and have been implicated in a variety of diseases, including Alzheimer’s disease and Parkinson’s disease.
However, these approaches are computationally intensive and require accurate models of the system. Furthermore, they may not be applicable to complex biological systems with many interacting components. Despite these limitations, statistical mechanics approaches provide valuable insights into the role of entropy in shaping the structure and function of biomolecules and cellular structures.
3.3. Image-Based Approaches
Image-based approaches, such as fractal analysis and texture analysis, can be used to quantify the structural complexity of biological tissues and organs. Fractal analysis involves calculating the fractal dimension of an image, which is a measure of its self-similarity at different scales. Tissues with a high degree of structural complexity, such as the brain and the lungs, typically have high fractal dimensions. Texture analysis involves quantifying the spatial distribution of pixel intensities in an image. Changes in texture can reflect alterations in tissue structure and organization, and have been used to diagnose a variety of diseases, including cancer and fibrosis.
For example, fractal analysis has been used to study the structural complexity of the brain in patients with Alzheimer’s disease. Studies have shown that the fractal dimension of the brain decreases with age and disease, indicating a loss of structural complexity. Similarly, texture analysis has been used to quantify the collagen content of fibrotic tissues. These image-based approaches provide a non-invasive way to assess the structural integrity of biological tissues and organs. One can argue that the quantification of entropy using image-based approaches is more of a description of the appearance of the biological material as opposed to a quantification of its randomness.
3.4. Challenges in Entropy Measurement
Despite the development of these various methods, quantifying entropy in biological systems remains a challenging task. One major challenge is the lack of a universally accepted definition of entropy in biological contexts. Another challenge is the difficulty of obtaining accurate and reliable data. Biological systems are inherently noisy and dynamic, and data may be affected by a variety of factors, such as measurement error, individual variability, and environmental conditions. Therefore, it is important to carefully consider the limitations of each method and to use multiple approaches to validate the results.
Many thanks to our sponsor Esdebe who helped us prepare this research report.
4. Entropy and Aging Mechanisms
The accumulation of entropy is increasingly recognized as a central driver of aging. At the molecular level, entropy manifests as DNA damage, protein misfolding, and lipid peroxidation. These forms of molecular damage impair cellular function and contribute to the development of age-related diseases. At the cellular level, entropy leads to cellular senescence, mitochondrial dysfunction, and impaired autophagy. These cellular changes disrupt tissue homeostasis and contribute to organ dysfunction. At the organismal level, entropy manifests as a decline in physiological complexity, reduced resilience to stress, and increased susceptibility to disease.
4.1. Molecular Entropy
DNA is constantly exposed to damaging agents, such as reactive oxygen species (ROS) and environmental toxins. These agents can cause DNA mutations, strand breaks, and other forms of DNA damage. DNA damage can disrupt gene expression, impair DNA replication, and lead to cellular dysfunction. The accumulation of DNA damage over time is a major contributor to aging. Proteins are also susceptible to damage, such as misfolding and aggregation. Protein misfolding can be caused by a variety of factors, including oxidative stress, heat shock, and genetic mutations. Misfolded proteins can aggregate and form toxic oligomers that disrupt cellular function and contribute to neurodegenerative diseases. Lipids are also susceptible to damage, such as peroxidation. Lipid peroxidation is a chain reaction initiated by ROS that can damage cell membranes and other lipid-containing structures. The accumulation of lipid peroxidation products can impair cellular function and contribute to aging.
4.2. Cellular Entropy
Cellular senescence is a state of irreversible cell cycle arrest that is induced by various stressors, such as DNA damage and telomere shortening. Senescent cells accumulate in tissues with age and secrete a variety of pro-inflammatory cytokines, growth factors, and proteases, collectively known as the senescence-associated secretory phenotype (SASP). The SASP can disrupt tissue homeostasis, promote inflammation, and contribute to age-related diseases. Mitochondrial dysfunction is another hallmark of aging. Mitochondria are the powerhouses of the cell, responsible for generating ATP through oxidative phosphorylation. Mitochondrial dysfunction can lead to decreased ATP production, increased ROS production, and impaired cellular function. Autophagy is a cellular process that removes damaged organelles and misfolded proteins. Autophagy declines with age, leading to the accumulation of damaged cellular components and impaired cellular function. A decreased capacity to eliminate accumulated waste is in itself an increase in entropy.
4.3. Organismal Entropy
At the organismal level, the accumulation of entropy manifests as a decline in physiological complexity. Physiological complexity refers to the intricate and dynamic interactions between different organ systems. As we age, these interactions become less coordinated and less responsive to stress. This loss of physiological complexity can lead to reduced resilience to stress and increased susceptibility to disease. For example, the cardiovascular system becomes less responsive to changes in blood pressure, the immune system becomes less effective at fighting off infections, and the nervous system becomes less able to maintain cognitive function. These age-related declines in organ function contribute to the overall decline in health and vitality.
Many thanks to our sponsor Esdebe who helped us prepare this research report.
5. Interventions to Mitigate Entropy
Given the central role of entropy in driving aging, interventions aimed at mitigating its effects hold great promise for promoting healthy aging and extending lifespan. Several interventions have shown potential in reducing entropy and improving healthspan in animal models and humans. These interventions include caloric restriction, autophagy induction, antioxidant therapies, and senolytics.
5.1. Caloric Restriction
Caloric restriction (CR), defined as a reduction in calorie intake without malnutrition, has been shown to extend lifespan and improve healthspan in a wide range of organisms, from yeast to primates. CR has been shown to reduce oxidative stress, improve mitochondrial function, and enhance autophagy. These effects are thought to be mediated by a variety of mechanisms, including activation of sirtuins, inhibition of mTOR signaling, and increased expression of stress resistance genes. While the exact mechanisms underlying the beneficial effects of CR are still being investigated, it is clear that CR can mitigate the effects of entropy and promote healthy aging.
5.2. Autophagy Induction
Autophagy is a cellular process that removes damaged organelles and misfolded proteins. Autophagy declines with age, leading to the accumulation of damaged cellular components and impaired cellular function. Interventions that induce autophagy, such as rapamycin and spermidine, have been shown to extend lifespan and improve healthspan in animal models. Rapamycin is an inhibitor of mTOR signaling, which is a negative regulator of autophagy. Spermidine is a polyamine that stimulates autophagy through a variety of mechanisms. These interventions have been shown to reduce the accumulation of damaged cellular components, improve mitochondrial function, and protect against age-related diseases.
5.3. Antioxidant Therapies
Oxidative stress is a major contributor to aging. Antioxidants are molecules that neutralize ROS and protect against oxidative damage. Interventions that increase antioxidant levels, such as supplementation with vitamin E, vitamin C, and N-acetylcysteine (NAC), have been shown to reduce oxidative stress and improve healthspan in some animal models. However, the results of antioxidant therapies in humans have been mixed. Some studies have shown that antioxidant supplementation can improve health outcomes, while others have found no benefit or even harm. It is possible that the effectiveness of antioxidant therapies depends on the specific antioxidant used, the dose administered, and the individual’s genetic background.
5.4. Senolytics
Senolytics are drugs that selectively kill senescent cells. Senescent cells accumulate in tissues with age and secrete a variety of pro-inflammatory cytokines, growth factors, and proteases, collectively known as the SASP. The SASP can disrupt tissue homeostasis, promote inflammation, and contribute to age-related diseases. Senolytics have been shown to extend lifespan and improve healthspan in animal models. For example, the combination of dasatinib and quercetin has been shown to selectively kill senescent cells and improve physical function in aged mice. Clinical trials are currently underway to evaluate the safety and efficacy of senolytics in humans.
Many thanks to our sponsor Esdebe who helped us prepare this research report.
6. Challenges and Future Directions
Despite significant advances in our understanding of entropy in biological systems, several challenges remain. One major challenge is the development of more accurate and reliable methods for quantifying entropy in vivo. Current methods are often limited by their sensitivity to noise, their reliance on specific assumptions, and their inability to capture the full complexity of biological systems. Future research should focus on developing new methods that can overcome these limitations and provide a more comprehensive assessment of entropy in living organisms.
Another challenge is the identification of the specific molecular and cellular mechanisms that link entropy to aging and disease. While we have identified several key players, such as DNA damage, protein misfolding, and mitochondrial dysfunction, the precise interplay between these factors is not fully understood. Future research should focus on elucidating the complex signaling pathways that regulate these processes and on identifying new targets for therapeutic intervention.
Furthermore, it is important to develop more effective interventions to mitigate the effects of entropy and promote healthy aging. While several interventions have shown promise in animal models, their effectiveness in humans remains to be determined. Future clinical trials should focus on evaluating the safety and efficacy of these interventions in diverse populations and on identifying the optimal strategies for personalized medicine. This may require considering an individual’s baseline entropy level, genetic predisposition, and environmental exposures.
Finally, a deeper understanding of the philosophical implications of entropy in biology is warranted. The concept of entropy challenges the traditional view of organisms as machines with perfectly orchestrated functions. Instead, it suggests that organisms are inherently subject to degradation and disorder. Embracing this perspective may lead to a more realistic and compassionate approach to healthcare, focusing on resilience, adaptation, and quality of life, rather than solely on extending lifespan at all costs.
Many thanks to our sponsor Esdebe who helped us prepare this research report.
7. Conclusion
Entropy is a fundamental concept in thermodynamics that has profound implications for biological systems. The accumulation of entropy, manifested as molecular damage, cellular dysfunction, and loss of organizational complexity, is a major driver of aging and disease. Quantifying and mitigating entropy in biological systems remains a challenging task, but advances in information theory, statistical mechanics, and systems biology have provided new tools for studying this phenomenon. Interventions aimed at mitigating the effects of entropy, such as caloric restriction, autophagy induction, and senolytics, hold great promise for promoting healthy aging and extending lifespan. Future research should focus on developing more accurate measurement techniques, elucidating the underlying mechanisms, and developing more effective interventions to combat the effects of entropy and promote a healthier and longer life.
Many thanks to our sponsor Esdebe who helped us prepare this research report.
References
- Kirkwood, T. B. L. (2005). Understanding the odd science of ageing. Cell, 120(4), 437-447.
- López-Otín, C., Blasco, M. A., Partridge, L., Serrano, M., & Kroemer, G. (2013). The hallmarks of aging. Cell, 153(6), 1194-1217.
- Shannon, C. E. (1948). A mathematical theory of communication. The Bell System Technical Journal, 27(3), 379-423.
- Richman, J. S., & Moorman, J. R. (2000). Physiological time-series analysis using approximate entropy and sample entropy. American Journal of Physiology-Heart and Circulatory Physiology, 278(6), H2039-H2049.
- Landauer, R. (1961). Irreversibility and heat generation in the computing process. IBM Journal of Research and Development, 5(3), 183-191.
- De Magalhães, J. P. (2013). From cells to ageing: A review of models and mechanisms. Experimental Cell Research, 319(14), 1955-1963.
- Boccardi, V., Bocchetta, M., Imarisio, A., & Santucci, G. (2011). Fractal dimension of gray matter as a biomarker for Alzheimer’s disease. PloS one, 6(8), e22854.
- Kennedy, B. K., Berger, S. L., Brunet, A., Campisi, J., Cuervo, A. M., Epel, E. S., … & Rando, T. A. (2014). Geroscience: linking aging to chronic disease. Cell, 159(4), 709-713.
- Fontana, L., Partridge, L., & Longo, V. D. (2010). Extending healthy life span–from yeast to humans. Science, 328(5976), 321-326.
- Madeo, F., Eisenberg, T., Büttner, S., Ruckenstuhl, C., & Kroemer, G. (2015). Spermidine: a physiological autophagy inducer acting as an anti-aging vitamin in yeast and mammals. Autophagy, 11(2), 281-283.
- Kirkland, J. L., Tchkonia, T., Zhu, Y., van Deursen, J. M., & Campisi, J. (2017). The clinical potential of senolytic drugs. Journal of the American Geriatrics Society, 65(10), 2297-2307.
Regarding the discussion on measuring entropy, the exploration of image-based approaches like fractal and texture analysis opens exciting avenues for non-invasive diagnostics. Could these methods be adapted to monitor the effectiveness of entropy-mitigating interventions, offering a visual and quantitative assessment of treatment success?
That’s a great point! Using image-based entropy measures to track the success of interventions is a compelling idea. Imagine using changes in fractal dimension in lung scans to visualize the impact of antioxidant therapies, offering a clear, measurable outcome. It may also be possible to track the treatments effect over time.
Editor: MedTechNews.Uk
Thank you to our Sponsor Esdebe
The mention of Landauer’s principle highlights an interesting connection between information processing and thermodynamics within biological systems. I wonder how the energy expenditure required for maintaining complex biological information compares across different species with varying lifespans.
That’s a fascinating question! It would be really insightful to explore the correlation between energy expenditure for information maintenance and lifespan across different species. Perhaps analyzing metabolic rates in conjunction with information processing complexity could reveal some interesting trends. Thanks for sparking this thought!
Editor: MedTechNews.Uk
Thank you to our Sponsor Esdebe
So, if we can’t perfectly orchestrate our functions and degradation is inevitable, does that mean my attempts at organizing my sock drawer are fundamentally futile? Is there an entropy-optimized sock folding technique I should know about?
That’s a hilarious and insightful question! While entropy might suggest sock drawer chaos is inevitable, perhaps strategically organizing them could slow down the inevitable spread of disorder, or at least make finding matching pairs faster. There may be scope for a research paper into entropy optimised sock folding!
Editor: MedTechNews.Uk
Thank you to our Sponsor Esdebe
So, if biological systems are fighting a losing battle against entropy, are we essentially elaborate sandcastles on the beach of time? Perhaps more resilient sandcastles, with clever drainage systems?
That’s a brilliant analogy! The ‘sandcastle’ concept really highlights the continuous effort needed to maintain biological order. Perhaps future research could explore how different ‘drainage systems’ (cellular repair mechanisms) influence the resilience of these ‘sandcastles’ against the entropic tides.
Editor: MedTechNews.Uk
Thank you to our Sponsor Esdebe