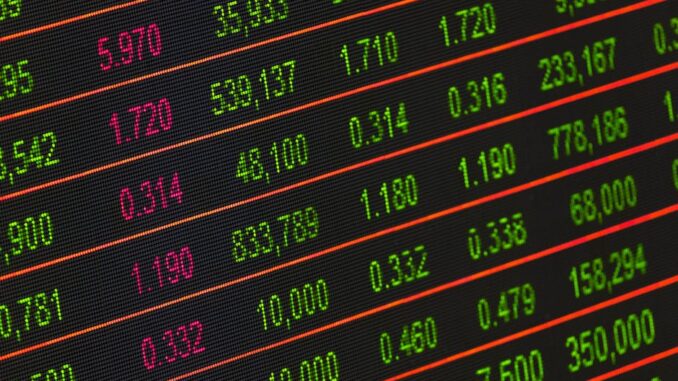
Navigating the Labyrinth: A Comprehensive Analysis of AI Regulation Across Diverse Sectors
Abstract
Artificial intelligence (AI) is rapidly permeating various sectors, from healthcare and finance to transportation and criminal justice. This widespread adoption presents both unprecedented opportunities and significant challenges, particularly concerning ethical considerations, bias, accountability, and safety. Consequently, the need for robust and adaptable regulatory frameworks governing AI is increasingly urgent. This report provides a comprehensive analysis of AI regulation across diverse sectors, examining existing regulatory landscapes, identifying key challenges in regulating AI, comparing different regulatory approaches adopted globally, and assessing the potential impact of AI regulation on innovation, fairness, and societal well-being. We delve into the intricacies of regulating AI in contexts far beyond medical devices to offer a holistic understanding of the issues involved. The report also explores the potential pitfalls of over-regulation and under-regulation, advocating for a balanced and adaptive approach that fosters innovation while safeguarding societal values. It also discusses the critical role of international cooperation in creating a cohesive and effective global regulatory landscape for AI.
1. Introduction
Artificial intelligence (AI) is no longer a futuristic concept but a present-day reality, profoundly impacting various facets of human life. Its applications span a wide range of sectors, including healthcare, finance, transportation, manufacturing, education, and even art. While AI offers the potential to solve complex problems, enhance efficiency, improve decision-making, and drive economic growth, it also poses significant challenges and risks. These include potential job displacement, algorithmic bias, privacy violations, security vulnerabilities, and ethical dilemmas regarding autonomy and accountability.
Given these transformative and potentially disruptive effects, the development and deployment of AI systems demand careful consideration and effective governance. This is where the role of regulation becomes crucial. Regulation aims to ensure that AI systems are developed and used responsibly, ethically, and in a manner that aligns with societal values and promotes the common good. However, regulating AI is a complex and multifaceted endeavor, fraught with challenges.
Traditional regulatory approaches often struggle to keep pace with the rapid advancements in AI technology. The inherent complexity of AI algorithms, their opacity (often referred to as the “black box” problem), and the dynamic nature of AI applications necessitate a more nuanced and adaptive regulatory framework. Furthermore, the global nature of AI development and deployment requires international cooperation and harmonization of regulatory standards.
This report aims to provide a comprehensive overview of the current state of AI regulation across diverse sectors. It examines the existing regulatory landscape, identifies key challenges in regulating AI, compares different regulatory approaches adopted globally, and assesses the potential impact of AI regulation on innovation and societal well-being. By providing a holistic understanding of the issues involved, this report aims to inform policymakers, industry stakeholders, and the broader public about the crucial role of regulation in shaping the future of AI.
2. Existing Regulatory Landscape for AI Across Sectors
The existing regulatory landscape for AI is fragmented and uneven, reflecting the diverse applications of AI and the varying levels of regulatory maturity across different jurisdictions and sectors. While there is no single, comprehensive AI regulation in most countries, several existing laws and regulations may apply to AI systems, depending on their specific functionalities and applications. These regulations often address issues such as data protection, consumer protection, product liability, and discrimination.
2.1 Data Protection and Privacy:
The General Data Protection Regulation (GDPR) in the European Union (EU) is a landmark regulation that has significant implications for AI. The GDPR sets strict rules for the processing of personal data, including data used to train and operate AI systems. It requires organizations to obtain explicit consent from individuals before collecting and processing their data, to provide transparency about how data is used, and to implement measures to ensure data security and privacy. The right to explanation, while not explicitly mandated in the GDPR, is often invoked in the context of automated decision-making, pushing for more transparency in AI systems. The California Consumer Privacy Act (CCPA) in the United States is another example of a data protection law that impacts AI, granting consumers greater control over their personal data. The ongoing debate in the US about a federal privacy law highlights the lack of a unified data protection framework, leading to a patchwork of state-level regulations that increase compliance complexity.
2.2 Consumer Protection:
Consumer protection laws, such as those related to unfair or deceptive trade practices, also apply to AI-powered products and services. These laws aim to protect consumers from harm caused by defective or misleading AI systems. For example, if an AI-powered chatbot provides inaccurate or misleading information to consumers, the company responsible for the chatbot may be held liable under consumer protection laws. The potential for AI to be used for deceptive marketing or to manipulate consumer behavior is a growing concern, necessitating stronger enforcement of consumer protection laws in the context of AI.
2.3 Product Liability:
Product liability laws hold manufacturers responsible for injuries or damages caused by defective products. These laws can apply to AI-powered products, such as autonomous vehicles or medical devices. Determining liability in cases involving AI systems can be challenging, particularly when the system’s behavior is unpredictable or difficult to explain. The question of who is responsible when an autonomous vehicle causes an accident – the manufacturer, the programmer, or the owner – is a complex legal issue that is still being debated.
2.4 Anti-Discrimination Laws:
Anti-discrimination laws prohibit discrimination based on protected characteristics such as race, gender, and religion. These laws can apply to AI systems that are used in areas such as hiring, lending, and housing. Algorithmic bias, where AI systems perpetuate or amplify existing societal biases, is a significant concern in this context. For example, an AI-powered hiring tool that is trained on biased data may discriminate against certain groups of job applicants. Ensuring fairness and non-discrimination in AI systems requires careful attention to data quality, algorithm design, and ongoing monitoring of system performance.
2.5 Sector-Specific Regulations:
In addition to general laws, several sectors have specific regulations that apply to AI. For example, the Food and Drug Administration (FDA) in the United States regulates AI-powered medical devices, while the Federal Aviation Administration (FAA) regulates AI-powered aviation systems. These sector-specific regulations often address issues such as safety, efficacy, and security. The regulatory landscape for AI in healthcare is particularly complex, given the potential for AI to improve patient outcomes but also the risks associated with inaccurate or unreliable AI systems.
2.6 Emerging AI-Specific Regulations:
While existing laws and regulations can be applied to AI, many policymakers and regulators believe that more specific AI regulations are needed. The EU’s proposed AI Act is a comprehensive regulatory framework that aims to address the risks posed by AI systems. The AI Act classifies AI systems based on their risk level, with high-risk systems subject to strict requirements, such as conformity assessments, transparency obligations, and human oversight. The UK is taking a different approach, focusing on a pro-innovation regulatory framework that is sector-specific and principles-based. This approach emphasizes flexibility and adaptability, allowing regulators to tailor their approach to the specific risks and opportunities presented by AI in different sectors.
3. Challenges in Regulating AI
Regulating AI presents a unique set of challenges that traditional regulatory frameworks often struggle to address. These challenges stem from the inherent characteristics of AI technology, its rapid pace of development, and its diverse range of applications.
3.1 The Black Box Problem:
Many AI systems, particularly those based on deep learning, are notoriously opaque. Their decision-making processes are often difficult to understand, even for the developers who created them. This lack of transparency, often referred to as the “black box” problem, makes it difficult to assess the fairness, safety, and reliability of AI systems. It also makes it challenging to identify and correct biases or errors in the system’s algorithms. Explainable AI (XAI) is an emerging field that aims to address the black box problem by developing techniques to make AI systems more transparent and understandable. However, XAI is still in its early stages, and its effectiveness in complex AI systems remains to be seen.
3.2 Rapid Pace of Technological Change:
AI technology is evolving at an unprecedented pace. New algorithms, techniques, and applications are constantly being developed. This rapid pace of change makes it difficult for regulators to keep up. Regulations that are based on current technology may quickly become obsolete as AI technology advances. This necessitates a more flexible and adaptive regulatory approach that can accommodate future technological developments. A principles-based approach, focusing on ethical considerations and broad objectives, may be more suitable than a rules-based approach that focuses on specific technical details.
3.3 Data Dependency and Bias:
AI systems are heavily dependent on data. The quality and quantity of data used to train AI systems can significantly impact their performance and behavior. If the data is biased, the AI system will likely perpetuate or amplify those biases. This can lead to unfair or discriminatory outcomes. Addressing data bias requires careful attention to data collection, data preprocessing, and algorithm design. It also requires ongoing monitoring of system performance to detect and mitigate potential biases. Furthermore, access to high-quality, representative datasets is crucial for developing fair and accurate AI systems.
3.4 Algorithmic Accountability:
Determining accountability for the actions of AI systems is a complex legal and ethical challenge. When an AI system makes a mistake or causes harm, it can be difficult to determine who is responsible. Is it the developer of the algorithm, the user of the system, or the company that deployed the system? Existing legal frameworks may not be well-suited to address this issue. New legal frameworks may be needed to assign responsibility for the actions of AI systems. Furthermore, mechanisms for redress and compensation for individuals harmed by AI systems need to be established.
3.5 Defining AI:
The very definition of AI is fluid and contested, making it difficult to regulate. What constitutes AI and what does not? A narrow definition may exclude some systems that pose significant risks, while a broad definition may capture systems that are not truly AI and do not require regulation. A clear and consistent definition of AI is essential for effective regulation.
3.6 Innovation vs. Regulation Dilemma:
Over-regulation of AI could stifle innovation and hinder the development of beneficial AI applications. Under-regulation, on the other hand, could lead to the deployment of unsafe or unethical AI systems. Striking the right balance between promoting innovation and protecting society is a critical challenge for policymakers. A risk-based approach, focusing on regulating AI systems that pose the greatest risks, may be a suitable strategy.
4. Different Regulatory Approaches Globally
Different countries and regions are adopting different approaches to regulating AI, reflecting their unique legal traditions, economic priorities, and societal values. These approaches can be broadly categorized as follows:
4.1 The European Union’s Risk-Based Approach:
The EU’s proposed AI Act is a risk-based regulatory framework that classifies AI systems based on their potential risks. High-risk AI systems, such as those used in critical infrastructure, healthcare, and law enforcement, are subject to strict requirements, including conformity assessments, transparency obligations, and human oversight. AI systems that pose minimal risk are subject to fewer regulations. This approach aims to strike a balance between promoting innovation and protecting fundamental rights and safety. The EU’s focus on human rights and ethical considerations is a defining characteristic of its regulatory approach.
4.2 The United States’ Sector-Specific Approach:
The United States is taking a more sector-specific approach to AI regulation, with different agencies regulating AI systems in their respective domains. For example, the FDA regulates AI-powered medical devices, while the FAA regulates AI-powered aviation systems. This approach allows for greater flexibility and adaptability, as regulators can tailor their approach to the specific risks and opportunities presented by AI in different sectors. However, it can also lead to fragmentation and inconsistency across different sectors. The US approach tends to be more market-driven, emphasizing innovation and economic growth.
4.3 China’s Government-Led Approach:
China is taking a more government-led approach to AI regulation, with the government playing a central role in setting standards and promoting the development of AI technology. China’s focus is on using AI to achieve its national strategic goals, such as economic growth, social stability, and technological leadership. China’s regulatory approach is often characterized by a greater emphasis on state control and less emphasis on individual rights.
4.4 Other Approaches:
Other countries, such as the United Kingdom, Canada, and Japan, are also developing their own approaches to AI regulation. The UK is focusing on a pro-innovation regulatory framework that is sector-specific and principles-based. Canada is emphasizing ethical considerations and human rights in its approach to AI regulation. Japan is promoting the development of AI technology while also addressing potential risks and ethical concerns.
4.5 International Cooperation:
Given the global nature of AI development and deployment, international cooperation is essential for creating a cohesive and effective global regulatory landscape for AI. Organizations such as the OECD, the G7, and the UN are working to promote international cooperation on AI regulation. However, achieving consensus on AI regulation is challenging, given the different priorities and values of different countries. Ensuring interoperability of regulatory frameworks and promoting data sharing across borders are key challenges in international cooperation on AI regulation.
5. Potential Impact of AI Regulation
The potential impact of AI regulation on innovation, fairness, and societal well-being is significant and multifaceted. Effective regulation can foster responsible innovation, protect fundamental rights, and promote public trust in AI systems. However, poorly designed regulation can stifle innovation, create barriers to entry, and hinder the development of beneficial AI applications.
5.1 Impact on Innovation:
Well-designed AI regulation can foster responsible innovation by providing clear guidelines and standards for the development and deployment of AI systems. This can reduce uncertainty for businesses and encourage investment in AI research and development. However, over-regulation can stifle innovation by increasing compliance costs, creating barriers to entry, and discouraging experimentation. Striking the right balance between promoting innovation and protecting society is crucial.
5.2 Impact on Fairness and Bias:
AI regulation can help to address algorithmic bias and promote fairness by requiring AI systems to be transparent, explainable, and non-discriminatory. This can help to ensure that AI systems are used in a manner that is consistent with societal values and promotes equal opportunity. However, achieving fairness in AI systems is a complex challenge, requiring careful attention to data quality, algorithm design, and ongoing monitoring of system performance.
5.3 Impact on Patient Safety (Example sector)
In sectors such as healthcare, AI regulation can help ensure patient safety by requiring AI-powered medical devices to be safe, effective, and reliable. This can help to prevent harm to patients caused by inaccurate or unreliable AI systems. The FDA, for example, plays a critical role in regulating AI-powered medical devices to ensure patient safety. However, striking the right balance between promoting innovation and protecting patient safety is essential.
5.4 Impact on Societal Well-being:
AI regulation can contribute to societal well-being by promoting the development and deployment of AI systems that benefit society as a whole. This includes AI systems that improve healthcare, education, transportation, and other essential services. However, it also requires addressing potential risks associated with AI, such as job displacement, privacy violations, and security vulnerabilities. Public engagement and stakeholder involvement are crucial for ensuring that AI regulation aligns with societal values and promotes the common good.
5.5 The Role of Standards:
Technical standards play a crucial role in facilitating AI regulation. Standards can provide a common framework for evaluating the performance, safety, and security of AI systems. Organizations such as the IEEE and ISO are developing standards for AI. The use of standards can help to reduce uncertainty and promote interoperability across different AI systems.
6. Recommendations and Conclusion
Regulating AI is a complex and ongoing process that requires a nuanced and adaptive approach. To effectively navigate the labyrinth of AI regulation, the following recommendations are proposed:
-
Adopt a Risk-Based Approach: Focus regulatory efforts on AI systems that pose the greatest risks to society. This allows for a more targeted and efficient allocation of resources.
-
Promote Transparency and Explainability: Require AI systems to be as transparent and explainable as possible. This will help to build trust in AI systems and facilitate accountability.
-
Address Algorithmic Bias: Implement measures to prevent and mitigate algorithmic bias. This requires careful attention to data quality, algorithm design, and ongoing monitoring of system performance.
-
Foster International Cooperation: Work with other countries to develop a cohesive and effective global regulatory landscape for AI.
-
Encourage Public Engagement: Engage with the public and stakeholders to ensure that AI regulation aligns with societal values and promotes the common good.
-
Support Research and Development: Invest in research and development to advance the understanding of AI and its potential impacts.
-
Develop AI-Specific Skills: Enhance workforce skills in AI-related fields to support the development and deployment of responsible AI systems.
In conclusion, AI regulation is essential for harnessing the benefits of AI while mitigating its potential risks. By adopting a balanced, adaptive, and internationally collaborative approach, policymakers can create a regulatory environment that fosters innovation, promotes fairness, and enhances societal well-being in the age of artificial intelligence. Navigating this intricate landscape requires continuous learning, adaptation, and a commitment to ethical principles.
References
- Brynjolfsson, E., & McAfee, A. (2014). The Second Machine Age: Work, Progress, and Prosperity in a Time of Brilliant Technologies. W. W. Norton & Company.
- Citron, D. K. (2008). Technological Due Process. Washington University Law Review, 85(6), 1249-1313.
- European Commission. (2021). Proposal for a Regulation Laying Down Harmonised Rules on Artificial Intelligence (Artificial Intelligence Act). COM(2021) 206 final.
- Goodman, B., & Flaxman, S. (2017). European Union regulations on algorithmic decision-making and a “right to explanation”. AI & Society, 32(3), 615-623.
- O’Neil, C. (2016). Weapons of Math Destruction: How Big Data Increases Inequality and Threatens Democracy. Crown.
- Pasquale, F. (2015). The Black Box Society: The Secret Algorithms That Control Money and Information. Harvard University Press.
- Shneiderman, B. (2020). Human-Centered AI. Oxford University Press.
- U.S. Food and Drug Administration. (2021). Artificial Intelligence and Machine Learning (AI/ML)-Enabled Medical Devices. Retrieved from https://www.fda.gov/medical-devices/software-medical-device-samd/artificial-intelligence-and-machine-learning-aiml-enabled-medical-devices
- UK Government. (2022). Establishing a pro-innovation approach to regulating AI. Retrieved from https://www.gov.uk/government/consultations/establishing-a-pro-innovation-approach-to-regulating-ai
This is a valuable overview of AI regulation. The discussion of differing global approaches highlights the complexity of creating unified standards. How can international bodies better facilitate consensus, given varying national priorities and ethical considerations around AI?
Thanks for your comment! I agree that harmonizing AI regulation globally is a challenge. Perhaps focusing on shared ethical principles and creating modular frameworks that allow for national adaptation could be a way forward. What specific mechanisms do you think could best facilitate this international consensus building?
Editor: MedTechNews.Uk
Thank you to our Sponsor Esdebe