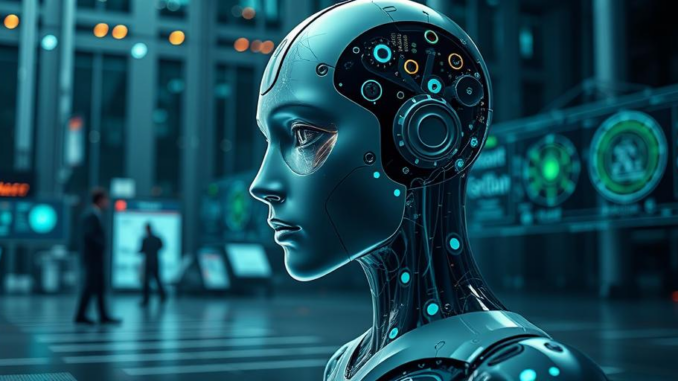
Navigating the Labyrinth: A Critical Analysis of Regulatory Oversight in the Age of Artificial Intelligence
Many thanks to our sponsor Esdebe who helped us prepare this research report.
Abstract
Artificial intelligence (AI) is rapidly permeating all facets of modern society, from healthcare and finance to transportation and criminal justice. This proliferation necessitates a robust and adaptable regulatory framework capable of fostering innovation while mitigating potential risks. This report provides a comprehensive analysis of existing and proposed AI regulations across various sectors, examining their effectiveness, potential impact on innovation, and the crucial role of ethical frameworks and international standards. We delve into specific challenges such as bias mitigation, data security, transparency, and accountability, assessing the suitability of current approaches and proposing avenues for improvement. Furthermore, we explore the evolving landscape of liability in AI-driven systems and discuss the responsibilities of government agencies and private sector stakeholders in ensuring safe and ethical AI deployment. This research underscores the urgent need for a dynamic and collaborative approach to AI regulation that balances the benefits of technological advancement with the protection of fundamental rights and societal well-being.
Many thanks to our sponsor Esdebe who helped us prepare this research report.
1. Introduction: The Imperative of AI Regulation
Artificial intelligence (AI), encompassing machine learning, deep learning, and related technologies, presents unprecedented opportunities for societal advancement. However, these opportunities are intertwined with significant risks, necessitating careful consideration and proactive regulatory intervention. The unregulated deployment of AI systems can lead to biased outcomes, privacy violations, security breaches, and a lack of accountability, potentially undermining public trust and hindering broader adoption [1]. This report addresses the crucial question of how to effectively regulate AI to maximize its benefits while mitigating its potential harms.
The core challenge lies in striking a balance between fostering innovation and ensuring responsible AI development and deployment. Overly restrictive regulations can stifle creativity and impede progress, while insufficient oversight can lead to unintended consequences and erode public confidence. The regulatory landscape must be dynamic and adaptable, capable of evolving alongside the rapid advancements in AI technology. This requires a multi-faceted approach, encompassing legal frameworks, ethical guidelines, technical standards, and collaborative partnerships between government, industry, and academia.
This report aims to provide a critical analysis of the current state of AI regulation, examining existing and proposed frameworks across various sectors. We will explore the effectiveness of these regulations, their potential impact on innovation, and the key challenges that must be addressed to ensure responsible AI development and deployment. The analysis will incorporate international perspectives, highlighting best practices and identifying areas where greater harmonization is needed. Finally, we will propose recommendations for a more robust and adaptable regulatory framework that can effectively navigate the complex challenges of the AI age.
Many thanks to our sponsor Esdebe who helped us prepare this research report.
2. Existing Regulatory Landscape: A Patchwork of Approaches
The current regulatory landscape for AI is characterized by a fragmented and sector-specific approach. While some jurisdictions have enacted comprehensive AI laws, others rely on existing regulations to address AI-related risks on a case-by-case basis. This lack of harmonization creates uncertainty for businesses operating across borders and can hinder the development of consistent ethical standards.
2.1 Sector-Specific Regulations:
Many existing regulations relevant to AI are embedded within sector-specific frameworks. For example, in the healthcare sector, regulations governing medical devices, data privacy (e.g., HIPAA in the US, GDPR in Europe), and patient safety apply to AI-powered diagnostic tools and treatment algorithms. Similarly, in the financial sector, regulations related to anti-money laundering (AML), fraud detection, and consumer protection govern the use of AI in credit scoring, algorithmic trading, and automated customer service [2].
These sector-specific regulations often lack specific provisions addressing the unique challenges posed by AI. For instance, existing data privacy laws may not adequately address the complexities of algorithmic bias or the use of AI for predictive policing. This necessitates the development of more tailored regulations that specifically address the risks associated with AI in each sector.
2.2 Comprehensive AI Regulations:
The European Union (EU) has taken a leading role in developing comprehensive AI regulations with its proposed AI Act [3]. This Act adopts a risk-based approach, categorizing AI systems based on their potential to cause harm and imposing corresponding regulatory requirements. High-risk AI systems, such as those used in critical infrastructure, education, and law enforcement, are subject to stringent requirements related to transparency, accountability, and human oversight. The AI Act also prohibits certain AI practices deemed inherently harmful, such as real-time biometric identification in public spaces.
The EU AI Act has been subject to much debate, with concerns raised about its potential impact on innovation and competitiveness. However, it represents a significant step towards establishing a comprehensive legal framework for AI regulation. Other jurisdictions, such as Canada and Singapore, are also exploring similar approaches.
2.3 Key Challenges in the Existing Landscape:
- Lack of Harmonization: The fragmented nature of the regulatory landscape creates uncertainty for businesses and hinders the development of consistent ethical standards.
- Adaptability: Existing regulations may not be flexible enough to keep pace with the rapid advancements in AI technology.
- Enforcement: Enforcing AI regulations can be challenging due to the complexity of AI systems and the lack of technical expertise among regulators.
- Bias Mitigation: Many existing regulations do not adequately address the issue of algorithmic bias, which can perpetuate and amplify existing societal inequalities.
Many thanks to our sponsor Esdebe who helped us prepare this research report.
3. Ethical Frameworks and International Standards
Beyond legal regulations, ethical frameworks and international standards play a crucial role in guiding the responsible development and deployment of AI. These frameworks provide principles and guidelines for ensuring that AI systems are aligned with human values and societal goals.
3.1 Core Ethical Principles:
Several core ethical principles underpin the development of responsible AI. These include:
- Beneficence: AI systems should be designed to benefit humanity and promote well-being.
- Non-maleficence: AI systems should avoid causing harm or unintended negative consequences.
- Autonomy: AI systems should respect human autonomy and enable individuals to make informed decisions.
- Justice: AI systems should be fair and equitable, avoiding discrimination and bias.
- Transparency: AI systems should be transparent and explainable, allowing users to understand how they work and how decisions are made.
- Accountability: There should be clear lines of responsibility for the development and deployment of AI systems.
3.2 Examples of Ethical Frameworks:
Numerous organizations have developed ethical frameworks for AI, including:
- The Asilomar AI Principles: A set of 23 principles developed at the Asilomar Conference on Beneficial AI in 2017 [4].
- The IEEE Ethically Aligned Design: A comprehensive framework for ethical AI design developed by the IEEE Standards Association [5].
- The OECD Principles on AI: A set of principles for the responsible stewardship of trustworthy AI adopted by the OECD in 2019 [6].
3.3 International Standards:
International standards organizations, such as ISO and IEC, are developing standards for various aspects of AI, including data quality, risk management, and explainability. These standards provide a common language and framework for ensuring the quality and reliability of AI systems.
3.4 Challenges in Implementing Ethical Frameworks:
While ethical frameworks provide valuable guidance, implementing them in practice can be challenging. Some of the key challenges include:
- Lack of Specificity: Ethical principles are often abstract and require interpretation and adaptation to specific contexts.
- Conflicting Values: Ethical principles can sometimes conflict with each other, requiring difficult trade-offs.
- Enforcement: Ethical frameworks are often voluntary and lack formal enforcement mechanisms.
- Cultural Differences: Ethical values can vary across cultures, making it difficult to develop universally applicable frameworks.
Many thanks to our sponsor Esdebe who helped us prepare this research report.
4. The Role of Government Agencies: Ensuring Patient Safety and Data Security
Government agencies play a crucial role in ensuring the safe and responsible development and deployment of AI. Their responsibilities include:
4.1 Regulatory Oversight:
Government agencies are responsible for developing and enforcing regulations that govern the use of AI in various sectors. This includes setting standards for data privacy, security, and transparency, as well as establishing mechanisms for monitoring and enforcement.
4.2 Funding and Research:
Government agencies can support AI research and development through funding grants and partnerships with academia and industry. This can help to accelerate innovation and ensure that AI is developed in a responsible and ethical manner.
4.3 Standardization and Certification:
Government agencies can promote the development of standards and certification programs for AI systems. This can help to ensure that AI systems meet certain quality and safety requirements.
4.4 Education and Training:
Government agencies can support education and training programs to develop the skills and expertise needed to effectively regulate and oversee AI. This includes training regulators, developers, and users of AI systems.
4.5 Public Awareness:
Government agencies can raise public awareness about the benefits and risks of AI and promote informed discussions about its ethical and societal implications.
4.6 Examples of Government Initiatives:
- The National Institute of Standards and Technology (NIST) AI Risk Management Framework: A voluntary framework to help organizations manage risks to individuals, organizations, and society associated with AI [7].
- The FDA’s Digital Health Center of Excellence: A center dedicated to advancing digital health technologies, including AI-powered medical devices [8].
- The FTC’s guidance on AI and advertising: Guidance to help businesses avoid deceptive or unfair practices when using AI in advertising [9].
Many thanks to our sponsor Esdebe who helped us prepare this research report.
5. Liability Issues: Assigning Responsibility in AI-Driven Systems
The increasing use of AI systems raises complex questions about liability. When an AI system causes harm, who is responsible? Is it the developer of the AI, the manufacturer of the hardware, the user of the system, or the AI itself?
5.1 Traditional Liability Frameworks:
Traditional liability frameworks, such as negligence and product liability, may not be well-suited to address the unique challenges posed by AI. These frameworks typically require a clear causal link between the actions of a specific actor and the harm that occurred. However, in the case of AI systems, it can be difficult to establish such a link due to the complexity of the algorithms and the autonomous nature of the systems.
5.2 Emerging Legal Theories:
Several new legal theories are emerging to address the liability issues raised by AI. These include:
- Strict Liability: Imposing liability on the developers or manufacturers of AI systems regardless of fault.
- Algorithmic Negligence: Holding developers liable for failing to adequately test and monitor their AI systems.
- Duty of Care: Imposing a duty of care on users of AI systems to ensure that they are used responsibly.
5.3 Challenges in Assigning Liability:
Assigning liability in AI-driven systems is challenging due to several factors:
- Complexity: AI systems can be highly complex and opaque, making it difficult to understand how they work and why they made a particular decision.
- Autonomy: AI systems can operate autonomously, making it difficult to predict their behavior and prevent them from causing harm.
- Data Dependency: AI systems are heavily reliant on data, and biased or inaccurate data can lead to biased or inaccurate outcomes.
- Human-Machine Interaction: AI systems often interact with humans, and the actions of both the AI and the human can contribute to the harm that occurred.
5.4 The Need for a Clear Legal Framework:
A clear and well-defined legal framework is needed to address the liability issues raised by AI. This framework should:
- Establish clear lines of responsibility for the development, deployment, and use of AI systems.
- Provide guidance on how to assess and mitigate the risks associated with AI.
- Offer mechanisms for resolving disputes and compensating victims of AI-related harm.
Many thanks to our sponsor Esdebe who helped us prepare this research report.
6. Addressing Bias and Discrimination in AI Systems
One of the most significant ethical and societal challenges posed by AI is the potential for bias and discrimination. AI systems can perpetuate and amplify existing societal inequalities if they are trained on biased data or designed with biased algorithms.
6.1 Sources of Bias in AI Systems:
Bias can creep into AI systems at various stages of the development and deployment process:
- Data Bias: The data used to train AI systems may reflect historical biases or stereotypes.
- Algorithmic Bias: The algorithms themselves may be biased, either intentionally or unintentionally.
- Selection Bias: The way in which data is collected or selected may introduce bias.
- Interpretation Bias: The way in which the results of AI systems are interpreted may be biased.
6.2 Consequences of Bias in AI Systems:
Bias in AI systems can have a wide range of negative consequences, including:
- Discrimination: AI systems can discriminate against certain groups of people based on race, gender, religion, or other protected characteristics.
- Unfairness: AI systems can produce unfair or unjust outcomes.
- Loss of Trust: Bias in AI systems can erode public trust in the technology.
- Perpetuation of Inequalities: AI systems can perpetuate and amplify existing societal inequalities.
6.3 Strategies for Mitigating Bias in AI Systems:
Several strategies can be used to mitigate bias in AI systems:
- Data Auditing: Carefully auditing the data used to train AI systems to identify and correct biases.
- Algorithmic Fairness Techniques: Using algorithmic fairness techniques to develop algorithms that are less biased.
- Diversity and Inclusion: Promoting diversity and inclusion in the AI workforce.
- Transparency and Explainability: Making AI systems more transparent and explainable so that biases can be more easily detected.
- Human Oversight: Implementing human oversight mechanisms to ensure that AI systems are used fairly and responsibly.
6.4 The Importance of Continuous Monitoring and Evaluation:
Mitigating bias in AI systems is an ongoing process that requires continuous monitoring and evaluation. AI systems should be regularly audited to ensure that they are not producing biased outcomes. When biases are detected, they should be promptly corrected.
Many thanks to our sponsor Esdebe who helped us prepare this research report.
7. Conclusion: Towards a Dynamic and Collaborative Approach
Regulating AI effectively requires a dynamic and collaborative approach that balances the benefits of technological advancement with the protection of fundamental rights and societal well-being. The current regulatory landscape is fragmented and often inadequate to address the unique challenges posed by AI. A more comprehensive and adaptable framework is needed, encompassing legal regulations, ethical guidelines, technical standards, and collaborative partnerships between government, industry, and academia.
The EU AI Act represents a significant step towards a more comprehensive regulatory framework, but its potential impact on innovation remains a concern. Other jurisdictions should learn from the EU’s experience and develop their own tailored approaches to AI regulation. Ethical frameworks and international standards play a crucial role in guiding the responsible development and deployment of AI, but they need to be translated into concrete actions and enforced effectively.
Government agencies must play a proactive role in ensuring patient safety, data security, and the mitigation of bias in AI systems. This includes investing in research, developing standards and certification programs, and promoting public awareness about the benefits and risks of AI. Addressing the liability issues raised by AI is essential for building trust in the technology and ensuring that victims of AI-related harm are adequately compensated.
Ultimately, the success of AI regulation depends on a collaborative effort involving all stakeholders. By working together, government, industry, academia, and civil society can create a regulatory framework that fosters innovation, protects fundamental rights, and ensures that AI benefits all of humanity.
Many thanks to our sponsor Esdebe who helped us prepare this research report.
References
[1] O’Neil, C. (2016). Weapons of Math Destruction: How Big Data Increases Inequality and Threatens Democracy. Crown.
[2] Barasz, M., & Jullien, B. (2021). Algorithmic Discrimination in Credit Markets. The Journal of Law, Economics, & Organization, 37(3), 489-521.
[3] European Commission. (2021). Proposal for a Regulation of the European Parliament and of the Council Laying Down Harmonised Rules on Artificial Intelligence (Artificial Intelligence Act) and Amending Certain Union Legislative Acts. COM(2021) 206 final.
[4] Future of Life Institute. (2017). Asilomar AI Principles. https://futureoflife.org/ai-principles/
[5] IEEE Standards Association. (2019). Ethically Aligned Design: A Vision for Prioritizing Human Well-being with Autonomous and Intelligent Systems. IEEE.
[6] OECD. (2019). OECD Principles on AI. https://www.oecd.org/going-digital/ai/principles/
[7] National Institute of Standards and Technology (NIST). (2023). AI Risk Management Framework. https://www.nist.gov/itl/ai-risk-management-framework
[8] U.S. Food and Drug Administration (FDA). Digital Health Center of Excellence. https://www.fda.gov/medical-devices/digital-health-center-excellence/digital-health-center-excellence
[9] Federal Trade Commission (FTC). Using Artificial Intelligence and Algorithms. https://www.ftc.gov/news-events/topics/protecting-consumers/artificial-intelligence-algorithms
The report rightly emphasizes the need for adaptable AI regulation. Sector-specific applications, like healthcare and finance, present unique challenges. Harmonizing international standards could foster innovation while addressing ethical considerations like bias and data security, promoting responsible AI deployment across borders.
Thanks for your insightful comment! The challenge of harmonizing international standards is definitely a key point. Exploring how different nations balance innovation with ethical considerations in their AI regulation would be a fascinating area for further research. It’s great to get different perspectives.
Editor: MedTechNews.Uk
Thank you to our Sponsor Esdebe
The report highlights the challenge of algorithmic bias. What methods, beyond data auditing and algorithmic fairness techniques, can be employed to ensure ongoing mitigation of newly introduced biases in continuously learning AI systems, especially in sensitive sectors?