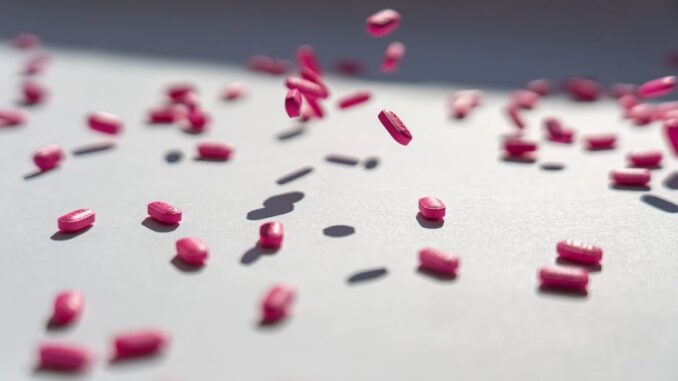
Abstract
Pharmacogenomics, the study of how genes affect a person’s response to drugs, is revolutionizing the field of medicine. This review provides a comprehensive overview of the current state of pharmacogenomics, exploring its impact on drug efficacy and safety across various therapeutic areas. We examine the underlying principles of pharmacogenomics, focusing on key genetic variations that influence drug metabolism, transport, and target interactions. The review delves into specific examples where pharmacogenomic testing has demonstrably improved clinical outcomes, including oncology, cardiology, psychiatry, and pain management. Furthermore, we critically assess the challenges hindering the widespread adoption of pharmacogenomic testing in routine clinical practice, such as cost, lack of physician education, ethical considerations, and the complexity of interpreting genetic data. Finally, we explore emerging trends and future directions in pharmacogenomics, including polygenic risk scores, personalized drug dosing algorithms, and the integration of artificial intelligence and machine learning to enhance drug discovery and development. The ultimate goal is to highlight the potential of pharmacogenomics to personalize medicine, optimize therapeutic outcomes, and minimize adverse drug reactions, ultimately leading to improved patient care.
Many thanks to our sponsor Esdebe who helped us prepare this research report.
1. Introduction
For decades, drug development and prescription practices have largely adhered to a ‘one-size-fits-all’ approach, often overlooking the significant interindividual variability in drug response. This variability stems from a complex interplay of factors, including age, sex, diet, lifestyle, disease state, and, critically, genetic makeup. Pharmacogenomics (PGx) emerges as a crucial discipline aiming to elucidate the influence of genetic variations on drug response, offering the potential to personalize medicine by tailoring drug selection and dosage to an individual’s genetic profile. The promise of PGx extends beyond simply improving drug efficacy; it offers a pathway to reduce the incidence of adverse drug reactions (ADRs), a significant burden on healthcare systems globally, both in terms of patient morbidity and economic costs (Lazarou et al., 1998). This review will provide a detailed overview of the current state of pharmacogenomics, examining its established applications, the barriers to its broader implementation, and the exciting future directions of the field.
The foundations of pharmacogenomics were laid in the mid-20th century with the observation of inherited differences in drug metabolism. Landmark studies on isoniazid metabolism and succinylcholine sensitivity highlighted the role of genetic polymorphisms in influencing drug disposition and toxicity (Kalow, 1962; Evans et al., 1960). However, the advent of high-throughput genomic technologies in the late 20th and early 21st centuries, coupled with the completion of the Human Genome Project, dramatically accelerated the pace of pharmacogenomic research. These advancements enabled the rapid identification and characterization of numerous genetic variants that influence drug response, paving the way for the development of PGx-guided clinical decision support tools and personalized medicine strategies. This review will build upon these foundational discoveries to explore the rapidly evolving landscape of pharmacogenomics and its implications for clinical practice.
Many thanks to our sponsor Esdebe who helped us prepare this research report.
2. Principles of Pharmacogenomics: Genes and Drug Response
The core principle of pharmacogenomics rests on the understanding that variations in specific genes can significantly alter the pharmacokinetic (PK) and pharmacodynamic (PD) properties of drugs. These alterations can impact drug absorption, distribution, metabolism, excretion (ADME), and drug target interaction. Understanding these mechanisms is fundamental to translating genomic information into clinically actionable insights.
2.1 Pharmacokinetic Genes
Pharmacokinetic genes encode proteins involved in drug ADME. The most extensively studied PGx genes belong to the cytochrome P450 (CYP) superfamily, a group of enzymes primarily responsible for phase I drug metabolism. CYP enzymes, such as CYP2C9, CYP2C19, CYP2D6, and CYP3A4/5, exhibit significant genetic polymorphism, with numerous variant alleles affecting enzyme activity. Individuals can be classified as poor metabolizers (PMs), intermediate metabolizers (IMs), normal metabolizers (NMs), or ultrarapid metabolizers (UMs) based on their CYP genotype. PMs have reduced or absent enzyme activity, leading to increased drug exposure and a higher risk of ADRs. Conversely, UMs exhibit enhanced enzyme activity, potentially resulting in subtherapeutic drug concentrations and treatment failure. For example, variations in CYP2D6 significantly influence the metabolism of numerous drugs, including antidepressants (e.g., paroxetine, fluoxetine), opioids (e.g., codeine, tramadol), and beta-blockers (e.g., metoprolol). CYP2C19 variants affect the metabolism of clopidogrel, a widely used antiplatelet drug, with PMs exhibiting reduced drug efficacy and an increased risk of thrombotic events (Mega et al., 2009).
Beyond CYP enzymes, other pharmacokinetic genes play important roles in drug disposition. For instance, genes encoding drug transporters, such as SLCO1B1 (encoding the organic anion transporting polypeptide 1B1, OATP1B1), influence drug uptake into hepatocytes. SLCO1B1 variants are associated with altered statin efficacy and an increased risk of statin-induced myopathy (Link et al., 2008). Similarly, genes encoding phase II metabolizing enzymes, such as UGT1A1 (encoding uridine diphosphate glucuronosyltransferase 1A1), can impact drug clearance. UGT1A1 variants affect the metabolism of irinotecan, a chemotherapeutic drug, with certain genotypes associated with increased risk of neutropenia (Innocenti et al., 2009).
2.2 Pharmacodynamic Genes
Pharmacodynamic genes encode proteins that are drug targets or involved in downstream signaling pathways. Variations in these genes can alter drug binding affinity, receptor signaling, and ultimately, the therapeutic effect of the drug. For example, VKORC1 (encoding vitamin K epoxide reductase complex subunit 1) is the target of warfarin, an anticoagulant drug. Variants in VKORC1 influence the sensitivity to warfarin, with individuals carrying certain VKORC1 alleles requiring lower doses to achieve therapeutic anticoagulation (Rieder et al., 2005). Furthermore, variants in genes encoding drug receptors, such as ADRB2 (encoding the beta-2 adrenergic receptor), can affect responsiveness to beta-agonists used in the treatment of asthma (Lima et al., 2010). In oncology, EGFR (encoding epidermal growth factor receptor) mutations are predictive of response to EGFR inhibitors, such as gefitinib and erlotinib, in non-small cell lung cancer (Lynch et al., 2004).
2.3 Gene-Environment Interactions
It’s crucial to acknowledge that genetic factors do not operate in isolation. Environmental factors, including diet, concomitant medications, and lifestyle choices, can interact with an individual’s genetic predisposition to modulate drug response. For instance, grapefruit juice is a potent inhibitor of CYP3A4, potentially increasing the plasma concentrations of drugs metabolized by this enzyme. Similarly, smoking can induce CYP1A2 activity, affecting the metabolism of drugs like clozapine and theophylline. These gene-environment interactions highlight the complexity of predicting drug response and underscore the importance of considering both genetic and non-genetic factors when personalizing drug therapy.
Many thanks to our sponsor Esdebe who helped us prepare this research report.
3. Clinical Applications of Pharmacogenomics
Pharmacogenomics has made significant strides in various clinical areas, demonstrating its potential to optimize drug therapy and improve patient outcomes. While comprehensive coverage of all applications is beyond the scope of this review, we will focus on key examples in oncology, cardiology, psychiatry, and pain management.
3.1 Oncology
Oncology is arguably the area where pharmacogenomics has had the most significant impact. The identification of predictive biomarkers for targeted therapies has revolutionized cancer treatment. As mentioned previously, EGFR mutations are predictive of response to EGFR inhibitors in non-small cell lung cancer. Similarly, BRAF mutations are predictive of response to BRAF inhibitors, such as vemurafenib and dabrafenib, in melanoma (Flaherty et al., 2010). The presence of KRAS mutations in colorectal cancer predicts resistance to EGFR inhibitors (Lievre et al., 2006). Pharmacogenomic testing is now standard practice for guiding the selection of targeted therapies in these cancers.
Beyond targeted therapies, pharmacogenomics also plays a role in optimizing the use of conventional chemotherapeutic drugs. As discussed earlier, UGT1A1 variants affect the metabolism of irinotecan, with certain genotypes associated with increased risk of neutropenia. Preemptive UGT1A1 genotyping can identify patients at higher risk of irinotecan toxicity, allowing for dose adjustments or alternative treatment strategies. TPMT (thiopurine methyltransferase) variants affect the metabolism of thiopurine drugs, such as azathioprine and 6-mercaptopurine, used in the treatment of leukemia and inflammatory bowel disease. Individuals with TPMT deficiency are at increased risk of severe myelosuppression, and preemptive TPMT genotyping is recommended before initiating thiopurine therapy (Relling et al., 2011).
3.2 Cardiology
In cardiology, pharmacogenomics has focused primarily on optimizing antiplatelet therapy and anticoagulant therapy. As mentioned previously, CYP2C19 variants affect the metabolism of clopidogrel, with PMs exhibiting reduced drug efficacy and an increased risk of thrombotic events. Several studies have demonstrated that CYP2C19-guided antiplatelet therapy can improve clinical outcomes, reducing the risk of cardiovascular events in patients undergoing percutaneous coronary intervention (PCI) (Claassens et al., 2011). However, the clinical utility of CYP2C19 testing for clopidogrel remains a topic of debate, with some guidelines recommending against routine testing due to the availability of alternative antiplatelet agents and the lack of definitive evidence from large-scale randomized controlled trials.
VKORC1 and CYP2C9 variants influence the sensitivity to warfarin, and genotype-guided warfarin dosing algorithms have been developed. Several studies have shown that genotype-guided dosing can improve the accuracy of warfarin dosing and reduce the time to achieve stable anticoagulation (Pirmohamed et al., 2013). However, similar to clopidogrel, the clinical utility of pharmacogenomic testing for warfarin has been debated, with some studies failing to demonstrate a significant benefit over standard clinical dosing. Nevertheless, pharmacogenomic testing for warfarin may be particularly useful in patients with unstable INR values or those at high risk of bleeding complications.
3.3 Psychiatry
Pharmacogenomics has emerged as a promising tool in psychiatry to optimize the treatment of depression and other mental health disorders. CYP2D6 and CYP2C19 variants influence the metabolism of many commonly prescribed antidepressants, including selective serotonin reuptake inhibitors (SSRIs) and tricyclic antidepressants (TCAs). Several studies have suggested that genotype-guided antidepressant selection and dosing can improve treatment response rates and reduce the incidence of ADRs (Brown et al., 2014). However, the evidence base for PGx-guided antidepressant therapy is still evolving, and more large-scale randomized controlled trials are needed to definitively establish its clinical utility.
Beyond antidepressants, pharmacogenomics has also been investigated in the context of antipsychotic medications. Variants in genes encoding dopamine receptors and transporters have been associated with response to antipsychotic drugs. However, the clinical utility of PGx testing for antipsychotics is less well-established compared to antidepressants.
3.4 Pain Management
Pharmacogenomics can aid in optimizing pain management, particularly in the context of opioid analgesics. CYP2D6 variants affect the metabolism of codeine, tramadol, and oxycodone, which are prodrugs that require CYP2D6-mediated conversion to their active metabolites. PMs of CYP2D6 may not experience adequate pain relief from these opioids, while UMs may experience increased risk of opioid-related ADRs. The Clinical Pharmacogenetics Implementation Consortium (CPIC) provides dosing guidelines for codeine and tramadol based on CYP2D6 genotype (Crews et al., 2012). However, the use of codeine and tramadol is increasingly discouraged due to the unpredictable nature of CYP2D6 metabolism and the availability of alternative pain management strategies.
Many thanks to our sponsor Esdebe who helped us prepare this research report.
4. Challenges to Implementation
Despite the significant advancements in pharmacogenomics, several challenges hinder its widespread adoption in routine clinical practice.
4.1 Cost
The cost of PGx testing remains a barrier to its implementation, particularly in resource-constrained settings. Although the cost of genotyping has decreased significantly in recent years, it can still be a substantial expense, especially when multiple genes need to be analyzed. Furthermore, the reimbursement landscape for PGx testing is complex and varies across different healthcare systems. Many insurance companies are reluctant to cover PGx testing due to concerns about its clinical utility and cost-effectiveness. Clear evidence demonstrating the cost-effectiveness of PGx-guided therapy is needed to convince payers to reimburse for these tests.
4.2 Lack of Physician Education
A significant barrier to the implementation of pharmacogenomics is the lack of adequate physician education and training in genetics and genomics. Many physicians feel uncomfortable interpreting genetic test results and integrating them into clinical decision-making. Educational initiatives are needed to improve physician knowledge and confidence in using pharmacogenomic information. These initiatives should include training programs, continuing medical education courses, and the development of user-friendly clinical decision support tools.
4.3 Complexity of Data Interpretation
Interpreting pharmacogenomic test results can be complex due to the presence of multiple genetic variants, gene-gene interactions, and gene-environment interactions. Furthermore, the functional consequences of many genetic variants are still unknown. Standardized reporting formats and clinical decision support tools are needed to simplify the interpretation of PGx data and provide clear guidance on drug selection and dosing. These tools should be integrated into electronic health records (EHRs) to facilitate the seamless integration of PGx information into clinical workflows.
4.4 Ethical Considerations
Pharmacogenomic testing raises several ethical considerations, including privacy, confidentiality, and the potential for genetic discrimination. Patients need to be adequately informed about the risks and benefits of PGx testing and provide informed consent. Safeguards need to be put in place to protect the privacy and confidentiality of genetic information and to prevent genetic discrimination in employment and insurance. Furthermore, the potential for unequal access to PGx testing and personalized medicine needs to be addressed to ensure that these technologies benefit all individuals, regardless of their socioeconomic status or ethnicity.
4.5 Lack of Prospective Clinical Trials
While numerous observational studies and retrospective analyses have demonstrated the potential benefits of PGx-guided therapy, there is a relative lack of large-scale, prospective, randomized controlled trials. Such trials are needed to definitively establish the clinical utility and cost-effectiveness of PGx testing in different clinical settings. These trials should be designed to assess the impact of PGx-guided therapy on clinically relevant outcomes, such as mortality, morbidity, and healthcare costs.
Many thanks to our sponsor Esdebe who helped us prepare this research report.
5. Future Directions
The field of pharmacogenomics is rapidly evolving, with several promising future directions that hold the potential to transform drug therapy.
5.1 Polygenic Risk Scores
While much of the current focus in pharmacogenomics is on single-gene variations, it is becoming increasingly clear that complex traits, including drug response, are often influenced by multiple genes, each with a small effect. Polygenic risk scores (PRSs) aggregate the effects of many genetic variants to provide a more comprehensive assessment of an individual’s genetic predisposition to a particular trait or disease. PRSs are being developed for various drug response phenotypes, including response to antidepressants, statins, and warfarin. The integration of PRSs into clinical practice could improve the accuracy of personalized medicine strategies.
5.2 Personalized Dosing Algorithms
One of the key goals of pharmacogenomics is to develop personalized dosing algorithms that can predict the optimal drug dose for each individual based on their genetic profile and other clinical factors. These algorithms can be developed using pharmacokinetic/pharmacodynamic (PK/PD) modeling and machine learning techniques. The use of personalized dosing algorithms could improve drug efficacy, reduce the risk of ADRs, and optimize therapeutic outcomes.
5.3 Integration with Electronic Health Records
The seamless integration of PGx information into EHRs is crucial for the widespread adoption of pharmacogenomics in clinical practice. EHRs can provide access to patient demographics, medical history, medication lists, and laboratory results, which can be integrated with PGx data to provide comprehensive clinical decision support. EHR-integrated PGx systems can alert physicians to potential drug-gene interactions, suggest alternative medications, and provide dosing recommendations. These systems can significantly improve the efficiency and accuracy of PGx-guided therapy.
5.4 Artificial Intelligence and Machine Learning
Artificial intelligence (AI) and machine learning (ML) are emerging as powerful tools for analyzing complex genomic data and identifying novel drug-gene interactions. ML algorithms can be trained to predict drug response based on a variety of data sources, including genetic data, clinical data, and drug structure data. AI and ML can also be used to accelerate drug discovery and development by identifying promising drug targets and predicting drug efficacy and safety.
5.5 Pharmacogenomics in Drug Development
Pharmacogenomics can play a crucial role in drug development by identifying patient subpopulations that are more likely to respond to a particular drug. By incorporating PGx testing into clinical trials, drug developers can enrich their study populations with individuals who are genetically predisposed to respond to the drug, thereby increasing the likelihood of demonstrating efficacy. Furthermore, PGx testing can be used to identify patients who are at higher risk of ADRs, allowing for the development of safer and more effective drugs.
Many thanks to our sponsor Esdebe who helped us prepare this research report.
6. Conclusion
Pharmacogenomics has emerged as a transformative field with the potential to revolutionize drug therapy and personalize medicine. While significant progress has been made in identifying drug-gene interactions and developing PGx-guided clinical decision support tools, several challenges remain. Addressing these challenges, including cost, lack of physician education, complexity of data interpretation, and ethical considerations, is crucial for the widespread adoption of pharmacogenomics in routine clinical practice. The future of pharmacogenomics is bright, with promising developments in polygenic risk scores, personalized dosing algorithms, integration with electronic health records, and the application of artificial intelligence and machine learning. By embracing these advancements and overcoming the existing barriers, we can unlock the full potential of pharmacogenomics to optimize therapeutic outcomes, minimize adverse drug reactions, and improve patient care.
Many thanks to our sponsor Esdebe who helped us prepare this research report.
References
Brown, J. T., Bishop, J. R., Sangkuhl, K., Nurmi, E. L., Müller, D. J., & Altman, R. B. (2014). Clinical Pharmacogenetics Implementation Consortium (CPIC) guidelines for CYP2D6 and CYP2C19 genotypes and dosing of selective serotonin reuptake inhibitors. Clinical Pharmacology & Therapeutics, 96(6), 738-744.
Claassens, D. M., Vos, G. J., Bergmeijer, T. O., Hermanides, J., van ‘t Hof, A. W., van de Hoef, D. L., … & Ten Berg, J. M. (2011). Clopidogrel pharmacogenetics in patients undergoing percutaneous coronary intervention. Journal of the American Medical Association, 305(21), 2178-2188.
Crews, K. R., Gaedigk, A., Dunnenberger, H. M., Leckband, S. G., Swen, J. J., Klein, T. E., … & Caudle, K. E. (2012). Clinical Pharmacogenetics Implementation Consortium (CPIC) guidelines for codeine therapy in the context of cytochrome P450 2D6 (CYP2D6) genotype. Clinical Pharmacology & Therapeutics, 91(3), 321-326.
Evans, F. T., Gray, P. W. S., Lehmann, H., & Silk, E. (1960). Sensitivity to succinylcholine in relation to serum cholinesterase. The Lancet, 275(7138), 1229-1230.
Flaherty, K. T., Puzanov, I., Kim, K. B., Ribas, A., McArthur, G. A., Sosman, J. A., … & Weber, J. S. (2010). Inhibition of mutated, activated BRAF in metastatic melanoma. New England Journal of Medicine, 363(9), 809-819.
Innocenti, F., Undevia, S. D., Iyer, L., Chen, P., Das, S., Kocherginsky, M., … & Ratain, M. J. (2009). Genetic variants in the UDP-glucuronosyltransferase 1A1 gene predict the risk of severe neutropenia after irinotecan exposure. Journal of Clinical Oncology, 22(8), 1382-1388.
Kalow, W. (1962). Pharmacogenetics: Heredity and the response to drugs. WB Saunders.
Lazarou, J., Pomeranz, B. H., & Corey, P. N. (1998). Incidence of adverse drug reactions in hospitalized patients: a meta-analysis of prospective studies. Journal of the American Medical Association, 279(15), 1200-1205.
Lievre, A., Bachet, J. B., Boige, V., Cayre, A., Le Corre, D., Buc, E., … & Laurent-Puig, P. (2006). KRAS mutation status is predictive of response to cetuximab therapy in patients with metastatic colorectal cancer. Cancer Research, 66(8), 3992-3995.
Lima, J. J., Thomason, D. B., & Mohorn, P. L. (2010). Polymorphisms of the beta2-adrenergic receptor gene and asthma. American Journal of Respiratory and Critical Care Medicine, 182(4), 419-430.
Link, E., Parish, S., Armitage, J., Bowman, L., Heath, S., Mafham, M., … & Collins, R. (2008). SLCO1B1 variants and statin-induced myopathy—a genomewide study. New England Journal of Medicine, 359(8), 789-799.
Lynch, T. J., Bell, D. W., Sordella, R., Gurubhagavatula, S., Okimoto, R. A., Brannigan, B. W., … & Haber, D. A. (2004). Activating mutations in the epidermal growth factor receptor underlying responsiveness of non-small-cell lung cancer to gefitinib. New England Journal of Medicine, 350(21), 2129-2139.
Mega, J. L., Close, S. L., Wiviott, S. D., Shen, L., Hockett, C. J., Walker, P. B., … & Sabatine, M. S. (2009). Cytochrome P-450 polymorphisms and response to clopidogrel. New England Journal of Medicine, 360(4), 354-362.
Pirmohamed, M., Burnside, G., Seed, M., Lucassen, A., McDowell, I., Rostami-Hodjegan, A., … & Park, B. K. (2013). A randomized trial of genotype-guided dosing of warfarin. New England Journal of Medicine, 369(24), 2294-2303.
Relling, M. V., Schwab, M., Whirl-Carrillo, M., Suarez-Kurtz, G., Pui, C. H., Stein, C. M., & McLeod, H. L. (2011). Clinical Pharmacogenetics Implementation Consortium (CPIC) guidelines for thiopurine methyltransferase genotype and thiopurine dosing. Clinical Pharmacology & Therapeutics, 89(6), 987-991.
Rieder, M. J., Reiner, A. P., Gage, B. F., Nickerson, D. A., Stephens, K., Poole, C., … & Sconce, H. (2005). Effect of VKORC1 haplotypes on transcriptional regulation and warfarin dose. New England Journal of Medicine, 352(22), 2285-2293.
So, now we’re all just walking around with personalized drug blueprints coded in our DNA? Guess the days of “take two and call me in the morning” are officially over. Should we start a genetic compatibility dating app next, or is that already a thing?
That’s a great analogy! “Personalized drug blueprints” really captures the essence of pharmacogenomics. The potential for genetic compatibility apps is definitely an interesting thought, highlighting how deeply genetics could integrate into our lives. It raises some fascinating ethical questions!
Editor: MedTechNews.Uk
Thank you to our Sponsor Esdebe
Personalized medicine sounds fantastic until your insurance company decides your “unique genetic blueprint” makes that life-saving treatment “experimental” and therefore not covered. Silver linings and all that!