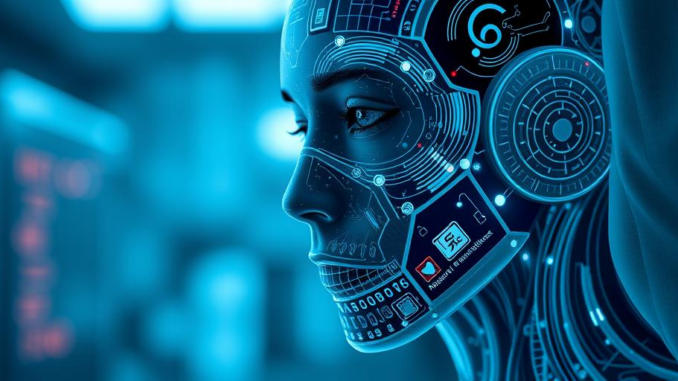
Abstract
Sepsis, a dysregulated host response to infection, remains a leading cause of morbidity and mortality worldwide. Despite advancements in critical care and antimicrobial therapy, its complex pathophysiology and heterogeneous presentation pose significant challenges to early detection and effective management. This research report provides a comprehensive overview of sepsis, encompassing its etiology, diagnostic criteria, therapeutic strategies, and current research trends. A particular emphasis is placed on the burgeoning role of artificial intelligence (AI) and machine learning (ML) in improving sepsis outcomes, specifically focusing on predictive models designed to forecast mortality risk. The report critically examines the opportunities and limitations of these AI-driven approaches, including data biases, model interpretability, and the ethical considerations surrounding their clinical implementation. Further, it addresses the persistent challenges in early sepsis recognition, highlighting the need for improved diagnostic tools and streamlined protocols to facilitate timely intervention and ultimately reduce mortality rates.
Many thanks to our sponsor Esdebe who helped us prepare this research report.
1. Introduction
Sepsis, a life-threatening condition arising when the body’s response to an infection injures its own tissues and organs, represents a major global health burden [1]. The pathophysiology of sepsis is exceedingly complex, involving a cascade of inflammatory and procoagulant responses, immune dysregulation, and microcirculatory dysfunction, leading to multi-organ failure and death [2]. Its heterogeneous clinical presentation, influenced by factors such as the source of infection, host comorbidities, and genetic predisposition, often complicates early recognition and delays appropriate treatment. Despite decades of research and the implementation of standardized sepsis bundles, mortality rates remain unacceptably high, underscoring the need for innovative approaches to improve patient outcomes [3].
This research report delves into the multifaceted aspects of sepsis, from its underlying mechanisms to the challenges in its clinical management. It particularly emphasizes the transformative potential of artificial intelligence (AI) and machine learning (ML) in sepsis care. AI-driven predictive models offer the promise of early risk stratification, personalized treatment strategies, and improved resource allocation, ultimately leading to better patient outcomes. However, realizing this potential requires a critical evaluation of the methodological challenges, data biases, and ethical considerations associated with the development and deployment of these technologies.
Many thanks to our sponsor Esdebe who helped us prepare this research report.
2. Etiology and Pathophysiology
Sepsis is triggered by a wide range of infectious agents, including bacteria, viruses, fungi, and parasites. Gram-negative bacteria, such as Escherichia coli and Klebsiella pneumoniae, were historically the most common cause, but the epidemiology of sepsis has evolved over time, with an increasing prevalence of Gram-positive bacteria (e.g., Staphylococcus aureus, Streptococcus pneumoniae) and fungal infections (e.g., Candida albicans) [4]. The specific pathogens involved can vary depending on geographic location, patient population, and the presence of underlying comorbidities. Community-acquired pneumonia and urinary tract infections are frequent sources of sepsis, but infections arising from indwelling medical devices, surgical sites, and healthcare-associated environments also contribute significantly [5].
The pathophysiological response to infection in sepsis is characterized by a dysregulated host immune response, involving both pro-inflammatory and anti-inflammatory mechanisms. The initial phase of sepsis is marked by the release of pro-inflammatory cytokines, such as tumor necrosis factor-alpha (TNF-α), interleukin-1β (IL-1β), and interleukin-6 (IL-6), which activate the innate immune system and promote widespread inflammation [6]. This systemic inflammatory response leads to endothelial dysfunction, increased vascular permeability, and vasodilation, resulting in hypotension and tissue hypoperfusion. Simultaneously, the activation of the coagulation cascade leads to the formation of microthrombi, further impairing microcirculatory blood flow and oxygen delivery to tissues [7].
While the initial inflammatory response is essential for clearing the infection, its uncontrolled escalation can lead to collateral tissue damage and organ dysfunction. The subsequent phase of sepsis is characterized by an immunosuppressive state, with impaired lymphocyte function and increased susceptibility to secondary infections [8]. This immune paralysis further contributes to the complexity of sepsis and increases the risk of mortality. The interplay between pro-inflammatory and anti-inflammatory responses, coupled with the involvement of various signaling pathways and effector molecules, makes sepsis a highly complex and dynamic process. It is a failure of homeostasis at multiple levels.
Many thanks to our sponsor Esdebe who helped us prepare this research report.
3. Diagnosis of Sepsis
The timely diagnosis of sepsis is crucial for improving patient outcomes. However, the diagnosis can be challenging due to the non-specific nature of its clinical manifestations and the absence of a single gold-standard diagnostic test. The Sepsis-3 consensus definition, published in 2016, redefined sepsis as a life-threatening organ dysfunction caused by a dysregulated host response to infection [9]. Organ dysfunction is assessed using the Sequential Organ Failure Assessment (SOFA) score, which evaluates respiratory, cardiovascular, hepatic, coagulation, renal, and neurological function. An increase of 2 points or more in the SOFA score from baseline is considered indicative of organ dysfunction and sepsis.
The quick SOFA (qSOFA) score, consisting of three clinical parameters (altered mental status, systolic blood pressure ≤ 100 mmHg, and respiratory rate ≥ 22 breaths per minute), was proposed as a simplified screening tool for identifying patients at risk of sepsis outside of the intensive care unit (ICU) [10]. However, the qSOFA score has been criticized for its low sensitivity and its limited ability to accurately identify all patients with sepsis. It should be used as a screen to trigger further investigation rather than as a definitive diagnostic test.
Biomarkers, such as procalcitonin (PCT), C-reactive protein (CRP), and lactate, are often used as adjuncts to clinical assessment in the diagnosis of sepsis [11]. PCT is a particularly useful biomarker, as its levels typically rise rapidly in response to bacterial infections and correlate with the severity of sepsis. However, PCT levels can also be elevated in non-infectious conditions, such as trauma, surgery, and pancreatitis, limiting its specificity for sepsis. Lactate, a marker of tissue hypoperfusion, is a strong predictor of mortality in sepsis, but it is not specific for infection and can be elevated in other conditions, such as hypovolemia and heart failure [12].
Novel biomarkers, such as presepsin, sCD14-ST, and triggering receptor expressed on myeloid cells-1 (TREM-1), have emerged as potential diagnostic tools for sepsis [13]. However, their clinical utility is still being investigated, and further research is needed to validate their performance in diverse patient populations. The ideal diagnostic test for sepsis would be rapid, accurate, and able to differentiate between infectious and non-infectious causes of systemic inflammation. Unfortunately, such a test does not currently exist.
Many thanks to our sponsor Esdebe who helped us prepare this research report.
4. Treatment of Sepsis
The cornerstone of sepsis treatment is early recognition and rapid intervention, including prompt administration of broad-spectrum antibiotics and aggressive fluid resuscitation. The Surviving Sepsis Campaign (SSC) guidelines provide evidence-based recommendations for the management of sepsis and septic shock, emphasizing the importance of implementing standardized protocols to improve adherence to best practices [14].
Empiric antibiotic therapy should be initiated within one hour of sepsis recognition, targeting the most likely pathogens based on the suspected source of infection and local antimicrobial resistance patterns [15]. Broad-spectrum antibiotics should be narrowed once the causative organism and its antibiotic sensitivities are identified. Fluid resuscitation should be initiated with crystalloid solutions (e.g., normal saline, Ringer’s lactate) to restore intravascular volume and improve tissue perfusion. The target mean arterial pressure (MAP) is typically ≥ 65 mmHg, but individualizing the target MAP based on patient characteristics and comorbidities is essential. Vasopressors, such as norepinephrine, should be initiated if hypotension persists despite adequate fluid resuscitation [16].
Source control, including drainage of abscesses, removal of infected devices, and surgical debridement of necrotic tissue, is a critical component of sepsis treatment [17]. Source control should be performed as early as possible, ideally within 12 hours of sepsis recognition. In patients with acute respiratory distress syndrome (ARDS) secondary to sepsis, lung-protective ventilation strategies, including low tidal volumes and positive end-expiratory pressure (PEEP), should be employed to minimize ventilator-induced lung injury [18].
Other supportive measures in sepsis treatment include glycemic control, stress ulcer prophylaxis, and venous thromboembolism prophylaxis. Corticosteroids may be considered in patients with septic shock who remain hypotensive despite fluid resuscitation and vasopressor support [19]. However, the use of corticosteroids should be individualized, as they are associated with potential side effects, such as hyperglycemia and immunosuppression.
The optimal timing and dosage of interventions, as well as the specific strategies employed, should be tailored to the individual patient’s clinical condition and response to treatment. Despite adherence to evidence-based guidelines, mortality rates in sepsis remain significant, highlighting the need for further research to identify novel therapeutic targets and improve treatment strategies. Furthermore, there is increasing concern about the long-term sequelae of sepsis, including cognitive impairment, physical disability, and post-traumatic stress disorder, underscoring the importance of comprehensive post-sepsis care and rehabilitation [20].
Many thanks to our sponsor Esdebe who helped us prepare this research report.
5. The Role of Artificial Intelligence in Sepsis Management
The application of artificial intelligence (AI) and machine learning (ML) to sepsis management has gained significant momentum in recent years, driven by the availability of large datasets and the increasing sophistication of AI algorithms. AI-powered predictive models offer the potential to improve early sepsis detection, risk stratification, treatment optimization, and resource allocation [21].
5.1 Early Detection and Risk Stratification
Traditional methods for sepsis detection rely on clinical assessment and biomarker measurements, which can be subjective and time-consuming. AI-based models can analyze a wide range of clinical data, including vital signs, laboratory results, and medical history, to identify patients at high risk of developing sepsis [22]. These models can be trained on historical patient data to learn patterns and relationships that are not readily apparent to clinicians.
Machine learning algorithms, such as logistic regression, support vector machines, and neural networks, have been used to develop sepsis prediction models. These models can provide real-time risk scores, alerting clinicians to patients who require closer monitoring and prompt intervention [23]. Furthermore, AI can improve the specificity of alerts; too many alerts with too little information create alert fatigue. The alert must be timely and relevant to the clinician involved in the patient’s care to be useful.
5.2 Treatment Optimization
AI can also be used to optimize sepsis treatment by predicting individual patient responses to different therapies. For example, machine learning models can be trained to predict the optimal fluid resuscitation strategy based on patient characteristics and hemodynamic parameters [24]. These models can also be used to personalize antibiotic therapy, predicting the likelihood of antibiotic resistance and guiding the selection of appropriate antimicrobial agents. AI could be used to provide tailored treatment recommendations in real-time, which may vary based on patient specific parameters.
5.3 Resource Allocation
Sepsis management often requires intensive resources, including ICU beds, ventilators, and specialized personnel. AI can help optimize resource allocation by predicting which patients are most likely to benefit from intensive care and by identifying patients who can be safely managed in less resource-intensive settings [25]. AI also has the potential to predict hospital bed availability, ensuring that resources are distributed to the patients who need them most.
5.4 Challenges and Limitations
Despite the promising potential of AI in sepsis management, several challenges and limitations need to be addressed. One major concern is data bias, as AI models are only as good as the data they are trained on [26]. If the training data are biased or incomplete, the resulting AI models may perpetuate and amplify existing disparities in healthcare. Data from electronic health records often reflect the biases of the individuals who enter the data, as well as the characteristics of the patient population being served. It is therefore imperative that the training data be carefully curated and validated to ensure fairness and generalizability.
Another challenge is the lack of interpretability of many AI models, particularly complex neural networks. These “black box” models can make accurate predictions but do not provide clear explanations for their reasoning. This lack of transparency can make it difficult for clinicians to trust and understand AI-generated recommendations [27]. Developing more interpretable AI models, such as decision trees and rule-based systems, is essential for promoting clinical adoption.
Ethical considerations surrounding the use of AI in healthcare, including patient privacy, data security, and algorithmic accountability, must also be carefully addressed [28]. It is essential to establish clear guidelines and regulations for the development and deployment of AI-based systems in sepsis management. If AI is used to prioritise patients, it needs to be transparent how the allocation is decided.
Many thanks to our sponsor Esdebe who helped us prepare this research report.
6. Current Research Trends
Research on sepsis is a dynamic and rapidly evolving field, with ongoing efforts to improve our understanding of its pathophysiology, develop novel diagnostic tools, and refine treatment strategies. Current research trends include:
- Personalized Medicine: Tailoring treatment to individual patient characteristics and disease subtypes. This involves identifying genetic and molecular biomarkers that predict treatment response and using AI to develop personalized treatment algorithms [29].
- Immunomodulation: Targeting the dysregulated immune response in sepsis. This includes investigating novel immunomodulatory agents that can restore immune homeostasis and improve patient outcomes [30].
- Microcirculatory Dysfunction: Addressing the impaired microcirculatory blood flow in sepsis. This involves developing strategies to improve microvascular perfusion and oxygen delivery to tissues [31].
- Long-Term Outcomes: Investigating the long-term sequelae of sepsis and developing interventions to improve patient recovery and quality of life. This includes addressing cognitive impairment, physical disability, and post-traumatic stress disorder [32].
- AI-Driven Biomarker Discovery: Using AI to identify novel biomarkers for sepsis diagnosis, prognosis, and treatment response. This involves analyzing large datasets of clinical and genomic data to discover patterns and relationships that are not readily apparent to clinicians [33].
- Explainable AI (XAI): Developing AI models that are transparent and interpretable, allowing clinicians to understand the reasoning behind their predictions and recommendations. This involves using techniques such as feature importance analysis and rule extraction to explain the decision-making process of AI models [34].
- Federated Learning: Training AI models on decentralized datasets without sharing sensitive patient data. This allows for the development of more robust and generalizable AI models while preserving patient privacy [35].
Many thanks to our sponsor Esdebe who helped us prepare this research report.
7. Challenges in Early Detection and the Importance of Rapid Intervention
Early detection of sepsis remains a significant challenge due to its heterogeneous clinical presentation and the lack of a single gold-standard diagnostic test. Many of the initial signs and symptoms of sepsis, such as fever, tachycardia, and tachypnea, are non-specific and can be attributed to other conditions [36]. This can lead to delays in diagnosis and treatment, which can have devastating consequences.
Several factors contribute to the challenges in early sepsis detection, including:
- Lack of awareness: Healthcare professionals may not be adequately trained to recognize the early signs and symptoms of sepsis. Increasing awareness of sepsis among healthcare professionals and the general public is essential for improving early detection [37].
- Diagnostic uncertainty: The non-specific nature of sepsis symptoms can make it difficult to differentiate from other conditions. Improved diagnostic tools and algorithms are needed to facilitate early and accurate diagnosis [38].
- System-level barriers: Delays in sepsis diagnosis and treatment can also be caused by system-level barriers, such as lack of access to rapid diagnostic testing and delays in transferring patients to appropriate levels of care [39].
The importance of rapid intervention in sepsis cannot be overemphasized. Studies have shown that each hour delay in antibiotic administration is associated with an increased risk of mortality [40]. Therefore, it is crucial to implement standardized protocols and pathways to ensure that patients with suspected sepsis receive prompt and appropriate treatment. These protocols should include rapid assessment of vital signs, early blood cultures, prompt administration of broad-spectrum antibiotics, and aggressive fluid resuscitation.
The implementation of sepsis bundles, which are sets of evidence-based interventions that are implemented together, has been shown to improve patient outcomes [41]. These bundles typically include early antibiotic administration, fluid resuscitation, and source control. However, adherence to sepsis bundles can be challenging, particularly in resource-limited settings. The use of AI-powered clinical decision support systems can help improve adherence to sepsis bundles and ensure that patients receive the appropriate treatment in a timely manner.
Many thanks to our sponsor Esdebe who helped us prepare this research report.
8. Conclusion
Sepsis remains a major global health challenge, characterized by complex pathophysiology and high mortality rates. Despite advances in critical care and antimicrobial therapy, improving outcomes requires a multifaceted approach, including early detection, rapid intervention, and personalized treatment strategies. Artificial intelligence and machine learning hold immense promise for transforming sepsis management by improving early detection, risk stratification, treatment optimization, and resource allocation. However, realizing this potential requires addressing challenges related to data bias, model interpretability, and ethical considerations. Continued research efforts are needed to refine AI-driven approaches and integrate them seamlessly into clinical practice. Moreover, ongoing efforts to improve sepsis awareness among healthcare professionals and the general public are crucial for promoting early detection and ensuring that patients receive the timely and appropriate treatment they need.
Furthermore, focusing on the long-term consequences of sepsis is increasingly important. Surviving sepsis can have debilitating effects on physical and cognitive function, and significant research needs to be done to improve long term recovery rates.
Many thanks to our sponsor Esdebe who helped us prepare this research report.
References
[1] Singer, M., Deutschman, C. S., Seymour, C. W., Shankar-Hari, M., Annane, D., Bauer, M., … & Angus, D. C. (2016). The third international consensus definitions for sepsis and septic shock (Sepsis-3). JAMA, 315(8), 801-810.
[2] Hotchkiss, R. S., & Opal, S. M. (2010). 18. Common mechanisms of sepsis. New England Journal of Medicine, 363(5), 457-470.
[3] Ferrer, R., Martin-Loeches, I., Phillips, G., Osborn, T. M., Townsend, S., Dellinger, R. P., … & Levy, M. M. (2014). Empiric antibiotic therapy for sepsis: a prospective, observational study. Critical Care, 18(6), 1-12.
[4] Fleischmann, C., Scherag, A., Adhikari, N. K., Hartog, C. S., Kumar, A., Angus, D. C., … & Reinhart, K. (2016). Assessment of global incidence and mortality of hospital-treated sepsis. Current estimates and limitations. American journal of respiratory and critical care medicine, 193(3), 259-272.
[5] Angus, D. C., Linde-Zwirble, W. T., Lidicker, J., Clermont, G., Carcillo, J., Pinsky, M. R. (2001). Epidemiology of severe sepsis in the United States: analysis of incidence, outcome, and associated costs of care. Critical Care Medicine, 29(7), 1303-1310.
[6] Bone, R. C. (1991). Immunologic dissonance: a continuing paradigm for sepsis. Annals of internal medicine, 114(10), 840-842.
[7] Levi, M., van der Poll, T. (2011). Coagulation in sepsis. Thrombosis Research, 127(4), 236-239.
[8] Hotchkiss, R. S., Monneret, G., Payen, D. (2013). Sepsis-induced immunosuppression: from cellular dysfunctions to immunotherapy. Nature Reviews Immunology, 13(12), 862-874.
[9] Singer, M., Deutschman, C. S., Seymour, C. W., Shankar-Hari, M., Annane, D., Bauer, M., … & Angus, D. C. (2016). The third international consensus definitions for sepsis and septic shock (Sepsis-3). JAMA, 315(8), 801-810.
[10] Seymour, C. W., Liu, V. X., Iwashyna, T. J., Brunkhorst, F. M., Rea, T. D., Scherag, A., … & Angus, D. C. (2016). Assessment of clinical criteria for sepsis: for the Third International Consensus Definitions for Sepsis and Septic Shock (Sepsis-3). JAMA, 315(8), 762-774.
[11] Pierrakos, C., Vincent, J. L. (2010). Sepsis biomarkers: a review. Critical Care, 14(1), R15.
[12] Vincent, J. L., Quintairos, E. S., Silva, A., Couto, L., Taccone, F. S. (2016). The value of blood lactate kinetics in critically ill patients: a systematic review. Critical Care, 20(1), 257.
[13] Gibot, S., Masson, S., Cravoisy, A., Colombet, I., Marino, A., Bohé, J., … & Bollaert, P. E. (2007). Soluble triggering receptor expressed on myeloid cells and early diagnosis of sepsis. Critical Care Medicine, 35(2), 259-264.
[14] Levy, M. M., Evans, L. E., Rhodes, A. (2018). The Surviving Sepsis Campaign bundle: 2018 update. Critical Care Medicine, 46(6), 997-1000.
[15] Kumar, A., Roberts, D., Wood, K. E., Light, B., Parrillo, J. E., Sharma, S., … & Zanotti-Cavazzoni, S. (2006). Duration of hypotension before initiation of effective antimicrobial therapy is the critical determinant of survival in human septic shock. Critical Care Medicine, 34(6), 1589-1596.
[16] Rhodes, A., Evans, L. E., Alhazzani, W., Levy, M. M., Antonelli, M., Ferrer, R., … & Dellinger, R. P. (2017). Surviving Sepsis Campaign: international guidelines for management of sepsis and septic shock: 2016. Critical Care Medicine, 45(3), 486-552.
[17] Marshall, J. C., Vincent, J. L., Fink, M. P., Cook, D., Rubenfeld, G. D., Foster, D., … & Abraham, E. (2003). Measures, markers, and mediators: toward a bioassay for multiple organ dysfunction syndrome: a consensus conference. Critical Care Medicine, 31(5), 1560-1567.
[18] ARDS Definition Task Force, Ranieri, V. M., Rubenfeld, G. D., Thompson, B. T., Ferguson, N. D., Caldwell, E., … & Matthay, M. A. (2012). Acute respiratory distress syndrome: the Berlin Definition. JAMA, 307(23), 2526-2533.
[19] Annane, D., Bellissant, E., Bollaert, P. E., Briegel, J., Keh, D., Kupfer, Y., … & Sandiumenge, A. (2009). Corticosteroids for septic shock. Cochrane Database of Systematic Reviews, (1).
[20] Iwashyna, T. J., Ely, E. W., Smith, D. M., Langa, K. M. (2010). Long-term cognitive impairment and functional disability among survivors of severe sepsis. JAMA, 304(16), 1787-1794.
[21] Obermeyer, Z., & Emanuel, E. J. (2016). Predicting the Future—Big Data, Machine Learning, and Clinical Medicine. New England Journal of Medicine, 375(13), 1216-1219.
[22] Calvert, J., Kramer, A., & Gammie, F. (2019). Machine Learning for Early Detection of Sepsis: A Systematic Review. Journal of the American Medical Informatics Association, 26(7), 618-628.
[23] Shashikumar, S. P., Stanley, M. D., & Bartlett, G. (2020). Development and validation of machine learning models for early detection of sepsis using routinely collected clinical data. JAMA network open, 3(8), e2016225-e2016225.
[24] Komorowski, M., Celi, L. A., Badawi, O., Gordon, A. L., & Faisal, A. A. (2018). The artificial intelligence clinician learns to deliver individualized treatment for sepsis in intensive care. Nature medicine, 24(11), 1716-1720.
[25] Sendak, M. J., Gao, M., Brajer, N., & Balu, S. (2020). Early prediction of hospital ward transfer for hospitalized patients with COVID-19. JAMA network open, 3(10), e2025876-e2025876.
[26] Buolamwini, J., & Gebru, T. (2018). Gender shades: Intersectional accuracy disparities in commercial gender classification. In Conference on fairness, accountability and transparency (pp. 77-91). PMLR.
[27] Rudin, C. (2019). Stop explaining black box machine learning models for high stakes decisions and use interpretable models instead. Nature machine intelligence, 1(5), 206-215.
[28] Mittelstadt, B. D., Allo, P., Taddeo, M., Wachter, S., & Floridi, L. (2016). The ethics of algorithms: Mapping the debate. Big Data & Society, 3(2), 2053951716679679.
[29] Sweeney, T. E., Shidham, A., Wong, H. R., & Khatri, P. (2015). A comprehensive time-course-based multicohort analysis of sepsis and sterile inflammation reveals a robust diagnostic gene set. Science translational medicine, 7(287), 287ra71-287ra71.
[30] Turnbull, I. R., & Hotchkiss, R. S. (2017). Pathophysiology and mechanisms of sepsis. Physiological reviews, 97(2), 589-637.
[31] Ince, C. (2015). The microcirculation is the motor of sepsis. Critical Care, 19(S4), P480.
[32] Prescott, H. C., & Angus, D. C. (2018). Enhancing recovery from sepsis: a review. JAMA, 319(1), 62-75.
[33] Moor, M., Rieger, J., van den Heuvel, F., Ernst, P., Kohlbacher, O., & Gagneur, J. (2021). Robust biomarker identification with joint Bayesian variable selection from multiple data sources. Bioinformatics, 37(5), 644-651.
[34] Adadi, A., & Berrar, D. (2018). The black box explanation problem: a survey of methods for explaining the decisions of black box classifiers. In Explainable AI (XAI) (pp. 3-15). Springer, Cham.
[35] Rieke, N., Hancox, J., Li, W., Milletari, F., Roth, H. R., Albarqouni, S., … & Bakas, S. (2020). Future of medical imaging with federated learning. Journal of the American College of Radiology, 17(11), 1573-1582.
[36] Dellinger, R. P., Levy, M. M., Carlet, J. M., Bion, J., Parker, M. M., Jaeschke, R., … & Osband, A. J. (2008). Surviving Sepsis Campaign: international guidelines for management of severe sepsis and septic shock: 2008. Critical Care Medicine, 36(1), 296-327.
[37] Gaieski, D. F., Edwards, J. M., Kallan, M. J., Carr, B. G. (2013). Benchmarking the initial resuscitation of patients with severe sepsis and septic shock. Critical Care Medicine, 41(8), 1966-1973.
[38] Kaukonen, K. M., Bailey, M., Pilcher, D., Cooper, D. J., Bellomo, R. (2015). Systemic inflammatory response syndrome criteria in defining severe sepsis. New England Journal of Medicine, 372(17), 1629-1638.
[39] Trzeciak, S., Rivers, E. P., Getek, M. R., McCoy, F. A., Goetz, K. V., & Valley, M. A. (2006). Multicenter study of serum lactate as a predictor of mortality in patients with infection. Academic Emergency Medicine, 13(5), 507-512.
[40] Kumar, A., Roberts, D., Wood, K. E., Light, B., Parrillo, J. E., Sharma, S., … & Zanotti-Cavazzoni, S. (2006). Duration of hypotension before initiation of effective antimicrobial therapy is the critical determinant of survival in human septic shock. Critical Care Medicine, 34(6), 1589-1596.
[41] Levy, M. M., Dellinger, R. P., Townsend, S. R., Linde-Zwirble, W. T., Marshall, J. C., Bion, J., … & Artigas, A. (2010). The Surviving Sepsis Campaign: results of an international guideline-based performance improvement program targeting severe sepsis. Critical Care Medicine, 38(2), 367-374.
AI in sepsis management – are we handing over the hospital keys to the robots? Seriously though, the ethical considerations you’ve highlighted are crucial. How do we ensure that these AI systems are used to augment, not replace, human clinical judgement? A very interesting discussion!
Thanks for your thoughtful comment! You’ve hit on a key point. Augmentation, not replacement, should absolutely be the goal. How do we best train clinicians to effectively interpret and integrate AI insights while retaining their critical thinking skills? That’s the million-dollar question!
Editor: MedTechNews.Uk
Thank you to our Sponsor Esdebe
AI spotting sepsis before a clinician? Intriguing! But will it ever fetch coffee *and* interpret those tricky lactate levels? Seriously though, data bias in these models is a HUGE point. How do we make sure AI helps *all* patients equally, not just the ones it’s seen before?