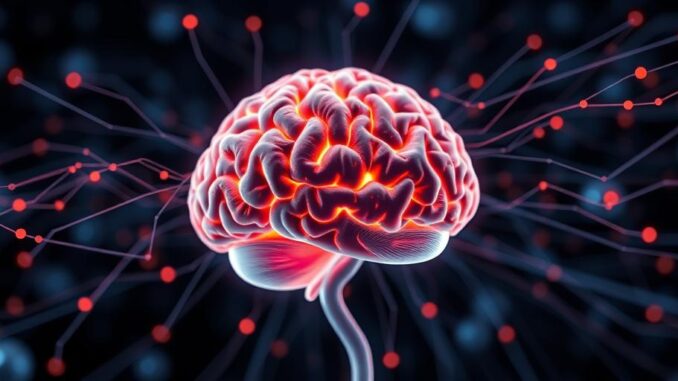
Abstract
Principal Component Analysis (PCA) is a widely used dimensionality reduction technique in various fields, including neuroimaging. This research report provides a comprehensive overview of PCA, covering its mathematical foundation, practical applications, limitations, and recent advances, particularly in the context of high-dimensional neuroimaging data analysis. We specifically examine its application in understanding Posterior Cortical Atrophy (PCA), a visual variant of Alzheimer’s disease, highlighting PCA’s utility in identifying disease-related patterns in neuroimaging data and discussing its strengths and weaknesses compared to other methods. The report also explores current research directions aimed at improving PCA’s performance and interpretability, such as incorporating sparsity constraints, kernel methods, and probabilistic frameworks, and its use in multimodal data fusion.
Many thanks to our sponsor Esdebe who helped us prepare this research report.
1. Introduction
In the era of big data, the analysis of high-dimensional datasets has become increasingly prevalent across various scientific disciplines. Neuroimaging, with its ability to capture intricate brain activity and structure, is a prime example of a field generating vast amounts of complex data. Techniques such as functional Magnetic Resonance Imaging (fMRI), Diffusion Tensor Imaging (DTI), and Positron Emission Tomography (PET) produce datasets with thousands or even millions of variables, posing significant challenges for data analysis and interpretation. One of the most fundamental challenges is the “curse of dimensionality,” where the number of variables exceeds the number of observations, leading to overfitting and reduced statistical power.
Dimensionality reduction techniques, therefore, become essential for extracting meaningful information from high-dimensional neuroimaging data. These techniques aim to reduce the number of variables while preserving the essential structure and information content of the data. Among the various dimensionality reduction methods, Principal Component Analysis (PCA) stands out as a particularly popular and versatile tool.
PCA is a linear transformation technique that identifies orthogonal directions (principal components) in the data that capture the maximum variance. These principal components can be used to represent the original data in a lower-dimensional space, thereby simplifying subsequent analyses and improving computational efficiency. PCA has been successfully applied to a wide range of neuroimaging problems, including denoising, feature extraction, data visualization, and the identification of disease-related patterns.
This research report provides a comprehensive overview of PCA, covering its theoretical underpinnings, practical applications, limitations, and recent advances. The report specifically focuses on the application of PCA in high-dimensional neuroimaging data analysis, with a particular emphasis on Posterior Cortical Atrophy (PCA), a visual variant of Alzheimer’s disease. PCA has been used in the analysis of structural and functional neuroimaging data in PCA to identify patterns of brain atrophy and dysfunction associated with the disease. This report will discuss the utility of PCA in this context, its strengths, and weaknesses relative to alternative methods. It will also explore current research directions aimed at improving PCA’s performance and interpretability, such as incorporating sparsity constraints, kernel methods, and probabilistic frameworks.
Many thanks to our sponsor Esdebe who helped us prepare this research report.
2. Mathematical Foundations of PCA
PCA is fundamentally based on the concept of orthogonal transformations and the eigenvalue decomposition of the data’s covariance matrix. The goal of PCA is to find a set of orthogonal vectors (principal components) that capture the maximum variance in the data. These components are ordered such that the first component captures the most variance, the second component captures the second most variance, and so on.
Formally, let X be an n x p data matrix, where n is the number of observations and p is the number of variables. The steps involved in PCA are as follows:
-
Data Preprocessing: The data is typically centered by subtracting the mean of each variable from its corresponding values. This ensures that the principal components are not influenced by the mean of the data.
-
Covariance Matrix Calculation: The covariance matrix C is calculated as:
C = (1/(n-1)) * XTX
where XT is the transpose of X.
-
Eigenvalue Decomposition: The covariance matrix C is then subjected to eigenvalue decomposition:
C = VΛVT
where V is a matrix whose columns are the eigenvectors of C, and Λ is a diagonal matrix containing the eigenvalues of C.
-
Principal Component Selection: The eigenvectors are sorted in descending order based on their corresponding eigenvalues. The first k eigenvectors, corresponding to the k largest eigenvalues, are selected as the principal components. These principal components capture the most variance in the data.
-
Dimensionality Reduction: The original data X is projected onto the selected principal components to obtain the reduced-dimensional representation:
Y = XV
where Y is an n x k matrix representing the data in the reduced-dimensional space.
The eigenvalues represent the amount of variance explained by each principal component. The proportion of variance explained (PVE) by each component is calculated as:
PVE<sub>i</sub> = λ<sub>i</sub> / Σλ<sub>j</sub>
where λ<sub>i</sub> is the eigenvalue of the i-th principal component and Σλ<sub>j</sub> is the sum of all eigenvalues.
The cumulative PVE can be used to determine the number of principal components to retain. A common approach is to retain enough components to explain a certain percentage of the total variance, such as 80% or 90%.
Discussion: The mathematical elegance of PCA lies in its ability to decompose complex data into a set of orthogonal components that capture the maximum variance. This allows for a significant reduction in dimensionality while preserving the essential information content of the data. The choice of the number of principal components to retain is a critical step, and various methods, such as the elbow method and scree plot, can be used to guide this decision. However, it is important to note that PCA is a linear technique and may not be suitable for capturing non-linear relationships in the data. Kernel PCA, discussed later, offers a solution to this limitation.
Many thanks to our sponsor Esdebe who helped us prepare this research report.
3. Applications of PCA in Neuroimaging
PCA has been widely applied in neuroimaging for a variety of purposes, including data denoising, feature extraction, data visualization, and the identification of disease-related patterns. Some specific examples of PCA applications in neuroimaging include:
- fMRI Data Analysis: PCA can be used to reduce the dimensionality of fMRI data, identifying patterns of brain activity that are correlated with specific tasks or stimuli. This can help to improve the statistical power of fMRI analyses and reduce the computational burden. For example, in task-based fMRI, PCA can extract task-related components, effectively separating the signal from noise and allowing researchers to identify brain regions that are significantly activated during the task.
- Structural MRI Analysis: PCA can be used to analyze structural MRI data, such as voxel-based morphometry (VBM) images, to identify patterns of brain atrophy associated with various neurological disorders. This can help to improve the diagnosis and monitoring of these disorders. Specifically, PCA can be used to identify regions of the brain that show significant atrophy in patients with Alzheimer’s disease or other neurodegenerative conditions. The principal components can then be used to classify patients and controls, or to track the progression of the disease over time.
- EEG and MEG Data Analysis: PCA can be used to reduce the dimensionality of EEG and MEG data, identifying patterns of brain activity that are associated with specific cognitive processes. This can help to improve the understanding of brain function and the diagnosis of neurological disorders. PCA can isolate independent components corresponding to different brain sources or artifacts, aiding in the analysis of event-related potentials and oscillatory activity.
- Multimodal Data Fusion: PCA can be used to integrate data from different neuroimaging modalities, such as fMRI, DTI, and PET, to obtain a more comprehensive understanding of brain structure and function. This can help to improve the diagnosis and treatment of neurological disorders. By combining data from multiple modalities, PCA can identify patterns that are not apparent when analyzing each modality separately.
Application to Posterior Cortical Atrophy (PCA): PCA has been particularly useful in studying Posterior Cortical Atrophy (PCA), a visual variant of Alzheimer’s disease. PCA patients typically present with prominent visuospatial and visuoperceptual deficits, rather than the memory impairment characteristic of typical Alzheimer’s disease. Neuroimaging studies using PCA have revealed specific patterns of brain atrophy and dysfunction in the parieto-occipital regions of the brain in PCA patients. These patterns can be used to differentiate PCA from typical Alzheimer’s disease and other neurological disorders.
For instance, researchers have used PCA on VBM data from PCA patients and healthy controls to identify principal components that distinguish between the two groups. The loadings on these components reveal the brain regions that are most affected in PCA, such as the parietal and occipital lobes. Similarly, PCA has been applied to fMRI data to identify patterns of functional connectivity that are disrupted in PCA. The principal components can be used to characterize the functional networks that are most affected by the disease, providing insights into the neural mechanisms underlying the visual and spatial deficits observed in PCA patients.
Discussion: PCA’s versatility makes it a valuable tool across various neuroimaging modalities and applications. Its ability to reduce dimensionality and extract meaningful features has contributed significantly to our understanding of brain structure, function, and disease. However, it is crucial to consider the limitations of PCA, particularly its linearity assumption, and explore alternative methods for capturing non-linear relationships in the data.
Many thanks to our sponsor Esdebe who helped us prepare this research report.
4. Limitations of PCA
Despite its widespread use and advantages, PCA has several limitations that must be considered when applying it to neuroimaging data:
- Linearity Assumption: PCA is a linear technique, meaning that it can only capture linear relationships between variables. In many cases, neuroimaging data may exhibit non-linear relationships, which PCA may fail to capture. This can lead to suboptimal dimensionality reduction and inaccurate representation of the data. Kernel PCA, discussed later, addresses this limitation.
- Sensitivity to Outliers: PCA is sensitive to outliers in the data. Outliers can significantly influence the principal components, leading to a biased representation of the data. Robust PCA methods, which are less sensitive to outliers, have been developed to address this limitation.
- Interpretability: While PCA can reduce the dimensionality of the data, the resulting principal components may not always be easy to interpret. The principal components are linear combinations of the original variables, and the loadings on these components may not always have a clear meaning. This can make it difficult to understand the underlying biological processes that are captured by the principal components. Techniques such as sparse PCA aim to improve interpretability by encouraging sparse loadings on the principal components.
- Data Scaling: PCA is sensitive to the scaling of the variables. If the variables have different scales, the principal components may be dominated by the variables with the largest scales. It is important to scale the data appropriately before applying PCA. Standardization, which involves subtracting the mean and dividing by the standard deviation, is a common scaling technique.
- Assumption of Gaussian Distribution: PCA assumes that the data follows a Gaussian distribution. While PCA can still be applied to non-Gaussian data, the results may not be optimal. Independent Component Analysis (ICA), which does not assume a Gaussian distribution, may be a more appropriate technique for analyzing non-Gaussian data.
Impact on PCA in the context of PCA patients: Consider applying PCA to structural MRI data from PCA patients and healthy controls. If there are significant outliers in the data, such as individuals with unusually large brain volumes or lesions, these outliers can distort the principal components and lead to inaccurate results. In addition, if the relationships between brain regions and disease severity are non-linear, PCA may fail to capture these relationships effectively. Thus, the identification of brain regions that show the most difference between PCA patients and controls may be incomplete, and the subsequent classifications may be inaccurate.
Discussion: The limitations of PCA highlight the importance of carefully considering the characteristics of the data and the research question when choosing a dimensionality reduction technique. While PCA is a powerful and versatile tool, it is not always the most appropriate choice for all situations. Alternative methods, such as kernel PCA, robust PCA, sparse PCA, and ICA, may be more suitable for addressing specific limitations of PCA.
Many thanks to our sponsor Esdebe who helped us prepare this research report.
5. Advances in PCA
Researchers have developed several extensions and modifications of PCA to address its limitations and improve its performance. Some of the most notable advances in PCA include:
- Kernel PCA: Kernel PCA is a non-linear extension of PCA that uses kernel functions to map the data into a higher-dimensional space, where linear PCA can be applied. This allows Kernel PCA to capture non-linear relationships between variables. Common kernel functions include the Gaussian kernel and the polynomial kernel. The choice of kernel function and its parameters can significantly impact the performance of Kernel PCA.
- Sparse PCA: Sparse PCA is a variant of PCA that encourages sparse loadings on the principal components. This improves the interpretability of the principal components by reducing the number of variables that contribute to each component. Sparse PCA can be implemented using various optimization techniques, such as L1 regularization.
- Robust PCA: Robust PCA is a variant of PCA that is less sensitive to outliers in the data. Robust PCA can be implemented using various techniques, such as M-estimation and trimming. These techniques reduce the influence of outliers on the principal components.
- Probabilistic PCA: Probabilistic PCA is a probabilistic formulation of PCA that provides a statistical framework for estimating the principal components and their associated variances. This allows for the incorporation of prior knowledge and the estimation of confidence intervals for the principal components. Probabilistic PCA can be implemented using expectation-maximization (EM) algorithms.
- Multilinear PCA: Multilinear PCA is an extension of PCA that can be used to analyze multi-dimensional data, such as tensor data. This is particularly useful for neuroimaging data, which often has a multi-dimensional structure (e.g., space x time x subject). Multilinear PCA can be used to reduce the dimensionality of each dimension separately or jointly.
Application to PCA patients: These advanced PCA methods can be particularly valuable in studying PCA. For example, Kernel PCA can be used to capture non-linear relationships between brain regions and disease severity. Sparse PCA can be used to identify a small set of brain regions that are most important for discriminating between PCA patients and healthy controls, improving the interpretability of the results. Robust PCA can be used to reduce the influence of outliers, such as individuals with atypical brain structures. Probabilistic PCA can provide a statistical framework for estimating the uncertainty in the principal components and their loadings.
Discussion: These advances in PCA have significantly expanded its applicability and improved its performance in various neuroimaging applications. The choice of which PCA variant to use depends on the specific characteristics of the data and the research question. It is important to carefully consider the advantages and disadvantages of each method before applying it to the data.
Many thanks to our sponsor Esdebe who helped us prepare this research report.
6. Alternative Dimensionality Reduction Techniques
While PCA is a powerful and widely used dimensionality reduction technique, it is not the only option available. Several alternative techniques may be more appropriate for certain types of data or research questions. Some of the most common alternative dimensionality reduction techniques include:
- Independent Component Analysis (ICA): ICA is a statistical technique that separates a multivariate signal into additive, statistically independent subcomponents. Unlike PCA, ICA does not assume that the components are orthogonal. ICA is particularly useful for analyzing data that is composed of a mixture of independent sources, such as EEG or MEG data.
- Linear Discriminant Analysis (LDA): LDA is a supervised dimensionality reduction technique that aims to find the linear combination of variables that best separates two or more classes. LDA is particularly useful for classification problems, where the goal is to predict the class membership of new observations. LDA requires labeled data, where the class membership of each observation is known.
- Non-negative Matrix Factorization (NMF): NMF is a matrix factorization technique that decomposes a matrix into two non-negative matrices. This constraint can be useful for analyzing data that is inherently non-negative, such as gene expression data or image data. NMF can also be used to identify underlying patterns or themes in the data.
- t-distributed Stochastic Neighbor Embedding (t-SNE): t-SNE is a non-linear dimensionality reduction technique that is particularly well-suited for visualizing high-dimensional data in a low-dimensional space (e.g., 2D or 3D). t-SNE aims to preserve the local structure of the data, such that points that are close to each other in the high-dimensional space are also close to each other in the low-dimensional space.
- Autoencoders: Autoencoders are neural networks that are trained to reconstruct their input. By forcing the network to pass through a bottleneck layer, the autoencoder learns a compressed representation of the data. Autoencoders can be used for dimensionality reduction, feature extraction, and anomaly detection. Variational Autoencoders (VAEs) further enhance this by learning a probability distribution of the encoded data, enabling generative capabilities.
Comparison in the context of PCA patients: Consider using these alternative techniques to analyze structural MRI data from PCA patients and healthy controls. ICA could be used to identify independent components corresponding to different brain networks, which may be differentially affected in PCA. LDA could be used to find the linear combination of brain regions that best separates PCA patients from healthy controls. NMF could be used to identify underlying patterns of brain atrophy in PCA. T-SNE could be used to visualize the high-dimensional MRI data in a low-dimensional space, allowing for the identification of clusters of patients with similar patterns of brain atrophy. Autoencoders could be used to learn a compressed representation of the MRI data, which could be used for classification or anomaly detection.
Discussion: The choice of which dimensionality reduction technique to use depends on the specific characteristics of the data and the research question. It is important to carefully consider the advantages and disadvantages of each method before applying it to the data. In some cases, it may be beneficial to combine multiple techniques to obtain a more comprehensive understanding of the data. For example, PCA could be used to reduce the dimensionality of the data, followed by t-SNE to visualize the reduced-dimensional data in a low-dimensional space.
Many thanks to our sponsor Esdebe who helped us prepare this research report.
7. Future Research Directions
Despite the significant progress that has been made in PCA and related techniques, there are still many opportunities for future research. Some promising directions for future research include:
- Developing more robust and efficient PCA algorithms: There is a need for PCA algorithms that are more robust to outliers and noise and that can handle large-scale datasets more efficiently. This could involve developing new optimization techniques or using parallel computing architectures.
- Incorporating prior knowledge into PCA: Incorporating prior knowledge, such as anatomical constraints or functional connectivity information, into PCA could improve the accuracy and interpretability of the results. This could involve using Bayesian PCA or developing new regularization techniques.
- Developing PCA-based methods for multimodal data fusion: There is a growing need for methods that can effectively integrate data from multiple neuroimaging modalities. PCA-based methods could be particularly well-suited for this task, as they can identify common patterns across different modalities.
- Applying PCA to longitudinal neuroimaging data: Longitudinal neuroimaging data provides valuable information about the progression of neurological disorders. Applying PCA to longitudinal data could help to identify patterns of brain change that are associated with disease progression.
- Developing interpretable machine learning models based on PCA: Combining PCA with other machine learning techniques, such as support vector machines or deep learning, could lead to more accurate and interpretable models for diagnosing and predicting neurological disorders.
Specific application to PCA patients: In the context of PCA, future research could focus on developing PCA-based methods for early detection and diagnosis of the disease. This could involve using PCA to identify patterns of brain atrophy or dysfunction that are present in the early stages of PCA. Additionally, PCA could be used to predict the rate of disease progression and to identify individuals who are at high risk of developing PCA. Ultimately the goal should be to build models that can be implemented and used in hospitals.
Discussion: These future research directions highlight the potential for PCA and related techniques to continue to advance our understanding of brain structure, function, and disease. By addressing the limitations of current methods and developing new approaches, researchers can unlock even greater insights from neuroimaging data.
Many thanks to our sponsor Esdebe who helped us prepare this research report.
8. Conclusion
Principal Component Analysis (PCA) is a versatile and powerful dimensionality reduction technique that has been widely applied in neuroimaging. It has proven valuable in various applications, including data denoising, feature extraction, data visualization, and the identification of disease-related patterns. Particularly, in the context of Posterior Cortical Atrophy (PCA), PCA has been instrumental in identifying specific patterns of brain atrophy and dysfunction, aiding in the differentiation of PCA from other neurological disorders. However, PCA also has limitations, such as its linearity assumption, sensitivity to outliers, and challenges in interpretability. To address these limitations, researchers have developed several advanced PCA methods, including Kernel PCA, Sparse PCA, Robust PCA, and Probabilistic PCA.
Furthermore, alternative dimensionality reduction techniques, such as Independent Component Analysis (ICA), Linear Discriminant Analysis (LDA), and t-distributed Stochastic Neighbor Embedding (t-SNE), offer complementary approaches to analyzing neuroimaging data. The choice of which technique to use depends on the specific characteristics of the data and the research question.
Future research directions should focus on developing more robust and efficient PCA algorithms, incorporating prior knowledge into PCA, developing PCA-based methods for multimodal data fusion, applying PCA to longitudinal neuroimaging data, and developing interpretable machine learning models based on PCA. These advancements will further enhance the utility of PCA and related techniques in advancing our understanding of brain structure, function, and disease.
Many thanks to our sponsor Esdebe who helped us prepare this research report.
References
- Jolliffe, I. T., & Cadima, J. (2016). Principal component analysis: a review and recent developments. Philosophical Transactions of the Royal Society A: Mathematical, Physical and Engineering Sciences, 374(2066), 20150202.
- Bishop, C. M. (2006). Pattern recognition and machine learning. Springer.
- Hastie, T., Tibshirani, R., & Friedman, J. (2009). The elements of statistical learning: data mining, inference, and prediction. Springer science & business media.
- Schölkopf, B., Smola, A., & Müller, K. R. (1998). Nonlinear component analysis as a kernel eigenvalue problem. Neural computation, 10(5), 1299-1319.
- Zou, H., Hastie, T., & Tibshirani, R. (2006). Sparse principal component analysis. Journal of computational and graphical statistics, 15(2), 265-286.
- Huber, P. J. (2009). Robust statistics. John Wiley & Sons.
- Tipping, M. E., & Bishop, C. M. (1999). Probabilistic principal component analysis. Journal of the Royal Statistical Society: Series B (Statistical Methodology), 61(3), 611-622.
- Vasilescu, M. A. O. (2005). Multilinear principal component analysis. In Proceedings of the seventh IEEE workshops on applications of computer vision, 2005. WACV/MOTION’05 (Vol. 1, pp. 557-562). IEEE.
- Hyvärinen, A., & Oja, E. (2000). Independent component analysis: algorithms and applications. Neural networks, 13(4-5), 411-430.
- Fisher, R. A. (1936). The use of multiple measurements in taxonomic problems. Annals of eugenics, 7(2), 179-188.
- Lee, D. D., & Seung, H. S. (2001). Algorithms for non-negative matrix factorization. Advances in neural information processing systems, 13.
- Van der Maaten, L., & Hinton, G. (2008). Visualizing data using t-SNE. Journal of machine learning research, 9(11), 2579-2605.
- Goodfellow, I., Bengio, Y., & Courville, A. (2016). Deep learning. MIT press.
- Whitwell, J. L., et al. (2007). Distinct atrophy patterns in early-onset Alzheimer disease and posterior cortical atrophy. Archives of Neurology, 64(8), 1100-1108.
- Frisoni, G. B., et al. (2007). The clinical syndrome of posterior cortical atrophy. Lancet Neurology, 6(4), 341-349.
The discussion of future research directions is particularly interesting. Applying PCA to longitudinal neuroimaging data could provide valuable insights into disease progression. Has anyone explored using PCA in conjunction with time-series analysis to model the dynamic changes in brain activity associated with Posterior Cortical Atrophy?
Thanks for your insightful comment! The combination of PCA and time-series analysis for longitudinal data is a great point. We haven’t specifically explored that combination in our research on Posterior Cortical Atrophy, but it’s definitely on our radar for future studies. It could reveal nuanced dynamic changes. Has anyone here tried this approach?
Editor: MedTechNews.Uk
Thank you to our Sponsor Esdebe
The discussion around interpretable machine learning models based on PCA is compelling. Has anyone explored the use of explainable AI (XAI) techniques, like SHAP or LIME, to further enhance the interpretability of PCA-reduced neuroimaging data, particularly in the context of Posterior Cortical Atrophy?
Great point about XAI techniques like SHAP and LIME. We haven’t yet explored those specifically with PCA-reduced neuroimaging data for Posterior Cortical Atrophy, but it’s an exciting avenue! The ability to understand feature importance after dimensionality reduction could really boost clinical applications. I wonder how XAI handles the transformed feature space of PCA? Has anyone had practical experience with this?
Editor: MedTechNews.Uk
Thank you to our Sponsor Esdebe
Given PCA’s sensitivity to outliers, have you explored robust PCA methods, such as those employing M-estimation, to mitigate the impact of atypical neuroimaging data in Posterior Cortical Atrophy studies? How might these approaches affect the identification of disease-related patterns?