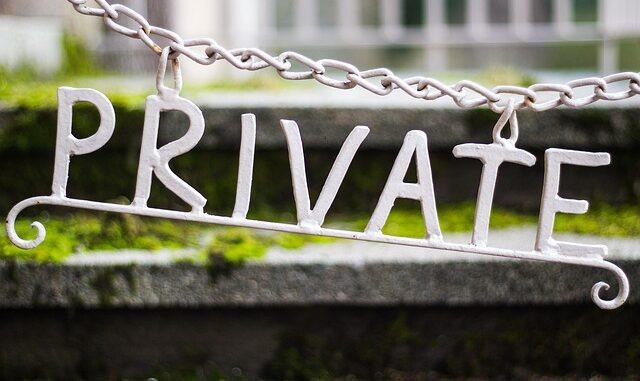
Privacy in the Age of Ubiquitous Data: A Comprehensive Analysis of Legal, Ethical, and Technological Dimensions
Abstract
In an era defined by unprecedented data generation and collection, privacy has emerged as a critical concern spanning legal, ethical, and technological domains. This research report provides a comprehensive analysis of the multifaceted challenges and opportunities surrounding privacy in the context of ubiquitous data. It explores the evolution and current state of legal frameworks governing data privacy, including the General Data Protection Regulation (GDPR), the California Consumer Privacy Act (CCPA), and other relevant legislation. The report delves into the ethical considerations surrounding data collection, use, and sharing, examining the potential for bias, discrimination, and erosion of autonomy. Furthermore, it investigates cutting-edge privacy-enhancing technologies (PETs) such as differential privacy, federated learning, and homomorphic encryption, assessing their strengths, limitations, and applicability across various sectors. The report also examines the challenges of balancing innovation with privacy, focusing on specific use cases such as healthcare, finance, and artificial intelligence. Finally, the report proposes a framework for responsible data governance, emphasizing transparency, accountability, and user empowerment, and suggesting avenues for future research and policy development.
Many thanks to our sponsor Esdebe who helped us prepare this research report.
1. Introduction
The digital revolution has ushered in an era of unprecedented data generation and collection. From social media interactions to sensor networks, from online transactions to healthcare records, data permeates nearly every aspect of modern life. This abundance of data holds immense potential for innovation, enabling advances in artificial intelligence, personalized medicine, targeted advertising, and countless other fields. However, the ubiquitous nature of data also poses significant challenges to individual privacy.
Privacy, traditionally defined as the right to be left alone, has evolved to encompass a broader set of concerns, including control over personal information, protection from unwanted surveillance, and freedom from discrimination based on data analysis. The increasing ease with which data can be collected, analyzed, and shared has heightened anxieties about the potential for misuse, abuse, and erosion of individual autonomy. News headlines are filled with stories of data breaches, surveillance scandals, and algorithmic bias, fueling public concern about the future of privacy.
This research report aims to provide a comprehensive analysis of the multifaceted challenges and opportunities surrounding privacy in the age of ubiquitous data. It will explore the evolution and current state of legal frameworks governing data privacy, delve into the ethical considerations surrounding data collection and use, and investigate cutting-edge privacy-enhancing technologies. Furthermore, the report will examine the challenges of balancing innovation with privacy, focusing on specific use cases such as healthcare, finance, and artificial intelligence. Finally, the report will propose a framework for responsible data governance, emphasizing transparency, accountability, and user empowerment.
Many thanks to our sponsor Esdebe who helped us prepare this research report.
2. Legal Frameworks for Data Privacy
Legal frameworks play a crucial role in defining and protecting data privacy rights. These frameworks establish rules and regulations governing the collection, use, storage, and sharing of personal information. Over the past several decades, numerous laws and regulations have been enacted at the national, regional, and international levels to address privacy concerns. This section examines some of the most significant legal frameworks for data privacy, including the GDPR, the CCPA, and other relevant legislation.
2.1 The General Data Protection Regulation (GDPR)
The General Data Protection Regulation (GDPR), which came into effect in May 2018, is a landmark piece of legislation that significantly strengthened data privacy rights for individuals in the European Union (EU). The GDPR applies to any organization that processes the personal data of EU residents, regardless of where the organization is located. Key provisions of the GDPR include:
- Data Minimization: Data should be adequate, relevant, and limited to what is necessary for the purposes for which they are processed (Article 5(1)(c)). This principle emphasizes that organizations should only collect the data they truly need and avoid collecting excessive or irrelevant information.
- Purpose Limitation: Data must be collected for specified, explicit, and legitimate purposes and not further processed in a manner incompatible with those purposes (Article 5(1)(b)). Organizations must clearly define the purposes for which they are collecting data and cannot use it for other, unrelated purposes without obtaining additional consent.
- Consent: Processing of personal data requires the explicit and informed consent of the data subject, which must be freely given, specific, informed, and unambiguous (Article 6(1)(a)). Consent must be affirmative and cannot be implied or inferred. Individuals have the right to withdraw their consent at any time.
- Right to Access: Individuals have the right to access their personal data and obtain information about how it is being processed (Article 15). Organizations must provide individuals with a copy of their data upon request.
- Right to Rectification: Individuals have the right to have inaccurate or incomplete personal data corrected (Article 16). Organizations must take reasonable steps to ensure that personal data is accurate and up-to-date.
- Right to Erasure (Right to be Forgotten): Individuals have the right to have their personal data erased under certain circumstances, such as when the data is no longer necessary for the purposes for which it was collected (Article 17). This right is not absolute and may be subject to certain exceptions.
- Data Portability: Individuals have the right to receive their personal data in a structured, commonly used, and machine-readable format and to transmit that data to another controller (Article 20).
- Data Protection Officer (DPO): Certain organizations are required to appoint a DPO to oversee data protection compliance (Article 37). DPOs are responsible for advising the organization on data protection matters, monitoring compliance, and acting as a point of contact for data protection authorities.
- Data Breach Notification: Organizations must notify data protection authorities of any data breach that is likely to result in a risk to the rights and freedoms of individuals (Article 33). In some cases, organizations may also be required to notify the affected individuals.
- Penalties: The GDPR imposes significant penalties for non-compliance, including fines of up to €20 million or 4% of the organization’s global annual turnover, whichever is higher (Article 83).
The GDPR has had a profound impact on data privacy practices worldwide, setting a new standard for data protection and influencing the development of similar legislation in other countries.
2.2 The California Consumer Privacy Act (CCPA) and California Privacy Rights Act (CPRA)
The California Consumer Privacy Act (CCPA), which came into effect in January 2020, is a state law that grants California consumers significant rights over their personal information. The CCPA applies to businesses that collect personal information of California residents and meet certain revenue or data processing thresholds. The California Privacy Rights Act (CPRA), which was approved by voters in November 2020 and went into effect January 1, 2023, amends and expands the CCPA, further strengthening consumer privacy rights.
Key provisions of the CCPA and CPRA include:
- Right to Know: Consumers have the right to know what personal information a business collects about them, the sources of the information, the purposes for which it is collected, and the categories of third parties with whom it is shared (Cal. Civ. Code § 1798.100).
- Right to Delete: Consumers have the right to request that a business delete their personal information, subject to certain exceptions (Cal. Civ. Code § 1798.105).
- Right to Opt-Out of Sale: Consumers have the right to opt-out of the sale of their personal information (Cal. Civ. Code § 1798.120). The CPRA expands this right to include the sharing of personal information for targeted advertising.
- Right to Correct: The CPRA introduces a new right to correct inaccurate personal information (Cal. Civ. Code § 1798.106).
- Right to Limit Use of Sensitive Personal Information: The CPRA grants consumers the right to limit the use and disclosure of their sensitive personal information, such as social security numbers, financial information, and health information (Cal. Civ. Code § 1798.121).
- Private Right of Action: Consumers have a private right of action for data breaches resulting from a business’s failure to implement reasonable security measures (Cal. Civ. Code § 1798.150).
- California Privacy Protection Agency (CPPA): The CPRA establishes a new state agency, the CPPA, to enforce the CCPA and CPRA and to issue regulations (Cal. Civ. Code § 1798.199.10).
The CCPA and CPRA have had a significant impact on data privacy practices in the United States, prompting businesses to re-evaluate their data collection and processing activities and to implement measures to comply with the new requirements. These laws have also served as a model for other states considering similar legislation.
2.3 Other Relevant Legislation
In addition to the GDPR and the CCPA/CPRA, numerous other laws and regulations address data privacy concerns in specific sectors or contexts. Some notable examples include:
- The Health Insurance Portability and Accountability Act (HIPAA): In the United States, HIPAA regulates the privacy and security of protected health information (PHI). It sets standards for the use and disclosure of PHI by healthcare providers, health plans, and healthcare clearinghouses. (45 CFR Parts 160 and 164)
- The Children’s Online Privacy Protection Act (COPPA): In the United States, COPPA protects the online privacy of children under the age of 13. It requires websites and online services to obtain parental consent before collecting, using, or disclosing personal information from children. (15 U.S.C. § 6501-6506)
- The Fair Credit Reporting Act (FCRA): In the United States, the FCRA regulates the collection, use, and disclosure of consumer credit information. It grants consumers the right to access their credit reports, to dispute inaccuracies, and to limit the use of their credit information. (15 U.S.C. § 1681 et seq.)
- The Electronic Communications Privacy Act (ECPA): In the United States, the ECPA protects the privacy of electronic communications, including email, telephone conversations, and data stored on computers. (18 U.S.C. § 2510 et seq.)
- The Personal Information Protection and Electronic Documents Act (PIPEDA): In Canada, PIPEDA governs the collection, use, and disclosure of personal information in the private sector. It is based on the ten principles of fair information practices outlined in the Canadian Standards Association’s Model Code for the Protection of Personal Information. (S.C. 2000, c. 5)
These are just a few examples of the many laws and regulations that address data privacy concerns around the world. The specific requirements and protections vary depending on the jurisdiction and the context.
Many thanks to our sponsor Esdebe who helped us prepare this research report.
3. Ethical Considerations in Data Privacy
Beyond legal compliance, ethical considerations play a crucial role in ensuring responsible data practices. Ethical frameworks provide guidance on how to balance the benefits of data collection and use with the potential risks to individual privacy and autonomy. This section explores some of the key ethical considerations surrounding data privacy, including informed consent, data security, bias and discrimination, and the right to be forgotten.
3.1 Informed Consent
Informed consent is a fundamental ethical principle that requires individuals to be informed about the potential risks and benefits of participating in a research study or data collection activity before agreeing to participate. In the context of data privacy, informed consent means that individuals should be informed about:
- What data is being collected: Individuals should be told what types of personal information are being collected, such as name, address, email address, browsing history, or location data.
- How the data will be used: Individuals should be told how their data will be used, such as for targeted advertising, personalized recommendations, or research purposes.
- Who will have access to the data: Individuals should be told who will have access to their data, such as third-party advertisers, researchers, or government agencies.
- How the data will be protected: Individuals should be told what security measures are in place to protect their data from unauthorized access, use, or disclosure.
- How long the data will be retained: Individuals should be told how long their data will be retained and how it will be disposed of when it is no longer needed.
- The risks and benefits of participating: Individuals should be informed about the potential risks and benefits of participating in the data collection activity. Risks might include identity theft, discrimination, or privacy violations. Benefits might include access to personalized services, improved products, or scientific advancements.
Obtaining informed consent can be challenging in the digital age, particularly when data is collected through complex and opaque algorithms. Traditional consent models, which often rely on lengthy and legalistic privacy policies, may not be effective in ensuring that individuals truly understand the implications of sharing their data. Alternative consent models, such as just-in-time notices and user-friendly privacy dashboards, may be more effective in empowering individuals to make informed decisions about their data.
3.2 Data Security
Data security is essential for protecting personal information from unauthorized access, use, or disclosure. Organizations have an ethical obligation to implement appropriate security measures to safeguard the data they collect and store. Data security measures can include:
- Encryption: Encrypting data both in transit and at rest can help protect it from unauthorized access.
- Access Controls: Limiting access to data to only those individuals who need it to perform their job duties can help prevent data breaches.
- Firewalls: Firewalls can help prevent unauthorized access to computer networks.
- Intrusion Detection Systems: Intrusion detection systems can help detect and prevent malicious activity on computer networks.
- Regular Security Audits: Regular security audits can help identify vulnerabilities in security systems and ensure that security measures are up-to-date.
- Employee Training: Employee training on data security best practices can help prevent accidental data breaches.
The increasing sophistication of cyberattacks and the growing volume of data being collected make data security a constant challenge. Organizations must continually invest in new security technologies and practices to stay ahead of the curve.
3.3 Bias and Discrimination
Data-driven decision-making has the potential to perpetuate and amplify existing biases and discrimination. Algorithms trained on biased data can produce discriminatory outcomes in areas such as hiring, lending, and criminal justice. Organizations have an ethical obligation to ensure that their data and algorithms are fair and unbiased. Addressing bias and discrimination in data requires:
- Data Auditing: Organizations should conduct regular audits of their data to identify and correct biases.
- Algorithm Transparency: Organizations should strive for transparency in their algorithms, so that users can understand how decisions are being made.
- Fairness Metrics: Organizations should use fairness metrics to evaluate the fairness of their algorithms and to identify and correct discriminatory outcomes.
- Diversity and Inclusion: Organizations should promote diversity and inclusion in their workforce, so that different perspectives are considered when developing and deploying data-driven systems.
The use of sensitive personal information, such as race, ethnicity, and gender, can be particularly problematic in data-driven decision-making. Organizations should carefully consider the potential for discrimination before using such information and should take steps to mitigate any potential risks.
3.4 The Right to be Forgotten
The right to be forgotten, also known as the right to erasure, is the right of individuals to have their personal data deleted from the internet and other online platforms. This right is recognized in the GDPR and other data privacy laws. The right to be forgotten is intended to give individuals more control over their online reputation and to prevent their past mistakes from haunting them indefinitely. The right to be forgotten is not absolute and may be subject to certain exceptions, such as when the data is necessary for freedom of expression or for compliance with a legal obligation.
Implementing the right to be forgotten can be technically challenging, particularly when data is distributed across multiple platforms and jurisdictions. Organizations must develop procedures for identifying and deleting personal data in a timely and effective manner. They must also consider the potential impact on freedom of expression and the public interest when processing requests for erasure.
Many thanks to our sponsor Esdebe who helped us prepare this research report.
4. Privacy-Enhancing Technologies (PETs)
Privacy-enhancing technologies (PETs) are a set of techniques designed to protect personal data and enhance privacy. These technologies can be used to minimize the amount of data collected, to anonymize or pseudonymize data, or to enable data analysis without revealing individual-level information. This section examines some of the most promising PETs, including differential privacy, federated learning, and homomorphic encryption.
4.1 Differential Privacy
Differential privacy (DP) is a mathematical framework for quantifying and controlling the privacy risks associated with data analysis. DP algorithms add carefully calibrated noise to the results of data queries to protect the privacy of individual data subjects. The amount of noise added is controlled by a privacy parameter, ε (epsilon), which determines the trade-off between privacy and accuracy. A smaller value of ε provides stronger privacy but may reduce the accuracy of the results.
DP provides a strong guarantee that the results of a data query will not reveal any sensitive information about any individual data subject. This guarantee holds even if an attacker has access to background knowledge about the data subjects.
DP has been used in a variety of applications, including census data analysis, location-based services, and machine learning. However, DP can be complex to implement and may require significant expertise in mathematics and statistics. Furthermore, DP may not be suitable for all types of data analysis, particularly those that require highly accurate results.
4.2 Federated Learning
Federated learning (FL) is a distributed machine learning technique that enables models to be trained on decentralized data sources without sharing the raw data. In FL, the model is trained locally on each data source, and only the model updates are shared with a central server. The central server aggregates the model updates and sends the updated model back to the local data sources. This process is repeated iteratively until the model converges.
FL offers several advantages over traditional machine learning approaches. First, it protects the privacy of individual data subjects by keeping the raw data on the local devices. Second, it enables models to be trained on large and diverse datasets that may be distributed across multiple organizations. Third, it reduces the communication costs associated with transferring large amounts of data to a central server.
FL has been used in a variety of applications, including mobile phone keyboard prediction, medical image analysis, and fraud detection. However, FL also poses several challenges, including dealing with heterogeneous data sources, ensuring the security of the model updates, and addressing the potential for bias in the training data.
4.3 Homomorphic Encryption
Homomorphic encryption (HE) is a form of encryption that allows computations to be performed on encrypted data without decrypting it first. This means that data can be processed and analyzed without revealing the underlying information. HE offers a strong guarantee of data privacy, as the data remains encrypted throughout the entire processing pipeline.
HE has been used in a variety of applications, including secure cloud computing, private information retrieval, and secure multiparty computation. However, HE is computationally expensive and may not be suitable for all types of computations. Furthermore, HE is still a relatively new technology and is not yet widely adopted.
4.4 Other PETs
In addition to differential privacy, federated learning, and homomorphic encryption, there are many other PETs that can be used to protect data privacy. Some notable examples include:
- Anonymization: Anonymization is the process of removing identifying information from data, such as name, address, and social security number. Anonymization can be used to protect the privacy of individuals while still allowing the data to be used for research or other purposes. However, anonymization is not always effective, as re-identification attacks can sometimes be used to recover the original identities of the data subjects.
- Pseudonymization: Pseudonymization is the process of replacing identifying information with pseudonyms, such as random numbers or codes. Pseudonymization can be used to protect the privacy of individuals while still allowing the data to be linked across different datasets. However, pseudonymization is not as strong as anonymization, as the pseudonyms can sometimes be linked back to the original identities of the data subjects.
- Secure Multiparty Computation (SMC): SMC is a cryptographic technique that allows multiple parties to compute a function on their private data without revealing the data to each other. SMC can be used to perform complex computations while protecting the privacy of all parties involved.
- Zero-Knowledge Proofs (ZKPs): ZKPs are a cryptographic technique that allows one party to prove to another party that a statement is true without revealing any information about why it is true. ZKPs can be used to verify the correctness of data or computations without revealing the underlying information.
Many thanks to our sponsor Esdebe who helped us prepare this research report.
5. Balancing Innovation and Privacy
Balancing innovation and privacy is a complex challenge that requires careful consideration of the potential benefits and risks of data collection and use. On the one hand, data-driven innovation can lead to significant advancements in healthcare, finance, education, and other fields. On the other hand, unchecked data collection and use can erode individual privacy, perpetuate biases, and lead to discrimination. Striking the right balance between innovation and privacy requires a multi-faceted approach that involves legal frameworks, ethical guidelines, privacy-enhancing technologies, and responsible data governance.
5.1 Use Case: Healthcare
The healthcare sector is a prime example of the challenges and opportunities of balancing innovation and privacy. Data from electronic health records (EHRs), wearable devices, and genomic sequencing can be used to develop new treatments, improve patient care, and personalize medicine. However, healthcare data is also highly sensitive and requires strong privacy protections.
HIPAA provides a legal framework for protecting the privacy of protected health information (PHI) in the United States. However, HIPAA may not be sufficient to address all of the privacy challenges posed by new technologies, such as artificial intelligence and machine learning. PETs, such as differential privacy and federated learning, can be used to enable data analysis without compromising individual patient privacy. Ethical guidelines, such as the principles of beneficence, non-maleficence, autonomy, and justice, can provide further guidance on how to balance the benefits of data use with the risks to individual privacy and well-being.
5.2 Use Case: Finance
The finance sector is another area where balancing innovation and privacy is critical. Data from credit card transactions, bank accounts, and investment portfolios can be used to detect fraud, personalize financial services, and improve risk management. However, financial data is also highly sensitive and requires strong privacy protections.
Numerous laws and regulations, such as the Gramm-Leach-Bliley Act (GLBA) and the Fair Credit Reporting Act (FCRA), govern the collection, use, and disclosure of financial data. PETs, such as homomorphic encryption and secure multiparty computation, can be used to enable data analysis without revealing individual financial information. Ethical guidelines, such as the principles of honesty, fairness, and transparency, can provide further guidance on how to balance the benefits of data use with the risks to individual privacy and financial security.
5.3 Use Case: Artificial Intelligence
Artificial intelligence (AI) raises unique privacy challenges due to its ability to infer sensitive information from seemingly innocuous data. For example, AI algorithms can be used to predict an individual’s sexual orientation, political views, or health status based on their online activity. This raises concerns about the potential for discrimination and manipulation.
Addressing the privacy challenges posed by AI requires a combination of legal frameworks, ethical guidelines, and technical solutions. Data minimization, algorithm transparency, and fairness metrics can help mitigate the potential for bias and discrimination in AI systems. Privacy-preserving AI techniques, such as differential privacy and federated learning, can enable AI models to be trained on sensitive data without compromising individual privacy. Furthermore, explainable AI (XAI) techniques can help users understand how AI systems are making decisions, which can increase trust and accountability.
Many thanks to our sponsor Esdebe who helped us prepare this research report.
6. Responsible Data Governance
Responsible data governance is essential for ensuring that data is collected, used, and shared in a way that respects individual privacy and promotes societal good. Responsible data governance involves establishing clear policies and procedures, implementing appropriate security measures, and promoting transparency and accountability. This section proposes a framework for responsible data governance, emphasizing transparency, accountability, and user empowerment.
6.1 Transparency
Transparency is a key principle of responsible data governance. Organizations should be transparent about what data they collect, how they use it, and who they share it with. This requires providing clear and accessible privacy policies, disclosing data breaches promptly, and being responsive to user inquiries about data practices.
Transparency can be enhanced through the use of privacy dashboards, which allow users to see what data is being collected about them and how it is being used. Transparency can also be enhanced through the use of open data initiatives, which make government data available to the public for research and other purposes.
6.2 Accountability
Accountability is another key principle of responsible data governance. Organizations should be accountable for their data practices and should be held responsible for any harm that results from their misuse of data. This requires establishing clear lines of responsibility, implementing appropriate oversight mechanisms, and providing remedies for data breaches and privacy violations.
Accountability can be enhanced through the appointment of data protection officers (DPOs), who are responsible for overseeing data protection compliance within an organization. Accountability can also be enhanced through the use of independent audits, which can assess an organization’s data practices and identify areas for improvement.
6.3 User Empowerment
User empowerment is essential for ensuring that individuals have control over their personal data. This requires providing users with the right to access, correct, and delete their data. It also requires providing users with the right to opt-out of data collection and use. User empowerment can be enhanced through the use of privacy-enhancing technologies, such as differential privacy and federated learning.
User empowerment also requires providing users with education and awareness about data privacy issues. This can be achieved through public service announcements, educational programs, and online resources.
Many thanks to our sponsor Esdebe who helped us prepare this research report.
7. Conclusion
Privacy in the age of ubiquitous data presents a complex and multifaceted challenge. This report has explored the legal, ethical, and technological dimensions of data privacy, highlighting the need for a comprehensive and multi-faceted approach to protecting individual privacy while enabling innovation. Legal frameworks, such as the GDPR and the CCPA/CPRA, provide a foundation for data privacy protection, but they must be continually updated to address new technologies and data practices. Ethical guidelines, such as the principles of informed consent, data security, and fairness, provide further guidance on how to balance the benefits of data use with the risks to individual privacy and autonomy. Privacy-enhancing technologies, such as differential privacy, federated learning, and homomorphic encryption, offer promising tools for protecting data privacy while enabling data analysis. Responsible data governance, based on the principles of transparency, accountability, and user empowerment, is essential for ensuring that data is collected, used, and shared in a way that respects individual privacy and promotes societal good.
Many thanks to our sponsor Esdebe who helped us prepare this research report.
8. Future Research and Policy Development
Future research and policy development should focus on the following areas:
- Developing more effective privacy-enhancing technologies: There is a need for more efficient and scalable PETs that can be used in a wider range of applications.
- Developing more robust methods for detecting and mitigating bias in AI systems: More research is needed on how to identify and correct biases in data and algorithms.
- Developing more effective methods for promoting transparency and accountability in data practices: More research is needed on how to provide users with more information about how their data is being used and to hold organizations accountable for their data practices.
- Developing more effective methods for educating and empowering users about data privacy issues: More research is needed on how to raise awareness about data privacy issues and to empower users to take control of their personal data.
- Harmonizing data privacy laws and regulations across different jurisdictions: Greater harmonization of data privacy laws and regulations would facilitate international data flows and reduce compliance costs for businesses.
- Exploring new models of data governance that promote innovation and protect privacy: New models of data governance are needed that can balance the benefits of data use with the risks to individual privacy and autonomy. This could include data trusts, data cooperatives, and other innovative approaches.
By focusing on these areas, we can create a future where data is used responsibly and ethically to benefit society while protecting individual privacy.
Many thanks to our sponsor Esdebe who helped us prepare this research report.
References
- California Consumer Privacy Act (CCPA). Cal. Civ. Code § 1798.100 et seq.
- California Privacy Rights Act (CPRA). Cal. Civ. Code § 1798.100 et seq.
- Children’s Online Privacy Protection Act (COPPA). 15 U.S.C. § 6501-6506.
- Electronic Communications Privacy Act (ECPA). 18 U.S.C. § 2510 et seq.
- Fair Credit Reporting Act (FCRA). 15 U.S.C. § 1681 et seq.
- General Data Protection Regulation (GDPR). Regulation (EU) 2016/679.
- Health Insurance Portability and Accountability Act (HIPAA). 45 CFR Parts 160 and 164.
- Dwork, C., & Roth, A. (2014). The algorithmic foundations of differential privacy. Foundations and Trends in Theoretical Computer Science, 9(3-4), 211-407.
- Hardt, M., Price, E., & Recht, B. (2016). Equality of opportunity in supervised learning. Advances in Neural Information Processing Systems, 29.
- Li, Q., Dwork, C., McSherry, F., & Roth, A. (2010). Differential privacy meets machine learning: A survey and perspective. Microsoft Research.
- McMahan, B., Moore, E., Ramage, D., Hampson, S., & Arcas, B. A. (2017). Communication-efficient learning of deep networks from decentralized data. Artificial Intelligence and Statistics, 1273-1282.
- Naehrig, M., Lauter, K., & Vaikuntanathan, V. (2012). Can homomorphic encryption be practical? Proceedings of the 3rd ACM workshop on Cloud computing security workshop, 113-124.
- Personal Information Protection and Electronic Documents Act (PIPEDA). S.C. 2000, c. 5.
The discussion of balancing innovation with privacy, particularly regarding AI’s ability to infer sensitive information, is critical. What safeguards can be implemented to ensure AI’s benefits are realized without enabling discriminatory practices based on these inferences?