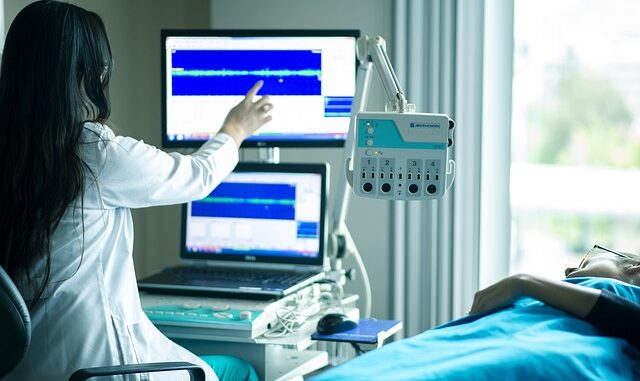
Abstract
Autoimmune diseases, a diverse group of disorders characterized by the immune system attacking the body’s own tissues, present a significant diagnostic and therapeutic challenge. The complexity of these diseases, coupled with their heterogeneous presentation and often insidious onset, contributes to delayed or inaccurate diagnoses. This report provides a comprehensive overview of autoimmune diseases, exploring their various types, prevalence, current diagnostic approaches, and the intricate interplay of genetic and environmental factors that contribute to their development. Furthermore, we critically analyze the potential of artificial intelligence (AI) and advanced immune fingerprinting techniques, exemplified by approaches like Mal-ID, to revolutionize the diagnosis and management of these conditions. Specifically, we examine how AI-driven analysis of complex immune profiles can facilitate earlier and more accurate diagnoses, enabling personalized treatment strategies tailored to individual patient needs. Finally, the report addresses the ethical considerations associated with the implementation of AI in autoimmune disease diagnosis, highlighting the need for responsible development and deployment of these technologies.
Many thanks to our sponsor Esdebe who helped us prepare this research report.
1. Introduction
Autoimmune diseases represent a significant burden on global healthcare systems. Affecting an estimated 3-5% of the population in developed countries, these disorders encompass a wide spectrum of conditions, including rheumatoid arthritis (RA), systemic lupus erythematosus (SLE), Type 1 diabetes mellitus (T1DM), multiple sclerosis (MS), and inflammatory bowel disease (IBD). The common thread uniting these diverse conditions is a breakdown in immunological tolerance, leading to the immune system mistakenly targeting and attacking the body’s own tissues and organs. This aberrant immune response results in chronic inflammation, tissue damage, and ultimately, organ dysfunction. The pathogenesis of autoimmune diseases is multifactorial, involving a complex interplay of genetic predisposition, environmental triggers, and stochastic events.
The diagnosis of autoimmune diseases is often challenging due to the heterogeneous nature of these conditions, their overlapping clinical presentations, and the lack of highly specific biomarkers. Current diagnostic approaches typically rely on a combination of clinical assessment, serological testing for autoantibodies, and imaging studies. However, these methods often lack the sensitivity and specificity required for early and accurate diagnosis, leading to diagnostic delays and suboptimal treatment outcomes. Furthermore, traditional diagnostic approaches often fail to capture the dynamic and personalized nature of immune responses in individual patients. This is where the potential of novel technologies such as artificial intelligence (AI) and immune fingerprinting comes into play. By leveraging the power of machine learning algorithms to analyze complex immune profiles, AI-driven diagnostic tools offer the promise of earlier and more accurate diagnoses, enabling personalized treatment strategies tailored to individual patient needs.
Many thanks to our sponsor Esdebe who helped us prepare this research report.
2. Types and Prevalence of Autoimmune Diseases
The landscape of autoimmune diseases is remarkably diverse, encompassing over 80 distinct conditions that affect a wide range of organ systems. Some of the most prevalent and well-studied autoimmune diseases include:
- Rheumatoid Arthritis (RA): A chronic inflammatory disorder primarily affecting the joints, characterized by synovial inflammation and progressive joint destruction. The global prevalence of RA is estimated to be between 0.5% and 1% [1].
- Systemic Lupus Erythematosus (SLE): A chronic, systemic autoimmune disease that can affect multiple organs, including the skin, joints, kidneys, brain, and blood vessels. The prevalence of SLE varies geographically, with higher rates observed in certain ethnic groups [2].
- Type 1 Diabetes Mellitus (T1DM): An autoimmune disease characterized by the destruction of insulin-producing beta cells in the pancreas, leading to insulin deficiency and hyperglycemia. The global incidence of T1DM is increasing, particularly in children and adolescents [3].
- Multiple Sclerosis (MS): A chronic, inflammatory disease of the central nervous system (CNS), characterized by demyelination and axonal damage. The prevalence of MS varies geographically, with higher rates observed in regions further from the equator [4].
- Inflammatory Bowel Disease (IBD): A group of chronic inflammatory conditions affecting the gastrointestinal tract, including Crohn’s disease and ulcerative colitis. The prevalence of IBD is increasing globally, particularly in industrialized countries [5].
- Psoriasis: A chronic skin condition characterized by scaly, red patches. It often occurs with psoriatic arthritis.
- Hashimoto’s Thyroiditis: An autoimmune disorder that causes chronic inflammation of the thyroid gland, leading to hypothyroidism.
The prevalence of autoimmune diseases varies significantly depending on the specific condition, geographic location, and ethnic background. Women are disproportionately affected by many autoimmune diseases, particularly SLE, RA, and autoimmune thyroid diseases. This gender bias is thought to be related to hormonal influences and sex chromosome effects on the immune system. Understanding the epidemiology of autoimmune diseases is crucial for developing effective public health strategies for prevention, early detection, and management.
Many thanks to our sponsor Esdebe who helped us prepare this research report.
3. Current Diagnostic Methods
The diagnosis of autoimmune diseases typically involves a comprehensive evaluation that includes:
- Clinical Assessment: A detailed medical history and physical examination are essential for identifying signs and symptoms suggestive of an autoimmune disorder. However, the clinical presentation of autoimmune diseases can be highly variable and often overlaps with other conditions, making diagnosis challenging.
- Serological Testing: The detection of autoantibodies in serum is a cornerstone of autoimmune disease diagnosis. Common autoantibodies include antinuclear antibodies (ANAs), rheumatoid factor (RF), anti-cyclic citrullinated peptide (anti-CCP) antibodies, anti-double-stranded DNA (anti-dsDNA) antibodies, and anti-thyroid antibodies. However, the presence of autoantibodies does not always indicate active disease, and some individuals may have autoantibodies without developing clinical symptoms. Furthermore, the sensitivity and specificity of autoantibody assays can vary, leading to false-positive and false-negative results.
- Inflammatory Markers: Measuring inflammatory markers such as C-reactive protein (CRP) and erythrocyte sedimentation rate (ESR) can help assess the degree of systemic inflammation. However, these markers are non-specific and can be elevated in a variety of inflammatory conditions, limiting their diagnostic utility.
- Imaging Studies: Imaging modalities such as X-ray, ultrasound, MRI, and CT scans can be used to evaluate organ damage and inflammation in specific autoimmune diseases. For example, joint imaging is commonly used in the diagnosis of RA, while brain MRI is used in the diagnosis of MS.
- Biopsy: In some cases, a tissue biopsy may be necessary to confirm the diagnosis of an autoimmune disease. Biopsy samples are typically examined under a microscope to identify characteristic histopathological features of the disease.
Despite these diagnostic tools, the diagnosis of autoimmune diseases remains a significant challenge. The diagnostic process is often lengthy and involves multiple visits to different specialists. Diagnostic delays can lead to irreversible organ damage and increased morbidity. Furthermore, the lack of highly specific biomarkers for many autoimmune diseases contributes to diagnostic uncertainty and the potential for misdiagnosis.
Many thanks to our sponsor Esdebe who helped us prepare this research report.
4. Challenges in Diagnosis
Several factors contribute to the challenges in diagnosing autoimmune diseases:
- Heterogeneous Presentation: Autoimmune diseases can manifest with a wide range of symptoms, making it difficult to distinguish them from other conditions. The symptoms may also vary over time, making it challenging to establish a definitive diagnosis.
- Overlapping Symptoms: Many autoimmune diseases share similar symptoms, making it difficult to differentiate between them. For example, fatigue, joint pain, and skin rashes are common symptoms in multiple autoimmune diseases.
- Lack of Specific Biomarkers: Many autoimmune diseases lack highly specific biomarkers that can be used to confirm the diagnosis. The autoantibodies that are commonly used in diagnosis are often not specific for a particular disease and can be present in healthy individuals.
- Diagnostic Delays: The diagnostic process for autoimmune diseases is often lengthy, leading to significant delays in treatment. These delays can result in irreversible organ damage and increased morbidity.
- Subjectivity in Diagnosis: The diagnosis of autoimmune diseases often relies heavily on clinical judgment, which can be subjective and prone to bias. This subjectivity can lead to inconsistencies in diagnosis between different physicians.
- Rarity of Certain Autoimmune Diseases: Some autoimmune diseases are relatively rare, making it difficult for physicians to gain experience in diagnosing and managing these conditions. This lack of experience can contribute to diagnostic errors.
Many thanks to our sponsor Esdebe who helped us prepare this research report.
5. The Role of Genetics and Environmental Factors
The etiology of autoimmune diseases is complex and multifactorial, involving a complex interplay of genetic predisposition, environmental triggers, and stochastic events.
- Genetic Predisposition: Genetic factors play a significant role in the susceptibility to autoimmune diseases. Genome-wide association studies (GWAS) have identified numerous genetic variants associated with increased risk of developing autoimmune diseases. Many of these variants are located in genes involved in immune regulation, such as the human leukocyte antigen (HLA) genes, which play a critical role in antigen presentation. However, genetic susceptibility is not deterministic, and most individuals with predisposing genes do not develop autoimmune diseases. This suggests that environmental factors and stochastic events are also necessary for disease development.
- Environmental Triggers: Environmental factors are thought to play a crucial role in triggering autoimmune responses in genetically susceptible individuals. These factors can include infections, exposure to toxins, drugs, and dietary factors. For example, infections with certain viruses, such as Epstein-Barr virus (EBV) and cytomegalovirus (CMV), have been linked to the development of autoimmune diseases. Exposure to cigarette smoke and air pollution has also been associated with increased risk of autoimmune diseases. Furthermore, dietary factors such as high salt intake and vitamin D deficiency have been implicated in the pathogenesis of autoimmune diseases.
- Epigenetics: Epigenetic modifications, such as DNA methylation and histone modification, can alter gene expression without changing the underlying DNA sequence. Epigenetic changes can be influenced by environmental factors and may contribute to the development of autoimmune diseases by altering the expression of genes involved in immune regulation.
- The Microbiome: The gut microbiome, the community of microorganisms that reside in the digestive tract, plays a critical role in immune development and homeostasis. Alterations in the composition and function of the gut microbiome have been linked to the development of autoimmune diseases. For example, dysbiosis, an imbalance in the gut microbiome, has been associated with increased risk of IBD, RA, and T1DM. The gut microbiome can influence immune responses through a variety of mechanisms, including the production of metabolites that modulate immune cell function.
Many thanks to our sponsor Esdebe who helped us prepare this research report.
6. Potential Future Treatments
The treatment of autoimmune diseases has evolved significantly in recent years, with the development of new therapies that target specific components of the immune system. Current treatment strategies typically aim to suppress the immune system and reduce inflammation, but these treatments can have significant side effects. Future treatment approaches are likely to focus on more targeted and personalized therapies that address the underlying causes of autoimmune diseases.
Several promising areas of research include:
- Targeted Immunotherapies: Biologic therapies that specifically target cytokines (e.g., TNF-alpha, IL-6, IL-17) or immune cells (e.g., B cells, T cells) have revolutionized the treatment of many autoimmune diseases. Future therapies are likely to target other key molecules and pathways involved in autoimmune pathogenesis.
- Cell-Based Therapies: Cell-based therapies, such as stem cell transplantation and regulatory T cell therapy, hold promise for restoring immune tolerance and reversing autoimmune disease. These therapies are still in early stages of development, but have shown encouraging results in clinical trials.
- Gene Therapies: Gene therapies aim to correct genetic defects that contribute to autoimmune disease susceptibility. These therapies are still in early stages of development, but have the potential to provide long-term cures for certain autoimmune diseases.
- Personalized Medicine: Personalized medicine approaches aim to tailor treatment strategies to individual patient characteristics, such as their genetic profile, immune status, and disease subtype. This approach is likely to improve treatment outcomes and reduce side effects.
- Microbiome Modulation: Strategies to modulate the gut microbiome, such as fecal microbiota transplantation (FMT) and dietary interventions, are being explored as potential therapies for autoimmune diseases. These approaches aim to restore a healthy gut microbiome and improve immune function.
Many thanks to our sponsor Esdebe who helped us prepare this research report.
7. AI and Immune Fingerprinting in Autoimmune Disease Diagnosis
Artificial intelligence (AI) and immune fingerprinting are emerging as powerful tools for improving the diagnosis and management of autoimmune diseases. AI algorithms can analyze complex immune profiles, including data from serological tests, flow cytometry, and gene expression studies, to identify patterns that are indicative of autoimmune disease. Immune fingerprinting techniques, such as the Mal-ID approach, aim to characterize the unique immune signatures of individual patients, providing insights into disease pathogenesis and potential therapeutic targets.
- AI-Powered Diagnosis: AI algorithms can be trained on large datasets of patient data to identify patterns that are associated with specific autoimmune diseases. These algorithms can then be used to predict the likelihood of a patient having a particular disease based on their clinical and laboratory findings. AI-powered diagnostic tools have the potential to improve the accuracy and speed of diagnosis, reducing diagnostic delays and improving patient outcomes.
- Immune Fingerprinting: Immune fingerprinting techniques aim to characterize the unique immune signatures of individual patients by analyzing a comprehensive panel of immune markers. These markers can include autoantibodies, cytokines, chemokines, and immune cell populations. By analyzing these complex immune profiles, researchers can identify patterns that are associated with specific autoimmune diseases and predict disease progression and treatment response.
- Personalized Treatment Strategies: AI and immune fingerprinting can be used to develop personalized treatment strategies for autoimmune diseases. By identifying the specific immune pathways that are dysregulated in individual patients, clinicians can select the most appropriate therapies and monitor treatment response. This approach has the potential to improve treatment outcomes and reduce side effects.
- Mal-ID and Similar Approaches: Approaches like Mal-ID use machine learning to analyze patterns of immune cell activation and cytokine production in response to various stimuli. This provides a functional assessment of the immune system that can be more sensitive and specific than traditional autoantibody testing. Such approaches can help identify individuals at high risk of developing autoimmune diseases before clinical symptoms appear, allowing for early intervention.
AI and immune fingerprinting are not without their limitations. The development of accurate and reliable AI-powered diagnostic tools requires large datasets of high-quality data. Furthermore, the interpretation of complex immune profiles requires expertise in immunology and bioinformatics. However, as these technologies continue to develop, they have the potential to revolutionize the diagnosis and management of autoimmune diseases.
Many thanks to our sponsor Esdebe who helped us prepare this research report.
8. Ethical Considerations of Using AI in Autoimmune Disease Diagnosis
The use of AI in autoimmune disease diagnosis raises several ethical considerations that must be addressed to ensure responsible development and deployment of these technologies. These considerations include:
- Data Privacy and Security: AI algorithms require large datasets of patient data to be trained. This data must be protected to ensure patient privacy and security. Data breaches could compromise sensitive patient information and erode public trust in AI-powered diagnostic tools. Robust data security measures and privacy policies are essential to mitigate these risks.
- Bias and Fairness: AI algorithms can be biased if they are trained on datasets that do not accurately represent the diversity of the population. This bias can lead to inaccurate diagnoses and unequal access to care for certain groups of patients. It is important to ensure that AI algorithms are trained on diverse datasets and that their performance is evaluated across different populations.
- Transparency and Explainability: The decision-making processes of AI algorithms can be opaque and difficult to understand. This lack of transparency can make it difficult for clinicians to trust the results of AI-powered diagnostic tools. It is important to develop AI algorithms that are transparent and explainable, allowing clinicians to understand how the algorithm arrived at its conclusions.
- Autonomy and Accountability: The use of AI in diagnosis raises questions about the autonomy and accountability of clinicians. If clinicians rely too heavily on AI-powered diagnostic tools, they may lose their own clinical judgment and become overly dependent on the technology. It is important to ensure that clinicians retain their autonomy and that they are ultimately accountable for the decisions they make.
- Access and Equity: The development and deployment of AI-powered diagnostic tools could exacerbate existing health inequities if these technologies are not accessible to all patients. It is important to ensure that AI-powered diagnostic tools are affordable and accessible to patients in all geographic locations and socioeconomic backgrounds.
Addressing these ethical considerations is essential to ensure that AI is used responsibly and ethically in autoimmune disease diagnosis. This requires collaboration between researchers, clinicians, policymakers, and patients to develop ethical guidelines and regulatory frameworks that promote the safe and equitable use of AI in healthcare.
Many thanks to our sponsor Esdebe who helped us prepare this research report.
9. Conclusion
Autoimmune diseases represent a significant diagnostic and therapeutic challenge due to their complexity, heterogeneity, and often insidious onset. Current diagnostic methods often lack the sensitivity and specificity required for early and accurate diagnosis, leading to diagnostic delays and suboptimal treatment outcomes. The development of AI-powered immune fingerprinting technologies, such as the Mal-ID approach, holds great promise for revolutionizing the diagnosis and management of these conditions. By leveraging the power of machine learning algorithms to analyze complex immune profiles, AI-driven diagnostic tools offer the potential for earlier and more accurate diagnoses, enabling personalized treatment strategies tailored to individual patient needs. However, the implementation of AI in autoimmune disease diagnosis raises several ethical considerations that must be addressed to ensure responsible development and deployment of these technologies. By addressing these challenges and embracing the potential of AI and immune fingerprinting, we can pave the way for a new era of precision medicine in autoimmune disease management.
Many thanks to our sponsor Esdebe who helped us prepare this research report.
References
[1] Cross M, Smith E, Hoy D, et al. The global prevalence of rheumatoid arthritis: a systematic review and meta-analysis. Arthritis Rheumatol. 2014;66(5):1223-1233. doi:10.1002/art.38462
[2] Pons-Estel BA, Alarcón GS, Scofield L, et al. Systemic lupus erythematosus global prevalence: systematic review and bayesian meta-analysis. Autoimmun Rev. 2010;9(9):557-568. doi:10.1016/j.autrev.2010.03.010
[3] Mobasseri M, Shirmohammadi M, Amiri M, Najafi M, Salmanpour MS. Prevalence and incidence of type 1 diabetes in the world: a systematic review and meta-analysis. Health Promot Perspect. 2020;10(2):98-115. doi:10.34172/hpp.2020.18
[4] Walton C, King R, Rechtman L, et al. Rising prevalence of multiple sclerosis worldwide: Problems in establishing true increases. Mult Scler. 2015;21(8):1033-1043. doi:10.1177/1352458515570330
[5] Molodecky NA, Soon IS, Rabi DM, et al. Increasing incidence and prevalence of the inflammatory bowel diseases with time, based on systematic review. Gastroenterology. 2012;142(1):46-54.e42; quiz e30. doi:10.1053/j.gastro.2011.10.001
[6] Smolen JS, Aletaha D, Barton A, et al. Rheumatoid Arthritis. Nat Rev Dis Primers. 2018;4:18001. Published 2018 Feb 15. doi:10.1038/nrdp.2018.1
[7] Anders HJ, Saxena R. Systemic lupus erythematosus. Kidney Int. 2023;103(3):472-482. doi:10.1016/j.kint.2022.09.026
[8] Insel RA, Dunne JL, Atkinson MA, et al. Type 1 diabetes immunopathology: new insights. Cold Spring Harb Perspect Med. 2020;10(10):a036922. Published 2020 Oct 1. doi:10.1101/cshperspect.a036922
[9] Reich DS, Lucchinetti CF, Calabresi PA. Multiple Sclerosis. N Engl J Med. 2018;378(2):169-180. doi:10.1056/NEJMra1401483
[10] Ungaro R, Mehandru S, Allen PB, Peyrin-Biroulet L, Colombel JF. Ulcerative colitis. Lancet. 2017;389(10080):1756-1770. doi:10.1016/S0140-6736(16)32126-5
[11] Rutter MD. Colorectal cancer risk in inflammatory bowel disease: What is the evidence?. Dig Dis. 2012;30 Suppl 3:44-8. doi: 10.1159/000341708.
[12] Hedström AK, Olsson T, Alfredsson L. Smoking and risk of multiple sclerosis. Mult Scler. 2009;15(4):417-24. doi: 10.1177/1352458508099588.
[13] Costenbader KH, Karlson EW. Cigarette smoking and systemic lupus erythematosus. Curr Rheumatol Rep. 2006;8(4):260-3.
[14] Okada Y, Wu D, Trynka G, et al. Genetics of rheumatoid arthritis contributes to biology and drug discovery. Nature. 2014;506(7488):376-381. doi:10.1038/nature12873
[15] Gianchecchi E, Fierabracci A. Recent Advances in Understanding the Molecular Mechanisms Orchestrating the Gut Microbiota Influence on Autoimmunity. Clin Rev Allergy Immunol. 2019;56(3):318-332. doi:10.1007/s12016-018-8709-9
[16] Arboleya S, Arribas B, Puente SL, et al. Intestinal Microbiota Development in Infancy: Impact of Mode of Delivery and Pre-Term Birth. Front Immunol. 2018;9:2192. Published 2018 Sep 27. doi:10.3389/fimmu.2018.02192
[17] Hu J, Elinav E, Huber S, et al. Mucin degradation, a sensitive marker of intestinal inflammation and dysbiosis, as a possible prognostic marker for inflammatory bowel diseases. Gut. 2016;65(9):1416-1417.
[18] Lee WJ, Haskard DO. The role of inflammation in the pathogenesis of type 2 diabetes. Diabet Med. 2012;29(9):1077-83. doi: 10.1111/j.1464-5491.2012.03673.x.
[19] Danese S, Fiocchi C. Ulcerative colitis. N Engl J Med. 2011;365(18):1713-25. doi: 10.1056/NEJMra1102942.
[20] National Institute of Allergy and Infectious Diseases. (2023). Autoimmune Diseases. https://www.niaid.nih.gov/diseases-conditions/autoimmune-diseases
AI diagnosing autoimmune diseases? How long until my fitness tracker starts telling me I’m allergic to Mondays and need a personalized algorithm-approved nap schedule? And what happens when the AI decides my immune system needs an upgrade I can’t afford?