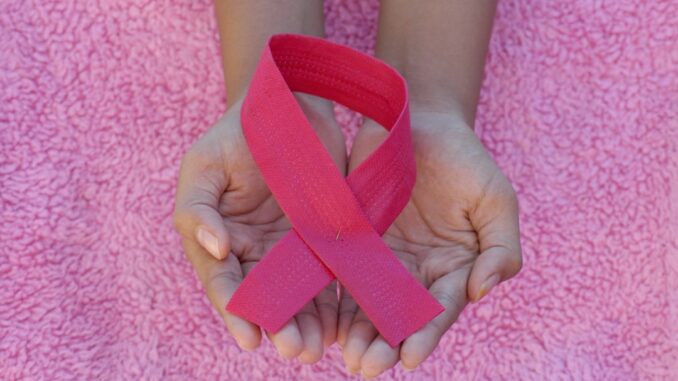
Abstract
Cancer, a complex and multifaceted disease, remains a leading cause of mortality worldwide. Its inherent heterogeneity, both inter- and intra-tumoral, poses significant challenges to accurate diagnosis, effective treatment, and ultimately, improved patient outcomes. This research report delves into the intricacies of cancer biology, examining the molecular mechanisms driving tumorigenesis and the complexities of the tumor microenvironment. We explore the limitations of conventional diagnostic and therapeutic approaches, highlighting the urgent need for innovative strategies that address the unique characteristics of individual cancers. The report further investigates the transformative potential of multi-omics data integration and artificial intelligence (AI) in advancing precision oncology. We discuss the application of AI-driven tools for early detection, personalized treatment selection, and the identification of novel therapeutic targets. Finally, we address the challenges and future directions in the field, emphasizing the importance of collaborative research efforts and the ethical considerations surrounding AI-driven healthcare.
Many thanks to our sponsor Esdebe who helped us prepare this research report.
1. Introduction
Cancer is not a single disease, but rather a collection of over 100 distinct diseases characterized by uncontrolled cell growth and the potential to invade other parts of the body. The global cancer burden is significant and increasing, with millions of new cases diagnosed each year [1]. Despite advances in cancer research and treatment, it remains a major public health challenge. The inherent complexity of cancer stems from its molecular heterogeneity, which manifests in diverse ways, including variations in gene expression, protein profiles, and metabolic pathways. This heterogeneity exists not only between different cancer types but also within individual tumors, making it difficult to develop universal diagnostic and therapeutic strategies [2].
Traditional approaches to cancer diagnosis and treatment have often relied on broad categorizations based on the site of origin and histological features. While these approaches have been successful in certain cases, they often fail to account for the underlying molecular drivers of the disease, leading to suboptimal outcomes for many patients. For example, two patients with the same type of breast cancer may respond differently to the same treatment due to variations in their tumor’s molecular characteristics [3].
The advent of high-throughput technologies, such as genomics, transcriptomics, proteomics, and metabolomics (collectively known as multi-omics), has provided unprecedented insights into the molecular complexity of cancer. These technologies generate vast amounts of data that can be used to characterize the unique molecular profiles of individual tumors. However, the sheer volume and complexity of multi-omics data pose significant challenges for analysis and interpretation. This is where artificial intelligence (AI) and machine learning (ML) come into play, offering powerful tools for integrating and analyzing multi-omics data to improve cancer diagnosis, treatment, and prevention [4].
Many thanks to our sponsor Esdebe who helped us prepare this research report.
2. The Molecular Landscape of Cancer: A Deep Dive
2.1 Hallmarks of Cancer
The ‘hallmarks of cancer’, first proposed by Hanahan and Weinberg, provide a conceptual framework for understanding the biological capabilities acquired during tumorigenesis. These hallmarks include:
- Sustaining proliferative signaling: Cancer cells acquire the ability to stimulate their own growth through autocrine signaling, growth factor receptor overexpression, or mutations in downstream signaling pathways [5].
- Evading growth suppressors: Cancer cells inactivate tumor suppressor genes, such as p53 and Rb, which normally regulate cell cycle progression and apoptosis [6].
- Resisting cell death: Cancer cells develop mechanisms to evade apoptosis, allowing them to survive and proliferate even in the presence of cellular stress or DNA damage [7].
- Enabling replicative immortality: Cancer cells reactivate telomerase, an enzyme that maintains telomere length, allowing them to bypass cellular senescence and achieve unlimited replicative potential [8].
- Inducing angiogenesis: Cancer cells stimulate the formation of new blood vessels (angiogenesis) to supply tumors with oxygen and nutrients, facilitating their growth and metastasis [9].
- Activating invasion and metastasis: Cancer cells acquire the ability to invade surrounding tissues and metastasize to distant sites, leading to the formation of secondary tumors [10].
More recent additions to the hallmarks include:
- Reprogramming energy metabolism: Cancer cells alter their metabolic pathways to support rapid growth and proliferation, often relying on glycolysis even in the presence of oxygen (the Warburg effect) [11].
- Evading immune destruction: Cancer cells develop mechanisms to evade detection and destruction by the immune system [12].
- Tumor-promoting inflammation: Inflammation can promote tumorigenesis by providing growth factors, cytokines, and other factors that stimulate cell proliferation, angiogenesis, and metastasis [13].
2.2 Genetic and Epigenetic Alterations
Cancer development is driven by a complex interplay of genetic and epigenetic alterations. Genetic mutations, such as point mutations, deletions, and amplifications, can directly alter the function of genes involved in cell growth, differentiation, and apoptosis. Epigenetic modifications, such as DNA methylation and histone modification, can alter gene expression without changing the underlying DNA sequence [14].
Driver mutations are genetic alterations that directly contribute to the development of cancer by conferring a selective growth advantage to cancer cells. These mutations often occur in oncogenes (genes that promote cell growth) or tumor suppressor genes (genes that inhibit cell growth). Passenger mutations are genetic alterations that do not directly contribute to cancer development but may arise as a consequence of genomic instability [15].
Epigenetic modifications play a critical role in regulating gene expression in cancer cells. Aberrant DNA methylation patterns, such as hypermethylation of tumor suppressor genes and hypomethylation of oncogenes, are commonly observed in cancer. Histone modifications, such as acetylation and methylation, can also alter chromatin structure and gene expression [16].
2.3 The Tumor Microenvironment
The tumor microenvironment (TME) is a complex ecosystem surrounding cancer cells that includes stromal cells (e.g., fibroblasts, immune cells, endothelial cells), extracellular matrix (ECM), and soluble factors (e.g., cytokines, growth factors). The TME plays a critical role in cancer development, progression, and response to therapy [17].
Stromal cells in the TME can either promote or inhibit cancer growth. For example, cancer-associated fibroblasts (CAFs) can secrete growth factors and ECM components that stimulate cancer cell proliferation, angiogenesis, and metastasis. Immune cells in the TME can either kill cancer cells or promote tumor growth by suppressing anti-tumor immunity [18].
The ECM provides structural support to the TME and can also influence cancer cell behavior. Alterations in ECM composition and organization can promote cancer cell invasion and metastasis. Soluble factors in the TME, such as cytokines and growth factors, can stimulate cancer cell proliferation, angiogenesis, and immune evasion [19].
Many thanks to our sponsor Esdebe who helped us prepare this research report.
3. Limitations of Current Diagnostic and Therapeutic Approaches
3.1 Diagnostic Challenges
Traditional cancer diagnostic methods, such as histopathology and imaging, have limitations in terms of sensitivity, specificity, and the ability to detect early-stage disease. Histopathology relies on the microscopic examination of tissue samples, which can be subjective and prone to inter-observer variability. Imaging techniques, such as X-rays, CT scans, and MRI, can detect tumors but may not be able to distinguish between benign and malignant lesions. Furthermore, these methods often fail to capture the molecular heterogeneity of cancer, leading to inaccurate diagnoses and inappropriate treatment decisions [20].
3.2 Therapeutic Challenges
Conventional cancer therapies, such as chemotherapy and radiation therapy, are often associated with significant side effects due to their lack of selectivity for cancer cells. These therapies can damage healthy cells, leading to a range of adverse effects, including nausea, fatigue, hair loss, and immune suppression. Furthermore, cancer cells can develop resistance to these therapies, limiting their long-term effectiveness [21].
The molecular heterogeneity of cancer also poses a significant challenge to effective treatment. Tumors with different molecular profiles may respond differently to the same therapy. Therefore, a one-size-fits-all approach to cancer treatment is often ineffective, leading to suboptimal outcomes for many patients. The ability to tailor treatment to the individual characteristics of a patient’s tumor is crucial for improving treatment outcomes [22].
Many thanks to our sponsor Esdebe who helped us prepare this research report.
4. The Rise of AI-Driven Precision Oncology
4.1 Multi-Omics Data Integration
Multi-omics data integration involves combining data from different omics platforms (e.g., genomics, transcriptomics, proteomics, metabolomics) to provide a comprehensive view of the molecular landscape of cancer. This approach can reveal complex relationships between different molecular layers and identify biomarkers that are not apparent when analyzing each data type separately. For example, integrating genomic and transcriptomic data can identify genes that are mutated and differentially expressed in cancer cells, providing insights into the molecular mechanisms driving tumorigenesis [23].
The challenge of multi-omics data integration lies in the high dimensionality and complexity of the data. Each omics platform generates vast amounts of data, and integrating these data requires sophisticated computational tools and statistical methods. AI and machine learning algorithms are particularly well-suited for this task, as they can automatically learn complex patterns from data and make predictions without explicit programming [24].
4.2 AI/ML Algorithms in Cancer Research
Several AI/ML algorithms have been successfully applied to cancer research, including:
- Supervised learning: Supervised learning algorithms are trained on labeled data (e.g., cancer vs. normal) to predict the class label of new, unseen data. These algorithms can be used for cancer diagnosis, prognosis, and treatment response prediction. Examples of supervised learning algorithms include support vector machines (SVMs), random forests, and neural networks [25].
- Unsupervised learning: Unsupervised learning algorithms are used to identify patterns in unlabeled data. These algorithms can be used for cancer subtype discovery, biomarker identification, and drug target identification. Examples of unsupervised learning algorithms include clustering algorithms (e.g., k-means) and dimensionality reduction techniques (e.g., principal component analysis) [26].
- Deep learning: Deep learning is a type of machine learning that uses artificial neural networks with multiple layers to learn complex patterns from data. Deep learning algorithms have shown remarkable performance in image recognition, natural language processing, and other tasks. In cancer research, deep learning can be used for image analysis (e.g., identifying cancer cells in histopathology images), genomics analysis (e.g., predicting gene expression from DNA sequence), and drug discovery (e.g., predicting drug efficacy) [27].
4.3 Applications of AI in Cancer
AI is being applied to various aspects of cancer research and clinical care, including:
- Early Detection: AI-powered tools are being developed to analyze medical images (e.g., mammograms, CT scans) to detect early signs of cancer. These tools can improve the accuracy and efficiency of cancer screening, leading to earlier diagnosis and improved patient outcomes [28].
- Diagnosis and Prognosis: AI algorithms can analyze multi-omics data to classify tumors into different subtypes and predict patient outcomes. This information can be used to personalize treatment decisions and improve patient survival [29].
- Personalized Treatment: AI can be used to predict which patients will respond to specific therapies based on their molecular profiles. This can help to avoid unnecessary treatment and side effects, and to select the most effective therapy for each patient [30].
- Drug Discovery: AI can accelerate the drug discovery process by identifying novel drug targets, predicting drug efficacy, and optimizing drug design [31].
4.4 OmicsFootPrint: An Example of AI-Powered Cancer Differentiation
Tools like OmicsFootPrint exemplify the application of AI in differentiating between cancer types with high accuracy. By integrating and analyzing various omics data, such tools can provide a comprehensive molecular profile of tumors, allowing for more precise classification and personalized treatment strategies. The ability to visualize and interpret complex data sets is crucial for clinicians and researchers alike, and platforms like OmicsFootPrint are paving the way for more effective cancer management [32].
Many thanks to our sponsor Esdebe who helped us prepare this research report.
5. Challenges and Future Directions
5.1 Data Availability and Quality
One of the major challenges in AI-driven cancer research is the limited availability and quality of data. Many cancer datasets are incomplete, biased, or lack standardized formats, which can hinder the development and validation of AI algorithms. Furthermore, data sharing is often restricted due to privacy concerns and intellectual property rights [33].
5.2 Interpretability and Explainability
Many AI algorithms, particularly deep learning models, are ‘black boxes,’ meaning that it is difficult to understand how they arrive at their predictions. This lack of interpretability can make it difficult for clinicians to trust AI-driven decisions and to identify potential biases in the algorithms. There is a growing need for more interpretable and explainable AI algorithms that can provide insights into the underlying biological mechanisms driving cancer [34].
5.3 Ethical Considerations
The use of AI in healthcare raises several ethical considerations, including data privacy, algorithmic bias, and the potential for job displacement. It is crucial to develop ethical guidelines and regulations to ensure that AI is used responsibly and equitably in cancer research and clinical care [35].
5.4 Future Directions
The future of AI-driven precision oncology is bright. As data availability and quality improve, and as AI algorithms become more sophisticated and interpretable, we can expect to see even more transformative applications of AI in cancer research and clinical care. Some promising future directions include:
- Development of AI-powered diagnostic tools for early cancer detection.
- Development of personalized treatment strategies based on multi-omics data and AI algorithms.
- Use of AI to identify novel drug targets and accelerate drug discovery.
- Integration of AI into clinical workflows to improve decision-making and patient outcomes.
- Development of more robust and ethical AI frameworks for healthcare.
Many thanks to our sponsor Esdebe who helped us prepare this research report.
6. Conclusion
Cancer remains a formidable challenge, demanding innovative approaches to diagnosis, treatment, and prevention. The integration of multi-omics data and AI offers a powerful means to overcome the limitations of conventional methods and to advance precision oncology. While challenges remain regarding data availability, interpretability, and ethical considerations, the potential benefits of AI in cancer research and clinical care are immense. By fostering collaborative research efforts and developing robust ethical guidelines, we can harness the power of AI to improve patient outcomes and ultimately conquer this devastating disease. The journey towards personalized cancer medicine, fueled by AI, holds the promise of a future where treatments are tailored to the unique molecular characteristics of each individual’s cancer, leading to more effective and less toxic therapies, and ultimately, saving lives.
Many thanks to our sponsor Esdebe who helped us prepare this research report.
References
[1] Sung, H., Ferlay, J., Siegel, R. L., Laversanne, M., Soerjomataram, I., Jemal, A., & Bray, F. (2021). Global cancer statistics 2020: GLOBOCAN estimates of incidence and mortality worldwide for 36 cancers in 185 countries. CA: a cancer journal for clinicians, 71(3), 209-249.
[2] Vogelstein, B., Papadopoulos, N., Velculescu, V. E., Zhou, S., Diaz Jr, L. A., & Kinzler, K. W. (2013). Cancer genome landscapes. Science, 339(6127), 1546-1558.
[3] Perou, C. M., Sørlie, T., Eisen, M. B., van de Rijn, M., Jeffrey, S. S., Rees, C. A., … & Borresen-Dale, A. L. (2000). Molecular portraits of human breast tumours. Nature, 406(6797), 747-752.
[4] Angione, C., Li, X., Ceccarelli, M., Giansanti, V., & Massucci, F. A. (2022). Artificial intelligence in cancer diagnosis and therapy. Computational and Structural Biotechnology Journal, 20, 4295-4309.
[5] Hanahan, D., & Weinberg, R. A. (2011). Hallmarks of cancer: the next generation. Cell, 144(5), 646-674.
[6] Vousden, K. H., & Prives, C. (2009). P53 and cancer: guilty by association. Cell, 137(3), 413-422.
[7] Lowe, S. W., Cepero, E., & Evan, G. (2004). Intrinsic tumour suppression. Nature, 432(7015), 307-315.
[8] Shay, J. W., & Wright, W. E. (2000). Hayflick, his limit, and cellular ageing. Nature Reviews Molecular Cell Biology, 1(1), 72-76.
[9] Folkman, J. (1971). Tumor angiogenesis: therapeutic implications. New England Journal of Medicine, 285(21), 1182-1186.
[10] Valastyan, S., & Weinberg, R. A. (2011). Tumor metastasis: molecular perspectives. Advances in Cancer Research, 111, 1-24.
[11] Vander Heiden, M. G., Cantley, L. C., & Thompson, C. B. (2009). Understanding the Warburg effect: the metabolic requirements of cell proliferation. Science, 324(5930), 1029-1033.
[12] Schreiber, R. D., Old, L. J., & Smyth, M. J. (2011). Cancer immunoediting: integrating immunity’s roles in cancer suppression and promotion. Science, 331(6024), 1565-1570.
[13] Mantovani, A., Allavena, P., Sica, A., & Balkwill, F. (2008). Cancer-related inflammation. Nature, 454(7203), 436-444.
[14] Baylin, S. B., & Jones, P. A. (2011). A decade of exploring the cancer epigenome–biological and translational implications. Nature Reviews Cancer, 11(10), 726-734.
[15] Stratton, M. R., Campbell, P. J., & Futreal, P. A. (2009). The cancer genome. Nature, 458(7239), 719-724.
[16] Dawson, M. A., Kouzarides, T., & Huntly, B. J. (2011). Targeting epigenetic readers in cancer. New England Journal of Medicine, 364(19), 1828-1837.
[17] Bissell, M. J., & Hines, W. C. (2011). Why don’t we get more cancer? A proposed role of the microenvironment in restraining cancer progression. Nature Medicine, 17(3), 320-329.
[18] Kalluri, R. (2016). The biology and function of fibroblasts in cancer. Nature Reviews Cancer, 16(9), 582-598.
[19] Lu, P., Weaver, V. M., & Werb, Z. (2012). The extracellular matrix: a dynamic niche in cancer progression. Journal of Cell Biology, 196(4), 395-406.
[20] Pantanowitz, L., Bui, M. M., Valenstein, P. N., Evans, A. J., Kaplan, K. J., Pfeifer, J. D., … & Wilbur, D. C. (2020). Introduction to digital pathology. Archives of Pathology & Laboratory Medicine, 144(12), 1433-1442.
[21] Chabner, B. A., & Roberts, T. G. (2005). Timeline: chemotherapy and the war on cancer. Nature Reviews Cancer, 5(1), 65-75.
[22] Jameson, J. L., & Longo, D. L. (2015). Precision medicine—personalized, problematic, and promising. New England Journal of Medicine, 372(23), 2229-2234.
[23] Subramanian, I., Verma, S., Kumar, S., Anamika, K., Tomar, S., & Ojha, A. (2020). Multi-omics data integration, interpretation, and its application. Bioinformatics, 36(1), 3-14.
[24] Danaee, P., Ghasemi, M., Hendouei, N., Montazeri, M., & Faghihi, F. (2017). A survey of data mining techniques for diagnosis of cancer. Artificial intelligence in medicine, 76, 41-59.
[25] Kourou, K., Exarchos, T. P., Exarchos, K. P., Karamouzis, M. V., & Fotiadis, D. I. (2015). Machine learning applications in cancer prognosis and prediction. Computational and structural biotechnology journal, 13, 8-17.
[26] Tavazoie, S., Hughes, J. D., Campbell, M. J., Cho, R. J., & Church, G. M. (1999). Systematic determination of genetic network architecture. Nature Genetics, 22(3), 281-285.
[27] LeCun, Y., Bengio, Y., & Hinton, G. (2015). Deep learning. Nature, 521(7553), 436-444.
[28] Esteva, A., Kuprel, B., Novoa, R. A., Ko, J., Swani, S. M., Blau, H. M., … & Thierer, T. (2017). Dermatologist-level classification of skin cancer with deep neural networks. Nature, 542(7639), 115-118.
[29] Libbrecht, M. W., & Noble, W. S. (2015). Machine learning applications in genetics and genomics. Nature Reviews Genetics, 16(6), 321-332.
[30] Grossman, R. L., Heath, A. P., Ferretti, V., Varmus, H. E., Antman, K. H., & Compton, C. C. (2016). Toward a shared vision for cancer data. New England Journal of Medicine, 375(12), 1117-1120.
[31] Paul, D., Sanap, G., Shenoy, S., Kalyane, D., Kalia, K., & Tekade, R. K. (2021). Artificial intelligence in drug discovery and development. Drug Discovery Today, 26(1), 80-93.
[32] Van Allen, E. M., Wagle, N., Stojanov, P., Perrin, D. L., Cibulskis, K., Marlow, S. E., … & Getz, G. (2014). Whole-exome sequencing and clinical interpretation of formalin-fixed paraffin-embedded tumor samples. Nature Medicine, 20(6), 682-688.
[33] Vayena, E., Blasimme, A., & Cohen, J. (2018). Machine learning in medicine: Addressing ethical challenges. PLOS Medicine, 15(10), e1002689.
[34] Rudin, C. (2019). Stop explaining black box machine learning models for high stakes decisions and use interpretable models instead. Nature Machine Intelligence, 1(5), 206-215.
[35] Price, W. N., Gerke, S., Luger, T., & Kahn Jr, J. P. (2019). What is precision medicine? Public Health Genomics, 22(1-2), 1-7.
AI fighting cancer, huh? So, are we talking Skynet helping oncologists now? I hope the algorithm considers lifestyle factors, or will it just tell everyone to “eat broccoli and exercise more”? Asking for a friend…who hates broccoli.