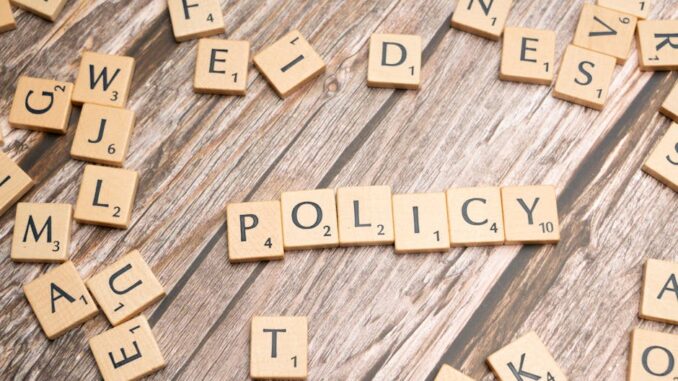
Abstract
Data governance has emerged as a critical discipline for organizations across diverse sectors, particularly in light of increasing data volumes, sophisticated cyber threats, and stringent regulatory requirements. While the protection of sensitive patient data in healthcare is a well-recognized concern, the broader implications of data governance extend far beyond this specific domain. This report provides a comprehensive analysis of the evolving landscape of data governance, exploring its core principles, technological advancements, and the challenges organizations face in establishing and maintaining effective governance frameworks. We delve into the regulatory landscape, including the General Data Protection Regulation (GDPR) and its global impact, as well as industry-specific standards and guidelines. The report also examines the crucial role of technology, focusing on data loss prevention (DLP) systems, anonymization techniques, and secure data sharing platforms. Furthermore, we address the ethical considerations surrounding data usage, particularly in the context of artificial intelligence (AI) and machine learning (ML) applications. The report concludes by identifying future trends and offering recommendations for organizations seeking to build robust and adaptable data governance strategies.
Many thanks to our sponsor Esdebe who helped us prepare this research report.
1. Introduction: The Data-Driven Imperative
The proliferation of data has fundamentally reshaped the modern world, driving innovation, enhancing decision-making, and creating new opportunities across various industries. However, this data revolution also presents significant challenges. Organizations must grapple with complex regulatory frameworks, increasing cybersecurity threats, and the ethical implications of data usage. Data governance, defined as the exercise of authority and control over the management of data assets (DAMA International, 2017), has become an essential discipline for navigating this complex landscape.
While healthcare has long been a focal point for data governance discussions due to the sensitive nature of patient information, the principles and practices of data governance are equally applicable to other sectors. Finance, for example, relies on robust data governance to ensure regulatory compliance, prevent fraud, and manage risk (Basel Committee on Banking Supervision, 2013). The manufacturing sector utilizes data governance to optimize supply chains, improve product quality, and enhance operational efficiency (Lee, 2001). Furthermore, government agencies rely on data governance to improve public services, enhance transparency, and inform policy decisions (OECD, 2017).
This report adopts a broad perspective on data governance, exploring its core principles, technological advancements, and the challenges organizations face in establishing and maintaining effective governance frameworks. It aims to provide valuable insights for professionals in various sectors who are responsible for data management, compliance, and security.
Many thanks to our sponsor Esdebe who helped us prepare this research report.
2. The Core Principles of Data Governance
Effective data governance frameworks are built upon a set of fundamental principles that guide the organization’s approach to data management and utilization. These principles include:
- Accountability: Clear lines of responsibility and ownership must be established for data assets. This involves designating data owners who are accountable for the quality, security, and usage of specific data sets. Data stewards, on the other hand, are responsible for implementing data policies and procedures within their respective domains.
- Transparency: Data governance policies and procedures should be transparent and accessible to all stakeholders. This ensures that individuals understand their roles and responsibilities in relation to data management. Transparency also promotes trust and encourages collaboration.
- Integrity: Data should be accurate, complete, and consistent. Organizations must implement data quality controls to prevent errors and ensure that data is reliable for decision-making.
- Compliance: Data governance frameworks must comply with relevant regulatory requirements and industry standards. This includes GDPR, HIPAA (Health Insurance Portability and Accountability Act), and other data protection laws. Compliance also extends to internal policies and procedures.
- Confidentiality: Sensitive data must be protected from unauthorized access and disclosure. Organizations must implement security measures such as access controls, encryption, and data masking to safeguard confidential information.
- Availability: Data should be readily available to authorized users when needed. Organizations must ensure that data is accessible through appropriate channels and that systems are in place to prevent data loss or downtime.
- Stewardship: Appointing data stewards within specific business units or functional areas is crucial for implementing and enforcing data governance policies. Data stewards act as liaisons between the data governance team and the business, ensuring that data is managed effectively and in accordance with established standards.
- Data Quality: Implementing robust data quality management processes is essential for ensuring the accuracy, completeness, and consistency of data. This includes data profiling, data cleansing, and data validation techniques.
Many thanks to our sponsor Esdebe who helped us prepare this research report.
3. The Regulatory Landscape: Navigating Data Protection Laws
The regulatory landscape surrounding data protection is constantly evolving, with new laws and regulations being introduced to address emerging challenges. Organizations must stay informed about these changes and ensure that their data governance frameworks are compliant. Some of the key regulations include:
- General Data Protection Regulation (GDPR): The GDPR, which came into effect in May 2018, is a comprehensive data protection law that applies to organizations operating within the European Union (EU) and the European Economic Area (EEA), as well as organizations that process the personal data of EU residents. The GDPR establishes strict requirements for data processing, including obtaining consent from individuals, providing transparency about data usage, and implementing security measures to protect personal data. Failure to comply with the GDPR can result in significant fines.
- California Consumer Privacy Act (CCPA): The CCPA, which came into effect in January 2020, is a California law that grants consumers various rights regarding their personal data, including the right to access, delete, and opt-out of the sale of their personal data. The CCPA applies to businesses that collect the personal data of California residents and meet certain revenue or data processing thresholds.
- Health Insurance Portability and Accountability Act (HIPAA): HIPAA is a US law that protects the privacy and security of protected health information (PHI). HIPAA establishes standards for the use and disclosure of PHI, as well as security requirements for electronic PHI.
- Industry-Specific Regulations: In addition to general data protection laws, many industries have their own specific regulations. For example, the financial services industry is subject to regulations such as the Gramm-Leach-Bliley Act (GLBA) and the Payment Card Industry Data Security Standard (PCI DSS), which require organizations to protect customer financial information. The healthcare industry is subject to HIPAA and other regulations that protect patient data.
The increasing complexity of the regulatory landscape necessitates a proactive and adaptable approach to data governance. Organizations must invest in legal and compliance expertise to ensure that their data governance frameworks are aligned with relevant regulations.
Many thanks to our sponsor Esdebe who helped us prepare this research report.
4. Technological Enablers of Data Governance
Technology plays a crucial role in enabling effective data governance. Various technological solutions can help organizations implement and enforce data governance policies and procedures. Some of the key technological enablers include:
- Data Loss Prevention (DLP) Systems: DLP systems are designed to prevent sensitive data from leaving the organization’s control. These systems monitor data traffic and identify instances where sensitive data is being transferred outside of the organization’s network or stored on unauthorized devices. DLP systems can block or quarantine the data transfer, alert administrators, or encrypt the data to protect it from unauthorized access.
- Data Masking and Anonymization Techniques: Data masking and anonymization techniques are used to protect sensitive data by replacing it with fictitious or generalized data. Data masking techniques include substitution, shuffling, and encryption. Anonymization techniques involve removing or modifying data elements in a way that makes it impossible to identify the individuals to whom the data relates. These techniques are particularly useful for protecting sensitive data during testing, development, and research.
- Secure Data Sharing Platforms: Secure data sharing platforms enable organizations to share data with external partners in a secure and controlled manner. These platforms typically include features such as access controls, encryption, and audit logging. They also allow organizations to track data usage and ensure that data is being used in accordance with established agreements.
- Data Catalogs and Metadata Management: Data catalogs provide a central repository for metadata, which is data about data. Metadata management tools help organizations manage and maintain metadata, ensuring that it is accurate, complete, and consistent. Data catalogs enable users to discover and understand data assets, facilitating data governance and promoting data reuse.
- Data Quality Tools: Data quality tools help organizations identify and correct data quality issues. These tools typically include features such as data profiling, data cleansing, and data validation. By improving data quality, organizations can ensure that data is reliable and accurate for decision-making.
- Data Governance Platforms: Integrated data governance platforms provide a comprehensive suite of tools and capabilities for managing data governance across the organization. These platforms typically include features such as data cataloging, metadata management, data quality management, data lineage tracking, and policy enforcement.
Many thanks to our sponsor Esdebe who helped us prepare this research report.
5. Ethical Considerations in Data Usage
The increasing use of data, particularly in the context of artificial intelligence (AI) and machine learning (ML), raises significant ethical concerns. Organizations must consider the ethical implications of data usage and ensure that data is used responsibly and ethically. Some of the key ethical considerations include:
- Bias and Discrimination: AI and ML algorithms can perpetuate and amplify existing biases in data, leading to discriminatory outcomes. Organizations must be aware of the potential for bias in their data and algorithms and take steps to mitigate it. This includes using diverse and representative data sets, auditing algorithms for bias, and implementing fairness metrics to assess the impact of algorithms on different groups.
- Privacy and Surveillance: The collection and use of personal data can raise privacy concerns, particularly when data is used for surveillance purposes. Organizations must be transparent about how they collect and use personal data and obtain consent from individuals when required. They must also implement security measures to protect personal data from unauthorized access and disclosure.
- Transparency and Explainability: AI and ML algorithms can be opaque, making it difficult to understand how they arrive at their decisions. This lack of transparency can undermine trust and make it difficult to hold algorithms accountable for their actions. Organizations should strive to make their AI and ML algorithms more transparent and explainable, providing explanations for their decisions and allowing users to understand how the algorithms work.
- Accountability and Responsibility: When AI and ML algorithms make decisions that have significant consequences, it is important to establish clear lines of accountability and responsibility. Organizations must determine who is responsible for the actions of AI and ML algorithms and ensure that there are mechanisms in place to address errors or unintended consequences.
- Informed Consent: In contexts like healthcare, obtaining informed consent for data usage is paramount. Patients should be fully informed about how their data will be used for research or AI applications, and they should have the right to withdraw their consent at any time. This adheres to ethical principles and builds trust between patients and healthcare providers.
Many thanks to our sponsor Esdebe who helped us prepare this research report.
6. Future Trends in Data Governance
The field of data governance is constantly evolving, with new trends and technologies emerging to address the challenges of the data-driven era. Some of the key future trends include:
- AI-Powered Data Governance: AI and ML are being increasingly used to automate and improve data governance processes. For example, AI can be used to identify data quality issues, classify data, and enforce data governance policies. AI-powered data governance can help organizations improve efficiency, reduce costs, and enhance the effectiveness of their data governance programs.
- Data Mesh Architecture: The data mesh is a decentralized approach to data governance that empowers domain teams to own and manage their own data. This approach allows organizations to scale data governance more effectively and respond more quickly to changing business needs. The data mesh promotes data ownership, accountability, and autonomy, while ensuring that data is governed in accordance with established standards.
- Data Observability: Data observability is the practice of monitoring and measuring the health of data systems. This includes tracking data quality, data lineage, and data usage. Data observability can help organizations identify and address data issues proactively, preventing data errors from impacting business operations.
- The Rise of Data Ethics Frameworks: Organizations are increasingly adopting formal data ethics frameworks to guide their data usage practices. These frameworks provide a structured approach to identifying and addressing ethical considerations related to data. Data ethics frameworks help organizations ensure that data is used responsibly and ethically, building trust with customers and stakeholders.
- Emphasis on Data Literacy: As data becomes more pervasive, there is a growing need for data literacy across organizations. Data literacy refers to the ability to understand, interpret, and communicate with data. Organizations are investing in data literacy training programs to empower employees to make data-driven decisions and contribute to data governance efforts.
Many thanks to our sponsor Esdebe who helped us prepare this research report.
7. Conclusion and Recommendations
Data governance is a critical discipline for organizations across all sectors. By implementing robust data governance frameworks, organizations can ensure that data is accurate, reliable, secure, and used ethically. This report has provided a comprehensive overview of the evolving landscape of data governance, exploring its core principles, technological advancements, and the challenges organizations face. To build effective data governance strategies, organizations should consider the following recommendations:
- Establish a Clear Data Governance Strategy: Develop a comprehensive data governance strategy that aligns with the organization’s business goals and objectives. This strategy should define the scope of data governance, identify key stakeholders, and establish clear roles and responsibilities.
- Invest in Data Governance Technology: Implement technological solutions that support data governance processes, such as DLP systems, data masking tools, secure data sharing platforms, and data catalogs.
- Foster a Data-Driven Culture: Promote data literacy across the organization and encourage employees to make data-driven decisions. Provide training and resources to help employees understand and interpret data.
- Prioritize Data Quality: Implement robust data quality management processes to ensure that data is accurate, complete, and consistent. Invest in data quality tools and techniques to identify and correct data quality issues.
- Embrace Ethical Data Practices: Adopt a formal data ethics framework to guide data usage practices. Ensure that data is used responsibly and ethically, protecting privacy and preventing bias and discrimination.
- Continuously Monitor and Adapt: The data landscape is constantly evolving, so it is important to continuously monitor and adapt data governance frameworks to address new challenges and opportunities. Regularly review and update data governance policies and procedures to ensure that they remain effective.
By embracing these recommendations, organizations can build robust and adaptable data governance strategies that enable them to harness the power of data while mitigating the risks. Ultimately, effective data governance is essential for driving innovation, enhancing decision-making, and building trust with stakeholders.
Many thanks to our sponsor Esdebe who helped us prepare this research report.
References
- Basel Committee on Banking Supervision. (2013). Principles for effective risk data aggregation and risk reporting. Bank for International Settlements.
- DAMA International. (2017). DAMA-DMBOK: Data Management Body of Knowledge. Technics Publications.
- Lee, G. K. (2001). Evaluating and implementing enterprise-wide data quality management. Journal of Management Information Systems, 18(3), 121-148.
- OECD. (2017). Data-driven innovation: Big data for growth and well-being. OECD Publishing.
- O’Reilly, T. (2007). What is Web 2.0: Design patterns and business models for the next generation of software. Communications & Strategies, 1(1), 17-37.
- Schmarzo, B. (2013). Big data: Understanding how data powers big business. John Wiley & Sons.
- Many prior examples can be found and these should be consulted to better understand expectations regarding what is considered sensitive patient data.
Data governance in manufacturing to optimize supply chains? Suddenly, I’m picturing a world where my online orders *never* get delayed. Is a perfectly on-time delivery utopia within our grasp, thanks to immaculate data? I can only dream!
That’s exactly the kind of future we’re aiming for! Imagine the efficiency gains across the board. Beyond just on-time deliveries, think about predictive maintenance reducing downtime and personalized product development based on real-time feedback. The possibilities are exciting!
Editor: MedTechNews.Uk
Thank you to our Sponsor Esdebe