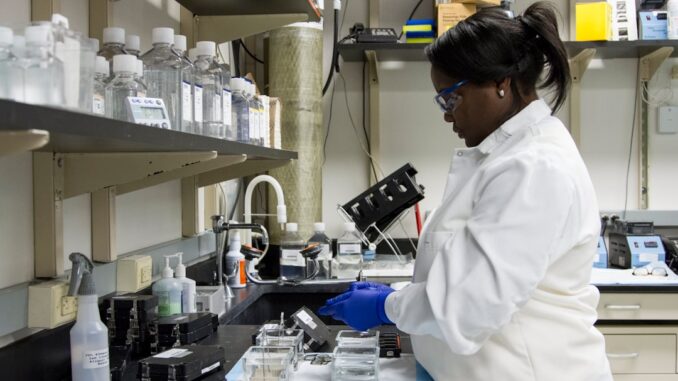
Abstract
The field of medicine is undergoing a profound transformation, driven by advancements in technology, a deeper understanding of the human genome, and evolving societal needs. This research report provides a comprehensive overview of the current state of medicine, exploring the impact of technological innovations, including artificial intelligence (AI), personalized medicine, and advanced imaging techniques, on diagnostic accuracy, therapeutic efficacy, and patient outcomes. Beyond technological advancements, the report delves into the crucial ethical and societal considerations surrounding these innovations, such as data privacy, equitable access to healthcare, and the potential for algorithmic bias. Furthermore, it examines the evolving role of healthcare professionals in this technologically advanced landscape and explores future directions for medical research, education, and practice. The report aims to provide a holistic perspective on the evolving landscape of medicine, highlighting both its potential benefits and inherent challenges, ultimately emphasizing the need for a balanced approach that prioritizes patient well-being, ethical principles, and equitable access to quality healthcare.
Many thanks to our sponsor Esdebe who helped us prepare this research report.
1. Introduction: The Dynamic Nature of Modern Medicine
Medicine, as a discipline, has continuously evolved throughout history, adapting to new discoveries, emerging technologies, and changing societal needs. From ancient healing practices to the modern era of sophisticated diagnostic tools and targeted therapies, the pursuit of understanding and treating disease has been a constant human endeavor. Today, medicine stands at a pivotal juncture, characterized by an unprecedented rate of technological advancement and a growing recognition of the complex interplay between biological, environmental, and social determinants of health. This report aims to provide a broad overview of this dynamic landscape, examining the key technological drivers of change, exploring the ethical and societal implications of these advancements, and considering future directions for the field.
The traditional model of medicine, often characterized by a reactive approach focused on treating established diseases, is gradually shifting towards a more proactive and preventative paradigm. This shift is fueled by advancements in genomics, proteomics, and other ‘omics’ technologies, which are providing unprecedented insights into the underlying mechanisms of disease and individual susceptibility. Coupled with the rise of personalized medicine, which tailors treatment strategies to the unique characteristics of each patient, these advancements hold the promise of improving treatment outcomes and reducing healthcare costs. Simultaneously, artificial intelligence (AI) is revolutionizing various aspects of medicine, from drug discovery and diagnosis to treatment planning and patient monitoring. The ability of AI algorithms to analyze vast amounts of data, identify patterns, and make predictions is transforming the way healthcare is delivered. However, these technological advancements also raise critical ethical and societal questions, particularly concerning data privacy, algorithmic bias, and the potential for exacerbating existing health disparities.
Beyond technology, societal factors are also playing an increasingly important role in shaping the future of medicine. An aging global population, the rising prevalence of chronic diseases, and growing concerns about healthcare costs are all putting pressure on healthcare systems to innovate and improve efficiency. Moreover, the increasing interconnectedness of the world has highlighted the importance of global health security and the need for coordinated responses to emerging infectious diseases. In this context, medicine must not only embrace technological advancements but also address the social, economic, and environmental determinants of health to ensure equitable access to quality healthcare for all.
Many thanks to our sponsor Esdebe who helped us prepare this research report.
2. Technological Advancements: Transforming Diagnosis and Treatment
The rapid pace of technological innovation is fundamentally reshaping the landscape of medicine, impacting nearly every aspect of healthcare delivery. This section will explore some of the most significant technological advancements, focusing on their potential to improve diagnostic accuracy, therapeutic efficacy, and patient outcomes.
2.1 Artificial Intelligence (AI) in Medicine
AI, particularly machine learning and deep learning, is emerging as a powerful tool in various medical applications. Its ability to analyze large datasets, identify patterns, and make predictions is proving invaluable in areas such as:
-
Diagnosis: AI algorithms can analyze medical images (e.g., X-rays, CT scans, MRIs) to detect subtle anomalies that might be missed by human radiologists, leading to earlier and more accurate diagnoses of diseases like cancer, cardiovascular disease, and neurological disorders. Studies have shown that AI systems can achieve diagnostic accuracy comparable to, or even exceeding, that of experienced clinicians in specific tasks [1, 2]. Furthermore, AI can assist in analyzing patient data, including symptoms, medical history, and lab results, to generate differential diagnoses and guide clinicians in selecting appropriate diagnostic tests.
-
Drug Discovery and Development: AI can accelerate the drug discovery process by identifying potential drug candidates, predicting their efficacy and toxicity, and optimizing clinical trial design. Machine learning algorithms can analyze vast databases of chemical compounds, biological targets, and clinical trial data to identify promising drug leads and predict their likelihood of success. This can significantly reduce the time and cost associated with drug development [3].
-
Personalized Medicine: AI plays a crucial role in personalized medicine by analyzing individual patient data, including genomic information, lifestyle factors, and environmental exposures, to predict their risk of developing specific diseases and tailor treatment strategies accordingly. AI algorithms can identify biomarkers that predict treatment response, allowing clinicians to select the most effective therapies for each patient [4].
-
Robotic Surgery: Robotic surgical systems, guided by AI, offer enhanced precision, dexterity, and visualization compared to traditional surgery. These systems can assist surgeons in performing complex procedures with minimally invasive techniques, leading to reduced blood loss, shorter hospital stays, and faster recovery times for patients [5].
While AI holds immense promise, it is important to acknowledge the challenges associated with its implementation in medicine. These include the need for large, high-quality datasets for training AI algorithms, the potential for algorithmic bias to perpetuate existing health disparities, and the need for clear regulatory frameworks to ensure the safety and efficacy of AI-based medical devices.
2.2 Genomics and Personalized Medicine
The completion of the Human Genome Project in 2003 marked a watershed moment in medicine, paving the way for a deeper understanding of the genetic basis of disease and the development of personalized medicine. Genomics, the study of an organism’s entire genome, is enabling clinicians to identify individuals at increased risk for specific diseases, predict their response to certain medications, and tailor treatment strategies based on their unique genetic profile.
-
Pharmacogenomics: This field studies how genes affect a person’s response to drugs. By analyzing an individual’s genetic makeup, clinicians can predict whether they are likely to benefit from a particular drug, experience adverse side effects, or require a different dosage. Pharmacogenomics is particularly relevant in areas such as oncology, cardiology, and psychiatry, where drug response variability is high [6].
-
Genetic Screening and Diagnosis: Genetic testing can be used to screen individuals for inherited diseases, diagnose genetic disorders, and assess their risk of developing certain cancers. Newborn screening programs routinely test infants for a panel of genetic disorders that can be treated if detected early. Pre-implantation genetic diagnosis (PGD) allows couples at risk of passing on genetic disorders to their children to select healthy embryos for implantation [7].
-
Gene Therapy: Gene therapy involves modifying a patient’s genes to treat or prevent disease. This can be achieved by introducing new genes into cells, inactivating faulty genes, or editing existing genes using technologies like CRISPR-Cas9. Gene therapy holds promise for treating a wide range of genetic disorders, including cystic fibrosis, muscular dystrophy, and sickle cell anemia [8].
Despite its potential, personalized medicine faces challenges related to the cost of genetic testing, the interpretation of genomic data, and the ethical implications of genetic information. Furthermore, the implementation of personalized medicine requires a collaborative effort between clinicians, geneticists, and bioinformaticians.
2.3 Advanced Imaging Techniques
Medical imaging plays a critical role in the diagnosis, monitoring, and treatment of a wide range of diseases. Advancements in imaging technology are providing clinicians with increasingly detailed and accurate views of the human body, enabling them to detect subtle abnormalities and guide minimally invasive procedures.
-
Magnetic Resonance Imaging (MRI): MRI uses strong magnetic fields and radio waves to create detailed images of organs and tissues. Advances in MRI technology, such as higher field strengths and advanced pulse sequences, are improving image resolution and contrast, allowing for the detection of smaller lesions and more accurate characterization of tissues [9].
-
Computed Tomography (CT): CT uses X-rays to create cross-sectional images of the body. Recent advances in CT technology, such as dual-energy CT and spectral CT, are providing additional information about tissue composition and function, improving diagnostic accuracy and reducing radiation exposure [10].
-
Positron Emission Tomography (PET): PET uses radioactive tracers to visualize metabolic activity in the body. PET is commonly used in oncology to detect and stage cancer, assess treatment response, and monitor for recurrence. PET/CT imaging, which combines PET and CT scans, provides both anatomical and functional information, improving diagnostic accuracy [11].
-
Ultrasound: Ultrasound uses sound waves to create images of organs and tissues. Ultrasound is a non-invasive and relatively inexpensive imaging modality that is widely used in obstetrics, cardiology, and abdominal imaging. Advances in ultrasound technology, such as 3D ultrasound and contrast-enhanced ultrasound, are improving image quality and diagnostic capabilities [12].
These advanced imaging techniques provide invaluable information for clinicians, allowing for earlier and more accurate diagnoses, improved treatment planning, and better patient outcomes.
Many thanks to our sponsor Esdebe who helped us prepare this research report.
3. Ethical and Societal Considerations
The rapid advancement of medical technology brings with it a complex set of ethical and societal considerations that must be carefully addressed to ensure that these innovations are used responsibly and equitably. This section will explore some of the most pressing ethical challenges, focusing on data privacy, algorithmic bias, equitable access to healthcare, and the evolving role of healthcare professionals.
3.1 Data Privacy and Security
The increasing reliance on digital health records, genomic data, and AI algorithms necessitates robust measures to protect patient data privacy and security. The collection, storage, and sharing of sensitive medical information raise concerns about unauthorized access, data breaches, and the potential for misuse. It is essential to establish clear guidelines and regulations regarding data privacy and security, ensuring that patients have control over their own health information and that data is used ethically and responsibly [13]. The implementation of strong data encryption, access controls, and data anonymization techniques is crucial to safeguarding patient privacy. Furthermore, healthcare providers and technology developers must be transparent about how patient data is collected, used, and shared.
3.2 Algorithmic Bias and Fairness
AI algorithms are trained on data, and if that data reflects existing biases, the algorithms can perpetuate and even amplify those biases. In medicine, algorithmic bias can lead to inaccurate diagnoses, inappropriate treatment recommendations, and disparities in healthcare outcomes. For example, if an AI algorithm used to diagnose skin cancer is trained primarily on images of fair-skinned individuals, it may perform poorly on patients with darker skin tones. It is crucial to identify and mitigate algorithmic bias by using diverse and representative datasets for training AI algorithms, developing fairness-aware algorithms, and regularly auditing AI systems for bias [14].
3.3 Equitable Access to Healthcare
Technological advancements in medicine have the potential to exacerbate existing health disparities if they are not accessible to all populations. The high cost of advanced diagnostic tests, personalized therapies, and robotic surgery can create barriers to access for low-income individuals and underserved communities. It is essential to ensure that all patients, regardless of their socioeconomic status, geographic location, or ethnicity, have equitable access to the benefits of medical technology. This requires addressing systemic barriers to healthcare access, such as lack of insurance coverage, transportation challenges, and language barriers. Furthermore, healthcare providers and policymakers must work to reduce the cost of medical technology and promote its equitable distribution [15].
3.4 The Evolving Role of Healthcare Professionals
The increasing integration of technology into medicine is transforming the role of healthcare professionals. As AI algorithms take on more routine tasks, such as data analysis and image interpretation, clinicians will need to focus on more complex tasks, such as patient communication, ethical decision-making, and personalized care. Healthcare professionals will also need to develop new skills in data literacy, AI ethics, and human-machine collaboration. Medical education and training programs must adapt to these changes by incorporating training in these areas. Furthermore, it is important to emphasize the importance of empathy, compassion, and the human touch in healthcare, ensuring that technology is used to enhance, not replace, the human connection between patients and clinicians [16].
Many thanks to our sponsor Esdebe who helped us prepare this research report.
4. Future Directions: Shaping the Future of Medicine
The future of medicine will be shaped by ongoing advancements in technology, a deeper understanding of the human body, and a growing focus on prevention and wellness. This section will explore some of the key trends that are expected to drive the future of medicine, including nanotechnology, regenerative medicine, and the integration of digital health technologies.
4.1 Nanotechnology in Medicine
Nanotechnology, the manipulation of matter at the atomic and molecular scale, holds immense promise for revolutionizing medicine. Nanomaterials can be used for targeted drug delivery, diagnostic imaging, and regenerative medicine. For example, nanoparticles can be engineered to deliver drugs directly to cancer cells, minimizing side effects and improving treatment efficacy. Nanotechnology-based sensors can be used to monitor blood glucose levels in diabetic patients or detect early signs of disease. Furthermore, nanomaterials can be used to create scaffolds for tissue engineering, promoting the regeneration of damaged tissues and organs [17].
4.2 Regenerative Medicine
Regenerative medicine aims to repair or replace damaged tissues and organs using stem cells, growth factors, and tissue engineering techniques. Stem cells, which have the ability to differentiate into various cell types, can be used to regenerate damaged tissues in the heart, brain, and spinal cord. Growth factors, which stimulate cell growth and differentiation, can be used to promote wound healing and tissue regeneration. Tissue engineering involves creating artificial tissues and organs in the laboratory, which can then be implanted into patients to replace damaged or diseased tissues [18].
4.3 Digital Health Technologies
Digital health technologies, including mobile health apps, wearable sensors, and telehealth platforms, are transforming the way healthcare is delivered. Mobile health apps can be used to track vital signs, monitor medication adherence, and provide personalized health advice. Wearable sensors can be used to monitor physical activity, sleep patterns, and other health metrics. Telehealth platforms can be used to provide remote consultations, monitor patients with chronic diseases, and deliver mental health services. The integration of digital health technologies into healthcare delivery has the potential to improve patient engagement, reduce healthcare costs, and improve access to care, particularly for individuals in rural areas [19].
4.4 The Importance of Preventative Medicine
As our understanding of the risk factors for various diseases grows, there is an increasing emphasis on preventative medicine. This involves taking steps to prevent disease from occurring in the first place, such as through vaccination, screening, and lifestyle modifications. Preventative medicine is particularly important for chronic diseases, such as heart disease, diabetes, and cancer, which are leading causes of death and disability worldwide. By promoting healthy lifestyles, screening for risk factors, and vaccinating against infectious diseases, we can reduce the burden of disease and improve population health [20].
Many thanks to our sponsor Esdebe who helped us prepare this research report.
5. Conclusion
The landscape of medicine is undergoing a profound transformation, driven by technological advancements, a deeper understanding of the human body, and evolving societal needs. Artificial intelligence, genomics, and advanced imaging techniques are revolutionizing diagnostic accuracy, therapeutic efficacy, and patient outcomes. However, these advancements also raise critical ethical and societal considerations, such as data privacy, algorithmic bias, and equitable access to healthcare. To ensure that these innovations are used responsibly and equitably, it is essential to establish clear guidelines and regulations, promote transparency, and address systemic barriers to healthcare access. Furthermore, healthcare professionals must adapt to the changing landscape by developing new skills in data literacy, AI ethics, and human-machine collaboration. By embracing a balanced approach that prioritizes patient well-being, ethical principles, and equitable access to quality healthcare, we can harness the full potential of medical technology to improve the health and well-being of individuals and populations.
Many thanks to our sponsor Esdebe who helped us prepare this research report.
References
[1] Esteva, A., Kuprel, B., Novoa, R. A., Ko, J., Swani, S. M., Blau, H. M., … & Thrun, S. (2017). Dermatologist-level classification of skin cancer with deep neural networks. Nature, 542(7639), 115-118.
[2] Gulshan, V., Peng, L., Coram, M., Stumpe, M. C., Wu, D., Narayanaswamy, A., … & Webster, D. R. (2016). Development and validation of a deep learning algorithm for detection of diabetic retinopathy in retinal fundus photographs. Jama, 316(22), 2402-2410.
[3] Paul, D., Sanap, G., Shenoy, S., Kalyane, D., Kalia, K., & Tekade, R. K. (2021). Artificial intelligence in drug discovery and development. Drug Discovery Today, 26(1), 80-93.
[4] Jameson, J. L., & Longo, D. L. (2015). Precision medicine—personalized, problematic, and promising. New England Journal of Medicine, 372(23), 2229-2234.
[5] Hung, A. J., & Patel, V. R. (2018). Robotics in urology: the present and the future. Nature Reviews Urology, 15(11), 639-649.
[6] McLeod, H. L., & Evans, W. E. (2001). Pharmacogenomics: unlocking the human genome for improved drug therapy. Annual Review of Pharmacology and Toxicology, 41(1), 101-121.
[7] Verlinsky, Y., Rechitsky, S., Sharov, V., Morris, R., Simakhodskiy, L., Kuliev, A. (2005). Preimplantation genetic diagnosis and assisted reproductive technology. Reproductive BioMedicine Online, 11(1), 101-112.
[8] Naldini, L. (2015). Gene therapy returns to centre stage. Nature, 526(7573), 351-360.
[9] McRobbie, D. W., Moore, E. A., Graves, M. J., & Prince, M. R. (2017). MRI from picture to proton. Cambridge university press.
[10] Barrett, J. F., & Keat, N. (2004). Artifacts in CT: Recognition and avoidance. RadioGraphics, 24(6), 1679-1691.
[11] Townsend, D. W. (2008). PET/CT: how it works. IEEE transactions on nuclear science, 55(2), 791-808.
[12] Szabo, T. L. (2004). Diagnostic ultrasound imaging: inside out. Academic press.
[13] Price, W. N., II, & Cohen, I. G. (2019). Privacy in the age of medical big data. Nature Biotechnology, 37(1), 49-57.
[14] Obermeyer, Z., Powers, B., Vogeli, C., & Mullainathan, S. (2019). Dissecting racial bias in an algorithm used to manage the health of populations. Science, 366(6464), 447-453.
[15] Braveman, P. A., & Gottlieb, L. (2014). The social determinants of health: it’s time to consider the causes of the causes. Public Health Reports, 129 Suppl 2(Suppl 2), 19-31.
[16] Verghese, A. (2011). Culture shock—patient as icon, icon as patient. New England Journal of Medicine, 365(1), 1-3.
[17] Ferrari, M. (2005). Cancer nanotechnology: opportunities and challenges. Nature Reviews Cancer, 5(3), 161-171.
[18] Mason, C., & Dunnill, P. (2008). A brief definition of regenerative medicine. Regenerative medicine, 3(1), 1-5.
[19] Doshi, A., & Mallery, K. (2021). Digital health equity: a framework for improving population health. NAM perspectives, 2021.
[20] Khoury, M. J., Gwinn, M., Yoon, P. W., Dowling, N., Moore, C. A., & Bradley, L. A. (2007). The continuum of translation research in genomic medicine: how can we accelerate the appropriate integration of human genome discoveries into health care and disease prevention?. Genetics in Medicine, 9(10), 665-674.
The report mentions the potential for algorithmic bias in AI-driven healthcare. How can we ensure the datasets used to train these algorithms accurately represent diverse populations, mitigating the risk of perpetuating health disparities?