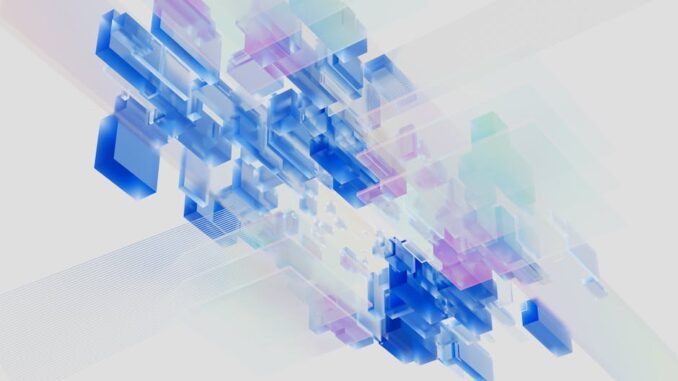
Abstract
Multiple sclerosis (MS) is a chronic, inflammatory, demyelinating, and neurodegenerative disease of the central nervous system (CNS). Its heterogeneous nature, both in clinical presentation and underlying pathophysiology, poses significant challenges for diagnosis, monitoring disease progression, and optimizing therapeutic strategies. This report provides a comprehensive overview of MS, encompassing its complex pathophysiology, current diagnostic criteria and limitations, the spectrum of available and emerging treatments, and the substantial economic burden associated with the disease. Furthermore, it explores the burgeoning role of artificial intelligence (AI) in revolutionizing MS management, from enhancing diagnostic accuracy and predicting disease trajectory to personalizing treatment approaches and accelerating drug discovery. The report highlights the potential of AI to address critical unmet needs in MS and ultimately improve patient outcomes.
Many thanks to our sponsor Esdebe who helped us prepare this research report.
1. Introduction
Multiple sclerosis (MS) is a debilitating autoimmune disease affecting an estimated 2.8 million people worldwide [1]. The disease is characterized by inflammatory demyelination and axonal damage within the brain and spinal cord, leading to a wide range of neurological symptoms, including motor dysfunction, sensory disturbances, cognitive impairment, and visual disturbances. The clinical course of MS is highly variable, ranging from relapsing-remitting MS (RRMS), characterized by distinct episodes of neurological dysfunction followed by periods of remission, to progressive forms of the disease, such as primary progressive MS (PPMS) and secondary progressive MS (SPMS), in which neurological disability accumulates steadily over time [2].
Despite significant advances in understanding the immunopathogenesis of MS and the development of disease-modifying therapies (DMTs), challenges remain in effectively managing the disease. Early and accurate diagnosis is crucial for initiating timely treatment and potentially slowing disease progression. However, the diagnostic process can be complex and time-consuming, often involving multiple clinical assessments, magnetic resonance imaging (MRI), and cerebrospinal fluid (CSF) analysis. Furthermore, monitoring disease activity and predicting long-term outcomes remain challenging, particularly in progressive forms of MS. The heterogeneity of MS and the limitations of current diagnostic and monitoring tools necessitate the development of novel approaches to improve patient care.
This report aims to provide a comprehensive overview of MS, addressing its multifaceted pathophysiology, current diagnostic and therapeutic landscapes, and the burgeoning role of artificial intelligence (AI) in transforming the management of this complex disease.
Many thanks to our sponsor Esdebe who helped us prepare this research report.
2. Pathophysiology of Multiple Sclerosis
The pathogenesis of MS is a complex interplay between genetic predisposition, environmental factors, and immune-mediated mechanisms. While the exact etiology remains elusive, significant progress has been made in elucidating the key immunological and neurological processes that contribute to disease development and progression.
2.1 Genetic Susceptibility
Genetic factors play a significant role in MS susceptibility, with studies demonstrating a higher risk of developing the disease among individuals with affected family members. The strongest genetic association is with the human leukocyte antigen (HLA) region on chromosome 6, particularly the HLA-DRB1*15:01 allele, which is consistently associated with increased risk of MS in various populations [3]. However, the HLA region accounts for only a small proportion of the overall genetic risk, and genome-wide association studies (GWAS) have identified numerous other non-HLA genetic variants that contribute to MS susceptibility. These variants are involved in various immune-related pathways, including T cell activation, B cell function, and cytokine signaling [4].
2.2 Environmental Triggers
Environmental factors are believed to play a critical role in triggering MS in genetically susceptible individuals. Several environmental factors have been implicated in MS pathogenesis, including Epstein-Barr virus (EBV) infection, vitamin D deficiency, smoking, and obesity [5]. EBV infection is strongly associated with MS risk, with nearly all MS patients being seropositive for EBV. Vitamin D deficiency has been consistently linked to increased MS risk and disease activity. Smoking is a well-established risk factor for MS, and obesity during adolescence or early adulthood has also been associated with increased MS risk.
2.3 Immune-Mediated Mechanisms
The hallmark of MS pathogenesis is an aberrant immune response that targets myelin, the insulating sheath surrounding nerve fibers in the CNS. The prevailing hypothesis is that autoreactive T cells, particularly CD4+ T helper cells and CD8+ cytotoxic T cells, infiltrate the CNS and initiate an inflammatory cascade that leads to myelin destruction and axonal damage [6]. These autoreactive T cells are activated in the periphery and migrate across the blood-brain barrier (BBB) into the CNS, where they recognize myelin antigens presented by antigen-presenting cells (APCs), such as dendritic cells and macrophages. Upon activation, T cells release pro-inflammatory cytokines, such as interferon-gamma (IFN-γ) and tumor necrosis factor-alpha (TNF-α), which contribute to inflammation and tissue damage.
B cells also play a critical role in MS pathogenesis. B cells can contribute to the disease process through several mechanisms, including the production of autoantibodies against myelin components, the presentation of myelin antigens to T cells, and the secretion of pro-inflammatory cytokines [7]. B cell depletion therapies, such as rituximab and ocrelizumab, have shown remarkable efficacy in RRMS, highlighting the importance of B cells in the disease.
2.4 Neurodegeneration
While inflammation and demyelination are prominent features of MS, neurodegeneration is increasingly recognized as a critical factor contributing to long-term disability. Axonal damage occurs early in the disease and progresses over time, leading to irreversible neurological deficits. Neurodegeneration can be driven by several mechanisms, including inflammation, oxidative stress, glutamate excitotoxicity, and mitochondrial dysfunction [8]. Furthermore, remyelination, the process of restoring myelin sheaths around damaged axons, is often incomplete in MS, leading to persistent axonal vulnerability.
Many thanks to our sponsor Esdebe who helped us prepare this research report.
3. Current Diagnostic Criteria and Challenges
The diagnosis of MS relies on clinical criteria, MRI findings, and, in some cases, CSF analysis. The McDonald criteria, revised in 2017, are the most widely used diagnostic criteria for MS [9]. These criteria require evidence of dissemination in space (DIS) and dissemination in time (DIT) within the CNS. DIS refers to the presence of lesions in multiple areas of the brain or spinal cord, while DIT refers to the occurrence of new lesions over time. MRI is the primary tool for assessing DIS and DIT, and gadolinium-enhancing lesions and T2-weighted lesions are used to fulfill these criteria.
3.1 Challenges in Diagnosis
Despite the established diagnostic criteria, several challenges remain in the accurate and timely diagnosis of MS. Early diagnosis can be challenging due to the non-specific nature of initial symptoms, which can mimic other neurological conditions. Furthermore, MRI findings can be subtle or absent in the early stages of the disease. The diagnostic process can be further complicated by the presence of clinically isolated syndrome (CIS), which refers to a single episode of neurological symptoms suggestive of MS but not fulfilling the diagnostic criteria. Patients with CIS have a variable risk of developing MS, and predicting who will progress to definite MS remains a challenge.
3.2 The Role of Biomarkers
There is a significant need for reliable biomarkers to aid in the diagnosis and prognosis of MS. Several potential biomarkers have been investigated, including neurofilament light chain (NfL), glial fibrillary acidic protein (GFAP), and myelin basic protein (MBP) [10]. NfL is a structural protein released from damaged neurons and is elevated in the CSF and serum of MS patients. Elevated NfL levels have been associated with increased disease activity and disability progression. GFAP is a structural protein found in astrocytes and is released upon astrocyte activation or damage. MBP is a component of myelin and is released upon myelin breakdown. While these biomarkers show promise, further research is needed to validate their clinical utility and establish their role in routine clinical practice.
Many thanks to our sponsor Esdebe who helped us prepare this research report.
4. Current and Emerging Treatment Modalities
The primary goal of MS treatment is to reduce disease activity, prevent disability accumulation, and improve quality of life. DMTs are the mainstay of MS treatment and aim to modify the underlying immune-mediated processes that drive disease progression.
4.1 Disease-Modifying Therapies
A wide range of DMTs are available for RRMS, including injectable therapies (e.g., interferon beta, glatiramer acetate), oral therapies (e.g., dimethyl fumarate, fingolimod, teriflunomide), and intravenous therapies (e.g., natalizumab, ocrelizumab, alemtuzumab). These therapies work through various mechanisms, including reducing lymphocyte proliferation, inhibiting lymphocyte trafficking into the CNS, and depleting B cells [11].
For progressive forms of MS, treatment options are more limited. Ocrelizumab is the only DMT approved for PPMS, and its efficacy is modest. For SPMS, siponimod is approved and demonstrates some benefit in slowing disability progression. The development of effective treatments for progressive MS remains a major unmet need.
4.2 Symptomatic Therapies
In addition to DMTs, symptomatic therapies are used to manage specific MS symptoms, such as fatigue, spasticity, pain, bladder dysfunction, and cognitive impairment. These therapies do not modify the underlying disease process but can significantly improve the quality of life for MS patients.
4.3 Emerging Therapies and Therapeutic Targets
Research is ongoing to develop new and more effective treatments for MS. Several promising therapies are in development, including Bruton’s tyrosine kinase (BTK) inhibitors, which target B cell and myeloid cell signaling; sphingosine-1-phosphate (S1P) receptor modulators, which modulate lymphocyte trafficking; and remyelination strategies, which aim to promote myelin repair [12]. Furthermore, research is focused on identifying novel therapeutic targets, such as inflammatory mediators, signaling pathways, and neuroprotective mechanisms.
4.4 Personalized Medicine Approaches
Given the heterogeneity of MS, there is a growing interest in personalized medicine approaches that tailor treatment strategies to individual patients based on their specific disease characteristics, genetic profile, and response to therapy. Biomarkers, imaging data, and clinical information can be integrated to predict treatment response and optimize therapeutic decisions [13]. AI and machine learning techniques are being used to develop predictive models that can identify patients who are most likely to benefit from specific treatments.
Many thanks to our sponsor Esdebe who helped us prepare this research report.
5. The Economic Burden of Multiple Sclerosis
MS imposes a substantial economic burden on patients, families, and society. The costs associated with MS include direct medical costs (e.g., medications, hospitalizations, physician visits), indirect costs (e.g., lost productivity, disability payments), and non-medical costs (e.g., home care, assistive devices) [14]. The economic burden of MS is particularly high due to the chronic nature of the disease, the high cost of DMTs, and the significant disability that can result from the disease. Early diagnosis and treatment can help to reduce the economic burden of MS by slowing disease progression and preventing disability accumulation.
Many thanks to our sponsor Esdebe who helped us prepare this research report.
6. The Role of Artificial Intelligence in Transforming MS Management
AI has the potential to revolutionize MS management in several key areas, including diagnosis, disease monitoring, treatment optimization, and drug discovery.
6.1 AI for Enhanced Diagnosis
AI algorithms can analyze complex MRI data to detect subtle lesions and patterns that may be missed by human radiologists. Machine learning models can be trained to differentiate MS lesions from other white matter abnormalities and to predict the risk of conversion from CIS to definite MS. Furthermore, AI can integrate clinical data, imaging data, and biomarker data to improve diagnostic accuracy and reduce diagnostic delay [15].
6.2 AI for Improved Disease Monitoring
AI can be used to develop predictive models that can forecast disease progression and treatment response. Machine learning algorithms can analyze longitudinal MRI data, clinical data, and patient-reported outcomes to identify factors that predict disability accumulation and treatment failure. These models can help clinicians to make more informed treatment decisions and to personalize treatment strategies [16]. Wearable sensors and mobile health technologies can be used to collect continuous data on patient activity, sleep, and symptoms. AI can analyze these data to detect changes in disease activity and to provide personalized feedback to patients.
6.3 AI for Personalized Treatment Approaches
AI can be used to identify patients who are most likely to benefit from specific treatments. Machine learning models can analyze patient data to predict treatment response and to optimize treatment selection. Furthermore, AI can be used to develop personalized treatment plans that take into account individual patient characteristics, preferences, and goals [17].
6.4 AI for Accelerated Drug Discovery
AI can accelerate the drug discovery process by identifying potential drug targets, predicting drug efficacy, and optimizing drug design. Machine learning algorithms can analyze large datasets of genomic, proteomic, and clinical data to identify novel therapeutic targets. Furthermore, AI can be used to screen large libraries of compounds to identify potential drug candidates and to predict their efficacy and toxicity [18].
Many thanks to our sponsor Esdebe who helped us prepare this research report.
7. Challenges and Future Directions
While AI holds great promise for transforming MS management, several challenges must be addressed to fully realize its potential. These challenges include the need for large, high-quality datasets, the development of robust and validated AI algorithms, and the integration of AI into clinical workflows. Furthermore, ethical considerations, such as data privacy and algorithmic bias, must be carefully addressed.
Future research should focus on developing AI-powered tools that can improve diagnostic accuracy, predict disease progression, personalize treatment strategies, and accelerate drug discovery. Furthermore, efforts should be made to integrate AI into routine clinical practice and to educate clinicians and patients about the benefits and limitations of AI.
Many thanks to our sponsor Esdebe who helped us prepare this research report.
8. Conclusion
Multiple sclerosis is a complex and heterogeneous disease that poses significant challenges for diagnosis, monitoring, and treatment. The pathophysiology of MS involves a complex interplay between genetic susceptibility, environmental triggers, and immune-mediated mechanisms. While significant advances have been made in understanding the immunopathogenesis of MS and the development of DMTs, challenges remain in effectively managing the disease. AI has the potential to revolutionize MS management by enhancing diagnostic accuracy, predicting disease trajectory, personalizing treatment approaches, and accelerating drug discovery. By addressing the challenges and pursuing future research directions, AI can play a critical role in improving outcomes for individuals living with MS.
Many thanks to our sponsor Esdebe who helped us prepare this research report.
References
[1] Walton, C., King, R., Rechtman, L., Kaye, W., Leray, E., Marrie, R. A., … & Banwell, B. (2020). Rising prevalence of multiple sclerosis worldwide: Insights from the Atlas of MS, third edition. Multiple Sclerosis Journal, 26(14), 1816-1821.
[2] Lublin, F. D., Reingold, S. C., Cohen, J. A., Cutter, G. R., Sørensen, P. S., Thompson, A. J., … & Miller, A. E. (2014). Defining the clinical course of multiple sclerosis: the 2013 revisions. Neurology, 83(3), 278-286.
[3] Sawcer, S., Hellenthal, G., Pirinen, M., Spencer, C. C., Patsopoulos, N. A., Moutsianas, L., … & Compston, A. (2011). Genetic risk and a primary role for cell-mediated immune mechanisms in multiple sclerosis. Nature, 476(7359), 214-219.
[4] Beecham, A. H., Patsopoulos, N. A., Xifara, D. K., Davis, M. F., Kemppinen, A., Cotsapas, C., … & De Jager, P. L. (2013). Analysis of immune-related loci identifies 48 new susceptibility variants for multiple sclerosis. Nature genetics, 45(11), 1353-1360.
[5] Ascherio, A., & Munger, K. L. (2007). Environmental risk factors for multiple sclerosis. Part I: the role of infection. Annals of Neurology, 61(4), 288-299.
[6] Frohman, E. M., Racke, M. K., & Raine, C. S. (2006). Multiple sclerosis—the plaque and its pathogenesis. New England Journal of Medicine, 354(9), 942-955.
[7] Hauser, S. L., & Cree, B. A. (2014). Treatment of multiple sclerosis with B-cell-depleting antibodies: a 20-year perspective. The Lancet Neurology, 13(3), 307-318.
[8] Dutta, R., & Trapp, B. D. (2011). Pathophysiology of multiple sclerosis. Neurologic Clinics, 29(2), 245-266.
[9] Thompson, A. J., Banwell, B. L., Barkhof, F., Carroll, W. M., Coetzee, T., Comi, G., … & Polman, C. H. (2018). Diagnosis of multiple sclerosis: 2017 revisions of the McDonald criteria. The Lancet Neurology, 17(2), 162-173.
[10] Disanto, G., Barro, C., Benkert, P., Naegelin, Y., Schädelin, S., Backes, D., … & Kuhle, J. (2017). Serum neurofilament light chain differentiates MS from mimics and predicts disease activity. Annals of Clinical and Translational Neurology, 4(12), 928-937.
[11] Goodin, D. S., Frohman, E. M., Hurwitz, B., Kinkel, R. P., Lincoff, N., Lublin, F. D., … & Wingerchuk, D. M. (2012). Disease modifying therapies for multiple sclerosis. Multiple Sclerosis Journal, 18(3), 275-286.
[12] Reich, D. S., Lucchinetti, C. F., Calabresi, P. A. (2018). Multiple Sclerosis. N Engl J Med, 378, 169-180.
[13] Baranzini, S. E. (2017). Personalized medicine in multiple sclerosis: dream or reality?. BMC medicine, 15(1), 1-5.
[14] Ghasemi, M., Razazian, N., Noorazar, S. G., & Movassaghi, S. (2017). Multiple sclerosis: a review. Advances in biomedical research, 6.
[15] Granberg, T., Rudie, K., D’Arcy, J., Stawiarz, L., Geijtenbeek, T.J., Wattjes, M.P., & Cortese, R. (2021). Artificial intelligence in multiple sclerosis magnetic resonance imaging: current status and future directions. Multiple Sclerosis Journal, 27(9), 1325–1335.
[16] Bellocchi, G., Conte, A., & Annovazzi, L. (2023). Artificial intelligence for advanced multiple sclerosis diagnostics: current status and future perspectives. Frontiers in Neuroscience, 17, 1086558.
[17] Haggiagi, A., Tsirka, V., Katsavos, A., & Kyritsis, A. P. (2023). Artificial intelligence-based predictive tools for personalized therapeutic choices in multiple sclerosis. Frontiers in Neurology, 14, 1122940.
[18] Dobson, R. J., & Munoz-Torrero, D. (2021). Machine learning for drug discovery. The Lancet, 397(10275), 775-777.
So, you’re saying AI might diagnose MS before I even realize my brain’s throwing a party without me? I’m slightly terrified, but also, can it tell me which wine pairs best with that diagnosis? Asking for a friend… who might be me.
That’s a great point! AI’s diagnostic capabilities are certainly advancing. While I can’t guarantee it’ll suggest a wine pairing (yet!), the potential for earlier diagnosis means potentially earlier intervention and personalized treatment strategies. Perhaps AI sommelier is not far away! Thanks for your comment!
Editor: MedTechNews.Uk
Thank you to our Sponsor Esdebe
The potential for AI to accelerate drug discovery, as highlighted in this report, could significantly impact the development of more effective treatments and potentially address the unmet needs in progressive MS. Further exploration in this area is warranted.