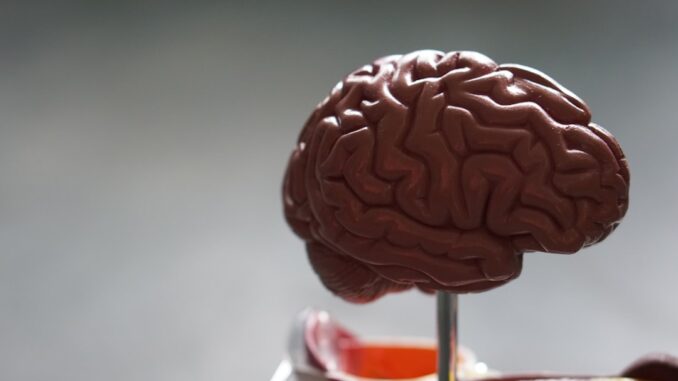
Abstract
Alzheimer’s disease (AD) poses a significant global health challenge, demanding earlier and more accurate diagnostic tools. While traditionally focused on brain imaging and cerebrospinal fluid analysis, recent research has increasingly highlighted the retina as a promising, non-invasive biomarker for AD and other neurodegenerative conditions. This research report comprehensively examines the anatomical and physiological parallels between the retina and the brain, explores retinal alterations observed in AD patients, and evaluates the diverse retinal imaging techniques employed for disease detection. We delve into the validity and reliability of using retinal imaging as a diagnostic tool, considering the strengths and limitations of various methodologies, including Optical Coherence Tomography (OCT), fundus photography, and electroretinography (ERG). Furthermore, we assess the role of Artificial Intelligence (AI) in enhancing retinal image analysis and prediction of AD risk. Beyond AD, this report explores the potential of retinal biomarkers in detecting and monitoring other neurodegenerative disorders such as Parkinson’s disease, Multiple Sclerosis and Frontotemporal Dementia. Finally, we address the current challenges in the field and discuss future research directions necessary to translate retinal imaging into a robust and clinically viable diagnostic platform for early neurodegenerative disease detection and management.
Many thanks to our sponsor Esdebe who helped us prepare this research report.
1. Introduction
Neurodegenerative diseases, characterized by the progressive loss of neuronal structure and function, represent a growing global health crisis. Alzheimer’s disease (AD), the most prevalent form of dementia, alone affects tens of millions worldwide, with the number expected to triple by 2050. Early and accurate diagnosis is crucial for effective intervention and potential disease-modifying therapies. However, traditional diagnostic methods, such as amyloid PET scans and cerebrospinal fluid (CSF) analysis, are invasive, expensive, and often only detect pathology at later stages of the disease. This has spurred research into more accessible and less invasive biomarkers for early AD detection.
The retina, an easily accessible extension of the central nervous system (CNS), has emerged as a particularly promising target. Sharing similar embryological origins, anatomical structures, and physiological pathways with the brain, the retina presents a unique opportunity to directly visualize neuronal changes associated with neurodegenerative processes. Retinal imaging techniques offer a non-invasive and cost-effective means of probing these changes, potentially providing a ‘window to the brain.’
This research report aims to provide a comprehensive overview of the current state of research on retinal biomarkers for neurodegenerative diseases, with a particular focus on AD. We will explore the anatomical and physiological basis for retinal involvement in neurodegeneration, review the specific retinal alterations observed in AD patients, evaluate the different retinal imaging techniques used, discuss the validity and reliability of retinal imaging as a diagnostic tool, and examine the potential of AI-driven analysis. We will also extend our analysis beyond AD to consider the application of retinal biomarkers to other neurodegenerative disorders.
Many thanks to our sponsor Esdebe who helped us prepare this research report.
2. The Retina as a Reflection of the Brain: Anatomical and Physiological Parallels
The retina and the brain share striking similarities in their development, structure, and function, making the retina a valuable model for studying neurodegenerative processes. Both structures originate from the neuroectoderm during embryonic development, giving rise to analogous cell types and signaling pathways.
Specifically, the retina’s layered structure closely mirrors that of the cerebral cortex. The retina contains various neuronal and glial cell types organized into distinct layers, including photoreceptors, bipolar cells, ganglion cells, amacrine cells, and horizontal cells. These cells are interconnected through complex synaptic circuits, analogous to the intricate neural networks in the brain. For instance, retinal ganglion cells (RGCs), the output neurons of the retina, project their axons through the optic nerve to the brain, forming direct connections with the visual cortex. The functionality of these cells can be assessed by way of ERG measurements.
Furthermore, both the retina and the brain are highly metabolically active tissues, making them vulnerable to oxidative stress, inflammation, and excitotoxicity, all of which are implicated in neurodegenerative diseases. Key proteins and pathways involved in AD pathogenesis, such as amyloid-beta (Aβ) and tau, have been found in the retina, suggesting that similar pathological mechanisms may operate in both tissues. The presence of these AD-related proteins in the retina, coupled with the ability to directly visualize retinal structures through non-invasive imaging, provides a compelling rationale for investigating retinal biomarkers for AD. It is worth noting that while the presence of amyloid in the retina is highly suggestive of AD, the expression and functionality of the blood retinal barrier can be altered with age, which means the presence of such biomarker needs to be interpreted carefully and in conjunction with other tests.
Many thanks to our sponsor Esdebe who helped us prepare this research report.
3. Retinal Alterations in Alzheimer’s Disease
Numerous studies have identified specific retinal changes associated with AD, providing evidence for the retina’s potential as a diagnostic biomarker. These changes encompass alterations in retinal thickness, vascular structure, electrical activity, and the presence of AD-related proteins.
3.1 Retinal Thickness Changes
Optical Coherence Tomography (OCT) studies have consistently demonstrated thinning of specific retinal layers in AD patients compared to age-matched controls. The retinal nerve fiber layer (RNFL), which comprises the axons of RGCs, is particularly susceptible to thinning in AD. A meta-analysis of multiple studies concluded that RNFL thinning is a consistent finding in AD and mild cognitive impairment (MCI), a prodromal stage of AD. Beyond the RNFL, thinning of the ganglion cell layer (GCL) and the inner plexiform layer (IPL) has also been reported. However, the magnitude of thinning varies across studies, potentially due to differences in patient populations, disease severity, OCT devices, and analysis methods. It is worth noting that RNFL thinning can also occur in other conditions such as glaucoma. It is therefore important to consider this when trying to diagnose AD from changes in retinal thickness.
3.2 Vascular Abnormalities
Retinal vasculature shares similar characteristics with cerebral microvasculature and is therefore susceptible to similar changes. Studies using fundus photography and OCT angiography (OCTA) have identified vascular abnormalities in AD patients, including decreased retinal vessel density, increased vessel tortuosity, and altered fractal dimension. These vascular changes may reflect impaired cerebral blood flow and vascular dysfunction associated with AD pathology. These changes may result in a reduction in oxygen and nutrient supply to neural tissue. Alterations to the blood retinal barrier is another potential factor. Some studies suggest that vascular changes may precede cognitive decline, making them potentially valuable for early detection.
3.3 Amyloid Deposition
While Aβ plaques are a hallmark of AD in the brain, evidence suggests that Aβ can also accumulate in the retina. Studies using curcumin-based fluorescence imaging have reported increased Aβ deposits in the retina of AD patients compared to controls. However, the sensitivity and specificity of this technique for detecting Aβ deposits in the retina are still under investigation. Furthermore, the relationship between retinal Aβ deposition and brain Aβ load remains unclear. Nevertheless, the presence of Aβ in the retina supports the concept of the retina as a ‘window to the brain’ and further strengthens the rationale for using retinal imaging as a diagnostic tool. Aβ plaque deposition and age-related macular degeneration (AMD) are both related and need to be considered when trying to diagnose AD from the presence of retinal amyloid.
3.4 Changes in Electrical Activity
Electroretinography (ERG) is a non-invasive technique that measures the electrical activity of the retina in response to light stimulation. Studies have shown that AD patients exhibit alterations in ERG waveforms, including reduced amplitude and prolonged implicit times of various ERG components, such as the a-wave and b-wave. These changes suggest impaired function of photoreceptors, bipolar cells, and other retinal neurons. While ERG is less commonly used than OCT in AD research, it provides complementary information about retinal function and may be particularly useful for detecting early changes in neuronal activity.
Many thanks to our sponsor Esdebe who helped us prepare this research report.
4. Retinal Imaging Techniques for Alzheimer’s Disease Detection
Several retinal imaging techniques are employed to assess retinal structure and function in AD patients. Each technique offers unique advantages and limitations in terms of resolution, invasiveness, and cost.
4.1 Optical Coherence Tomography (OCT)
OCT is a non-invasive imaging modality that uses light waves to capture high-resolution cross-sectional images of the retina. It allows for precise measurement of retinal layer thickness, making it particularly useful for detecting RNFL thinning and other structural changes associated with AD. OCT is widely available, relatively inexpensive, and easy to perform, making it a popular choice for AD research. However, OCT image quality can be affected by media opacities, such as cataracts, and the accuracy of layer segmentation algorithms can vary depending on the software used. There are two main types of OCT: spectral-domain OCT (SD-OCT) and swept-source OCT (SS-OCT). SS-OCT has a longer wavelength and can penetrate deeper into the tissue, providing better visualization of the choroid and deeper retinal layers. The type of OCT device used and the segmentation algorithm applied can significantly affect the measured retinal layer thicknesses and therefore influence the results of studies investigating retinal biomarkers for AD.
4.2 Fundus Photography
Fundus photography is a non-invasive technique that captures color images of the retina. It is useful for visualizing retinal blood vessels, optic disc, and macula. Fundus photography can be used to assess retinal vascular abnormalities, such as decreased vessel density and increased tortuosity, which may be associated with AD. However, fundus photography provides only a two-dimensional image of the retina and does not offer the same level of detail as OCT. In addition, assessment of retinal vascular changes based on fundus photographs is often subjective and requires experienced graders.
4.3 OCT Angiography (OCTA)
OCTA is a relatively new imaging technique that combines OCT with motion contrast imaging to visualize retinal and choroidal vasculature without the need for dye injection. OCTA allows for quantitative assessment of retinal vessel density, perfusion, and other vascular parameters. Studies have shown that OCTA can detect retinal vascular abnormalities in AD patients, such as decreased vessel density in the superficial and deep capillary plexuses. OCTA offers several advantages over traditional fluorescein angiography, including non-invasiveness, faster acquisition time, and higher resolution. However, OCTA image quality can be affected by motion artifacts and media opacities. It is therefore important to use appropriate image processing techniques to minimize artifacts and ensure accurate quantification of vascular parameters.
4.4 Electroretinography (ERG)
ERG is a non-invasive technique that measures the electrical activity of the retina in response to light stimulation. It can be used to assess the function of various retinal cell types, including photoreceptors, bipolar cells, and ganglion cells. ERG is particularly useful for detecting early changes in neuronal activity that may precede structural changes detectable by OCT. Studies have shown that AD patients exhibit alterations in ERG waveforms, suggesting impaired retinal function. However, ERG is less commonly used than OCT in AD research due to its complexity and variability. Standardized protocols and normative data are needed to improve the reliability and interpretability of ERG findings.
4.5 Adaptive Optics Imaging
Adaptive optics (AO) imaging is a high-resolution technique that corrects for optical aberrations in the eye, allowing for visualization of individual retinal cells and structures, such as photoreceptors and ganglion cells. AO imaging can potentially detect subtle changes in retinal cell morphology and function that may be associated with AD. However, AO imaging is technically challenging and requires specialized equipment. It is also time-consuming and not widely available. Nevertheless, AO imaging holds promise for providing a more detailed and sensitive assessment of retinal pathology in AD.
Many thanks to our sponsor Esdebe who helped us prepare this research report.
5. Validity and Reliability of Retinal Imaging as a Diagnostic Tool
While retinal imaging shows promise as a diagnostic tool for AD, several challenges remain regarding its validity and reliability. Validity refers to the accuracy of a test in measuring what it is intended to measure, while reliability refers to the consistency and reproducibility of the test results.
5.1 Diagnostic Accuracy
The diagnostic accuracy of retinal imaging for AD is typically assessed using sensitivity and specificity. Sensitivity refers to the ability of a test to correctly identify individuals with AD, while specificity refers to the ability of a test to correctly identify individuals without AD. Studies have reported variable sensitivity and specificity values for different retinal imaging parameters in distinguishing AD patients from controls. For example, some studies have reported high sensitivity and specificity for RNFL thickness in differentiating AD patients from healthy controls, while others have reported lower values. The diagnostic accuracy of retinal imaging may be affected by several factors, including the stage of disease, the choice of imaging technique, the analysis method, and the presence of other ocular conditions. It is important to note that many studies have relatively small sample sizes, which may limit the generalizability of the findings. In addition, most studies use cross-sectional designs, which cannot establish whether retinal changes precede or follow cognitive decline. Longitudinal studies are needed to determine the predictive value of retinal biomarkers for AD.
5.2 Reproducibility and Standardization
The reproducibility of retinal imaging measurements is crucial for clinical applications. Studies have investigated the intra- and inter-session reproducibility of OCT, OCTA, and ERG measurements. Generally, OCT measurements of retinal layer thickness have good reproducibility, while OCTA measurements of retinal vessel density have moderate to good reproducibility. ERG measurements can be more variable, especially if standardized protocols are not followed. Standardization of imaging protocols, data analysis methods, and reporting standards is essential to improve the reliability and comparability of retinal imaging results across different studies and clinical settings. International consortia, such as the International Alzheimer’s Disease Neuroimaging Initiative (IADNI), are working to establish standardized protocols for retinal imaging in AD research.
5.3 Confounding Factors
Several confounding factors can affect the interpretation of retinal imaging results in AD patients. These include age-related changes in the retina, other ocular diseases (e.g., glaucoma, age-related macular degeneration), systemic diseases (e.g., diabetes, hypertension), and medications. These confounding factors can affect retinal thickness, vascular structure, and electrical activity, potentially leading to false-positive or false-negative results. Careful consideration of these confounding factors is essential when interpreting retinal imaging results in AD patients. In clinical practice, it is important to obtain a thorough medical history and perform a comprehensive eye examination to rule out other potential causes of retinal abnormalities. Statistical methods can be used to adjust for the effects of confounding factors in research studies. In addition, there is genetic variation in retinal thickness that needs to be taken into account.
Many thanks to our sponsor Esdebe who helped us prepare this research report.
6. Artificial Intelligence (AI) for Enhanced Retinal Image Analysis
Artificial intelligence (AI), particularly machine learning (ML) and deep learning (DL), is rapidly transforming the field of medical image analysis. AI algorithms can be trained to automatically detect subtle patterns and features in retinal images that may be difficult or impossible for human observers to discern.
6.1 AI-Driven Retinal Image Analysis
AI algorithms have been developed to automatically segment retinal layers in OCT images, quantify retinal vessel density in OCTA images, and detect Aβ deposits in fundus photographs. These AI-driven tools can improve the speed, accuracy, and reproducibility of retinal image analysis. In addition, AI algorithms can be trained to predict the presence of AD based on retinal imaging data. Several studies have reported high accuracy for AI models in distinguishing AD patients from controls using retinal images. For example, a DL model trained on OCT images was able to distinguish AD patients from healthy controls with an accuracy of over 90%. However, the performance of AI models can vary depending on the quality of the training data, the architecture of the model, and the validation method used. In addition, AI models are often ‘black boxes,’ making it difficult to understand the reasoning behind their predictions.
6.2 Future Directions for AI in Retinal Imaging
Future research should focus on developing more robust and interpretable AI models for retinal image analysis. Explainable AI (XAI) techniques can be used to understand how AI models make their predictions, which can increase trust and acceptance of AI-driven diagnostic tools. In addition, AI models can be integrated with other clinical data, such as cognitive test scores and genetic information, to improve the prediction of AD risk. Federated learning, a decentralized approach to AI training, can be used to train AI models on data from multiple sites without sharing sensitive patient information. This can improve the generalizability and robustness of AI models. Finally, AI models can be used to personalize treatment decisions for AD patients based on their retinal imaging data. The application of AI to retinal imaging has enormous potential, however it is currently not possible to automatically derive high quality retinal image data and some human interaction is still required. The reliance on large high quality datasets makes this difficult and is a current limitation.
Many thanks to our sponsor Esdebe who helped us prepare this research report.
7. Retinal Biomarkers Beyond Alzheimer’s Disease
While the focus of this report has been on AD, retinal biomarkers are also being investigated for other neurodegenerative diseases, including Parkinson’s disease (PD), multiple sclerosis (MS), and frontotemporal dementia (FTD).
7.1 Parkinson’s Disease (PD)
Similar to AD, PD is characterized by the progressive loss of neurons, primarily in the substantia nigra. Studies have shown that PD patients exhibit retinal changes, including RNFL thinning, decreased retinal vessel density, and alterations in ERG waveforms. These retinal changes may reflect the neurodegenerative processes occurring in the brain in PD. A recent meta-analysis found that RNFL thinning was significantly associated with PD, suggesting that RNFL thickness may be a potential biomarker for PD diagnosis and monitoring. In addition, studies have shown that retinal dopamine levels are reduced in PD patients, which may contribute to the observed retinal changes. The use of retinal imaging in PD is less advanced than in AD.
7.2 Multiple Sclerosis (MS)
MS is a chronic autoimmune disease that affects the brain and spinal cord. Optic neuritis, an inflammation of the optic nerve, is a common manifestation of MS. OCT is widely used in MS to detect RNFL thinning, which is a marker of axonal damage in the optic nerve. Studies have shown that RNFL thickness is correlated with disease severity and progression in MS. In addition, OCT can be used to detect macular volume loss and other retinal changes in MS patients. The use of OCT is often used in conjuction with VEP (Visual Evoked Potentials).
7.3 Frontotemporal Dementia (FTD)
FTD is a group of neurodegenerative disorders that primarily affect the frontal and temporal lobes of the brain. Limited research has investigated retinal biomarkers in FTD. However, some studies have reported RNFL thinning and other retinal changes in FTD patients. Future research is needed to determine the potential of retinal imaging for diagnosing and monitoring FTD. FTD is a far less researched area when compared to AD.
Many thanks to our sponsor Esdebe who helped us prepare this research report.
8. Challenges and Future Directions
Despite the promising findings discussed in this report, several challenges remain in translating retinal imaging into a robust and clinically viable diagnostic platform for early neurodegenerative disease detection and management.
8.1 Longitudinal Studies
Most studies on retinal biomarkers in neurodegenerative diseases are cross-sectional, which limits our understanding of the temporal relationship between retinal changes and disease progression. Longitudinal studies are needed to determine whether retinal changes precede or follow cognitive decline and to assess the predictive value of retinal biomarkers for disease onset and progression. Such studies should involve repeated retinal imaging and cognitive assessments over several years.
8.2 Multi-Center Studies
Many studies on retinal biomarkers in neurodegenerative diseases are conducted at single centers, which may limit the generalizability of the findings. Multi-center studies are needed to validate the findings across different populations and clinical settings. Such studies should use standardized imaging protocols and data analysis methods to ensure comparability of the results. In addition, multi-center studies can increase the sample size and statistical power, allowing for more robust conclusions.
8.3 Integration with Other Biomarkers
Retinal imaging should not be viewed as a standalone diagnostic tool but rather as part of a multimodal approach that integrates information from other biomarkers, such as brain imaging, CSF analysis, and blood-based biomarkers. Combining retinal imaging data with other biomarker data can improve the diagnostic accuracy and predictive value for neurodegenerative diseases. Integrative models can be developed using AI techniques to combine data from multiple sources and predict individual disease risk and prognosis. Combining several markers would offer a more robust result.
8.4 Technological Advancements
Continued technological advancements in retinal imaging are needed to improve the resolution, sensitivity, and specificity of retinal imaging techniques. For example, new AO-OCT technologies can provide even higher resolution images of retinal cells and structures. Development of new contrast agents and imaging modalities can enhance the detection of Aβ deposits and other pathological features in the retina. In addition, advanced image processing and analysis techniques can improve the quantification of retinal changes.
8.5 Clinical Trials
Clinical trials are needed to evaluate the efficacy of interventions targeting retinal changes in neurodegenerative diseases. For example, studies can investigate whether lifestyle modifications, such as exercise and diet, can improve retinal health and slow the progression of retinal changes in AD patients. Clinical trials can also evaluate the efficacy of pharmacological interventions targeting specific pathways involved in retinal neurodegeneration. Retinal imaging can be used as an outcome measure in these clinical trials to assess the effectiveness of the interventions.
Many thanks to our sponsor Esdebe who helped us prepare this research report.
9. Conclusion
The retina holds significant promise as a non-invasive and accessible window to the brain, offering a unique opportunity for early detection and monitoring of neurodegenerative diseases such as Alzheimer’s disease. Retinal imaging techniques, including OCT, fundus photography, and ERG, have revealed a range of retinal alterations associated with AD, including thinning of specific retinal layers, vascular abnormalities, amyloid deposition, and changes in electrical activity. The application of AI to retinal image analysis has further enhanced the potential for accurate and efficient diagnosis. However, challenges remain regarding the validity, reliability, and standardization of retinal imaging as a diagnostic tool. Future research should focus on longitudinal studies, multi-center collaborations, integration with other biomarkers, technological advancements, and clinical trials to translate retinal imaging into a robust and clinically viable diagnostic platform for early neurodegenerative disease detection and management. Ultimately, leveraging the potential of retinal biomarkers may pave the way for earlier intervention and improved outcomes for individuals at risk of or affected by devastating neurodegenerative conditions.
Many thanks to our sponsor Esdebe who helped us prepare this research report.
References
- Alzheimer’s Association. (2023). 2023 Alzheimer’s Disease Facts and Figures. Alzheimer’s & Dementia, 19(4), 1598-1695.
- Blanks, J. C., Torigoe, Y., Hinton, D. R., Blanks, R. H., & Sadun, A. A. (1996). Retinal pathology in Alzheimer’s disease. II. Regional neuron loss and glial proliferation in the inner retina. Neurobiology of Aging, 17(3), 385-395.
- La Morgia, C., Barboni, P., Rizzo, G., Pinna, A., Caporali, L., Monte, G., … & Carelli, V. (2016). Retinal nerve fiber layer thickness and CSF biomarkers in Alzheimer’s disease. PloS one, 11(2), e0149655.
- Cheung, C. Y., Ong, S. J., Ikram, M. K., Gupta, P., & Wong, T. Y. (2010). Retinal microvascular network: a window to systemic disease. Asia-Pacific Journal of Ophthalmology, 9(5), 329.
- Koronyo, Y., Salumbides, B. C., Black, K. L., Koronyo-Hamaoui, M. (2012). Amyloid plaques in human retina: association with age and Alzheimer’s disease. Brain, 135(Pt 3), 798–809.
- Polo, V., Garcia-Martin, E., Larrosa, J. M., Garcıa-de-la-Riva, I., Romero-Miguel, R., Lopez de Eguileta, A., … & Satue, M. (2015). Exploring retinal layer thinning in Alzheimer’s disease: A meta-analysis. Current Alzheimer Research, 12(7), 658-666.
- van der Flier, W. M., Pijnenburg, Y. A., Prins, N., Lemstra, A. W., van Duijn, C. M., & Scheltens, P. (2011). Optimizing cut-off values for cerebrospinal fluid biomarkers in the diagnosis of Alzheimer’s disease. Alzheimer’s & Dementia, 7(1), 92-100.
- O’Bryhim, B. E., Apte, R. S., Kung, N., Coble, D., Van Stavern, G. P., & Mishra, P. S. (2018). Association of thinner retinal nerve fiber layer with cognitive decline and Alzheimer disease. JAMA ophthalmology, 136(9), 987-994.
- Bulut, M., Kurt, A., Gozkaya, O., Erol, M. K., Cakar, O. Z., & Akidan, M. A. (2016). Evaluation of retinal nerve fiber layer and macular thickness in patients with Alzheimer disease using spectral-domain optical coherence tomography. The Turkish Journal of Ophthalmology, 46(3), 103.
- Chrysou, A., Stathopoulos, P., & Asproudis, I. (2023). Optical Coherence Tomography Angiography as a Potential Diagnostic Tool for Neurodegenerative Diseases. Diagnostics, 13(11), 1867.
- Mirzaei, Z., Derakhshan, A., Asadi-Aliabadi, M., Mowla, A., Farahi, N., & Khadamy, J. (2023). Diagnostic accuracy of optical coherence tomography angiography (OCTA) findings in Alzheimer’s disease: A systematic review and meta-analysis. BMC neurology, 23(1), 145.
- Parisi, V., Restuccia, R., Fattapposta, F., Mina, C., Gismondo, R., Coluzzi, P., … & Pierelli, F. (2001). Morphological and functional retinal impairment in Alzheimer’s disease patients. Clinical neurophysiology, 112(10), 1860-1867.
- Ascaso, F. J., Huerva, V., Garcés, M. J., Cristóbal, J. A., & Alió, J. L. (2006). Electroretinographic changes in patients with Alzheimer’s disease. Ophthalmic Research, 38(4), 267-272.
- Risacher, S. L., Kim, N., Nho, K., Foroud, T., Shen, L., Petersen, R. C., … & Saykin, A. J. (2010). APOE effect on Alzheimer’s disease biomarkers in older adults with no cognitive impairment. Archives of neurology, 67(6), 697-703.
- Liu, R., Zhou, X., Zhang, X., Chen, X., Jiang, Y., & Zhang, S. (2022). Deep learning for Alzheimer’s disease diagnosis based on retinal optical coherence tomography images: a review. Frontiers in Aging Neuroscience, 14, 1045231.
- Gupta, P., Cheung, C. Y., Tan, B., Sadda, S. R., & Wong, T. Y. (2011). Retinal optical coherence tomography angiography: a review of technical principles and clinical applications. American Journal of Ophthalmology, 152(5), 667-678.
- Kromer, R., Serbecic, N., Hausner, L., Froelich, L., Aboulenein-Djamshidian, F., Lanthaler, K., … & Wirths, O. (2014). Detection of Amyloid-β peptides in human retinal biopsies of Alzheimer’s disease patients. PloS one, 9(4), e95501.
- Baykara, M., Ozcan, M. O., Gencer, M., Bayraktar, Z., & Yilmaz, U. (2016). Evaluation of retinal nerve fiber layer thickness in Parkinson’s disease using spectral-domain optical coherence tomography. Eye and vision, 3, 13.
- Satue, M., Garcia-Martin, E., Almarcegui, C., Otin, S., Larrosa, J. M., Sierra, M., … & Polo, V. (2016). Analysis of the macular and peripapillary retinal nerve fiber layer thickness in multiple sclerosis using optical coherence tomography. Multiple sclerosis journal, 22(2), 185-194.
- Ferrari, C. D., Hübers, A., Machts, J., Bittner, D. M., Riedel-Heller, S. G., & Hegerl, U. (2017). Retinal nerve fiber layer and macular volume in frontotemporal dementia. Journal of Alzheimer’s disease, 57(1), 241-251.
So, you’re saying my eyes might reveal my future cognitive decline before my brain does? Are we sure this isn’t just another excuse to sell more eye exams with an AI twist? Because, honestly, my eyeballs have enough problems already.
That’s a great point! It’s vital to ensure this research isn’t just about selling more exams. We’re aiming for a non-invasive, early detection method, but further validation is crucial. Combining retinal scans with other biomarkers could be a more reliable way to predict cognitive decline. Thanks for raising this important question!
Editor: MedTechNews.Uk
Thank you to our Sponsor Esdebe
So, you’re suggesting my next eye exam could double as a crystal ball for my brain? Maybe I should start practicing my “surprised” face now, just in case my retinas reveal I’m destined to forget where I put my car keys…again. What about using AI to correct the underlying issues?