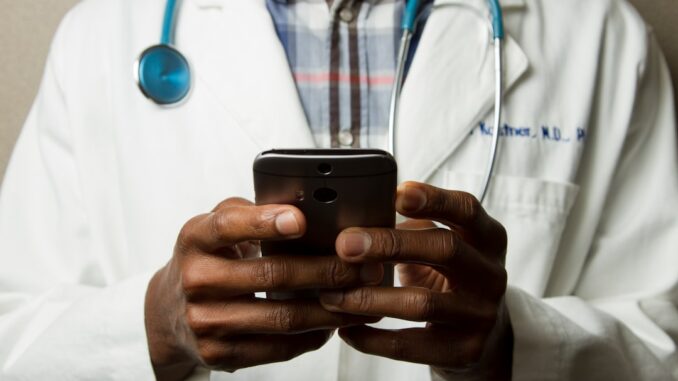
Abstract
Wearable technology has emerged as a transformative force in healthcare, offering continuous and personalized monitoring of physiological parameters. This report provides a comprehensive overview of wearable technology in healthcare, encompassing diverse applications, underlying technologies, and associated challenges. We examine the landscape of wearable sensors, data processing techniques, and clinical applications across various medical domains, with a particular emphasis on cardiovascular health management. Furthermore, we delve into the critical aspects of data privacy, security, accuracy, and usability, exploring the ethical considerations and regulatory frameworks surrounding the deployment of these technologies. Finally, we discuss future directions and potential advancements in wearable technology, highlighting the opportunities for improved patient outcomes, reduced healthcare costs, and enhanced preventive care strategies.
Many thanks to our sponsor Esdebe who helped us prepare this research report.
1. Introduction
The advent of wearable technology has revolutionized various aspects of our lives, from fitness tracking and entertainment to communication and healthcare. Wearable devices, such as smartwatches, fitness trackers, and smart clothing, are equipped with sensors capable of continuously monitoring physiological parameters, including heart rate, blood pressure, activity levels, sleep patterns, and even biochemical markers [1]. This continuous monitoring capability offers unprecedented opportunities for personalized healthcare, enabling early detection of health risks, proactive intervention, and improved disease management.
In the healthcare sector, wearable technology holds immense promise for transforming traditional approaches to diagnosis, treatment, and prevention. The ability to collect real-time physiological data empowers patients to actively participate in their own healthcare, fostering self-management and adherence to treatment plans. Furthermore, the vast amounts of data generated by wearable devices can be leveraged to develop predictive models and personalized interventions, leading to more effective and efficient healthcare delivery. However, the integration of wearable technology into healthcare also presents several challenges, including data privacy concerns, accuracy limitations, usability issues, and the need for robust regulatory frameworks.
This report aims to provide a comprehensive overview of wearable technology in healthcare, exploring its potential benefits, associated challenges, and future directions. We will examine the diverse types of wearable sensors, data processing techniques, and clinical applications across various medical domains, with a particular emphasis on cardiovascular health management. Furthermore, we will delve into the critical aspects of data privacy, security, accuracy, and usability, exploring the ethical considerations and regulatory frameworks surrounding the deployment of these technologies.
Many thanks to our sponsor Esdebe who helped us prepare this research report.
2. Types of Wearable Sensors and Technologies
Wearable devices utilize a wide range of sensors to capture physiological data. These sensors can be broadly categorized into several types, each with its own strengths and limitations:
-
Motion Sensors: Accelerometers, gyroscopes, and magnetometers are commonly used to track movement patterns, activity levels, and posture. These sensors are fundamental for activity tracking, fall detection, and gait analysis [2]. The accuracy of these sensors is generally high, but their interpretation can be complex, requiring sophisticated algorithms to differentiate between different activities.
-
Optical Sensors: Photoplethysmography (PPG) sensors use light to measure blood flow and heart rate. PPG sensors are widely used in smartwatches and fitness trackers due to their low cost and ease of integration [3]. However, their accuracy can be affected by factors such as skin tone, motion artifacts, and ambient light.
-
Electrophysiological Sensors: Electrocardiography (ECG) sensors measure the electrical activity of the heart, providing detailed information about heart rate, rhythm, and morphology. Electroencephalography (EEG) sensors measure brain activity, enabling applications such as sleep monitoring and seizure detection. Electromyography (EMG) sensors measure muscle activity, which can be used for rehabilitation and prosthetic control [4]. These sensors generally require skin contact and can be more susceptible to noise and interference.
-
Environmental Sensors: Temperature sensors measure body temperature, while humidity sensors measure ambient humidity. These sensors can be used to monitor environmental conditions and detect potential health risks such as heatstroke or hypothermia. Air quality sensors can also be incorporated to monitor exposure to pollutants.
-
Biochemical Sensors: These sensors measure various biochemical markers in bodily fluids such as sweat, saliva, or interstitial fluid. Examples include glucose sensors for diabetes management, lactate sensors for athletic performance monitoring, and cortisol sensors for stress assessment [5]. The development of reliable and non-invasive biochemical sensors is a rapidly evolving area of research.
Beyond the sensors themselves, the data processing capabilities of wearable devices are crucial for extracting meaningful information. This involves signal processing, noise reduction, feature extraction, and machine learning algorithms. Edge computing, where data processing occurs directly on the wearable device, is becoming increasingly important for reducing latency and improving data privacy. The choice of sensor and data processing technique depends on the specific application and the desired level of accuracy and sensitivity.
Many thanks to our sponsor Esdebe who helped us prepare this research report.
3. Clinical Applications of Wearable Technology
Wearable technology has found applications in a wide range of clinical domains, including:
-
Cardiovascular Health Management: Wearable devices are used to monitor heart rate, blood pressure, and ECG, enabling early detection of arrhythmias, hypertension, and other cardiovascular conditions. Remote monitoring of patients with heart failure is becoming increasingly common [6].
-
Diabetes Management: Continuous glucose monitors (CGMs) provide real-time glucose readings, allowing patients to adjust their insulin dosage and lifestyle choices. Wearable insulin pumps can deliver insulin automatically based on CGM readings [7].
-
Neurological Disorders: EEG sensors are used to monitor brain activity in patients with epilepsy, sleep disorders, and other neurological conditions. Wearable devices can also be used to track movement patterns and tremors in patients with Parkinson’s disease [8].
-
Respiratory Health Management: Wearable sensors can monitor respiratory rate, oxygen saturation, and lung function in patients with asthma, COPD, and other respiratory conditions. Remote monitoring can help to prevent exacerbations and hospitalizations [9].
-
Mental Health Monitoring: Wearable devices can track heart rate variability, sleep patterns, and activity levels, which can be used to assess stress levels, mood, and cognitive function. These data can be used to personalize mental health interventions [10].
-
Rehabilitation and Physical Therapy: Motion sensors can track movement patterns and range of motion, providing valuable feedback for patients undergoing rehabilitation after injury or surgery. Wearable devices can also be used to motivate patients to adhere to their exercise programs [11].
The effectiveness of wearable technology in these clinical applications depends on several factors, including the accuracy of the sensors, the usability of the device, and the integration with existing healthcare systems. Clinical trials are needed to validate the efficacy of wearable devices and to determine their impact on patient outcomes and healthcare costs.
Many thanks to our sponsor Esdebe who helped us prepare this research report.
4. Challenges and Opportunities of Integrating AI into Wearable Devices
The integration of artificial intelligence (AI) into wearable devices presents both significant opportunities and challenges. AI algorithms can enhance the capabilities of wearable devices by enabling more sophisticated data analysis, personalized insights, and predictive modeling.
Opportunities:
-
Improved Accuracy and Reliability: AI algorithms can be used to filter noise, correct for artifacts, and improve the accuracy of sensor data. Machine learning models can be trained to recognize patterns and anomalies that may be missed by traditional methods [12].
-
Personalized Insights and Recommendations: AI can personalize insights and recommendations based on individual physiological data, lifestyle factors, and medical history. This can lead to more effective interventions and improved patient engagement [13].
-
Predictive Modeling: AI can be used to develop predictive models that forecast future health risks and outcomes. This can enable proactive intervention and prevent adverse events [14]. For example, AI could predict an impending heart failure exacerbation based on subtle changes in heart rate variability, activity level, and sleep patterns.
-
Automated Monitoring and Alerting: AI can automate the monitoring of physiological data and trigger alerts when critical thresholds are breached. This can free up healthcare professionals to focus on more complex tasks and improve the efficiency of healthcare delivery [15].
Challenges:
-
Data Privacy and Security: Wearable devices collect sensitive personal data, raising concerns about data privacy and security. AI algorithms require access to large datasets, which can further exacerbate these concerns. Robust security measures and data anonymization techniques are needed to protect patient privacy [16].
-
Algorithmic Bias: AI algorithms can be biased if they are trained on datasets that do not accurately represent the target population. This can lead to inaccurate predictions and inequitable outcomes. Careful attention must be paid to data collection and algorithm design to mitigate bias [17].
-
Explainability and Transparency: AI algorithms can be complex and difficult to understand, making it challenging to explain their predictions and decisions. This lack of transparency can erode trust and hinder adoption. Explainable AI (XAI) techniques are needed to make AI algorithms more transparent and understandable [18].
-
Regulatory Approval: The use of AI in medical devices is subject to regulatory scrutiny. AI algorithms must be rigorously validated and tested to ensure their safety and effectiveness. Regulatory pathways for AI-enabled medical devices are still evolving [19].
-
Computational Resources: Training and deploying AI algorithms can require significant computational resources. Edge computing can help to reduce the computational burden on wearable devices, but it also presents challenges related to data security and model updating [20].
Overcoming these challenges is crucial for realizing the full potential of AI in wearable technology. This requires a multidisciplinary approach involving data scientists, engineers, clinicians, ethicists, and regulators.
Many thanks to our sponsor Esdebe who helped us prepare this research report.
5. Data Privacy, Security, and Ethical Considerations
The widespread adoption of wearable technology in healthcare raises significant concerns about data privacy, security, and ethical considerations. Wearable devices collect sensitive personal data, including physiological parameters, activity levels, and location information. This data is vulnerable to unauthorized access, misuse, and disclosure.
Data Privacy:
-
Data Ownership: It is important to clarify who owns the data collected by wearable devices. Is it the patient, the device manufacturer, the healthcare provider, or a third-party data aggregator? Clear data ownership policies are needed to protect patient rights [21].
-
Data Anonymization: Anonymization techniques can be used to protect patient privacy by removing identifying information from the data. However, anonymization is not always foolproof, and re-identification attacks are possible. Robust anonymization methods are needed to minimize the risk of re-identification [22].
-
Data Sharing: Patients should have control over how their data is shared with third parties. They should be informed about the purposes for which their data will be used and given the opportunity to opt out of data sharing [23].
Data Security:
-
Encryption: Data should be encrypted both in transit and at rest to protect it from unauthorized access. Strong encryption algorithms should be used to ensure data security [24].
-
Access Control: Access to data should be restricted to authorized personnel only. Role-based access control can be used to limit access based on job function [25].
-
Vulnerability Management: Wearable devices and their associated software should be regularly scanned for vulnerabilities and patched promptly. Security updates should be installed automatically to protect against known threats [26].
Ethical Considerations:
-
Informed Consent: Patients should be fully informed about the risks and benefits of using wearable devices before agreeing to participate in data collection. Informed consent should be obtained in a clear and understandable manner [27].
-
Data Bias: AI algorithms can be biased if they are trained on datasets that do not accurately represent the target population. This can lead to inequitable outcomes. Careful attention must be paid to data collection and algorithm design to mitigate bias [28].
-
Algorithmic Transparency: AI algorithms can be complex and difficult to understand, making it challenging to explain their predictions and decisions. This lack of transparency can erode trust and hinder adoption. Explainable AI (XAI) techniques are needed to make AI algorithms more transparent and understandable [29].
Addressing these data privacy, security, and ethical considerations is essential for building trust in wearable technology and ensuring its responsible use in healthcare. This requires a collaborative effort involving patients, healthcare providers, device manufacturers, regulators, and policymakers.
Many thanks to our sponsor Esdebe who helped us prepare this research report.
6. Future Directions and Potential Advancements
The field of wearable technology is rapidly evolving, with numerous opportunities for future advancements and improvements. Some key areas of focus include:
-
Advanced Sensor Technologies: The development of more accurate, reliable, and non-invasive sensors is crucial for expanding the range of applications for wearable technology. This includes sensors for monitoring biochemical markers, brain activity, and other physiological parameters. Miniaturization and improved energy efficiency are also important goals [30].
-
AI-Driven Personalized Healthcare: AI algorithms will play an increasingly important role in analyzing data from wearable devices and providing personalized insights and recommendations. This includes developing predictive models for disease prevention and personalized treatment plans [31].
-
Integration with Electronic Health Records (EHRs): Seamless integration of data from wearable devices into EHRs is essential for enabling clinicians to access and utilize this information effectively. This requires standardized data formats and interoperability standards [32].
-
Remote Patient Monitoring (RPM): Wearable technology will continue to play a key role in RPM programs, enabling healthcare providers to monitor patients remotely and provide timely interventions. This can improve patient outcomes, reduce hospitalizations, and lower healthcare costs [33].
-
Digital Therapeutics: Wearable devices can be used to deliver digital therapeutics, which are software-based interventions that are designed to treat or manage medical conditions. This includes apps for managing diabetes, mental health, and other chronic diseases [34].
-
Smart Textiles and Implants: The development of smart textiles and implantable sensors will expand the range of wearable technology applications. Smart textiles can be used to monitor physiological parameters and provide therapeutic interventions, while implantable sensors can provide continuous and long-term monitoring of vital signs [35].
-
Human-Computer Interaction (HCI): Enhancing the user experience of wearable devices is crucial for improving adherence and engagement. This includes developing intuitive interfaces, personalized feedback mechanisms, and gamified interventions [36].
The future of wearable technology in healthcare is bright, with the potential to transform the way we prevent, diagnose, and treat diseases. However, realizing this potential requires addressing the challenges related to data privacy, security, accuracy, and usability, as well as fostering collaboration between researchers, clinicians, and industry partners.
Many thanks to our sponsor Esdebe who helped us prepare this research report.
7. Conclusion
Wearable technology holds tremendous promise for revolutionizing healthcare by enabling continuous and personalized monitoring of physiological parameters. From cardiovascular health management to neurological disorder monitoring, wearable devices are already transforming how we approach diagnosis, treatment, and prevention. The integration of AI further amplifies these capabilities, enabling more sophisticated data analysis, personalized insights, and predictive modeling. However, realizing the full potential of wearable technology requires careful consideration of data privacy, security, accuracy, and ethical considerations. Addressing these challenges through robust security measures, data anonymization techniques, and transparent AI algorithms is crucial for building trust and ensuring the responsible use of these technologies. As sensor technology advances and AI algorithms become more sophisticated, wearable devices will continue to play an increasingly important role in transforming healthcare delivery, leading to improved patient outcomes, reduced healthcare costs, and a more proactive and personalized approach to health management. Future research should focus on developing more accurate and reliable sensors, enhancing AI-driven personalized healthcare solutions, and ensuring seamless integration with existing healthcare systems to maximize the impact of wearable technology on patient care and public health.
Many thanks to our sponsor Esdebe who helped us prepare this research report.
References
[1] Patel, S., Park, H., Bonato, P., Chan, L., & Rodgers, M. (2012). A review of wearable sensors and systems with application in rehabilitation. Journal of NeuroEngineering and Rehabilitation, 9(1), 21.
[2] Najafi, B., Aminian, K., Paraschiv-Ionescu, A., Loew, F., Bula, C. J., & Robert, P. (2003). Ambulatory system for human motion analysis using a miniature gyroscope and accelerometer. IEEE Transactions on Biomedical Engineering, 50(5), 614-623.
[3] Allen, J. (2007). Photoplethysmography and its application in clinical physiological measurement. Physiological Measurement, 28(3), R1.
[4] Reaz, M. B. I., Hussain, M. S., & Mohd-Yasin, F. (2006). Techniques of EMG signal analysis: detection, processing, classification and applications. Biological Procedures Online, 8(1), 11-35.
[5] Kim, J., Campbell, A. S., de Ávila, B. E. F., & Wang, J. (2019). Wearable biosensors for healthcare monitoring. Nature Biotechnology, 37(4), 389-406.
[6] Chaudhry, S. I., Phillips, C. O., Stewart, S. S., Riegel, B., Mattera, J. A., Jerant, A. F., … & Young, J. B. (2003). Telemonitoring for patients with chronic heart failure. Cochrane Database of Systematic Reviews, (2).
[7] Klonoff, D. C., & Curry, J. (2017). Continuous glucose monitoring: a review of the technology and its use. Diabetes Technology & Therapeutics, 19(S1), S4-S15.
[8] Poewe, W., Seppi, K., Tanner, G. R., Halliday, G. M., Brundin, P., Volkmann, J., … & Lang, A. E. (2017). Parkinson disease. Nature Reviews Disease Primers, 3(1), 1-21.
[9] Doshi, A., Jacobson, N. C., Getchell, M., Tiase, V. L., Griggs, R. K., Merrill, J., & Mooney, C. (2021). Wearable sensors for remote health monitoring in patients with chronic obstructive pulmonary disease: a systematic review. *International Journal of Chronic Obstructive Pulmonary Disease, 16, 2087.
[10] Arevian, A. C., Orland, C. M., Cohen, A. S., Hamilton, J. E., Olino, T. M., Abramson, L. Y., … & Alloy, L. B. (2022). Using wearable technology to predict changes in depressive symptoms: A systematic review. *Journal of Affective Disorders, 300, 1-16.
[11] Lau, K. H., Vahid, B., & Chen, F. (2020). A review of wearable sensor technologies and their applications in rehabilitation. Sensors, 20(17), 4523.
[12] Estévez, A. M., Saaidi, T., Álvarez, M., García, J., & Domínguez, J. A. (2021). Deep learning for wearable sensor-based activity recognition: An overview. Sensors, 21(14), 4752.
[13] Kumar, S., Weatherall, J., Gadaleta, D., Ali, D., Black, R., Kumar, P., … & Kulkarni, S. (2022). Personalized health insights using wearables: A systematic review. Journal of the American Medical Informatics Association, 29(1), 173-188.
[14] Topol, E. J. (2019). High-performance medicine: the convergence of human and artificial intelligence. Nature Medicine, 25(1), 44-56.
[15] Haluza, D., Naszay, M., Stockinger, A., Jungwirth, D., & Kundi, M. (2020). Benefits and risks of using wearable sensors in healthcare. Wien Klin Wochenschr, 132(3-4), 74-80.
[16] Price, W. N., & Cohen, I. G. (2019). Privacy in the age of medical big data. Nature Medicine, 25(1), 37-43.
[17] Obermeyer, Z., Powers, B., Vogeli, C., & Mullainathan, S. (2019). Dissecting racial bias in an algorithm used to manage the health of populations. Science, 366(6464), 447-453.
[18] Adadi, A., & Berrada, M. (2018). Peeking inside the black-box: Explainable AI (XAI). *IEEE Access, 6, 52138-52160.
[19] Benjamins, R., Hind, M., & Foote, D. (2020). Regulatory review of artificial intelligence-based medical devices. Journal of Law and the Biosciences, 7(1), lsaa003.
[20] Lane, N. D., Georgiev, P., Qendro, L., Liswianto, I., Musa, A. I., Radu, P., & Berenzweig, R. (2015). DeepX: A software accelerator for low-power deep learning on mobile devices. Proceedings of the 13th Annual International Conference on Mobile Systems, Applications, and Services, 517-530.
[21] Kaye, J., Curren, L., Anderson, N., Edwards, K., Fullerton, S. M., Kanellopoulou, N., … & Meslin, E. M. (2015). From patients to partners: participant-centric initiatives in biomedical research. Nature Reviews Genetics, 16(6), 371-382.
[22] Narayanan, A., & Shmatikov, V. (2008). Robust de-anonymization of large sparse datasets. 2008 IEEE Symposium on Security and Privacy (sp 2008), 111-125.
[23] Ohm, P. (2010). Broken promises of privacy: Responding to the surprising failure of anonymization. *UCLA Law Review, 57, 1701.
[24] Stallings, W. (2017). Cryptography and network security: principles and practice. Pearson Education.
[25] Sandhu, R. S., Coyne, E. J., Feinstein, H. L., & Youman, C. E. (1996). Role-based access control models. IEEE Computer, 29(2), 38-47.
[26] Weber, R. H. (2015). Internet of Things–New security and privacy challenges. Computer Law & Security Review, 31(3), 321-331.
[27] Beauchamp, T. L., & Childress, J. F. (2019). Principles of biomedical ethics. Oxford University Press.
[28] Angwin, J., Larson, J., Mattu, S., & Kirchner, L. (2016). Machine bias. ProPublica, 23.
[29] Miller, T. (2019). Explanation in artificial intelligence: Insights from the social sciences. *Artificial Intelligence, 267, 1-38.
[30] Mukerjee, E. V., Banerjee, A., Singh, B., Verma, A., & Koley, C. (2022). Advances in wearable sensors and their diverse applications. *Sensors and Actuators A: Physical, 337, 113431.
[31] Jiang, F., Jiang, Y., Jiang, X., Li, W., Chen, H., Xiao, M., … & Wang, Y. (2017). Artificial intelligence in healthcare: past, present and future. Stroke and Vascular Neurology, 2(4), 230-243.
[32] Holtz, B., & Patel, V. (2014). Healthcare information technology and patient engagement: a review of the literature. Journal of Medical Systems, 38(1), 1-9.
[33] McLean, S., Nurmatov, U., Liu, J. L., Pagliari, C., Car, J., Sheikh, A. (2011). Telehealthcare for chronic diseases. Cochrane Database of Systematic Reviews, (3).
[34] Hamine, S., Gerull, R., & Mansfield, C. (2015). Mobile phone text messaging intervention for disease management: a systematic review. Journal of Medical Internet Research, 17(2), e4.
[35] Atalay, A., Winkler, J., Wiorek, A., Pflaum, M., Ortmaier, T., & Haring, M. (2017). Integration of textile sensors and implants in health monitoring applications: A review. Sensors, 17(12), 2947.
[36] Yardley, L., Spring, B. J., Riper, H., Morrison, L. G., Crane, D., Curtis, K., … & Dennison, L. (2016). New digital technologies to support adherence to health behavior change interventions. *Current Opinion in Behavioral Sciences, 9, 119-125.
The report mentions the potential of AI in predictive modeling. Could this extend to creating personalized risk scores based on combined wearable sensor data, lifestyle information, and even genetic predispositions, thus enabling truly preventative, individualized healthcare strategies?
That’s an excellent point! Combining wearable data with lifestyle and genetic factors for personalized risk scores is definitely a promising direction. The challenge will be ethically and effectively integrating these diverse data streams while ensuring privacy and avoiding bias in the risk assessments. Exciting times ahead!
Editor: MedTechNews.Uk
Thank you to our Sponsor Esdebe
The discussion of challenges is critical. Algorithmic bias, especially in diverse populations, is an area needing constant vigilance. Continual monitoring and refinement of algorithms are vital to equitable healthcare.
Thanks for highlighting the crucial issue of algorithmic bias! Your point about diverse populations is spot-on. We need to ensure algorithms are trained on representative datasets and rigorously tested to prevent unintended consequences in healthcare. Let’s keep pushing for fairness and transparency in these systems!
Editor: MedTechNews.Uk
Thank you to our Sponsor Esdebe