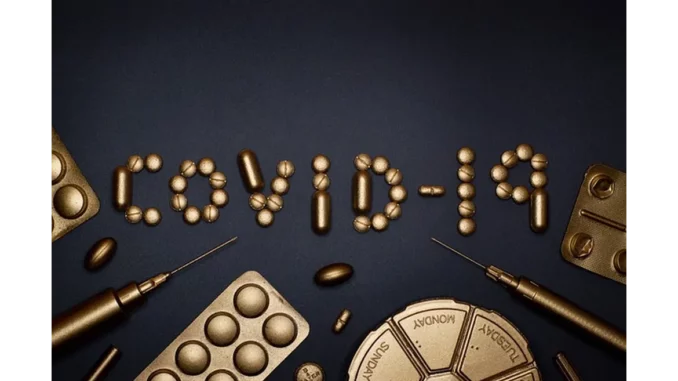
In the wake of the COVID-19 pandemic, long COVID, or post-COVID-19 syndrome, has surfaced as a pressing public health issue. This condition, characterised by a diverse array of symptoms persisting long after the initial infection, includes chronic fatigue, cognitive difficulties often referred to as “brain fog,” and various respiratory challenges. While initial research efforts indicated that approximately 7 percent of the population might be affected by long COVID, recent insights from a study employing an artificial intelligence (AI) tool developed by Mass General Brigham suggest a significantly higher prevalence of 22.8 percent.
The innovative AI tool leverages electronic health records to provide clinicians with a robust framework for identifying cases of long COVID. Constructed using anonymised patient data from nearly 300,000 individuals across 14 hospitals and 20 community health centres, this tool represents a major advancement in the diagnosis and understanding of this complex syndrome. Published in the journal Med, the study underscores AI’s transformative potential in the diagnostic landscape of long COVID, offering a more precise and less biased alternative to traditional methods.
Diagnosing long COVID presents unique challenges due to its nature as a diagnosis of exclusion, where other potential causes for symptoms must be rigorously ruled out. The AI tool, developed by Estiri and colleagues at the Center for AI and Biomedical Informatics of the Learning Healthcare System (CAIBILS) at Mass General Brigham, employs a technique known as “precision phenotyping.” This method meticulously analyses individual health records to pinpoint symptoms and conditions associated with COVID-19, effectively distinguishing them from other ailments.
The implications of this technological advancement are profound, particularly in addressing the biases inherent in existing diagnostic practices. Conventional methods often depend on the ICD-10 diagnostic code for long COVID, which can inadvertently favour populations with greater healthcare access. The AI tool, conversely, reflects the diverse demographic composition of Massachusetts, ensuring that underrepresented communities are considered in the diagnostic process.
Nevertheless, the study does acknowledge certain limitations. The health record data used by the algorithm might not be as comprehensive as the information contained in post-visit clinical notes. Moreover, the algorithm may not fully capture the exacerbation of pre-existing conditions that could signify long COVID. The decline in COVID-19 testing in recent years also complicates the task of pinpointing when a patient might have initially contracted the virus.
Looking to the future, researchers plan to apply the algorithm to cohorts of patients with particular conditions, such as chronic obstructive pulmonary disease (COPD) or diabetes. There is also an intention to make the algorithm publicly accessible, enabling healthcare professionals and systems worldwide to integrate it into their patient care practices. This initiative could lead to enhanced clinical care and foster targeted research into the genetic and biochemical underpinnings of long COVID’s various subtypes.
The development of this AI tool signifies a pivotal advancement in demystifying long COVID. By equipping clinicians with a more refined diagnostic process, it sets the stage for more effective treatment strategies and a deeper understanding of this perplexing condition. As research progresses, the aspiration is that these advancements will yield improved outcomes for those afflicted by long COVID, ultimately easing its impact on individuals and healthcare systems around the globe.
Be the first to comment