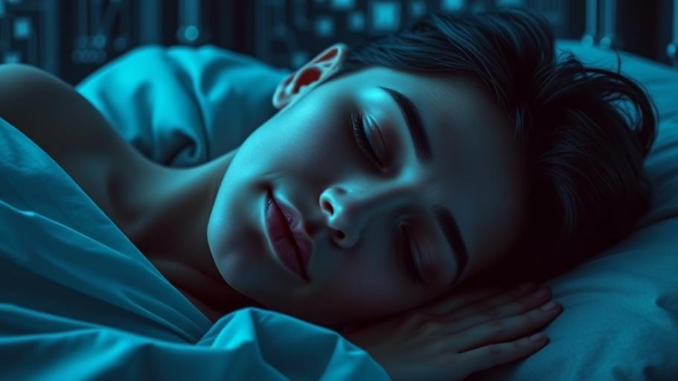
Summary
Researchers developed an AI model, PFTSleep, capable of analyzing full nights of sleep with high accuracy. Trained on over a million hours of sleep data, PFTSleep analyzes brain waves, muscle activity, and other physiological signals. This breakthrough has the potential to revolutionize sleep disorder diagnosis and treatment.
** Main Story**
AI Revolutionizes Sleep Analysis: The Arrival of PFTSleep
Researchers at the Icahn School of Medicine at Mount Sinai have unveiled a groundbreaking AI model, PFTSleep (patch foundational transformer for sleep). This innovative tool analyzes an entire night’s sleep with remarkable accuracy, marking a significant advancement in sleep medicine. Published in the journal Sleep, the study used a massive dataset of over one million hours of sleep data, making it the largest of its kind. PFTSleep leverages the same transformer architecture found in large language models like ChatGPT, processing brain waves, muscle activity, heart rate, and breathing patterns to classify sleep stages. This comprehensive approach represents a major improvement over traditional methods, which typically involve manual scoring of short sleep segments or AI models incapable of analyzing full-night sleep.
PFTSleep: A New Era in Sleep Analysis
Traditional sleep analysis methods suffer from limitations, relying on either human experts manually scoring short segments of sleep data or AI models unable to process a full night of sleep. This reliance on fragmented data can miss crucial patterns and nuances in sleep architecture. Furthermore, manual scoring can introduce variability and be time-consuming. PFTSleep overcomes these limitations by taking a holistic view of sleep. Trained on full-length sleep data, it identifies patterns throughout the night and across diverse populations and settings. This comprehensive analysis provides a standardized and scalable method for both research and clinical applications, promising more accurate and efficient sleep analysis.
The Potential of AI in Sleep Medicine
The development of PFTSleep heralds a new era in sleep medicine, offering numerous potential benefits:
- Streamlined Analysis: PFTSleep automates and standardizes sleep stage classification, making analysis faster and more efficient.
- Reduced Variability: By eliminating manual scoring, PFTSleep reduces subjectivity and produces more consistent results.
- Improved Diagnostic Accuracy: The comprehensive analysis capabilities of PFTSleep can lead to more accurate diagnosis of sleep disorders.
- Personalized Treatment: This AI tool allows for personalized insights into individual sleep patterns, paving the way for tailored interventions.
- Early Detection of Health Risks: Sleep quality is linked to various health risks. PFTSleep can aid in early identification of individuals at risk based on their sleep patterns.
Beyond its immediate applications, PFTSleep opens doors for future research. The model can detect subtle changes in sleep architecture that might be indicative of underlying health conditions. It can also help researchers better understand the relationship between sleep and various physical and mental health issues. As AI technology continues to evolve, tools like PFTSleep are poised to transform sleep medicine, leading to improved diagnosis, treatment, and overall sleep health.
One million hours of sleep data? So, if I hook myself up, will it finally explain why I’m convinced my brain is just a screensaver at 3 AM? Asking for a friend… who is definitely me.
That’s a great question! While PFTSleep isn’t quite ready for home use, your experience highlights the very issues we hope to address. Analyzing your sleep patterns could potentially offer some insight into that late-night “screensaver” mode. Hopefully we can provide a personalized experience soon!
Editor: MedTechNews.Uk
Thank you to our Sponsor Esdebe
The ability to analyze physiological signals comprehensively is fascinating. Could PFTSleep be adapted to detect sleep-related movement disorders, such as restless legs syndrome, with greater precision than current methods?
That’s an insightful question! The comprehensive nature of PFTSleep, with its analysis of brain waves, muscle activity, and heart rate, does lend itself to potentially detecting subtle movement patterns associated with conditions like restless legs syndrome. Further research exploring this is certainly warranted! Thanks for sparking that thought.
Editor: MedTechNews.Uk
Thank you to our Sponsor Esdebe
Given the model’s capability to process diverse physiological signals, could PFTSleep be utilized to identify specific biomarkers within sleep data indicative of broader systemic health issues beyond sleep disorders?
That’s a fantastic point! The potential for PFTSleep to identify biomarkers is something we’re actively exploring. By analyzing subtle physiological variations during sleep, we hope to uncover connections to conditions beyond sleep disorders. It could truly revolutionize preventative healthcare!
Editor: MedTechNews.Uk
Thank you to our Sponsor Esdebe