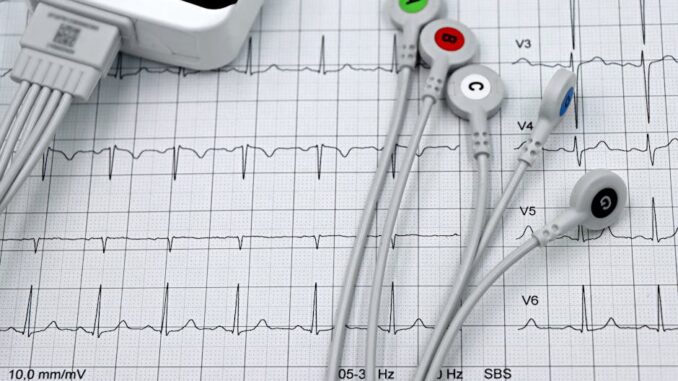
Summary
FSU researchers demonstrate how AI can significantly improve the accuracy of differential diagnoses, potentially revolutionizing healthcare. The study highlighted the importance of lab data in enhancing AI’s diagnostic capabilities, with GPT-4 achieving particularly impressive results. This breakthrough paves the way for more informed clinical decisions and improved patient outcomes.
Healthcare data growth can be overwhelming scale effortlessly with TrueNAS by Esdebe.
** Main Story**
AI: A Game-Changer in Differential Diagnosis?
Researchers at Florida State University’s eHealth Lab are exploring the transformative potential of Artificial Intelligence (AI) in healthcare, specifically its ability to enhance the accuracy of differential diagnoses. Differential diagnosis, the process of distinguishing between diseases with similar symptoms, is a cornerstone of effective medical practice. The FSU team’s research, published in npj Digital Medicine, has garnered significant attention, highlighting the growing interest in AI’s role in healthcare.
This study builds upon FSU’s LabGenie project, an initiative designed to improve older adults’ understanding of lab test results. The team investigated the use of Large Language Models (LLMs), a type of AI trained on vast amounts of text data, to assist clinicians in making more precise and efficient differential diagnoses. Five different LLMs were tested: GPT-4, GPT-3.5, Llama-2-70b, Claude-2, and Mixtral-8x7B. Researchers used clinical vignettes, or narrative patient case studies, derived from 50 case reports to evaluate the LLMs’ performance.
The Power of Lab Data
A key finding of the study was the significant impact of lab test data on diagnostic accuracy. When provided with lab results, the LLMs demonstrated a marked improvement in their ability to identify the correct diagnosis. GPT-4, in particular, achieved remarkable results, boasting 55% top-one accuracy (meaning the correct diagnosis was the AI’s first suggestion) and 60% top-ten accuracy when incorporating lab data. Even more impressively, GPT-4’s lenient accuracy, which considers related or similar diagnoses, reached 80%. These findings underscore the importance of integrating lab information into AI-driven diagnostic tools.
The study also revealed that the LLMs effectively interpreted various lab tests, including liver function tests, metabolic/toxicology panels, and serology/immune tests. This ability to accurately process and analyze lab data is crucial for AI to effectively assist clinicians in complex diagnostic scenarios. Furthermore, the study demonstrated the LLMs’ proficiency in identifying the correct diagnosis even in cases of rare diseases, further showcasing their potential to revolutionize medical practice.
Implications for the Future of Healthcare
The FSU researchers’ findings have far-reaching implications for the future of healthcare. By enhancing the accuracy and efficiency of differential diagnoses, AI can empower clinicians to make more informed decisions, leading to improved patient outcomes and potentially even saving lives. The integration of AI into healthcare also promises to streamline workflows, reduce diagnostic errors, and provide more personalized treatment plans.
While this research demonstrates the remarkable potential of AI in healthcare, it’s crucial to recognize that AI is a tool meant to augment, not replace, human expertise. Clinicians’ judgment, experience, and patient interaction remain essential components of effective healthcare. AI should be viewed as a powerful ally, providing clinicians with valuable insights and support to enhance their diagnostic capabilities. As AI technology continues to evolve, we can expect even more profound advancements in healthcare, paving the way for a future where AI and human expertise work in synergy to deliver the best possible patient care.
The study’s findings on GPT-4’s accuracy are compelling. How might the integration of real-time patient data, such as continuous glucose monitoring or heart rate variability, further enhance AI’s diagnostic precision in dynamic clinical scenarios?