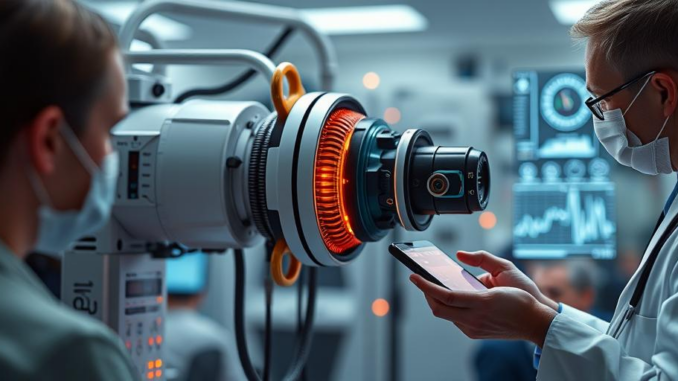
Summary
A recent study reveals that machine learning models for predicting mortality in hospital settings fail to identify two-thirds of severe injuries. This highlights a significant gap in current AI capabilities, raising concerns about patient safety and the need for improved model training. The article explores the study’s findings, discusses the implications for AI in healthcare, and suggests potential solutions for enhancing predictive accuracy.
Healthcare data growth can be overwhelming scale effortlessly with TrueNAS by Esdebe.
** Main Story**
AI is making waves in healthcare, promising incredible advancements in how we diagnose, treat, and care for patients. But, and this is a big ‘but’, a recent study published in Nature Communications Medicine throws a bit of cold water on the hype surrounding AI’s predictive capabilities, specifically when it comes to predicting in-hospital mortality. Basically, these models that are supposed to flag patients at high risk of death? They apparently missed about 66% of severe injuries that could actually lead to a patient’s demise. That’s, um, not ideal, is it?
What the Study Showed, and Why It Matters
Researchers at Virginia Tech, along with some other smart folks, put a bunch of ML mortality prediction models through their paces. The models had been trained using only patient data. The results weren’t pretty. It turns out, these models often missed critical health events and signs that a patient was going downhill fast. Consequently, the predictions they made, well they were deemed unreliable for effective intervention. What does that even mean? It means that relying on these inaccurate predictions could actually delay life-saving treatments and, frankly, that could compromise patient outcomes. Nobody wants that, right? Remember that time our hospital implemented a new AI system for scheduling, and it doubled the workload for everyone in the first month? It’s kinda like that, except, you know, with potentially much graver consequences.
So, What’s Going Wrong?
It seems that just dumping a bunch of patient data into ML models isn’t enough to train them to accurately gauge future health risks. The study suggests that the models just don’t get the complex interplay of factors that ultimately lead to mortality. The really need to have a better understanding of like the body’s responses to serious injury and illness if they are going to get this right. That is why, we need much better training methods to make any of this useful in a practical sense.
How to Make These Models Better
Alright, so the current models aren’t perfect. But, don’t worry, there are some ways to improve how they work:
- Real-Time Data is Key: Think about it: hooking these models up to real-time data streams – vital signs monitors, lab results, all that jazz. It’d give them a more dynamic and complete view of what’s happening with a patient.
- Context Matters, Especially with Injuries: The severity of an injury is really important to consider. Models need to factor in things like, how did the injury happen? Does the patient have any pre-existing conditions? Are there individual quirks that might influence the outcome?
- Explainable AI (XAI) – Demystifying the Machine: We need to be able to understand why the model is making a particular prediction. This helps spot potential biases or inaccuracies, allowing clinicians to make better informed decisions. Are there red flags in the underlying data that caused the AI to assume a outcome? We need to know.
- Human Oversight is a Must: Even with the best AI, we can’t just take a hands-off approach. Human oversight, that crucial clinical judgement, can act as a check on the model’s predictions. It will ensure that critical cases don’t fall through the cracks, and that the assumptions made by the AI are correct.
- Constant Learning and Feedback: We should be constantly comparing model predictions to what actually happens with patients. That can help refine the model’s accuracy and make it more responsive over time. After all, AI is supposed to learn, right? If its making incorrect assumtions, then we need to step in to refine its learning.
- Let’s Share Data (Responsibly): Healthcare institutions and researchers need to work together more and share data (while respecting privacy, of course!). This could lead to more robust and generalizable models that actually work across different populations.
Stepping Back: AI’s Role in Healthcare
This study really highlights the importance of thoroughly testing and validating any AI tools before we start using them in healthcare settings. AI has incredible potential to improve patient care, but we can’t ignore its limitations or potential risks. How would we even handle, ethically, if an AI misdiagnosed a condition? What legal ramifications would that have? Using AI responsibly means thinking carefully about ethical issues, data privacy, and how clinicians will work alongside these systems. And, as AI keeps evolving, we’re going to need clear guidelines and regulations to keep patients safe and get the most out of this technology.
Where Do We Go From Here?
The bottom line? Current mortality prediction models need more work. A lot more work. But, by investing in better training methods, incorporating real-time data, and prioritizing human oversight, we can build AI tools that actually empower clinicians and, most importantly, improve patient outcomes. It’s a challenge, sure, but it’s one worth tackling. Because, at the end of the day, isn’t patient safety what really matters?
The study mentions the need for “explainable AI.” How might focusing on the *process* by which these models arrive at their predictions, rather than solely the outcome, contribute to increased trust and adoption among healthcare professionals?
Great point! Focusing on the *process* behind AI predictions, rather than just the outcome, is crucial. If healthcare professionals can understand *how* an AI arrived at a certain conclusion, they’re more likely to trust it and integrate it into their workflows. This transparency is key for building confidence and ensuring responsible use.
Editor: MedTechNews.Uk
Thank you to our Sponsor Esdebe