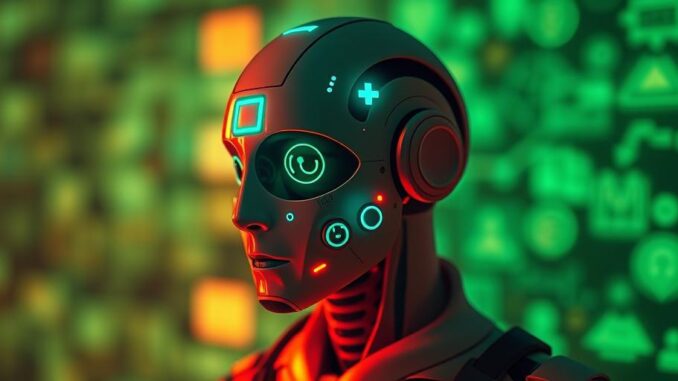
Summary
This article explores the crucial role of trustworthy AI in revolutionizing healthcare. It discusses key principles like transparency, explainability, and fairness, and highlights the need for rigorous evaluation and validation to ensure patient safety and positive health outcomes. Collaboration between stakeholders is essential for building trust and maximizing the benefits of AI in medicine.
Start with a free consultation to discover how TrueNAS can transform your healthcare data management.
** Main Story**
AI’s making waves in healthcare, no doubt about it. We’re seeing potential for massive improvements in diagnostics, treatments, and overall patient care. Think early disease detection and personalized medicine – it’s pretty revolutionary stuff. But here’s the thing: before we fully embrace AI in these critical settings, we have to be absolutely certain that it’s trustworthy, right? Let’s dive into what it takes to build and maintain that trust.
Transparency and Explainability: Peeking Inside the ‘Black Box’
So, one of the biggest roadblocks to trusting AI is that it can often feel like a ‘black box.’ You know, the algorithm does its thing, spits out an answer, but how did it get there? It is important for clinicians to understand this. They won’t trust something they can’t understand. Explainable AI (XAI) aims to fix this. It gives us insights into the AI’s thought process. Techniques like feature attribution, for example, help us see why a particular diagnosis is suggested.
This transparency is key. For instance, if an AI flags a particular symptom as high-risk, XAI can show you which factors led to that conclusion. Was it the patient’s age, specific test results, or something else? It builds trust, yes, but, it also lets clinicians spot any biases or flaws that might be lurking in the model. I remember a project where the AI was consistently misdiagnosing a rare condition in women over 50. Turned out the training data was heavily skewed towards male patients – a classic case of bias creeping in.
Fairness and Impartiality: Equal Access for Everyone
If we’re not careful, AI could make existing healthcare inequalities even worse, and it’s important that we are careful. AI models need to be trained on diverse datasets. That means including data from different demographics, ethnicities, and socioeconomic backgrounds. Otherwise, the AI might end up favoring one group over another.
Imagine an AI trained mostly on data from urban populations. It might struggle to accurately diagnose patients from rural areas, where symptoms and risk factors could be different. Monitoring and evaluation are crucial, so we can catch any emerging biases early. And let’s be honest; it’s not just about avoiding discrimination, it’s about ensuring that everyone benefits from the advancements in AI-powered healthcare.
Robustness and Reliability: Making Sure It Works Every Time
In healthcare, the stakes are sky-high. We can’t afford AI models that are flaky or unreliable. Rigorous testing and validation are non-negotiable, I can’t stress this enough. We need to make sure these systems perform accurately, no matter who the patient is or what the clinical scenario looks like.
Conformal prediction is one way to do this. It provides a set of probable diagnoses, with a strong guarantee that the correct one is included. It’s like saying, “We’re 99% confident the diagnosis is one of these three possibilities.” This gives clinicians more confidence, you know? It’s far more reassuring than a single, definitive answer with no context.
Navigating Uncertainty: When AI Isn’t 100% Sure
Think about medical imaging – it’s not always clear-cut. Conditions like pleural effusion and pulmonary infiltrates can look similar on a chest X-ray. AI can help, but there’s often uncertainty in the predictions. That’s where conformal classification comes in.
Instead of giving a single answer, it offers a range of possible diagnoses. It acknowledges the ambiguity and provides clinicians with multiple options to consider. It can be used in conjunction with test-time augmentation (TTA). TTA involves creating multiple variations of a single image, running the model on each, and then combining the results. This can lead to more reliable predictions, less possibilities and better accuracy for clinicians.
Collaboration and Regulation: Building a Solid Foundation
Building trust in healthcare AI isn’t something we can do in isolation. It requires everyone to be on board: clinicians, researchers, policymakers, and patients. Clear ethical guidelines and regulatory frameworks are essential. They ensure that AI is developed and used responsibly. International collaborations, like the FUTURE-AI consortium, are working on these guidelines. They’re covering everything from design and validation to regulation and monitoring. The goal? To ensure that AI is fair, universal, traceable, usable, robust, and explainable, across the whole AI lifecycle.
The Ultimate Goal: Patient Safety and Positive Outcomes
At the end of the day, it’s all about improving patient safety and health outcomes. By focusing on transparency, fairness, robustness, and continuous evaluation, we can unlock the incredible potential of AI while minimizing the risks. Academia and industry must work together. They need to bridge the gap between research and real-world application. Only then can we create AI systems that are not only effective but also ethical and worthy of our trust.
AI misdiagnosing conditions in women over 50 due to skewed training data? Sounds like we need an AI ‘diversity and inclusion’ officer! Perhaps AI needs to attend mandatory sensitivity training? Just kidding… mostly. Seriously though, excellent points about the need for diverse data sets.