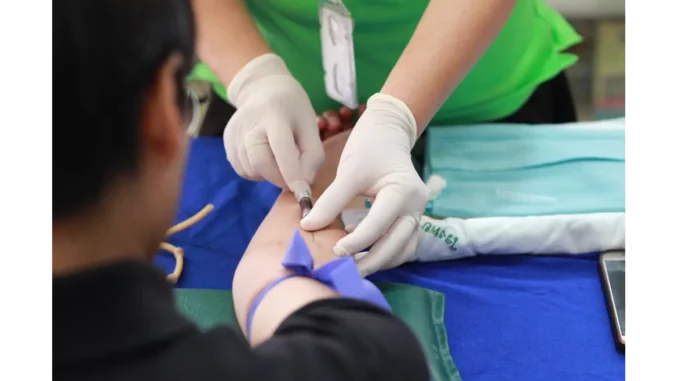
In a world progressively reliant on technology, the imperative to ensure fairness and equity within these systems has reached unprecedented significance. This is particularly true within the healthcare sector, where algorithms wield considerable influence over patient outcomes. In a recent conversation, I spoke with Amber Nigam, a committed health data scientist and co-founder of a generative AI-driven healthcare company, who is on a mission to eliminate racial bias in medical technology. His narrative is a compelling blend of personal tragedy and professional dedication, sparked by a profound experience that reshaped his career trajectory.
Amber’s journey took root at the bedside of his father, who was battling Covid-19. “Observing my father’s pulse oximeter struggle with his darker skin was a pivotal moment,” Amber recalled. “One instant it indicated a normal reading, and the next it would drop alarmingly. This inconsistency was disconcerting and exacerbated the distress of an already harrowing experience.” Although the inaccuracies of the pulse oximeter were not directly responsible for his father’s passing, they illuminated a critical flaw in medical technology that Amber felt compelled to address.
As a researcher at the Massachusetts Institute of Technology, Amber was already aware of emerging studies on racial biases in medical devices. However, this personal encounter galvanized his resolve to confront these disparities in his work. “Making healthcare AI more trustworthy wasn’t merely a professional duty—it became a deeply personal mission,” Amber explained. His pursuit of creating unbiased AI models was fraught with challenges. “Despite access to vast datasets from reputable sources like the Mayo Clinic, we quickly realised that large datasets don’t necessarily equate to fair ones,” Amber noted. “These datasets often lacked the racial and ethnic diversity required to make accurate predictions across all demographics.”
To address this, Amber and his team embarked on a rigorous process of testing and refining their algorithms. They introduced “dataset diversification and balancing,” striving to ensure their models fairly represented all races and ethnicities. “We collaborated with partners like Mayo to tackle blind spots, particularly in detecting cardiovascular events among Black Americans,” he said. “This required understanding how historical exclusions from medical trials had created these blind spots.” The team also implemented manual validation checkpoints to ensure data accuracy and balance. “Through these checks, we identified missing demographic data for minority patients, such as age and family medical history, which skewed predictions,” Amber elaborated. “Correcting these assumptions was crucial for enhancing our model’s accuracy.”
When real-world data proved insufficient, they resorted to synthetic data to fill the gaps. “For Hispanic populations, we used synthetic scenarios to expose our model to a more diverse sample,” Amber explained. This approach enabled them to simulate realistic situations, thereby enhancing the model’s predictive capabilities across different ethnic groups. The team also addressed inconsistencies in socioeconomic data, which often skewed predictions for lower-income patients. “We developed an algorithmic intervention to flag and re-weight problematic data, ensuring our predictions remain equitable,” he said.
Among their most innovative measures was the establishment of algorithmic guardrails to prevent racial biases. “We included a fairness audit mechanism that triggers whenever the model’s performance for any racial group falls below a certain threshold,” Amber noted. “During testing, for example, we identified lower accuracy for Asian populations and adjusted the dataset accordingly.” The results of these efforts were significant, albeit not without trade-offs. “Our initial accuracy was about 98%, but after addressing these biases, it dropped by about 7%,” Amber acknowledged. “This might seem like a setback, but it means our model is less likely to make incorrect confident predictions in sensitive cases. We’re now more confident in our model’s race-related objectivity by 15-20%.”
Amber emphasised that while no model can be perfect, continuous monitoring and improvement are essential. “We treat fairness as an evolving commitment, regularly refining our models to detect and address emerging biases,” he stated. “This ensures equitable outcomes in healthcare.” Reflecting on the broader implications, Amber expressed optimism about generative AI’s potential to help solve racial bias in healthcare. “Racial bias is a human problem first, and technology reflects those human biases,” he said. “With the right attention and intention, healthcare leaders can address this issue across their organisations, not just in IT.”
Amber’s journey underscores the importance of vigilance and dedication in tackling racial bias in AI. His work serves as a poignant reminder that while technology can perpetuate biases, it also holds the potential to rectify them—with the right leadership and commitment. As we navigate the complexities of AI in healthcare, Amber’s story is a stirring call to action for those in the industry to prioritise fairness and equity.
Be the first to comment