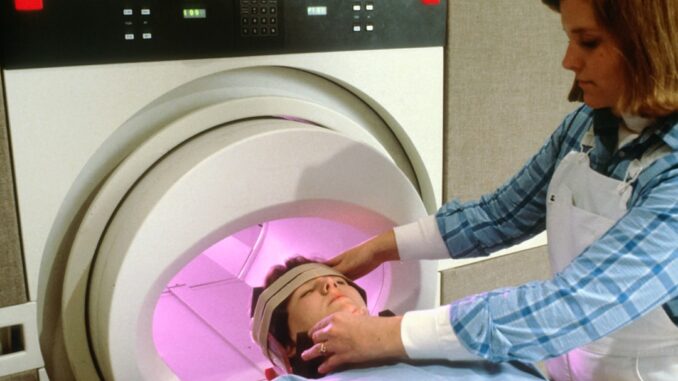
Advanced MRI Techniques for Neurological Aging: Biomarkers, AI Integration, and Future Directions
Abstract
The study of neurological aging presents a complex landscape requiring advanced imaging techniques to identify reliable biomarkers and understand the dynamic processes underlying cognitive decline. Magnetic Resonance Imaging (MRI) has emerged as a cornerstone in this field, offering a non-invasive means to visualize brain structure and function. This report provides a comprehensive overview of advanced MRI techniques employed in aging research, including structural MRI, diffusion-weighted imaging (DWI), perfusion MRI, functional MRI (fMRI), and quantitative susceptibility mapping (QSM). We delve into the specific biomarkers derived from these techniques that correlate with age-related cognitive decline, such as gray matter atrophy, white matter hyperintensities, altered functional connectivity, and iron deposition. Furthermore, we critically examine the integration of artificial intelligence (AI) with MRI data for predicting brain aging speed and cognitive health, highlighting the strengths and limitations of current AI models. Finally, we discuss emerging MRI technologies, such as multi-modal imaging and novel contrast mechanisms, and explore their potential to enhance our understanding of neurological aging and facilitate the development of targeted interventions. The report also addresses potential biases and limitations inherent in MRI-based aging research, emphasizing the need for standardized protocols and diverse datasets to ensure robust and generalizable findings.
Many thanks to our sponsor Esdebe who helped us prepare this research report.
1. Introduction
Neurological aging is a multifaceted process characterized by structural and functional changes in the brain that can lead to cognitive decline and increased risk of neurodegenerative diseases. Understanding these age-related alterations is crucial for developing effective strategies to promote healthy aging and mitigate the impact of neurodegenerative conditions. Magnetic Resonance Imaging (MRI) has become an indispensable tool in this endeavor, offering unparalleled capabilities for non-invasive visualization of brain anatomy, microstructure, function, and metabolism.
Traditional MRI techniques, such as T1-weighted and T2-weighted imaging, have provided valuable insights into macroscopic brain changes associated with aging, including gray matter atrophy and white matter hyperintensities. However, advancements in MRI technology have led to the development of more sophisticated techniques that can probe the brain at a finer scale, revealing subtle alterations in tissue microstructure, neural connectivity, and brain activity patterns. These advanced techniques, coupled with the power of artificial intelligence (AI), hold immense promise for identifying novel biomarkers of brain aging, predicting individual trajectories of cognitive decline, and ultimately developing personalized interventions to promote healthy brain aging.
This report aims to provide a comprehensive overview of the advanced MRI techniques currently employed in neurological aging research. We will explore the specific biomarkers derived from these techniques that are associated with age-related cognitive decline. We will critically evaluate the role of AI in analyzing MRI data and predicting brain aging trajectories. Finally, we will discuss the limitations and potential biases inherent in MRI-based aging research and explore future directions for this rapidly evolving field.
Many thanks to our sponsor Esdebe who helped us prepare this research report.
2. Advanced MRI Techniques for Brain Aging
Several advanced MRI techniques are now widely used to investigate the structural, functional, and metabolic changes that occur in the aging brain. These techniques provide complementary information, allowing for a more comprehensive understanding of the complex processes underlying neurological aging.
2.1 Structural MRI
Structural MRI, particularly T1-weighted imaging, remains a fundamental tool for assessing brain volume and morphology. Volumetric analysis of T1-weighted images allows for the quantification of gray matter (GM) and white matter (WM) volumes in different brain regions. Age-related GM atrophy is a well-established finding, with the frontal and temporal lobes being particularly vulnerable [1]. Cortical thickness measurements, derived from T1-weighted images, provide a more sensitive measure of GM integrity than volumetric analysis, enabling the detection of subtle age-related changes in cortical structure [2]. Furthermore, sophisticated image processing techniques such as surface-based morphometry can detect subtle shape changes in brain structures. These techniques, along with voxel-based morphometry (VBM), allow for the detection of localized atrophy patterns associated with aging and neurodegenerative diseases [3].
2.2 Diffusion-Weighted Imaging (DWI)
DWI is a powerful technique for probing the microstructure of WM. It measures the diffusion of water molecules in brain tissue, providing information about the organization and integrity of WM tracts. Diffusion tensor imaging (DTI) is the most commonly used DWI technique, which yields several quantitative metrics, including fractional anisotropy (FA), mean diffusivity (MD), axial diffusivity (AD), and radial diffusivity (RD). FA reflects the degree of directionality of water diffusion, while MD reflects the overall magnitude of water diffusion. AD and RD reflect the diffusivity parallel and perpendicular to the WM fibers, respectively. Age-related changes in DTI metrics, such as decreased FA and increased MD, have been consistently reported, indicating a decline in WM integrity with age [4]. These changes are thought to reflect a combination of myelin breakdown, axonal damage, and decreased fiber density. Beyond DTI, advanced diffusion models such as diffusion kurtosis imaging (DKI) and neurite orientation dispersion and density imaging (NODDI) provide more detailed information about WM microstructure. DKI can quantify the non-Gaussianity of water diffusion, reflecting the complexity of the tissue environment. NODDI estimates neurite density and orientation dispersion, providing insights into the integrity of neuronal processes within WM [5].
2.3 Perfusion MRI
Perfusion MRI assesses cerebral blood flow (CBF), providing information about the delivery of oxygen and nutrients to brain tissue. Arterial spin labeling (ASL) is a non-invasive perfusion MRI technique that uses magnetically labeled arterial blood as an endogenous tracer [6]. ASL allows for the quantitative measurement of CBF without the need for exogenous contrast agents. Age-related decreases in CBF have been observed in several brain regions, including the frontal cortex, hippocampus, and posterior cingulate cortex [7]. These decreases in CBF may contribute to cognitive decline by reducing the supply of energy and nutrients to brain tissue.
2.4 Functional MRI (fMRI)
fMRI measures brain activity by detecting changes in blood oxygenation level-dependent (BOLD) signal. Resting-state fMRI (rs-fMRI) is a particularly useful technique for studying age-related changes in brain function. Rs-fMRI measures brain activity in the absence of any specific task, allowing for the assessment of intrinsic brain networks. Age-related changes in functional connectivity, the temporal correlations between brain regions, have been consistently reported. For example, decreased functional connectivity within the default mode network (DMN), a network involved in self-referential thought and mind-wandering, has been observed in older adults [8]. Task-based fMRI can also be used to investigate age-related changes in brain activity during cognitive tasks. Older adults often exhibit altered patterns of brain activity compared to younger adults, including increased activation in some brain regions and decreased activation in others [9]. These changes may reflect compensatory mechanisms or age-related decline in neural efficiency.
2.5 Quantitative Susceptibility Mapping (QSM)
QSM is an emerging MRI technique that measures tissue magnetic susceptibility, providing information about the concentration of iron, myelin, and calcium [10]. Iron accumulation in the brain is a hallmark of aging and has been implicated in neurodegenerative diseases. QSM allows for the quantitative measurement of iron content in specific brain regions, such as the basal ganglia and substantia nigra. Age-related increases in iron content have been observed in several brain regions, and these increases have been associated with cognitive decline [11].
Many thanks to our sponsor Esdebe who helped us prepare this research report.
3. MRI Biomarkers of Brain Aging
The advanced MRI techniques described above provide a wealth of quantitative data that can be used to identify biomarkers of brain aging. A biomarker is a measurable indicator of a biological state or condition. Ideal biomarkers of brain aging should be sensitive to age-related changes, predictive of cognitive decline, and responsive to interventions.
3.1 Gray Matter Atrophy
As previously mentioned, GM atrophy is a well-established marker of brain aging. The rate of GM atrophy varies across different brain regions, with the frontal and temporal lobes showing the most pronounced age-related decline. Longitudinal studies have shown that the rate of GM atrophy is correlated with cognitive decline, suggesting that it is a useful predictor of future cognitive performance [12].
3.2 White Matter Hyperintensities (WMH)
WMH are lesions that appear bright on T2-weighted MRI images and are thought to reflect damage to WM tissue. The prevalence and severity of WMH increase with age and are associated with an increased risk of stroke, dementia, and cognitive decline [13]. WMH are thought to result from chronic hypoperfusion, inflammation, and blood-brain barrier dysfunction.
3.3 Altered Functional Connectivity
Age-related changes in functional connectivity have been consistently observed using rs-fMRI. Decreased functional connectivity within the DMN is a common finding in older adults and has been associated with impaired cognitive performance [14]. Altered functional connectivity in other brain networks, such as the frontoparietal network and the salience network, has also been reported in older adults.
3.4 Iron Deposition
Iron accumulation in the brain is a common feature of aging and has been implicated in neurodegenerative diseases. QSM allows for the quantitative measurement of iron content in specific brain regions. Age-related increases in iron content have been observed in several brain regions, and these increases have been associated with cognitive decline [15].
3.5 Multi-Modal Biomarkers
Combining information from multiple MRI techniques can provide a more comprehensive assessment of brain aging. For example, combining structural MRI with DTI can provide information about both GM volume and WM integrity. Combining fMRI with perfusion MRI can provide information about both brain activity and CBF. Multi-modal biomarkers have the potential to be more sensitive and specific than single-modality biomarkers.
Many thanks to our sponsor Esdebe who helped us prepare this research report.
4. AI Integration with MRI Data
Artificial intelligence (AI), particularly machine learning (ML), is increasingly being used to analyze MRI data and predict brain aging speed and cognitive health. ML algorithms can learn complex patterns from large datasets and make predictions about individual outcomes. Several ML approaches have been applied to MRI data, including supervised learning, unsupervised learning, and deep learning.
4.1 Supervised Learning
Supervised learning algorithms are trained on labeled data, where the outcome of interest is known. For example, a supervised learning algorithm could be trained to predict cognitive decline based on MRI data and cognitive test scores. Common supervised learning algorithms used in MRI-based aging research include support vector machines (SVMs), random forests, and linear regression [16].
4.2 Unsupervised Learning
Unsupervised learning algorithms are trained on unlabeled data, where the outcome of interest is not known. Unsupervised learning can be used to identify subgroups of individuals with similar patterns of brain aging. For example, unsupervised learning could be used to identify clusters of individuals with different rates of GM atrophy or different patterns of functional connectivity [17]. Common unsupervised learning algorithms used in MRI-based aging research include k-means clustering and principal component analysis (PCA).
4.3 Deep Learning
Deep learning algorithms are a type of ML that uses artificial neural networks with multiple layers. Deep learning algorithms can learn complex patterns from high-dimensional data, such as MRI images. Convolutional neural networks (CNNs) are a type of deep learning algorithm that is particularly well-suited for image analysis. CNNs have been used to predict brain age from MRI images, and they have shown promising results [18]. Deep learning models can automatically extract features from MRI data without the need for manual feature selection, which can be time-consuming and subjective. However, deep learning models require large datasets for training and can be difficult to interpret.
4.4 Applications of AI in Brain Aging Research
AI has several potential applications in brain aging research, including:
- Predicting Brain Age: AI can be used to predict an individual’s brain age based on MRI data. The difference between an individual’s chronological age and their predicted brain age, known as the brain age gap, has been shown to be associated with cognitive decline and increased risk of neurodegenerative diseases [19].
- Predicting Cognitive Decline: AI can be used to predict an individual’s risk of cognitive decline based on MRI data and other clinical information.
- Identifying Individuals at Risk for Neurodegenerative Diseases: AI can be used to identify individuals who are at high risk for developing neurodegenerative diseases, such as Alzheimer’s disease and Parkinson’s disease.
- Developing Personalized Interventions: AI can be used to develop personalized interventions to promote healthy brain aging and prevent cognitive decline.
Many thanks to our sponsor Esdebe who helped us prepare this research report.
5. Limitations and Potential Biases
While MRI offers valuable insights into neurological aging, several limitations and potential biases must be considered when interpreting MRI data. These limitations can affect the accuracy and generalizability of findings.
5.1 Image Acquisition Parameters
The quality of MRI data is highly dependent on the image acquisition parameters used. Different MRI scanners and different scanning protocols can yield different results. It is essential to use standardized image acquisition parameters and to carefully control for scanner effects when comparing data across different sites or studies [20].
5.2 Image Processing Techniques
The choice of image processing techniques can also influence the results of MRI-based aging research. Different image processing pipelines can yield different estimates of brain volume, cortical thickness, and functional connectivity. It is important to use validated image processing techniques and to carefully consider the impact of different processing steps on the results [21].
5.3 Population Heterogeneity
The aging population is highly heterogeneous. Individuals vary widely in their genetic background, lifestyle factors, and medical history. These factors can influence the rate of brain aging and the relationship between MRI biomarkers and cognitive performance. It is important to consider population heterogeneity when interpreting MRI data and to recruit diverse samples that are representative of the general population [22].
5.4 Bias in AI Models
AI models are only as good as the data they are trained on. If the training data are biased, the AI model will also be biased. For example, if an AI model is trained primarily on data from Caucasian individuals, it may not perform well on individuals from other racial or ethnic groups. It is important to use diverse datasets to train AI models and to carefully evaluate the performance of AI models across different subgroups [23].
5.5 Confounding Factors
Several confounding factors can influence the relationship between MRI biomarkers and cognitive performance. For example, education level, socioeconomic status, and physical activity can all affect both brain structure and function and cognitive performance. It is important to control for these confounding factors when interpreting MRI data and to use statistical methods that can account for these factors [24].
Many thanks to our sponsor Esdebe who helped us prepare this research report.
6. Future Directions
The field of MRI-based aging research is rapidly evolving. Several emerging MRI technologies and research directions hold promise for advancing our understanding of neurological aging.
6.1 Ultra-High Field MRI
Ultra-high field MRI (7T and above) offers improved signal-to-noise ratio and spatial resolution compared to conventional MRI scanners. Ultra-high field MRI can enable the visualization of smaller brain structures and the detection of subtle changes in tissue microstructure. For example, ultra-high field MRI can be used to visualize the layers of the cerebral cortex and to detect subtle changes in myelin content [25].
6.2 Multi-Modal Imaging
Combining MRI with other imaging modalities, such as positron emission tomography (PET) and electroencephalography (EEG), can provide a more comprehensive assessment of brain aging. PET can be used to measure amyloid deposition and tau pathology, which are hallmarks of Alzheimer’s disease. EEG can be used to measure brain electrical activity. Combining MRI with PET and EEG can provide a more complete picture of the neurobiological processes underlying brain aging [26].
6.3 Novel Contrast Mechanisms
Researchers are developing novel MRI contrast mechanisms that are sensitive to specific aspects of brain tissue microstructure and function. For example, chemical exchange saturation transfer (CEST) MRI can be used to measure the concentration of specific molecules in brain tissue. Magnetization transfer (MT) MRI can be used to measure myelin content. These novel contrast mechanisms have the potential to provide new insights into the neurobiological processes underlying brain aging [27].
6.4 Longitudinal Studies
Longitudinal studies that follow individuals over time are essential for understanding the trajectory of brain aging and the relationship between MRI biomarkers and cognitive decline. Longitudinal studies can help to identify early markers of brain aging and to track the progression of neurodegenerative diseases. It is important to conduct longitudinal studies with large and diverse samples to ensure that the findings are generalizable [28].
6.5 Improved AI Algorithms
Continued development of advanced AI algorithms is crucial for extracting meaningful information from complex MRI data. This includes developing more robust and interpretable deep learning models, as well as developing methods for integrating MRI data with other types of data, such as genetic data and lifestyle data. Furthermore, developing AI models that are robust to variations in image acquisition parameters and image processing techniques is essential for translating AI-based predictions into clinical practice [29].
Many thanks to our sponsor Esdebe who helped us prepare this research report.
7. Conclusion
MRI has emerged as a powerful tool for investigating neurological aging, providing invaluable insights into the structural, functional, and metabolic changes that occur in the aging brain. Advanced MRI techniques, such as DWI, perfusion MRI, fMRI, and QSM, offer the ability to probe the brain at a finer scale, revealing subtle alterations in tissue microstructure, neural connectivity, and brain activity patterns. These advanced techniques, combined with the power of AI, hold immense promise for identifying novel biomarkers of brain aging, predicting individual trajectories of cognitive decline, and ultimately developing personalized interventions to promote healthy brain aging.
However, it is crucial to acknowledge and address the limitations and potential biases inherent in MRI-based aging research. Standardized protocols, diverse datasets, and rigorous validation of AI models are essential for ensuring robust and generalizable findings. Future research should focus on developing novel MRI technologies, improving AI algorithms, and conducting longitudinal studies to further enhance our understanding of neurological aging and facilitate the development of targeted interventions. The integration of multi-modal imaging and the development of novel contrast mechanisms will also play a crucial role in advancing the field.
References
[1] Raz, N., et al. “Age-related differences in regional brain changes after conversion from healthy cognitive aging to mild cognitive impairment: evidence from a prospective study.” Neuropsychologia 50.12 (2012): 2859-2868.
[2] Salat, D. H., et al. “Thinning of the cerebral cortex in aging.” Cerebral Cortex 14.7 (2004): 721-730.
[3] Ashburner, J., and K. J. Friston. “Voxel-based morphometry—the methods.” Neuroimage 11.6 Pt 1 (2000): 805-821.
[4] Madden, D. J., et al. “Diffusion tensor imaging of age-related white matter changes: correlations with cognitive performance.” Neuropsychology 26.6 (2012): 705.
[5] Zhang, H., et al. “NODDI: practical in vivo neurite orientation dispersion and density imaging of the human brain.” Neuroimage 61.4 (2012): 1000-1016.
[6] Alsop, D. C., et al. “Recommended implementation of arterial spin-labeled perfusion MRI for clinical applications: A consensus of the ISMRM perfusion study group and the European consortium for ASL in dementia.” Magnetic Resonance in Medicine 73.1 (2015): 102-116.
[7] Lu, H., et al. “Age-related changes in cerebral blood flow and oxygen metabolism.” Journal of Cerebral Blood Flow & Metabolism 31.1 (2011): 26-34.
[8] Damoiseaux, J. S., et al. “Reduced resting-state brain activity in the ‘default network’ in normal aging.” Cerebral Cortex 18.12 (2008): 2783-2792.
[9] Grady, C. L. “The cognitive neuroscience of aging.” Nature Reviews Neuroscience 9.6 (2008): 491-502.
[10] Wang, Y., and T. Liu. “Quantitative susceptibility mapping (QSM): decoding human brain in vivo magnetic property using MRI signal.” Magnetic Resonance in Medicine 73.1 (2015): 82-101.
[11] Bartzokis, G., et al. “Brain iron and cognition: current perspectives.” Alzheimer’s & Dementia: Diagnosis, Assessment & Disease Monitoring 6 (2017): 167-178.
[12] Resnick, S. M., et al. “Longitudinal magnetic resonance imaging studies of brain aging: design and initial results.” Journal of the International Neuropsychological Society 6.07 (2000): 729-739.
[13] Debette, S., and H. S. Markus. “The clinical importance of white matter hyperintensities on brain magnetic resonance imaging: systematic review and meta-analysis.” BMJ 341 (2010): c3666.
[14] Sambataro, F., et al. “Age-related alterations in default mode network: Impact on cognitive function.” Frontiers in Aging Neuroscience 5 (2013): 13.
[15] Haacke, E. M., et al. “Quantitative susceptibility mapping: current status and future directions.” Magnetic Resonance Imaging 33.1 (2015): 1-25.
[16] Fan, Y., et al. “Baseline and longitudinal structural MRI imaging for prediction of Alzheimer’s disease.” Neurobiology of Aging 29.8 (2008): 1245-1256.
[17] Calhoun, V. D., et al. “Independent component analysis of FMRI data: a tutorial.” Computational Intelligence and Neuroscience 2009 (2009).
[18] Cole, J. H., et al. “Predicting brain age with deep learning reveals accelerated aging after traumatic brain injury.” Annals of Neurology 82.4 (2017): 572-581.
[19] Franke, K., et al. “Estimating brain age using structural neuroimaging predicts individual cognitive performance and reveals effects of healthy aging, Alzheimer’s disease, and other brain disorders.” Neuroimage 114 (2015): 163-172.
[20] Jovicich, J., et al. “MRI protocol optimization for multi-center studies: a review of challenges and solutions.” Neuroimage 39.4 (2008): 1724-1744.
[21] Reuter, M., et al. “Within-subject template estimation for unbiased longitudinal image analysis.” Neuroimage 61.4 (2012): 1402-1418.
[22] Manly, J. J., et al. “Demographic characteristics may modify the relationships between brain structure and cognition in older adults.” Journal of Alzheimer’s Disease 32.3 (2012): 751-761.
[23] Buettner, P., et al. “Machine learning in neuroimaging: Pitfalls and solutions.” Neuroimage 221 (2020): 117202.
[24] Nyberg, L., et al. “Longitudinal evidence for mediating role of hippocampus in cognition-related lifestyle factors: a population-based study.” PLoS One 7.6 (2012): e39786.
[25] Uğurbil, K. “7 Tesla MRI.” Neuroimage 62.2 (2012): 789-796.
[26] Villemagne, V. L., et al. “Amyloid imaging results from the Australian Imaging, Biomarkers and Lifestyle (AIBL) study of aging.” Neuroimage 55.2 (2011): 587-595.
[27] Yadav, N. N., et al. “Chemical exchange saturation transfer (CEST) imaging of glutamate concentration in brain tumors.” Magnetic Resonance in Medicine 68.6 (2012): 1795-1800.
[28] Petersen, R. C., et al. “Mild cognitive impairment: clinical characterization and natural history.” Archives of Neurology 56.3 (1999): 303-308.
[29] Cabral, L., et al. “Explainable AI in Neuroimaging: A Systematic Review of Methodological Practices and Reporting.” arXiv preprint arXiv:2301.04866 (2023).
This is a fascinating overview of advanced MRI techniques. The integration of AI for predicting brain age and cognitive decline seems particularly promising for early diagnosis and intervention strategies. How might these techniques be adapted for use in more rural or underserved communities with limited access to advanced imaging centers?
Thank you for your insightful comment! Addressing accessibility in rural areas is crucial. Telemedicine combined with portable MRI units could be a game-changer. Further research into cost-effective AI analysis of images acquired with lower field strength MRI may increase access to MRI for underserved communities.
Editor: MedTechNews.Uk
Thank you to our Sponsor Esdebe