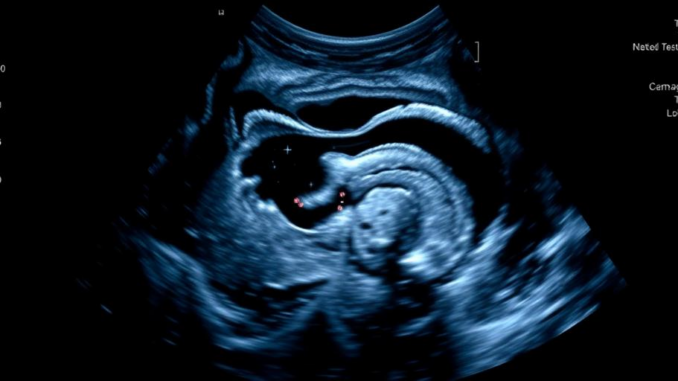
Abstract
Photoacoustic imaging (PAI) has emerged as a powerful biomedical imaging modality, offering unique advantages in visualizing tissue structures and physiological processes by combining the high contrast of optical imaging with the deep penetration of ultrasound. This research report delves into the advanced principles underpinning PAI, exploring recent innovations across various applications, and critically assessing its strengths and limitations compared to established techniques such as magnetic resonance imaging (MRI) and ultrasound. The current research landscape, focusing on areas like multi-wavelength PAI, high-resolution techniques, and contrast agent development, is thoroughly reviewed. We further discuss the potential future developments in the field, including the integration of artificial intelligence, advancements in laser technology, and exploration of novel applications in areas such as immunotherapy monitoring and early cancer detection. This report aims to provide an in-depth understanding of the state-of-the-art in PAI, catering to experts in the field and highlighting key areas for future research and development.
Many thanks to our sponsor Esdebe who helped us prepare this research report.
1. Introduction
Photoacoustic imaging (PAI) is a non-invasive hybrid imaging technique that exploits the photoacoustic effect. Upon absorption of pulsed laser light, biological tissues undergo thermoelastic expansion, generating broadband ultrasonic waves that are detected by ultrasound transducers. The amplitude of the generated ultrasonic waves is proportional to the local optical absorption, providing valuable information about tissue composition and physiological parameters such as hemoglobin concentration, oxygen saturation, and lipid content. This intrinsic sensitivity to optical absorption enables PAI to achieve high contrast in imaging tissues with varying optical properties, offering a significant advantage over traditional ultrasound imaging. Furthermore, the use of ultrasound detection overcomes the limitations of purely optical imaging techniques, which suffer from severe scattering in biological tissues, limiting their penetration depth.
PAI has demonstrated considerable potential in a wide range of biomedical applications, including cancer detection and monitoring, cardiovascular imaging, dermatology, and brain imaging. The technique’s ability to visualize microvasculature, monitor drug delivery, and assess tissue metabolism makes it a valuable tool for preclinical research and clinical diagnostics. With advancements in laser technology, transducer design, and image reconstruction algorithms, PAI has evolved rapidly, enabling higher resolution, deeper penetration, and faster imaging speeds. This report provides a comprehensive overview of the current state of PAI, highlighting key innovations, challenges, and future directions in this rapidly evolving field.
Many thanks to our sponsor Esdebe who helped us prepare this research report.
2. Principles of Photoacoustic Imaging
The photoacoustic effect, the cornerstone of PAI, involves three fundamental processes: light absorption, thermoelastic expansion, and ultrasound detection. Understanding these processes is crucial for optimizing PAI system design and image quality.
2.1 Light Absorption and Thermoelastic Expansion
When a pulsed laser beam illuminates biological tissue, photons are absorbed by endogenous chromophores, such as hemoglobin, melanin, lipids, and water, as well as exogenous contrast agents. The absorbed energy is rapidly converted into heat, causing a localized temperature rise. If the laser pulse duration is shorter than the thermal relaxation time of the tissue, the temperature increase leads to a rapid thermoelastic expansion, generating a pressure wave. The magnitude of this pressure wave is directly proportional to the product of the Grüneisen parameter, the optical absorption coefficient, and the local light fluence.
Mathematically, the initial photoacoustic pressure, p0, can be expressed as:
p0 = Γ μa F
where:
- Γ is the Grüneisen parameter, representing the efficiency of converting absorbed energy into pressure.
- μa is the optical absorption coefficient of the tissue.
- F is the local optical fluence (energy per unit area).
The Grüneisen parameter is a material property that depends on the thermal expansion coefficient, the isobaric specific heat, and the speed of sound in the tissue. Its value varies significantly between different tissues and physiological conditions, impacting the amplitude of the generated photoacoustic signals.
2.2 Ultrasound Detection and Image Reconstruction
The generated ultrasonic waves propagate through the tissue and are detected by ultrasound transducers. The transducers convert the pressure waves into electrical signals, which are then amplified and digitized. The spatial distribution of the initial photoacoustic pressure is reconstructed from the detected signals using various image reconstruction algorithms.
Several reconstruction algorithms are commonly employed in PAI, including:
- Time-domain back-projection: This is a widely used algorithm that reconstructs the image by summing the time-delayed signals received at each transducer element. The delay is calculated based on the distance between the transducer element and the potential source location.
- Frequency-domain k-space algorithms: These algorithms utilize the Fourier transform to reconstruct the image in the frequency domain, offering improved computational efficiency compared to time-domain methods. However, they often require accurate knowledge of the speed of sound in the tissue.
- Model-based iterative reconstruction: These algorithms incorporate a forward model of the photoacoustic wave propagation and iteratively refine the reconstructed image by minimizing the difference between the simulated and measured signals. While computationally intensive, these methods can improve image quality, particularly in complex geometries or heterogeneous media.
The choice of reconstruction algorithm depends on factors such as the imaging geometry, the desired image resolution, and the available computational resources.
Many thanks to our sponsor Esdebe who helped us prepare this research report.
3. Instrumentation and System Design
A typical PAI system consists of a pulsed laser source, an ultrasound transducer array, a data acquisition system, and image reconstruction software. The performance of the system is heavily influenced by the characteristics of each component.
3.1 Laser Sources
The selection of the laser source is critical for PAI, as it determines the penetration depth, contrast, and resolution of the image. Key parameters to consider include the wavelength, pulse duration, pulse repetition rate, and average power.
- Wavelength: The choice of wavelength depends on the targeted chromophores and the desired penetration depth. Near-infrared (NIR) wavelengths (650-950 nm) are commonly used due to the lower absorption and scattering of light in biological tissues in this spectral region. However, shorter wavelengths can be used to target specific chromophores with strong absorption in the visible spectrum. Multi-wavelength PAI can provide spectral information, enabling the differentiation of various tissue components.
- Pulse Duration: Short laser pulses (nanoseconds or picoseconds) are preferred to ensure efficient thermoelastic expansion and minimize thermal diffusion artifacts. The pulse duration should be shorter than the thermal relaxation time of the targeted tissue.
- Pulse Repetition Rate: A higher pulse repetition rate allows for faster data acquisition and improved signal-to-noise ratio (SNR). However, it also increases the average power delivered to the tissue, which can lead to thermal damage. Careful consideration must be given to the safety limits for laser exposure.
- Laser Types: Commonly used laser types include Nd:YAG lasers, optical parametric oscillators (OPOs), and diode lasers. OPOs offer tunable wavelengths, allowing for spectral PAI. Diode lasers are compact and cost-effective but may have lower pulse energies compared to Nd:YAG lasers.
3.2 Ultrasound Transducers
The ultrasound transducer array detects the generated photoacoustic signals and converts them into electrical signals. The transducer characteristics, such as center frequency, bandwidth, element size, and number of elements, influence the spatial resolution, sensitivity, and field of view of the PAI system.
- Center Frequency: Higher frequency transducers offer better spatial resolution but have lower penetration depth due to increased attenuation of ultrasound in tissue. Lower frequency transducers provide deeper penetration but sacrifice resolution. The optimal frequency depends on the application and the targeted tissue depth.
- Bandwidth: A wider bandwidth improves the axial resolution of the image. Broadband transducers can also capture a wider range of frequencies, allowing for improved image quality and artifact reduction.
- Element Size and Number of Elements: Smaller element sizes and a larger number of elements increase the sampling density and improve the spatial resolution of the image. However, a larger number of elements also increases the complexity and cost of the system.
- Transducer Types: Different types of transducers are used in PAI, including linear arrays, curved arrays, and two-dimensional arrays. Two-dimensional arrays enable volumetric imaging but require more complex data acquisition and processing.
3.3 Data Acquisition and Processing
The data acquisition system digitizes and stores the signals received from the ultrasound transducer array. Key parameters include the sampling rate, number of channels, and dynamic range. Signal processing techniques are used to improve the SNR and reduce artifacts in the acquired data.
Common signal processing techniques include:
- Averaging: Averaging multiple acquisitions reduces random noise and improves the SNR.
- Filtering: Bandpass filtering removes unwanted frequencies and improves the image contrast.
- Time-gain compensation (TGC): TGC compensates for the attenuation of ultrasound in tissue, improving the uniformity of the image.
- Beamforming: Beamforming focuses the ultrasound beam and improves the spatial resolution of the image.
Many thanks to our sponsor Esdebe who helped us prepare this research report.
4. Applications of Photoacoustic Imaging
PAI has found diverse applications in preclinical and clinical settings, owing to its unique ability to visualize both anatomical structures and physiological processes.
4.1 Oncology
PAI is particularly promising in oncology for tumor detection, staging, and monitoring of treatment response. The increased angiogenesis and metabolic activity in tumors lead to elevated hemoglobin concentration and oxygen consumption, which can be readily visualized by PAI.
- Early Cancer Detection: PAI can detect small tumors and micro-metastases that may be missed by conventional imaging techniques. The high sensitivity to hemoglobin allows for the visualization of tumor-associated vasculature, providing early indicators of malignancy.
- Monitoring Treatment Response: PAI can monitor the changes in tumor vascularity and oxygenation during and after treatment, providing valuable information about the efficacy of chemotherapy, radiation therapy, and immunotherapy. The technique can also be used to assess drug delivery to tumors.
- Image-Guided Interventions: PAI can be integrated with interventional procedures, such as biopsies and ablations, to provide real-time guidance and improve the accuracy of targeting.
4.2 Cardiovascular Imaging
PAI offers unique advantages in cardiovascular imaging, allowing for the visualization of plaque morphology, blood flow dynamics, and vessel wall inflammation.
- Atherosclerosis Imaging: PAI can differentiate between stable and vulnerable plaques based on their lipid content and inflammatory activity. The technique can also be used to monitor the progression of atherosclerosis and the response to therapeutic interventions.
- Coronary Artery Imaging: Intravascular photoacoustic (IVPA) imaging can visualize the coronary arteries with high resolution, providing detailed information about the vessel wall structure and the presence of plaques. IVPA can be used to guide stent placement and assess the efficacy of angioplasty.
- Peripheral Vascular Disease: PAI can assess the blood flow in peripheral arteries and detect areas of stenosis or occlusion. The technique can also be used to monitor the healing of ulcers and wounds.
4.3 Dermatology
PAI is well-suited for dermatological applications, allowing for the visualization of skin structures, such as the epidermis, dermis, and subcutaneous fat. The technique can be used to diagnose skin cancer, assess wound healing, and monitor cosmetic procedures.
- Skin Cancer Diagnosis: PAI can differentiate between benign and malignant skin lesions based on their vascularity and melanin content. The technique can also be used to assess the depth of tumor invasion.
- Wound Healing Assessment: PAI can monitor the formation of new blood vessels during wound healing, providing valuable information about the healing process. The technique can also be used to assess the efficacy of wound dressings and topical treatments.
- Cosmetic Applications: PAI can visualize the effects of cosmetic procedures, such as laser resurfacing and dermal fillers. The technique can also be used to assess the depth of wrinkles and the thickness of the skin.
4.4 Brain Imaging
PAI can be used to image the brain, providing information about cerebral blood flow, oxygenation, and neuronal activity. However, the limited penetration depth of light through the skull poses a significant challenge.
- Functional Brain Imaging: PAI can measure changes in cerebral blood flow and oxygenation in response to neuronal activity, providing insights into brain function. The technique can be used to study cognitive processes, such as learning, memory, and attention.
- Stroke Imaging: PAI can detect areas of ischemia and infarction in the brain following a stroke. The technique can also be used to monitor the recovery of blood flow after thrombolysis.
- Brain Tumor Imaging: PAI can visualize brain tumors and assess their vascularity. The technique can also be used to guide surgical resection of brain tumors.
Many thanks to our sponsor Esdebe who helped us prepare this research report.
5. Advantages and Limitations
PAI offers several advantages over other imaging modalities, but also faces certain limitations that need to be addressed for its widespread clinical adoption.
5.1 Advantages
- High Contrast: PAI provides high contrast based on the optical absorption properties of tissues, allowing for the visualization of specific chromophores and physiological processes.
- Deep Penetration: PAI achieves deeper penetration than purely optical imaging techniques, due to the use of ultrasound detection.
- Non-Ionizing Radiation: PAI uses non-ionizing radiation, making it a safe imaging modality for repeated examinations.
- Multimodal Imaging: PAI can be combined with other imaging modalities, such as ultrasound and MRI, to provide complementary information.
- Real-Time Imaging: PAI can provide real-time images, allowing for the monitoring of dynamic processes.
5.2 Limitations
- Limited Penetration Depth: The penetration depth of PAI is limited by the attenuation of light and ultrasound in tissue.
- Image Artifacts: Image artifacts can arise due to scattering, absorption, and reflection of light and ultrasound.
- Laser Safety: Careful consideration must be given to the safety limits for laser exposure to avoid thermal damage.
- Cost: PAI systems can be expensive, due to the cost of the laser and ultrasound transducer array.
- Image Reconstruction Complexity: Image reconstruction algorithms can be computationally intensive, particularly for volumetric imaging.
Many thanks to our sponsor Esdebe who helped us prepare this research report.
6. Recent Innovations and Research Landscape
The field of PAI is continuously evolving, with ongoing research focused on improving image quality, expanding applications, and developing new technologies.
6.1 Multi-Wavelength Photoacoustic Imaging
Multi-wavelength PAI exploits the spectral dependence of optical absorption to differentiate between various tissue components. By acquiring images at multiple wavelengths, it is possible to quantify the concentrations of different chromophores, such as hemoglobin, melanin, and lipids. Spectral unmixing algorithms are used to separate the contributions of each chromophore to the photoacoustic signal. This technique has been used for a range of applications, including oxygen saturation mapping, lipid quantification in atherosclerotic plaques, and monitoring of drug delivery.
6.2 High-Resolution Photoacoustic Microscopy (PAM)
Photoacoustic microscopy (PAM) achieves high spatial resolution by focusing the laser beam to a small spot and scanning it across the sample. Two main types of PAM exist: optical-resolution PAM (OR-PAM) and acoustic-resolution PAM (AR-PAM). OR-PAM achieves lateral resolution limited by the optical diffraction limit, while AR-PAM achieves lateral resolution limited by the ultrasound diffraction limit. PAM has been used for imaging microvasculature, cell morphology, and subcellular structures.
6.3 Contrast Agent Development
Exogenous contrast agents can enhance the contrast and sensitivity of PAI. Various types of contrast agents have been developed, including nanoparticles, dyes, and microbubbles. Nanoparticles can be designed to target specific cells or tissues, allowing for targeted imaging. Dyes can be used to enhance the absorption of light at specific wavelengths. Microbubbles can be used to enhance the generation of photoacoustic signals.
6.4 Photoacoustic Computed Tomography (PACT)
Photoacoustic computed tomography (PACT) acquires photoacoustic signals from multiple angles around the sample, allowing for three-dimensional image reconstruction. PACT can provide high-resolution images of deep tissues. Different PACT configurations exist, including linear array PACT, circular array PACT, and spherical array PACT.
6.5 Artificial Intelligence in PAI
Artificial intelligence (AI) is playing an increasingly important role in PAI. AI algorithms can be used for image reconstruction, artifact reduction, and image analysis. Deep learning algorithms have shown promise in improving the quality and speed of image reconstruction. AI can also be used to automatically detect and classify tumors in photoacoustic images.
Many thanks to our sponsor Esdebe who helped us prepare this research report.
7. Future Directions
The future of PAI is bright, with numerous opportunities for further research and development. Some key areas for future exploration include:
7.1 Advanced Laser Technologies
Advancements in laser technology, such as the development of compact and cost-effective tunable lasers, will enable broader application of multi-wavelength PAI. The development of high-repetition-rate lasers will allow for faster imaging speeds and improved SNR. The use of novel laser modalities, such as femtosecond lasers, could potentially improve the spatial resolution and contrast of PAI.
7.2 Enhanced Ultrasound Transducers
The development of high-density ultrasound transducer arrays will improve the spatial resolution and sensitivity of PAI. The integration of microfabricated ultrasound transducers with integrated circuits will enable the creation of miniaturized PAI probes for endoscopic and intravascular imaging. The use of novel transducer materials, such as capacitive micromachined ultrasonic transducers (CMUTs), could potentially improve the bandwidth and sensitivity of ultrasound detection.
7.3 AI-Powered Image Reconstruction and Analysis
AI will play an increasingly important role in PAI, enabling faster and more accurate image reconstruction, artifact reduction, and image analysis. Deep learning algorithms can be trained to recognize patterns and features in photoacoustic images, allowing for automated diagnosis and monitoring of disease. The integration of AI with PAI will lead to the development of smart imaging systems that can adapt to the specific needs of each patient.
7.4 Novel Applications
PAI has the potential to be used for a wide range of novel applications, including:
- Immunotherapy Monitoring: PAI can be used to monitor the response of tumors to immunotherapy by visualizing changes in immune cell infiltration and activation.
- Early Detection of Alzheimer’s Disease: PAI can be used to detect amyloid plaques in the brain, which are a hallmark of Alzheimer’s disease.
- Point-of-Care Diagnostics: PAI can be integrated into portable devices for point-of-care diagnostics, enabling rapid and accurate diagnosis of diseases in resource-limited settings.
Many thanks to our sponsor Esdebe who helped us prepare this research report.
8. Conclusion
Photoacoustic imaging has emerged as a powerful and versatile biomedical imaging modality, offering unique advantages in visualizing tissue structures and physiological processes. The technique’s ability to combine high contrast with deep penetration makes it a valuable tool for preclinical research and clinical diagnostics. Ongoing research efforts are focused on improving image quality, expanding applications, and developing new technologies. With continued advancements in laser technology, transducer design, and image reconstruction algorithms, PAI has the potential to revolutionize the field of biomedical imaging and contribute significantly to improved healthcare outcomes. The integration of artificial intelligence and the exploration of novel applications will further enhance the capabilities and impact of PAI in the future.
Many thanks to our sponsor Esdebe who helped us prepare this research report.
References
[1] Beard, P. (2011). Photoacoustic imaging for disease detection and diagnosis. Interface Focus, 1(4), 602-631.
[2] Wang, L. V., & Yao, J. (2016). A practical guide to photoacoustic tomography in the life sciences. Nature methods, 13(8), 627-638.
[3] Xu, M., & Wang, L. V. (2006). Photoacoustic imaging in biomedicine. Review of Scientific Instruments, 77(4), 041101.
[4] Weber, J., Beard, P. C., & Bohndiek, S. E. (2016). Contrast agents for molecular photoacoustic imaging. Nature Photonics, 10(3), 164-177.
[5] Deán-Ben, X. L., & Razansky, D. (2014). Advanced optoacoustic techniques for multiscale biomedical imaging. Chemical Society Reviews, 43(1), 27-43.
[6] Mallidi, S., Luke, G. P., Emelianov, S. Y., & Viator, J. A. (2009). Photoacoustic imaging in cancer detection, diagnosis, and therapy guidance. Trends in Biotechnology, 27(4), 213-221.
[7] Razansky, D., Vinegoni, C., Ntziachristos, V., & Weissleder, R. (2009). Multiscale optoacoustic imaging of vascular anatomy and function in vivo. Nature Photonics, 3(5), 258-262.
[8] Taruttis, A., Herzog, B. A., Beziere, N., & Razansky, D. (2015). Real-time three-dimensional optoacoustic tomography with full-ring transducer array. IEEE transactions on medical imaging, 34(2), 556-566.
[9] Wang, K., Maslov, K. I., Zhang, H. F., Hu, S., & Wang, L. V. (2013). High-resolution in vivo photoacoustic microscopy of microcirculation in human skin. Journal of Biomedical Optics, 18(12), 126009.
[10] Yao, J., Wang, L. V., & Nie, L. (2019). Photoacoustic molecular imaging. *Annual review of biomedical engineering, 21, 17-45.
[11] Antholzer, T., Haltmeier, M., & Schwab, M. (2017). Deep learning for photoacoustic tomography from sparse data. Inverse Problems, 34(1), 015004.
The discussion of AI-powered image reconstruction is compelling. How might advancements in AI, particularly in handling noisy or incomplete data, further enhance the accuracy and applicability of PAI in complex biological environments?
That’s a great point! Addressing noisy data is critical. Improved AI algorithms, perhaps using generative adversarial networks (GANs), could be trained to ‘fill in the blanks’ and distinguish true signal from noise in complex biological settings. This could significantly improve PAI’s diagnostic capabilities. What are your thoughts?
Editor: MedTechNews.Uk
Thank you to our Sponsor Esdebe
The discussion of AI-powered image reconstruction highlights exciting possibilities. Could AI also be leveraged to optimize laser pulse parameters in real-time, adapting to varying tissue types and depths to maximize signal quality and minimize potential tissue damage?