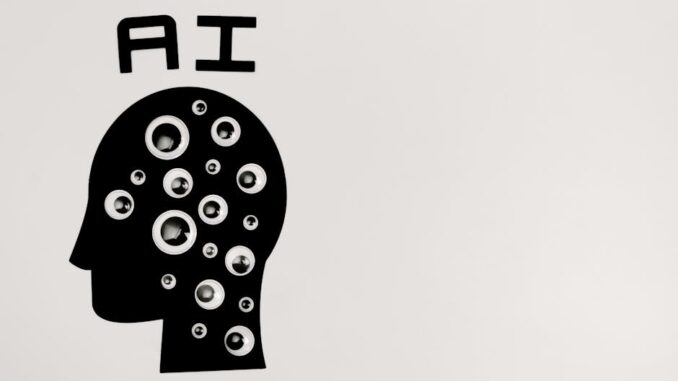
Abstract
Artificial intelligence (AI) is rapidly transforming numerous sectors, and healthcare is no exception. Beyond well-publicized applications such as sepsis prediction, AI is being deployed across a diverse range of areas, including diagnostics, personalized medicine, drug discovery, robotic surgery, and healthcare administration. This research report provides a comprehensive review of these applications, exploring their potential benefits, current limitations, and future directions. Furthermore, the report delves into the critical ethical, legal, and regulatory considerations surrounding AI in healthcare, focusing on data privacy, algorithmic bias, the evolving role of healthcare professionals, and the challenges of ensuring responsible innovation. We examine existing frameworks and propose avenues for fostering ethical and equitable AI deployment in healthcare, ultimately aiming to maximize its positive impact while mitigating potential risks.
Many thanks to our sponsor Esdebe who helped us prepare this research report.
1. Introduction
Artificial intelligence (AI) represents a paradigm shift in how we approach complex problems. Its ability to analyze vast datasets, identify patterns, and make predictions with remarkable accuracy has spurred innovation across various domains. In healthcare, AI is particularly promising, offering the potential to revolutionize diagnostics, treatment, drug discovery, and administrative processes. While early applications like expert systems showed promise, the recent surge in AI’s capabilities is primarily driven by advancements in machine learning (ML), particularly deep learning (DL), and the increasing availability of large-scale healthcare data.
The potential benefits are substantial. AI can assist clinicians in making more accurate and timely diagnoses, leading to improved patient outcomes. It can personalize treatment plans based on individual patient characteristics, optimizing therapeutic efficacy and minimizing adverse effects. AI-powered drug discovery platforms can accelerate the identification of novel drug targets and the development of new therapies. Furthermore, AI can streamline administrative tasks, freeing up healthcare professionals to focus on patient care. This report aims to provide a thorough examination of these applications, offering a critical assessment of their current state and future prospects.
However, the integration of AI into healthcare is not without its challenges. Ethical considerations, such as data privacy, algorithmic bias, and the impact on the human element of care, must be carefully addressed. Legal and regulatory frameworks need to be adapted to ensure responsible innovation and protect patient rights. Moreover, the successful deployment of AI in healthcare requires addressing issues related to data quality, interoperability, and the need for trust and transparency in AI systems.
This research report will explore these issues in detail, providing a balanced perspective on the potential and challenges of AI in healthcare. We will examine the key applications of AI, discuss the ethical and regulatory landscape, and propose recommendations for ensuring responsible and equitable AI deployment in this critical sector.
Many thanks to our sponsor Esdebe who helped us prepare this research report.
2. AI Applications in Healthcare
AI is being applied across a wide spectrum of healthcare functions. This section details some of the most significant areas of application.
2.1 Diagnostics
AI-powered diagnostic tools are rapidly evolving, demonstrating impressive capabilities in various medical specialties. In radiology, AI algorithms can analyze medical images, such as X-rays, CT scans, and MRIs, to detect anomalies, such as tumors, fractures, and other abnormalities, often with accuracy comparable to or even exceeding that of human radiologists. For example, deep learning models have shown promise in detecting lung cancer from CT scans [1], diagnosing diabetic retinopathy from retinal images [2], and identifying skin cancer from dermatoscopic images [3].
In pathology, AI can assist in analyzing tissue samples to identify cancerous cells and other pathological features. This can significantly speed up the diagnostic process and improve the accuracy of diagnoses. Computational pathology, facilitated by AI, allows for the quantification of biomarkers and the identification of subtle patterns that might be missed by human observers [4].
AI is also being used in other diagnostic areas, such as cardiology, where it can analyze electrocardiograms (ECGs) to detect arrhythmias and other heart conditions, and neurology, where it can analyze electroencephalograms (EEGs) to diagnose seizures and other neurological disorders. The integration of AI in diagnostics promises to enhance the efficiency and accuracy of disease detection, leading to earlier and more effective treatment.
2.2 Personalized Treatment
One of the most promising areas of AI in healthcare is personalized treatment. AI algorithms can analyze patient data, including genomic information, medical history, lifestyle factors, and environmental exposures, to develop individualized treatment plans that are tailored to each patient’s unique needs. This approach, often referred to as precision medicine, aims to optimize therapeutic efficacy and minimize adverse effects.
For example, in oncology, AI can be used to predict a patient’s response to chemotherapy or immunotherapy based on their genomic profile and other clinical characteristics. This can help oncologists select the most effective treatment regimen for each patient, increasing the likelihood of a positive outcome. AI can also be used to identify patients who are at high risk of developing certain diseases, such as cardiovascular disease or diabetes, allowing for early intervention and preventative measures.
Furthermore, AI is being used to develop personalized drug therapies. AI-powered drug discovery platforms can identify novel drug targets that are specific to certain patient populations, and AI algorithms can be used to design drugs that are tailored to these targets. This approach has the potential to revolutionize the development of new therapies for a wide range of diseases.
2.3 Drug Discovery
The traditional drug discovery process is time-consuming, expensive, and often unsuccessful. AI is transforming this process by accelerating the identification of potential drug candidates and reducing the cost of drug development. AI algorithms can analyze vast datasets of biological and chemical information to identify novel drug targets and predict the efficacy and safety of potential drugs.
AI is being used in various stages of the drug discovery process, including target identification, drug design, preclinical testing, and clinical trials. For example, AI algorithms can analyze genomic data to identify genes that are associated with certain diseases, which can then be used as targets for drug development. AI can also be used to design drugs that are more likely to bind to their targets and have fewer side effects. In preclinical testing, AI can be used to predict the toxicity of drugs and identify potential safety concerns. In clinical trials, AI can be used to optimize trial design, recruit patients, and analyze trial data [5].
Several companies are already using AI to develop new drugs. For example, BenevolentAI is using AI to identify potential drug candidates for various diseases, including amyotrophic lateral sclerosis (ALS) and Parkinson’s disease. Atomwise is using AI to screen millions of molecules for potential drug candidates. These companies are demonstrating the potential of AI to accelerate drug discovery and bring new therapies to market faster and more efficiently.
2.4 Administrative Tasks
AI can also be used to automate administrative tasks in healthcare, freeing up healthcare professionals to focus on patient care. For example, AI-powered chatbots can be used to answer patient questions, schedule appointments, and provide basic medical advice. AI can also be used to automate billing and coding processes, reducing the administrative burden on healthcare providers. Furthermore, AI can be used to improve supply chain management, optimizing inventory levels and reducing costs.
Natural language processing (NLP) techniques are particularly useful in automating administrative tasks. NLP can be used to extract information from medical records, process insurance claims, and generate reports. This can significantly improve the efficiency of healthcare operations and reduce administrative costs. Several hospitals and healthcare systems are already using AI to automate administrative tasks, and the adoption of these technologies is expected to increase in the coming years.
Many thanks to our sponsor Esdebe who helped us prepare this research report.
3. Ethical Considerations
The integration of AI into healthcare raises several ethical concerns that must be addressed to ensure responsible and equitable deployment. These concerns include data privacy, algorithmic bias, the impact on the role of healthcare professionals, and the need for transparency and accountability.
3.1 Data Privacy
AI algorithms require large amounts of data to train effectively. In healthcare, this data often includes sensitive patient information, such as medical history, genetic information, and lifestyle factors. Protecting the privacy of this data is crucial to maintaining patient trust and preventing potential harm.
Several regulations, such as the Health Insurance Portability and Accountability Act (HIPAA) in the United States and the General Data Protection Regulation (GDPR) in Europe, aim to protect patient data. However, these regulations may not be sufficient to address the unique challenges posed by AI. For example, AI algorithms can potentially infer sensitive information from seemingly anonymized data. Therefore, it is essential to develop new methods for protecting patient data in the age of AI. Techniques such as differential privacy and federated learning offer promising avenues for preserving privacy while still allowing AI algorithms to learn from data [6].
3.2 Algorithmic Bias
AI algorithms can perpetuate and even amplify existing biases in healthcare. If the data used to train an AI algorithm is biased, the algorithm will likely produce biased results. For example, if an AI algorithm is trained on data that primarily includes patients from one demographic group, it may not perform well on patients from other demographic groups. This can lead to disparities in healthcare outcomes.
Addressing algorithmic bias requires careful attention to data collection, algorithm design, and evaluation. It is essential to ensure that the data used to train AI algorithms is representative of the population it will be used to serve. Furthermore, algorithms should be designed to be fair and unbiased. This may involve using techniques such as fairness-aware machine learning, which aims to minimize bias in AI algorithms. Finally, AI algorithms should be rigorously evaluated to identify and mitigate any potential biases.
3.3 Impact on Healthcare Professionals
The integration of AI into healthcare may change the role of healthcare professionals. Some fear that AI will replace human doctors and nurses, leading to job losses. However, most experts believe that AI will augment, rather than replace, healthcare professionals. AI can automate routine tasks, freeing up healthcare professionals to focus on more complex and challenging cases. AI can also provide healthcare professionals with decision support, helping them to make more informed and accurate diagnoses and treatment decisions.
To ensure a smooth transition to an AI-augmented healthcare system, it is essential to provide healthcare professionals with the training and education they need to use AI effectively. This includes training on how to interpret AI outputs, how to identify potential biases, and how to use AI to improve patient care. It is also important to foster a culture of collaboration between humans and AI, where healthcare professionals and AI systems work together to provide the best possible care.
3.4 Transparency and Accountability
AI algorithms are often complex and opaque, making it difficult to understand how they arrive at their decisions. This lack of transparency can undermine trust in AI systems and make it difficult to hold them accountable for their actions. It is essential to develop methods for making AI algorithms more transparent and explainable. This includes techniques such as explainable AI (XAI), which aims to provide insights into how AI algorithms work and why they make certain decisions [7].
Furthermore, it is important to establish clear lines of accountability for AI systems. This includes determining who is responsible for the accuracy, safety, and fairness of AI algorithms. It is also important to develop mechanisms for addressing errors and biases in AI systems and for compensating patients who are harmed by AI. Developing clear ethical guidelines and regulatory frameworks is crucial for ensuring that AI is used responsibly and ethically in healthcare.
Many thanks to our sponsor Esdebe who helped us prepare this research report.
4. Legal and Regulatory Considerations
The legal and regulatory landscape for AI in healthcare is still evolving. Existing regulations, such as HIPAA and GDPR, provide some guidance, but they may not be sufficient to address the unique challenges posed by AI. New regulations and guidelines are needed to ensure that AI is used safely, effectively, and ethically in healthcare.
One of the key legal challenges is determining liability for errors and biases in AI systems. If an AI algorithm makes a mistake that harms a patient, who is responsible? Is it the developer of the algorithm, the healthcare provider who used the algorithm, or the hospital that deployed the algorithm? This is a complex legal question that will need to be addressed by courts and regulators.
Another legal challenge is protecting intellectual property in AI algorithms. AI algorithms can be very valuable, and companies that develop them want to protect their intellectual property. However, it can be difficult to patent AI algorithms, particularly those that are based on open-source software. This can create uncertainty and discourage innovation in the field.
Regulatory bodies, such as the Food and Drug Administration (FDA) in the United States and the European Medicines Agency (EMA) in Europe, are developing new frameworks for regulating AI in healthcare. These frameworks aim to ensure that AI systems are safe, effective, and reliable before they are deployed in clinical settings. They also aim to promote transparency and accountability in AI development and deployment.
The development of clear legal and regulatory frameworks is crucial for fostering responsible innovation in AI and ensuring that patients are protected from harm. These frameworks should balance the need to encourage innovation with the need to protect patient safety and privacy.
Many thanks to our sponsor Esdebe who helped us prepare this research report.
5. Future Directions
The future of AI in healthcare is bright. As AI technology continues to advance, we can expect to see even more innovative applications emerge. Some of the key areas of future development include:
- Explainable AI (XAI): Developing AI algorithms that are more transparent and explainable will be crucial for building trust and acceptance among healthcare professionals and patients. XAI techniques will allow users to understand how AI algorithms arrive at their decisions, making it easier to identify and correct errors and biases.
- Federated Learning: This approach allows AI algorithms to learn from data without requiring the data to be centralized. This is particularly important in healthcare, where patient data is often highly sensitive and cannot be easily shared. Federated learning can enable AI to learn from data across multiple healthcare organizations while preserving patient privacy.
- AI-powered Robotics: AI is being integrated into robotics to create surgical robots that are more precise and efficient than human surgeons. AI-powered robots can also be used to assist with other healthcare tasks, such as dispensing medications and delivering supplies.
- AI-driven Virtual Assistants: AI-powered virtual assistants can provide patients with personalized health advice, answer their questions, and help them manage their health conditions. These virtual assistants can be particularly helpful for patients with chronic diseases who require ongoing support.
- Integration with Wearable Devices: AI can be used to analyze data from wearable devices, such as smartwatches and fitness trackers, to provide patients with personalized health insights and recommendations. This can help patients to proactively manage their health and prevent disease.
These are just a few of the many exciting developments that are expected to shape the future of AI in healthcare. As AI technology continues to evolve, it has the potential to transform healthcare in profound ways, leading to improved patient outcomes, reduced costs, and a more efficient and equitable healthcare system. Addressing the ethical, legal, and regulatory challenges outlined in this report will be critical for realizing this potential.
Many thanks to our sponsor Esdebe who helped us prepare this research report.
6. Conclusion
AI holds immense potential to revolutionize healthcare, offering solutions to challenges in diagnostics, personalized treatment, drug discovery, and administrative efficiency. However, the successful and ethical integration of AI into healthcare requires careful consideration of several critical factors. Data privacy must be paramount, with robust safeguards in place to protect sensitive patient information. Algorithmic bias must be actively addressed to prevent disparities in healthcare outcomes. The role of healthcare professionals needs to be redefined, emphasizing collaboration between humans and AI. Transparency and accountability are essential for building trust in AI systems. Furthermore, clear legal and regulatory frameworks are needed to ensure responsible innovation and protect patient rights.
By addressing these challenges proactively, we can harness the transformative power of AI to create a healthcare system that is more efficient, equitable, and patient-centered. Future research should focus on developing XAI techniques, promoting federated learning, exploring AI-powered robotics, creating AI-driven virtual assistants, and integrating AI with wearable devices. Ultimately, a collaborative and ethical approach is essential to unlock the full potential of AI in healthcare and improve the lives of patients worldwide.
Many thanks to our sponsor Esdebe who helped us prepare this research report.
References
[1] Ardila, D., Kiraly, A. P., Bharadwaj, S., Choi, J., Reicher, J. J., Peng, L., … & Ko, J. (2019). End-to-end lung cancer screening with three-dimensional deep learning on low-dose chest computed tomography. Nature Medicine, 25(6), 954-961.
[2] Gulshan, V., Peng, L., Coram, M., Stumpe, M. C., Wu, D., Narayanaswamy, A., … & Webster, D. R. (2016). Development and validation of a deep learning algorithm for detection of diabetic retinopathy in retinal fundus photographs. Jama, 316(22), 2402-2410.
[3] Esteva, A., Kuprel, B., Novoa, R. A., Ko, J., Swani, S. M., Blau, H. M., … & Threlfall, C. J. (2017). Dermatologist-level classification of skin cancer with deep neural networks. Nature, 542(7639), 115-118.
[4] Madabhushi, A., & Lee, G. (2016). Image analysis and machine learning in digital pathology: Opportunities and challenges. Archives of Pathology & Laboratory Medicine, 140(11), 1102-1115.
[5] Paul, D., Sanap, G., Shenoy, S., Kalyane, D., Kalia, K., & Tekade, R. K. (2021). Artificial intelligence in drug discovery and development. Drug Discovery Today, 26(1), 80-93.
[6] Rieke, N., Hancox, J., Li, W., Milletari, F., Roth, H. R., Albarqouni, S., … & Bakas, S. (2020). Future of digital health with federated learning. NPJ Digital Medicine, 3(1), 1-7.
[7] Tjoa, E., & Guan, C. (2021). A survey on explainable AI (XAI): Toward medical explainable AI. IEEE Transactions on Neural Networks and Learning Systems, 32(11), 4794-4813.
The discussion on algorithmic bias is particularly crucial. What strategies can be implemented to ensure diverse datasets are used in training AI, and how can ongoing monitoring detect and correct bias in real-time applications?
That’s a great point! Ensuring diverse datasets is key. Beyond just variety, we need to actively curate data, audit for representation, and use techniques like adversarial training to build more robust models. Real-time bias detection is also crucial, and I think explainable AI will be vital here.
Editor: MedTechNews.Uk
Thank you to our Sponsor Esdebe