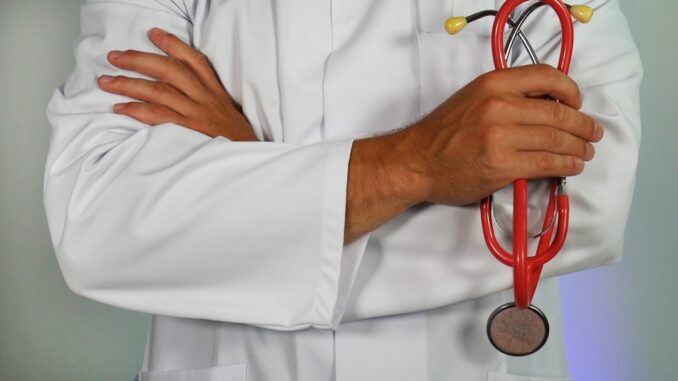
Beyond Binary: Reimagining Benchmarks for Artificial Intelligence in Healthcare
Abstract
Artificial intelligence (AI) is rapidly transforming healthcare, promising to enhance diagnostics, treatment, and patient care. However, the current suite of benchmarks used to evaluate AI systems in this domain often falls short of capturing the complexities of real-world clinical practice. This research report critically examines existing AI benchmarks in healthcare, highlighting their limitations in mirroring clinical scenarios, assessing essential clinical skills, and incorporating the nuanced perspectives of medical staff, particularly nurses. We explore the potential negative impacts of relying on insufficient benchmarks, including the risk of deploying AI systems that perform well in controlled environments but fail to deliver safe and effective care in dynamic clinical settings. Furthermore, we propose innovative methods and ideas for improving benchmarking techniques, emphasizing task-oriented evaluations, incorporating clinical reasoning assessments, and leveraging qualitative data from healthcare professionals. Ultimately, this report advocates for a more holistic and clinically relevant approach to AI benchmarking in healthcare, promoting the development and deployment of AI systems that truly benefit patients and support the healthcare workforce.
1. Introduction
The integration of Artificial Intelligence (AI) into healthcare holds immense potential to revolutionize various aspects of medical practice, from disease diagnosis and personalized treatment plans to drug discovery and operational efficiency [1]. The anticipated benefits include improved patient outcomes, reduced healthcare costs, and alleviation of the burden on healthcare professionals. However, realizing this potential hinges on the rigorous and appropriate evaluation of AI systems before their deployment in clinical settings. Benchmarks serve as crucial tools for this evaluation, providing standardized metrics to assess the performance, reliability, and safety of AI algorithms across a range of tasks [2].
Currently, many AI benchmarks in healthcare focus on narrow, well-defined tasks, such as image classification for radiology or natural language processing (NLP) for analyzing electronic health records (EHRs) [3]. While these benchmarks have contributed to significant advancements in specific AI capabilities, they often fail to adequately capture the complexities and nuances of real-world clinical scenarios. Existing benchmarks can also be biased, leading to the over- or under-estimation of AI performance in different populations, or clinical settings. This can have serious consequences, impacting patient care and exacerbating existing health disparities [4].
This research report delves into the shortcomings of current AI benchmarks in healthcare, emphasizing the need for a more holistic and clinically relevant approach. We argue that benchmarks must go beyond assessing technical accuracy and incorporate evaluations of clinical reasoning, communication skills, ethical considerations, and the ability to collaborate with human healthcare professionals. Furthermore, we highlight the importance of incorporating the perspectives and expertise of nurses and other medical staff, who play a crucial role in patient care and are often the primary users of AI systems in clinical settings.
2. Existing AI Benchmarks in Healthcare: A Critical Analysis
Numerous benchmarks have been developed to evaluate AI systems in healthcare, each focusing on specific tasks and modalities. A review of these benchmarks reveals several common limitations that warrant careful consideration.
2.1 Image-Based Benchmarks
Image-based benchmarks, particularly in radiology and pathology, are among the most widely used in healthcare AI evaluation. Datasets like ImageNet [5], while not specific to healthcare, have served as a foundation for pre-training models used in medical image analysis. Specialized datasets, such as the NIH Chest X-ray dataset [6] and the LIDC-IDRI lung nodule dataset [7], have been developed for specific diagnostic tasks.
- Limitations: These benchmarks often rely on curated datasets with well-defined labels, which may not accurately reflect the variability and complexity of real-world clinical images. The datasets often lack representation from diverse patient populations, potentially leading to biased performance. Moreover, these benchmarks primarily assess diagnostic accuracy, neglecting other crucial aspects of clinical image interpretation, such as the ability to explain findings and integrate them into a broader clinical context. Another issue is that some datasets may have been “poisoned” with information leakage, where information about the test set is inadvertently included in the training set.
2.2 Natural Language Processing (NLP) Benchmarks
NLP benchmarks evaluate AI systems’ ability to process and understand medical text, such as clinical notes, research articles, and patient-generated data. MIMIC-III [8] and i2b2 [9] are widely used datasets for tasks like named entity recognition, relation extraction, and clinical note summarization.
- Limitations: These benchmarks often focus on extracting specific information from text, without considering the overall meaning and context. They may struggle with ambiguous language, inconsistent terminology, and the diverse writing styles found in clinical documentation. Furthermore, the datasets may contain biases that reflect the demographics and practices of the institutions where the data were collected. The ability to understand and respond to complex medical questions, infer underlying patient conditions, and engage in meaningful conversations are areas that are not thoroughly evaluated by current NLP benchmarks.
2.3 Prediction and Decision-Making Benchmarks
Benchmarks in this category evaluate AI systems’ ability to predict patient outcomes, such as hospital readmission rates or risk of developing a particular disease, or to support clinical decision-making, such as recommending optimal treatment strategies. Examples include datasets for predicting sepsis onset [10] and for recommending medication dosages [11].
- Limitations: These benchmarks often rely on observational data, which may be subject to confounding factors and biases. They may also oversimplify the decision-making process, failing to account for the complex interplay of clinical factors, patient preferences, and ethical considerations. Furthermore, the benchmarks often assess performance based on historical data, which may not accurately reflect current clinical practices or emerging healthcare trends. This is especially true as treatments advance over time, making data from previous time periods obsolete for predictive modeling.
2.4 General Concerns Across Benchmarks
Beyond the specific limitations of each type of benchmark, several overarching concerns affect the validity and relevance of AI evaluation in healthcare:
- Lack of Clinical Realism: Many benchmarks rely on simplified or synthetic data that do not accurately represent the complexity and variability of real-world clinical scenarios. This can lead to AI systems that perform well in controlled environments but fail to generalize to the challenges of everyday clinical practice.
- Insufficient Consideration of Clinical Skills: Current benchmarks primarily focus on technical accuracy, neglecting other essential clinical skills such as communication, empathy, teamwork, and ethical reasoning. These skills are crucial for effective patient care and should be incorporated into AI evaluation.
- Limited Involvement of Healthcare Professionals: The development and evaluation of AI benchmarks often lack sufficient input from healthcare professionals, particularly nurses and other medical staff who are the primary users of AI systems in clinical settings. This can lead to benchmarks that are not aligned with clinical needs and priorities.
- Overfitting and Data Contamination: The intense competition to achieve high scores on benchmarks can incentivize researchers to overfit their models to the specific characteristics of the benchmark dataset, leading to poor performance on unseen data. Similarly, data contamination, where information from the test set inadvertently leaks into the training set, can artificially inflate performance scores.
- Neglect of Fairness and Bias: Many benchmarks fail to adequately address the potential for bias in AI systems, which can lead to disparities in patient care based on race, ethnicity, socioeconomic status, or other protected characteristics. It is crucial to develop benchmarks that explicitly assess and mitigate bias in AI algorithms.
3. Potential Impacts of Insufficient Benchmarking
The reliance on inadequate AI benchmarks in healthcare carries significant risks, with potentially detrimental impacts on patient care, healthcare costs, and the overall trust in AI technology. Some of the key consequences include:
- Deployment of Unsafe or Ineffective AI Systems: AI systems that perform well on insufficient benchmarks may fail to deliver safe and effective care in real-world clinical settings. This can lead to misdiagnoses, inappropriate treatments, and adverse patient outcomes. For example, an AI diagnostic tool that is trained on a limited dataset of medical images may fail to detect subtle abnormalities in images from patients with diverse backgrounds or comorbidities, leading to delayed or missed diagnoses.
- Increased Healthcare Costs: Ineffective AI systems can contribute to increased healthcare costs through unnecessary tests, treatments, and hospitalizations. Moreover, the cost of developing and deploying AI systems that ultimately fail to improve patient care represents a significant waste of resources. AI that generates incorrect alerts can also lead to alert fatigue which ultimately costs money through decreased efficiency.
- Erosion of Trust in AI Technology: When AI systems fail to meet expectations or produce inaccurate or biased results, it can erode trust among healthcare professionals and patients. This can hinder the adoption of AI technology in healthcare and limit its potential to improve patient care. If doctors begin to distrust AI systems then they may be less willing to follow its recommendations, even when the AI is correct.
- Exacerbation of Health Disparities: Biased AI systems can perpetuate and exacerbate existing health disparities, leading to unequal access to quality care for underserved populations. For example, an AI system that is trained on a dataset that is predominantly composed of data from white patients may perform poorly on patients from other racial or ethnic groups, leading to disparities in diagnosis and treatment. This can further contribute to mistrust of the healthcare system, which will ultimately impact health outcomes.
- Legal and Ethical Implications: The deployment of AI systems in healthcare raises complex legal and ethical questions, particularly regarding liability for errors and biases. Insufficient benchmarking can make it difficult to determine whether an AI system is performing appropriately and who is responsible when things go wrong. It can also raise concerns about patient privacy, data security, and the potential for AI to automate decisions in ways that violate ethical principles.
4. Improving Benchmarking Techniques: Towards a More Holistic Approach
To address the limitations of current AI benchmarks in healthcare and mitigate the risks associated with their use, a more holistic and clinically relevant approach is needed. This approach should encompass several key elements:
4.1 Task-Oriented Evaluations
Benchmarks should focus on evaluating AI systems’ performance on specific clinical tasks, rather than just assessing technical accuracy on isolated datasets. This requires a deeper understanding of the tasks that AI systems are intended to perform in clinical practice and the skills that are required to perform those tasks effectively.
For example, instead of just evaluating an AI system’s ability to classify medical images, a task-oriented benchmark might assess its ability to assist radiologists in making diagnostic decisions, including identifying relevant findings, generating differential diagnoses, and communicating those findings to other healthcare professionals and patients. This kind of task-oriented evaluation requires a multi-faceted approach.
4.2 Incorporation of Clinical Skills
Benchmarks should incorporate evaluations of clinical skills such as communication, empathy, teamwork, and ethical reasoning. This can be achieved through the use of simulated clinical scenarios, where AI systems are evaluated on their ability to interact with patients, collaborate with other healthcare professionals, and navigate ethical dilemmas. This will likely require a level of qualitative assessment.
For example, an AI system that is designed to assist nurses in providing patient education could be evaluated on its ability to communicate complex medical information in a clear and empathetic manner, as well as its ability to tailor its communication to the individual needs and preferences of the patient. These simulated clinical scenarios can be made to be more realistic by using realistic patient simulators.
4.3 Leveraging Qualitative Data
Qualitative data from healthcare professionals, particularly nurses and other medical staff, should be incorporated into the development and evaluation of AI benchmarks. This can be achieved through interviews, focus groups, and surveys, which can provide valuable insights into the challenges and opportunities associated with using AI systems in clinical practice.
For example, nurses can provide feedback on the usability and clinical relevance of AI-powered decision support tools, as well as identify potential biases or limitations that may not be apparent from quantitative data alone. Collecting this data is resource intensive, however there are ways to make it easier, such as the use of online surveys and focus groups.
4.4 Addressing Fairness and Bias
Benchmarks should explicitly assess and mitigate bias in AI systems. This requires the use of diverse datasets that accurately represent the patient populations that the AI system is intended to serve. It also requires the development of metrics that can detect and quantify bias in AI algorithms.
For example, an AI system that is used to predict hospital readmission rates should be evaluated on its performance across different racial and ethnic groups to ensure that it is not unfairly penalizing patients from certain groups. Fairness metrics such as disparate impact and equal opportunity can be used to assess and mitigate bias in AI systems.
4.5 Promoting Transparency and Explainability
Benchmarks should promote transparency and explainability in AI systems. This requires the development of methods for explaining how AI systems arrive at their conclusions, as well as methods for assessing the trustworthiness and reliability of AI systems. The need for explainability is especially important when considering AI in healthcare.
For example, an AI system that is used to diagnose cancer should be able to explain its reasoning in a way that is understandable to clinicians, allowing them to critically evaluate the AI’s conclusions and make informed decisions about patient care. Techniques such as attention maps and feature importance can be used to provide insights into the decision-making process of AI systems.
4.6 Dynamic and Adaptive Benchmarks
Benchmarks should be dynamic and adaptive, evolving to reflect changes in clinical practice, emerging healthcare trends, and advancements in AI technology. This requires the development of ongoing monitoring and evaluation mechanisms to ensure that benchmarks remain relevant and effective over time.
For example, as new treatments and diagnostic techniques emerge, benchmarks should be updated to reflect these changes and to assess AI systems’ ability to incorporate them into their decision-making processes. The use of continuous learning techniques can enable AI systems to adapt to changing clinical environments and maintain their performance over time.
4.7 Community-Driven Benchmark Development
The development of AI benchmarks in healthcare should be a community-driven effort, involving collaboration among researchers, clinicians, patients, and other stakeholders. This can help ensure that benchmarks are aligned with clinical needs and priorities, as well as promote transparency and trust in the evaluation process. All stakeholders should be included in the process in a fair and transparent manner.
For example, open-source benchmark datasets and evaluation tools can be developed and shared among researchers, allowing for greater collaboration and innovation. The creation of multi-disciplinary teams that include clinicians, data scientists, and ethicists can help ensure that benchmarks are developed in a responsible and ethical manner.
5. Conclusion
AI holds tremendous promise for transforming healthcare and improving patient outcomes. However, realizing this potential requires a rigorous and clinically relevant approach to AI benchmarking. Current benchmarks often fall short of capturing the complexities of real-world clinical practice, neglecting essential clinical skills, and failing to incorporate the perspectives of healthcare professionals. The reliance on insufficient benchmarks can lead to the deployment of unsafe or ineffective AI systems, increased healthcare costs, erosion of trust in AI technology, and exacerbation of health disparities.
To address these challenges, we must move beyond binary assessments of technical accuracy and embrace a more holistic approach to AI evaluation. This approach should encompass task-oriented evaluations, incorporation of clinical skills, leveraging qualitative data, addressing fairness and bias, promoting transparency and explainability, developing dynamic and adaptive benchmarks, and fostering community-driven benchmark development.
By reimagining AI benchmarks in healthcare, we can promote the development and deployment of AI systems that truly benefit patients, support the healthcare workforce, and contribute to a more equitable and sustainable healthcare system. The goal should be to develop AI systems that make the job of healthcare workers easier, and that improve patient outcomes for everyone.
References
[1] Jiang, F., Jiang, Y., Xiao, Y., Dong, Y., Li, S., Zhang, H., … & Wang, Y. (2017). Artificial intelligence in healthcare: past, present and future. Stroke and vascular neurology, 2(4), 230-243.
[2] Rajpurkar, P., Irvin, J., Ball, R. L., Zhu, K., Yang, B., Mehta, H., … & Ng, A. Y. (2017). CheXNet: Radiologist-level pneumonia detection on chest X-rays with deep learning. arXiv preprint arXiv:1711.05225.
[3] Beam, A. L., & Kohane, I. S. (2016). Big data and machine learning in health care. Jama, 316(11), 1149-1150.
[4] Obermeyer, Z., Powers, B., Vogeli, C., & Mullainathan, S. (2019). Dissecting racial bias in an algorithm used to manage the health of populations. Science, 366(6464), 447-453.
[5] Deng, J., Dong, W., Socher, R., Li, L. J., Li, K., & Fei-Fei, L. (2009). Imagenet: A large-scale hierarchical image database. In CVPR09.
[6] Wang, X., Peng, Y., Lu, L., Lu, Z., Summers, R. M. (2017). ChestX-ray8: Hospital-scale Chest X-ray Database and Benchmarks on Weakly-Supervised Classification and Localization of Common Thorax Diseases. CVPR 2017.
[7] Armato III, S. G., McLennan, G., Bidaut, L., McNitt-Gray, M. F., Meyer, C. R., Reeves, A. P., … & Clarke, L. P. (2011). The lung image database consortium (LIDC) and image database resource initiative (IDRI): a completed reference database for lung nodule research. Medical physics, 38(2), 915-931.
[8] Johnson, A. E., Pollard, T. J., Shen, L., Lehman, L. H., Feng, M., Ghassemi, M., … & Mark, R. G. (2016). MIMIC-III, a freely accessible critical care database. Scientific data, 3(1), 1-9.
[9] Uzuner, Ö., South, B. R., Shen, S., & Denny, J. C. (2011). 2010 i2b2/VA challenge on concepts, assertions, and relations in clinical text. Journal of the American Medical Informatics Association, 18(5), 552-556.
[10] Escobar, G. J., Turk, B. J., Ragins, A., Ha, Y., Chi, V., Lawson, A. B., … & Draper, D. (2020). Early prediction of sepsis in the emergency department using machine learning. Journal of hospital medicine, 15(2), 61-68.
[11] Sendak, M. J., Gao, M., Brajer, N., & Balu, S. (2020). Comparing machine learning to conventional statistical methods for clinical risk prediction. Annals of internal medicine, 172(10), 669-676.
The call for community-driven benchmark development is compelling. How can we ensure diverse patient representation in benchmark datasets to mitigate potential biases in AI healthcare applications?
That’s a vital point! Ensuring diverse patient representation is key. We could explore federated learning approaches, using data from various institutions without directly sharing patient records. Synthetic data generation, reflecting real-world diversity, could also supplement existing datasets. What are your thoughts on these options?
Editor: MedTechNews.Uk
Thank you to our Sponsor Esdebe
Reimagining benchmarks is a great idea! Task-oriented evaluations seem key. Imagine an AI learning bedside manner from “Grey’s Anatomy” reruns. Would it pass the empathy test, or just prescribe tequila and sage advice for every ailment?
Thanks for your comment! Task-oriented evaluations are definitely the way forward. An AI learning from ‘Grey’s Anatomy’ is a fun thought experiment! It highlights the challenge of teaching empathy and nuanced communication. How do we build benchmarks that measure those qualitative aspects effectively? #AI #Healthcare
Editor: MedTechNews.Uk
Thank you to our Sponsor Esdebe
So, AI bedside manner school? Forget Grey’s Anatomy, let’s get it binge-watching old episodes of “House.” Imagine the AI diagnosing lupus with a smirk. Now *that’s* a benchmark I’d like to see. Maybe we need a sarcasm detector in the algorithm!
Haha, the “House” AI is a hilarious (and maybe terrifying!) thought! A sarcasm detector is definitely needed. But you raise a crucial point: how do we teach AI to understand and respond appropriately to different communication styles and emotional cues? That’s the next frontier for benchmarks, for sure!
Editor: MedTechNews.Uk
Thank you to our Sponsor Esdebe