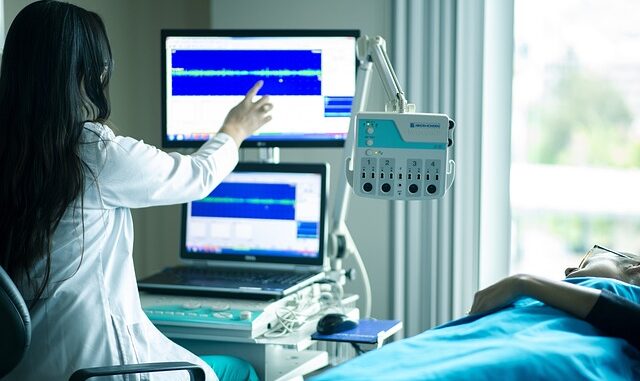
Abstract
Spirometry, a cornerstone of respiratory function testing for over a century, remains a vital tool in the diagnosis and management of pulmonary diseases. This report delves into the continued relevance of spirometry in modern respiratory medicine, moving beyond its fundamental principles to explore its evolving applications, integration with advanced diagnostic modalities, and the ongoing efforts to refine its accuracy and interpretability. We examine the factors influencing spirometric measurements, address the challenges in standardizing and interpreting results across diverse populations, and discuss the potential of artificial intelligence to enhance the diagnostic power of spirometry. Furthermore, we investigate the use of spirometry in monitoring disease progression and treatment response, highlighting its role in personalized respiratory care. This comprehensive analysis emphasizes that while newer technologies offer valuable insights, spirometry, when strategically employed and interpreted within a broader clinical context, continues to provide essential information for respiratory disease management.
Many thanks to our sponsor Esdebe who helped us prepare this research report.
1. Introduction
Spirometry, the measurement of lung volumes and airflow rates, has served as a fundamental diagnostic tool in respiratory medicine since its inception. While advanced imaging techniques like computed tomography (CT) and magnetic resonance imaging (MRI), alongside sophisticated physiological assessments such as diffusing capacity and fractional exhaled nitric oxide (FeNO) measurements, have expanded our ability to characterize respiratory disease, spirometry maintains a crucial position in clinical practice. Its accessibility, relatively low cost, and ease of administration make it a valuable tool for both initial screening and longitudinal monitoring. However, the interpretation of spirometric data is not always straightforward. Factors such as patient effort, technician expertise, and the applicability of reference equations to diverse populations can significantly impact the accuracy and reliability of results. Furthermore, spirometry provides a global assessment of lung function, lacking the spatial resolution offered by imaging techniques. Therefore, a nuanced understanding of spirometry’s strengths and limitations is essential for its effective integration into contemporary respiratory medicine. This report aims to provide a comprehensive exploration of spirometry, examining its continued relevance, the challenges in its interpretation, and the ongoing efforts to enhance its clinical utility.
Many thanks to our sponsor Esdebe who helped us prepare this research report.
2. Factors Influencing Spirometric Measurements
The accurate and reliable measurement of lung volumes and airflow rates during spirometry depends on a complex interplay of factors, broadly categorized as patient-related, equipment-related, and technician-related. Understanding these factors is crucial for minimizing variability and ensuring the validity of spirometric results.
2.1 Patient-Related Factors
- Effort and Cooperation: Spirometry is an effort-dependent test, requiring the patient to perform maximal inspiratory and expiratory maneuvers. Suboptimal effort, often due to pain, fatigue, or lack of understanding, can lead to underestimation of forced vital capacity (FVC) and forced expiratory volume in one second (FEV1). Strategies to improve patient effort include clear instructions, encouragement, and visual feedback.
- Age and Sex: Lung volumes and airflow rates naturally decline with age and differ significantly between sexes. Reference equations used to predict normal values must account for these factors to avoid misdiagnosis.
- Height and Weight: Taller individuals typically have larger lung volumes than shorter individuals. While weight is not directly incorporated into reference equations, obesity can affect chest wall compliance and respiratory mechanics, potentially impacting spirometric measurements.
- Ethnicity and Ancestry: Significant differences in lung size and function exist across different ethnic and ancestral groups. The use of inappropriate reference equations derived from populations with different body proportions can lead to systematic errors in interpretation, particularly in minority populations. Current research focuses on developing ethnicity-specific reference equations to address this limitation.
- Medical Conditions: Existing respiratory or cardiovascular conditions can obviously impact spirometric measurements. Similarly, non-respiratory conditions such as neuromuscular disorders can also affect a patient’s ability to perform the test properly.
- Medications: Certain medications, such as bronchodilators, can directly affect airflow rates and lung volumes, while others may indirectly influence spirometric measurements by altering respiratory drive or muscle strength.
2.2 Equipment-Related Factors
- Calibration: Regular calibration of spirometers is essential to ensure accurate volume and flow measurements. Calibration procedures should be performed according to manufacturer recommendations and documented meticulously.
- Temperature and Humidity: Ambient temperature and humidity can affect the density and viscosity of air, influencing flow measurements. Some spirometers incorporate correction factors to account for these environmental variations.
- Resistance and Dead Space: The resistance of the spirometer and the dead space within the mouthpiece can affect the maximal expiratory flow rates measured. Modern spirometers are designed to minimize these effects.
2.3 Technician-Related Factors
- Training and Experience: Proper training and experience are crucial for administering spirometry effectively and ensuring the quality of the test. Technicians should be proficient in instructing patients, monitoring their effort, and identifying potential sources of error.
- Adherence to Guidelines: Standardized guidelines for spirometry testing, such as those published by the American Thoracic Society (ATS) and the European Respiratory Society (ERS), should be followed meticulously to ensure consistency and reproducibility.
- Quality Control: Rigorous quality control procedures, including visual inspection of flow-volume loops and volume-time curves, are essential for identifying technically unacceptable tests. Technicians should be trained to recognize and correct common errors, such as false starts, premature terminations, and coughs during the maneuver.
Many thanks to our sponsor Esdebe who helped us prepare this research report.
3. Challenges in Standardizing and Interpreting Spirometric Results
Despite the widespread use of spirometry, significant challenges remain in standardizing and interpreting results, particularly across diverse populations and in the context of complex respiratory diseases.
3.1 Reference Equations and Ethnic Variability
The selection of appropriate reference equations is a critical step in interpreting spirometric data. Traditionally, reference equations derived from predominantly Caucasian populations have been used universally. However, studies have demonstrated that these equations systematically overestimate lung function in individuals of African, Asian, and Hispanic ancestry. This can lead to underdiagnosis of obstructive lung diseases in these populations. The development and implementation of ethnicity-specific reference equations are crucial for reducing diagnostic bias and improving the accuracy of spirometry in diverse populations. Several approaches have been proposed, including the use of correction factors applied to existing equations and the development of entirely new equations based on local populations. However, the optimal strategy remains a subject of ongoing debate.
3.2 Defining the Lower Limit of Normal (LLN)
The lower limit of normal (LLN) is a statistical boundary used to define abnormal lung function. Traditionally, the LLN has been defined as a fixed percentage (e.g., 80%) of the predicted value. However, this approach can lead to both false-positive and false-negative diagnoses, particularly in individuals with values close to the LLN. A more accurate approach is to use the fifth percentile of the predicted value, which accounts for the inherent variability in lung function within a healthy population. This approach is now recommended by the ATS/ERS guidelines. However, even with the use of the fifth percentile, defining the LLN remains challenging, particularly in individuals with borderline results.
3.3 Interpreting Discordant Spirometric Patterns
In some cases, spirometric results may exhibit discordant patterns, such as a reduced FEV1 with a normal FVC. These patterns can be challenging to interpret and may indicate specific types of lung disease. For example, a disproportionately reduced FEV1 may suggest early airflow obstruction, while a normal FVC may indicate restrictive physiology without significant lung volume reduction. Consideration of the clinical context, including symptoms, medical history, and other diagnostic tests, is essential for accurate interpretation of discordant spirometric patterns. Furthermore, serial spirometry measurements can help to differentiate between transient and persistent abnormalities.
3.4 Accounting for Individual Variability
Spirometric reference equations provide population-based estimates of normal lung function. However, significant individual variability exists within any population. Factors such as genetic predisposition, environmental exposures, and individual lifestyle choices can influence lung function independently of age, sex, height, and ethnicity. Therefore, interpreting spirometric results solely based on reference equations can be misleading. Comparing an individual’s spirometric results to their previous measurements, if available, can provide valuable information about changes in lung function over time, independent of population norms. This approach is particularly useful for monitoring disease progression and treatment response.
Many thanks to our sponsor Esdebe who helped us prepare this research report.
4. Integration of Spirometry with Advanced Diagnostic Modalities
While spirometry remains a valuable tool for assessing lung function, it provides a global assessment that lacks the spatial resolution of advanced imaging techniques such as CT and MRI. Integrating spirometry with these modalities can provide a more comprehensive understanding of respiratory disease.
4.1 Spirometry and CT Imaging
CT imaging can provide detailed information about the structure of the lungs, including the presence of emphysema, fibrosis, and airway abnormalities. Combining spirometry with CT imaging can help to correlate physiological abnormalities with specific anatomical features. For example, in patients with chronic obstructive pulmonary disease (COPD), the severity of airflow obstruction on spirometry can be correlated with the extent of emphysema on CT. This information can be used to guide treatment decisions and predict disease progression. Furthermore, quantitative CT analysis, which involves measuring lung density and volume, can provide more precise and objective assessments of lung disease than visual interpretation alone.
4.2 Spirometry and MRI
MRI offers several advantages over CT imaging, including the absence of ionizing radiation and the ability to assess lung ventilation and perfusion. Combining spirometry with MRI can provide insights into the dynamic function of the lungs. For example, ventilation-perfusion MRI can identify areas of the lung that are poorly ventilated or poorly perfused, which can be useful in diagnosing and managing pulmonary embolism and other respiratory diseases. Furthermore, MRI can be used to assess lung mechanics and airway responsiveness, providing information that is not readily available from spirometry alone.
4.3 Spirometry and other Physiological Measurements
Spirometry is often complemented by other physiological measurements, such as diffusing capacity (DLCO) and fractional exhaled nitric oxide (FeNO). DLCO measures the ability of the lungs to transfer gas from the alveoli to the blood, while FeNO measures the amount of nitric oxide in exhaled air, which can be elevated in patients with asthma. Combining spirometry with DLCO and FeNO can help to differentiate between obstructive and restrictive lung diseases, and to identify specific subtypes of asthma. For example, a patient with airflow obstruction on spirometry, a reduced DLCO, and a normal FeNO may have emphysema, while a patient with airflow obstruction, a normal DLCO, and an elevated FeNO may have allergic asthma. Furthermore, impulse oscillometry (IOS) provides additional insight into airway resistance and reactance, offering complementary information to spirometry, especially in patients where effort-dependent maneuvers are difficult.
Many thanks to our sponsor Esdebe who helped us prepare this research report.
5. The Role of Artificial Intelligence in Enhancing Spirometry
Artificial intelligence (AI) is rapidly transforming many areas of medicine, and spirometry is no exception. AI algorithms can be used to automate various aspects of spirometry, from quality control to interpretation, potentially improving the accuracy and efficiency of the test.
5.1 Automated Quality Control
AI algorithms can be trained to identify technically unacceptable spirometric maneuvers based on visual inspection of flow-volume loops and volume-time curves. These algorithms can automatically flag tests that do not meet quality criteria, reducing the need for manual review by technicians. This can save time and improve the consistency of quality control.
5.2 Improved Interpretation of Spirometric Data
AI algorithms can be used to analyze spirometric data and identify patterns that may be missed by human interpreters. These algorithms can be trained on large datasets of spirometric measurements and clinical data to predict the likelihood of specific respiratory diseases. This can help to improve the accuracy of diagnosis and guide treatment decisions. Furthermore, AI algorithms can be used to personalize spirometric interpretation by accounting for individual variability and risk factors.
5.3 Personalized Prediction of Disease Progression
AI algorithms can be used to predict disease progression based on spirometric measurements and other clinical data. These algorithms can identify patients who are at high risk of developing complications, such as exacerbations or hospitalization. This can allow for more targeted interventions to prevent disease progression and improve patient outcomes. For example, machine learning models can predict COPD exacerbations based on spirometric trends, environmental factors, and patient-reported symptoms.
5.4 Challenges and Future Directions
While AI holds great promise for enhancing spirometry, several challenges remain. One challenge is the need for large, high-quality datasets to train AI algorithms. Another challenge is the potential for bias in AI algorithms if they are trained on data that are not representative of the population. Furthermore, it is important to ensure that AI algorithms are transparent and explainable, so that clinicians can understand how they arrive at their conclusions. Future research should focus on developing AI algorithms that are robust, unbiased, and explainable, and on evaluating their clinical utility in real-world settings.
Many thanks to our sponsor Esdebe who helped us prepare this research report.
6. Spirometry in Monitoring Disease Progression and Treatment Response
Spirometry plays a critical role in monitoring disease progression and treatment response in a variety of respiratory diseases, including COPD, asthma, and interstitial lung disease (ILD).
6.1 COPD
In COPD, spirometry is used to assess the severity of airflow obstruction and to monitor changes in lung function over time. The Global Initiative for Chronic Obstructive Lung Disease (GOLD) guidelines recommend using FEV1 to classify COPD severity. Serial spirometry measurements can help to identify patients who are experiencing a decline in lung function, which may indicate disease progression or inadequate treatment. Spirometry is also used to assess the response to bronchodilator therapy and to monitor for the development of complications, such as pulmonary hypertension.
6.2 Asthma
In asthma, spirometry is used to assess airflow obstruction and airway reversibility. A significant improvement in FEV1 after bronchodilator administration is a hallmark of asthma. Serial spirometry measurements can help to monitor asthma control and to assess the response to inhaled corticosteroids and other medications. Spirometry is also used to identify patients with severe asthma who may benefit from biologic therapies. However, it is important to note that spirometry may be normal in patients with well-controlled asthma.
6.3 Interstitial Lung Disease (ILD)
In ILD, spirometry is used to assess the extent of restrictive lung disease and to monitor changes in lung function over time. A decline in FVC is a common finding in patients with progressive ILD. Serial spirometry measurements can help to identify patients who are responding to antifibrotic therapies and to monitor for the development of pulmonary hypertension. However, it is important to note that spirometry may not be sensitive enough to detect early changes in lung function in some patients with ILD.
6.4 Considerations for Longitudinal Monitoring
When using spirometry for longitudinal monitoring, it is important to minimize variability and ensure consistency. Spirometry should be performed using the same equipment and techniques each time. Patients should be instructed to perform maximal effort maneuvers, and technicians should provide encouragement and feedback. Furthermore, it is important to consider the clinical context when interpreting changes in spirometric measurements. Factors such as exacerbations, infections, and changes in medication can all affect lung function.
Many thanks to our sponsor Esdebe who helped us prepare this research report.
7. Conclusion
Spirometry remains a valuable tool in modern respiratory medicine, providing essential information for diagnosis, monitoring, and treatment of respiratory diseases. While advanced imaging techniques and other physiological measurements offer valuable insights, spirometry’s accessibility, low cost, and ease of administration make it a crucial component of respiratory care. However, the accurate interpretation of spirometric data requires a thorough understanding of the factors that can influence measurements, the challenges in standardizing and interpreting results across diverse populations, and the potential of AI to enhance the diagnostic power of spirometry. By integrating spirometry with other diagnostic modalities and by continuously refining its accuracy and interpretability, we can ensure that it continues to play a vital role in improving the lives of patients with respiratory diseases.
Many thanks to our sponsor Esdebe who helped us prepare this research report.
References
- American Thoracic Society/European Respiratory Society. (2005). Standardization of spirometry. American Journal of Respiratory and Critical Care Medicine, 172(1), 1-12.
- Miller, M. R., Hankinson, J., Brusasco, V., Burgos, F., Casaburi, R., Coates, A., … & Wanger, J. (2005). Standardisation of spirometry. European Respiratory Journal, 26(2), 319-338.
- Quanjer, P. H., Stanojevic, S., Cole, T. J., Baur, X., Hall, G. L., Culver, B. H., … & Stocks, J. (2012). Multi-ethnic reference values for spirometry for the 3-95-yr age range: the global lung function initiative. European Respiratory Journal, 40(6), 1324-1343.
- Culver, B. H., Graham, B. L., Coates, A. L., Wanger, J., Berry, C. E., Clarke, J. R., … & Enright, P. L. (2017). Recommendations for a standardized pulmonary function report. An Official American Thoracic Society Technical Statement. American Journal of Respiratory and Critical Care Medicine, 196(11), 1463-1472.
- Hall, G. L., Bradtke, K. J., Burgel, P. R., Cooper, B. G., Culver, B. H., Davis, K. J., … & Stocks, J. (2023). Global Lung Function Initiative 2022 reference equations for spirometry. European Respiratory Journal, 61(5).
- van der Lee, I., Zanen, P., Wouters, E. F. M., & Postma, D. S. (2006). Detection of airway obstruction in asthma and COPD with impulse oscillometry. European Respiratory Journal, 27(4), 702-707.
- Ferreira, A. M., Melo, N., & Gomes, E. (2021). Machine Learning in Chronic Obstructive Pulmonary Disease (COPD): A Systematic Review. Journal of Personalized Medicine, 11(10), 1029.
- Saha, S., Storey, K. B., Burns, M. J., Navaratnam, P., McKeough, Z. J., & Ward, M. P. (2023). The value of machine learning in predicting COPD exacerbations: A systematic review. Respiratory Medicine, 218, 107392.
- Raghavan, D., Vittinghoff, E., Koth, L. L., Putcha, N., Kalhan, R., Woodruff, P. G., … & Investigators, S. C. T. (2015). Longitudinal decline in FEV1 in early chronic obstructive pulmonary disease. American Journal of Respiratory and Critical Care Medicine, 191(5), 523-531.
- Global Initiative for Chronic Obstructive Lung Disease (GOLD). (2024). Global strategy for the diagnosis, management, and prevention of chronic obstructive pulmonary disease. Retrieved from https://goldcopd.org/
- Global Initiative for Asthma (GINA). (2023). Global strategy for asthma management and prevention. Retrieved from https://ginasthma.org/
- du Bois, R. M., Albera, C., Bradford, W. Z., Costabel, U., Flaherty, K. R., Noble, P. W., … & Tasko, S. M. (2011). Pirfenidone in patients with idiopathic pulmonary fibrosis (CAPACITY): two randomised trials. The Lancet, 377(9779), 1760-1769.
The integration of AI for automated quality control in spirometry is a promising avenue. Has anyone explored using federated learning to train these AI models across diverse datasets while preserving patient privacy? This approach could address bias and improve generalizability.
That’s a great point about federated learning! It’s definitely an area worth exploring further. The challenge is securing access to diverse datasets while adhering to privacy regulations. Has anyone had experience navigating the regulatory landscape for federated learning in healthcare? I’d love to hear about it.
Editor: MedTechNews.Uk
Thank you to our Sponsor Esdebe
Fascinating report! Who knew spirometry had so much going on under the hood? Makes you wonder if one day we’ll need AI to interpret the AI that’s interpreting the spirometry results! Thanks for sharing.
Thanks so much for your comment! That’s a fun thought about AI interpreting AI. It really highlights how quickly things are evolving. The potential for AI to refine diagnostics is huge, but keeping it understandable and trustworthy will be key as we move forward. Appreciate you taking the time to read!
Editor: MedTechNews.Uk
Thank you to our Sponsor Esdebe