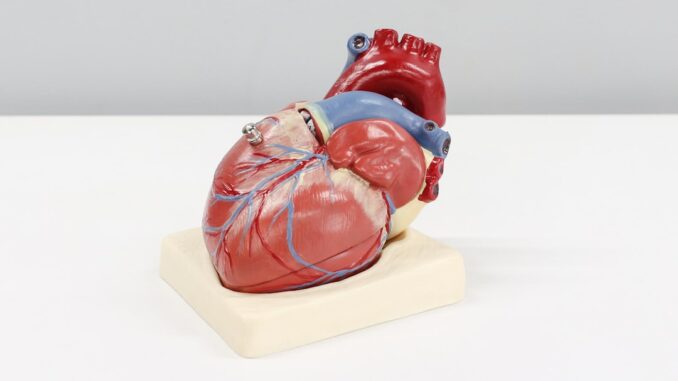
Abstract
Electrocardiography (ECG) has remained a cornerstone of cardiovascular diagnostics for over a century. While traditional ECG interpretation relies on identifying specific morphological features and rhythm disturbances, its diagnostic sensitivity and specificity, particularly for complex or subtle conditions, can be limited. This report explores the evolution of ECG technology, from its fundamental principles to cutting-edge advancements, focusing on the integration of ECG data with multi-omics approaches to provide a more comprehensive and personalized assessment of cardiovascular risk. The core technology discussed is the application of machine learning models, specifically ECG-LM, for enhanced ECG analysis. We delve into the potential of combining ECG features with genomic, proteomic, metabolomic, and imaging data to overcome the limitations of conventional ECG interpretation and to unlock new avenues for early detection, personalized treatment, and improved patient outcomes. Finally, we discuss the challenges associated with data integration, computational complexity, and clinical translation, highlighting the need for robust validation studies and standardized data formats.
Many thanks to our sponsor Esdebe who helped us prepare this research report.
1. Introduction
The electrocardiogram (ECG) is a non-invasive diagnostic tool that records the electrical activity of the heart over time, providing valuable insights into cardiac function and rhythm. Since its inception by Willem Einthoven in the early 20th century, the ECG has become an indispensable part of clinical cardiology, used for the detection, diagnosis, and management of a wide range of cardiovascular disorders, including arrhythmias, myocardial ischemia, and structural heart disease [1]. Traditional ECG interpretation relies on identifying specific morphological features within the ECG waveform, such as the P wave, QRS complex, and T wave, and analyzing their durations, amplitudes, and relationships to one another. However, despite its widespread use and established clinical utility, traditional ECG interpretation has limitations, particularly in patients with complex or subtle cardiac conditions.
The limitations of conventional ECG analysis stem from several factors, including inter-observer variability in interpretation, low sensitivity for certain conditions (e.g., early-stage ischemia, subtle cardiomyopathies), and its inability to capture the dynamic nature of cardiovascular disease. Furthermore, the ECG provides a snapshot of cardiac electrical activity at a specific point in time, and may not reflect the underlying pathophysiology of the disease process. The complexity of cardiovascular disease necessitates a holistic approach that integrates multiple layers of information, including genetic predispositions, protein expression patterns, metabolic profiles, and imaging data. Therefore, there is a growing interest in integrating ECG data with other -omics data to develop more comprehensive and personalized approaches to cardiovascular risk assessment and management.
This report aims to provide a comprehensive overview of the evolution of ECG technology, from its fundamental principles to cutting-edge advancements, with a focus on the integration of ECG data with multi-omics approaches. We will explore the underlying physiological principles of ECG, how ECGs are performed and interpreted, the different types of abnormalities detectable via ECG, the limitations of traditional ECG interpretation, recent advancements in ECG technology and analysis, and the potential of combining ECG features with genomic, proteomic, metabolomic, and imaging data. We will also discuss the challenges associated with data integration, computational complexity, and clinical translation, highlighting the need for robust validation studies and standardized data formats.
Many thanks to our sponsor Esdebe who helped us prepare this research report.
2. Physiological Basis of the Electrocardiogram
The ECG reflects the sum of electrical activity generated by the heart’s individual myocardial cells. The cardiac cycle involves a complex interplay of electrical and mechanical events, beginning with the generation of an action potential in the sinoatrial (SA) node, the heart’s natural pacemaker. This electrical impulse spreads throughout the atria, causing atrial depolarization and contraction, represented by the P wave on the ECG [2]. The electrical impulse then travels to the atrioventricular (AV) node, where it is briefly delayed before being conducted down the bundle of His and its branches to the ventricles. This delay allows for atrial contraction to complete before ventricular contraction begins.
Ventricular depolarization, which is the process of electrical activation of the ventricles, is represented by the QRS complex on the ECG. The morphology of the QRS complex is determined by the sequence of ventricular activation, which begins with the septum and then spreads to the free walls of the ventricles. The T wave represents ventricular repolarization, which is the process of restoring the ventricular cells to their resting electrical state. The ST segment, which lies between the QRS complex and the T wave, represents the period of time when the ventricles are fully depolarized. Abnormalities in the ST segment, such as ST-segment elevation or depression, can indicate myocardial ischemia or injury [3].
The ECG is a valuable tool for assessing the heart’s rhythm, conduction, and morphology. Abnormalities in the ECG waveform can indicate a wide range of cardiovascular disorders, including arrhythmias, myocardial ischemia, hypertrophy, and conduction abnormalities. However, the ECG is not a perfect diagnostic tool, and its sensitivity and specificity can be limited by several factors, including inter-observer variability in interpretation, patient-specific factors, and the dynamic nature of cardiovascular disease.
Many thanks to our sponsor Esdebe who helped us prepare this research report.
3. ECG Acquisition and Interpretation
3.1. ECG Acquisition Techniques
Electrocardiography is a non-invasive procedure that involves placing electrodes on the patient’s skin to record the electrical activity of the heart. The standard 12-lead ECG uses ten electrodes placed on the limbs and chest, providing 12 different views of the heart’s electrical activity [4]. The placement of the electrodes is critical to ensure accurate and reproducible ECG recordings.
The ECG signal is typically amplified and filtered to reduce noise and artifacts. Modern ECG machines also incorporate digital signal processing techniques to further improve the quality of the ECG signal. The ECG recording is displayed on a monitor or printed on paper, and the ECG waveform is analyzed by a trained healthcare professional.
Other ECG acquisition techniques include Holter monitoring, which involves continuous ECG recording over 24-48 hours, and event monitoring, which involves recording ECG data only when the patient experiences symptoms. These techniques are useful for detecting intermittent arrhythmias or other transient cardiac events [5].
3.2. ECG Interpretation Principles
ECG interpretation involves a systematic analysis of the ECG waveform, including the P wave, QRS complex, T wave, and ST segment. The interpreter evaluates the rate, rhythm, axis, and morphology of the ECG, looking for abnormalities that may indicate underlying cardiac disease. It requires a deep understanding of cardiac electrophysiology and clinical correlation.
- Rate: The heart rate is determined by measuring the time interval between consecutive QRS complexes. Normal heart rate is typically between 60 and 100 beats per minute.
- Rhythm: The heart rhythm is determined by analyzing the regularity of the QRS complexes and the relationship between the P waves and QRS complexes. Common rhythm disturbances include sinus arrhythmias, atrial fibrillation, atrial flutter, and ventricular tachycardia.
- Axis: The cardiac axis is the average direction of the electrical activity of the ventricles. Abnormalities in the cardiac axis can indicate ventricular hypertrophy or conduction abnormalities.
- Morphology: The morphology of the ECG waveform is analyzed to identify abnormalities such as Q waves, ST-segment elevation or depression, T-wave inversion, and prolonged QT interval. These abnormalities can indicate myocardial infarction, ischemia, hypertrophy, or electrolyte imbalances [6].
ECG interpretation requires expertise and experience, and it is often performed by cardiologists or trained electrophysiologists. Computer-aided ECG interpretation systems are also available, but these systems should be used with caution and always be reviewed by a qualified healthcare professional.
Many thanks to our sponsor Esdebe who helped us prepare this research report.
4. Limitations of Traditional ECG Interpretation
Despite its clinical utility, traditional ECG interpretation has limitations, particularly in the context of complex and subtle cardiovascular conditions. These limitations include:
- Inter-observer variability: ECG interpretation is subjective, and different interpreters may have different opinions about the presence or absence of certain abnormalities. This variability can lead to inconsistent diagnoses and treatment decisions [7].
- Low sensitivity for certain conditions: The ECG may not be sensitive enough to detect early-stage ischemia, subtle cardiomyopathies, or other conditions that do not cause significant changes in the ECG waveform. A normal ECG does not rule out cardiac disease.
- Inability to capture the dynamic nature of cardiovascular disease: The ECG provides a snapshot of cardiac electrical activity at a specific point in time and may not reflect the underlying pathophysiology of the disease process. For example, transient ischemia may not be detected on a resting ECG. The limited duration of a standard ECG recording further hinders the detection of paroxysmal events.
- Dependence on specific morphological features: Traditional ECG interpretation relies on identifying specific morphological features within the ECG waveform. However, these features can be subtle or atypical, making them difficult to detect and interpret. Furthermore, the reliance on these predefined patterns may limit the ability to identify novel or unexpected patterns of cardiac electrical activity.
- Limited ability to predict future events: While the ECG can provide valuable information about current cardiac function, its ability to predict future cardiovascular events is limited. Risk stratification often requires additional tests and clinical information [8].
These limitations highlight the need for more advanced ECG analysis techniques and the integration of ECG data with other clinical and -omics data to improve diagnostic accuracy, personalize treatment, and predict future cardiovascular events.
Many thanks to our sponsor Esdebe who helped us prepare this research report.
5. Advancements in ECG Technology and Analysis
Recent advancements in ECG technology and analysis have aimed to address the limitations of traditional ECG interpretation and improve the diagnostic and prognostic value of the ECG. These advancements include:
- High-resolution ECG: High-resolution ECG, also known as signal-averaged ECG, uses advanced signal processing techniques to reduce noise and artifacts and to enhance the detection of subtle ECG abnormalities, such as late potentials. Late potentials are low-amplitude, high-frequency signals that occur at the end of the QRS complex and are associated with increased risk of ventricular arrhythmias [9].
- Body surface mapping: Body surface mapping involves recording ECG data from multiple electrodes placed on the chest and back, providing a more comprehensive view of the heart’s electrical activity. This technique can be used to identify the location of arrhythmias and to guide catheter ablation procedures [10].
- Vectorcardiography (VCG): Vectorcardiography represents the electrical activity of the heart as a series of vectors in three-dimensional space. VCG can provide additional information about the heart’s electrical activity that is not readily apparent on a standard 12-lead ECG. However, it is not routinely used in clinical practice due to its complexity and lack of widespread availability.
- Wearable ECG devices: Wearable ECG devices, such as smartwatches and chest straps, allow for continuous ECG monitoring in ambulatory settings. These devices can detect arrhythmias and other cardiac events that may not be detected on a standard ECG. However, the accuracy and reliability of wearable ECG devices can vary, and their use should be carefully considered [11].
- Artificial intelligence (AI) and machine learning (ML): AI and ML algorithms are increasingly being used to analyze ECG data and to improve diagnostic accuracy and risk stratification. These algorithms can be trained to identify specific ECG patterns associated with various cardiovascular disorders, and they can also be used to predict future cardiovascular events. The ECG-LM model is one such example, leveraging language models to analyze ECG waveforms as a sequential signal. While the application of ECG-LM is novel, the technology needs further study.
Many thanks to our sponsor Esdebe who helped us prepare this research report.
6. Integrating ECG with Multi-Omics for Holistic Cardiovascular Risk Stratification
The integration of ECG data with multi-omics data holds great promise for improving cardiovascular risk stratification, personalized treatment, and patient outcomes. Multi-omics approaches involve the simultaneous analysis of multiple layers of biological information, including genomics, proteomics, metabolomics, and imaging data [12]. By combining ECG features with multi-omics data, it is possible to gain a more comprehensive understanding of the underlying pathophysiology of cardiovascular disease and to identify novel biomarkers for early detection and risk prediction.
6.1. ECG and Genomics
Genomic studies have identified numerous genetic variants associated with increased risk of cardiovascular disease. Integrating ECG data with genomic data can help to identify individuals who are at high risk of developing specific cardiac conditions and to tailor treatment strategies accordingly. For example, genetic variants associated with prolonged QT interval can increase the risk of sudden cardiac death, and individuals with these variants may benefit from prophylactic treatment with beta-blockers or implantable cardioverter-defibrillators (ICDs) [13].
6.2. ECG and Proteomics
Proteomics involves the study of proteins and their functions. Integrating ECG data with proteomic data can help to identify novel protein biomarkers for early detection and risk prediction of cardiovascular disease. For example, elevated levels of cardiac troponin are indicative of myocardial damage, and integrating troponin levels with ECG findings can improve the accuracy of diagnosing acute myocardial infarction [14].
6.3. ECG and Metabolomics
Metabolomics involves the study of small molecules (metabolites) in biological samples. Integrating ECG data with metabolomic data can help to identify metabolic pathways that are altered in cardiovascular disease and to develop novel therapeutic strategies. For example, alterations in glucose metabolism have been linked to increased risk of heart failure, and targeting these pathways with drugs or lifestyle interventions may improve outcomes [15].
6.4. ECG and Imaging Data
Imaging modalities such as echocardiography, cardiac magnetic resonance imaging (MRI), and computed tomography (CT) can provide detailed information about the structure and function of the heart. Integrating ECG data with imaging data can help to improve diagnostic accuracy and risk stratification. For example, echocardiography can be used to assess left ventricular function, and integrating echocardiographic findings with ECG findings can improve the prediction of heart failure events [16].
Many thanks to our sponsor Esdebe who helped us prepare this research report.
7. Challenges and Future Directions
While the integration of ECG data with multi-omics data holds great promise, there are several challenges that need to be addressed before this approach can be widely implemented in clinical practice. These challenges include:
- Data integration: Integrating data from multiple sources can be challenging due to differences in data formats, data quality, and data availability. Standardized data formats and data sharing platforms are needed to facilitate data integration and analysis.
- Computational complexity: Analyzing large and complex datasets requires significant computational resources and expertise in bioinformatics and data science. Cloud-based computing platforms and user-friendly data analysis tools can help to overcome these challenges.
- Clinical validation: The clinical utility of multi-omics approaches needs to be rigorously validated in large, prospective clinical trials. These trials should assess the impact of multi-omics-guided treatment strategies on patient outcomes and cost-effectiveness.
- Ethical considerations: The use of multi-omics data raises ethical considerations related to data privacy, data security, and the potential for discrimination based on genetic or other information. Robust ethical frameworks and regulatory guidelines are needed to ensure the responsible use of multi-omics data.
Future directions in this field include the development of more sophisticated AI and ML algorithms for ECG analysis, the integration of wearable sensor data with multi-omics data, and the development of personalized treatment strategies based on individual risk profiles. Furthermore, larger and more diverse datasets are needed to train and validate AI algorithms and to ensure that they are applicable to a wide range of patient populations. The development of point-of-care multi-omics testing platforms could also facilitate the widespread adoption of this approach in clinical practice.
Many thanks to our sponsor Esdebe who helped us prepare this research report.
8. Conclusion
Electrocardiography remains a fundamental tool in cardiovascular diagnostics, but its limitations in sensitivity and specificity, particularly in complex cases, necessitate advancements in its application. Integrating ECG data with multi-omics approaches offers a powerful strategy to overcome these limitations, enabling a more comprehensive and personalized assessment of cardiovascular risk. While challenges related to data integration, computational complexity, clinical validation, and ethical considerations exist, the potential benefits of this approach are significant. Future research should focus on developing standardized data formats, robust analytical tools, and large-scale validation studies to translate these advancements into improved clinical outcomes for patients with cardiovascular disease.
Many thanks to our sponsor Esdebe who helped us prepare this research report.
References
[1] Goldberger, A. L. (2007). Clinical electrocardiography: a simplified approach. Mosby Elsevier.
[2] Fuster, V., Walsh, R. A., & Harrington, R. A. (2011). Hurst’s The Heart. McGraw-Hill Medical.
[3] Wagner, G. S. (2001). Marriott’s Practical Electrocardiography. Lippincott Williams & Wilkins.
[4] Mirvis, D. M. (2016). Electrocardiography: A Physiologic Approach. Springer.
[5] Zimetbaum, P. J., & Josephson, M. E. (2003). The evolving role of ambulatory electrocardiographic monitoring. Annals of Internal Medicine, 130(1), 848-856.
[6] Surawicz, B., Knilans, T. K., & Chou, T. C. (2008). Chou’s electrocardiography in clinical practice: adult and pediatric. Saunders Elsevier.
[7] Batchvarov, V. N., Malik, M., Camm, A. J., Task Force of the European Society of Cardiology. (1998). Measurement variability in electrocardiography. Journal of the American College of Cardiology, 31(7), 1467-1472.
[8] Greenland, P., Alpert, J. S., Beller, G. A., Benjamin, E. J., Brill-Edwards, P., Champion, R. W., … & Gibbons, R. J. (2010). 2010 ACCF/AHA guideline for assessment of cardiovascular risk in asymptomatic adults: a report of the American College of Cardiology Foundation/American Heart Association Task Force on Practice Guidelines. Journal of the American College of Cardiology, 56(25), e50-e103.
[9] Breithardt, G., Borggrefe, M., Haerten, K., Seidl, K., Abendroth, R. R., Ostermeyer, J., … & Martinez, C. (1983). Role of programmed ventricular stimulation and noninvasive techniques as predictors of antiarrhythmic drug efficacy: a prospective, randomized study. Circulation, 68(6), 1131-1149.
[10] Ideker, R. E., Mikroulis, N. J., Walston II, A., Wallace, A. G., Peter, R. H., & Rosse, C. (1979). A method for detection of electrically active ischemic regions using multiple epicardial electrodes. Circulation, 59(1), 15-25.
[11] Perez, M. V., Mahaffey, K. W., Qualls, D. A., Wilkoff, B., Ho, C. Y., Steinberg, C., … & Runge, M. S. (2019). Large-scale assessment of a smartwatch to identify atrial fibrillation. New England Journal of Medicine, 381(20), 1909-1917.
[12] Hasin, Y., Seldin, M., & Lusis, A. (2017). Multi-omics approaches to disease. Genome medicine, 9(1), 38.
[13] Priori, S. G., Schwartz, P. J., Napolitano, C., Bloise, R., Crotti, L., Datino, R., … & Moss, A. J. (2003). Risk stratification in the long-QT syndrome. New England Journal of Medicine, 348(19), 1866-1874.
[14] Thygesen, K., Alpert, J. S., Jaffe, A. S., Simoons, M. L., Chaitman, B. R., White, H. D., … & Katus, H. A. (2018). Fourth universal definition of myocardial infarction (2018). Journal of the American College of Cardiology, 72(22), 2231-2264.
[15] Lopaschuk, G. D., Ussher, J. R., Folmes, C. D., Jaswal, J. S., Stanley, W. C. (2010). Myocardial fatty acid metabolism in health and disease. Physiological reviews, 90(1), 207-269.
[16] Lang, R. M., Bierig, M., Devereux, R. B., Flachskampf, F. A., Foster, E., Pellikka, P. A., … & Picard, M. H. (2005). Recommendations for chamber quantification: a report from the American Society of Echocardiography’s Guidelines and Standards Committee and the Chamber Quantification Writing Group, developed in conjunction with the European Association of Echocardiography, a branch of the European Society of Cardiology. Journal of the American Society of Echocardiography, 18(12), 1440-1463.
Multi-omics approaches, you say? So, when can I upload my daily Wordle score to predict my cardiovascular health? Finally, a use for all that brainpower!
That’s a creative idea! While Wordle scores might not directly translate to heart health (yet!), the concept of using daily data to track trends is definitely relevant. Wearable tech already uses similar principles to monitor activity and sleep patterns, and we’re exploring how to integrate such lifestyle data with multi-omics for personalized cardiovascular risk assessment. Thanks for the insightful comment!
Editor: MedTechNews.Uk
Thank you to our Sponsor Esdebe
So, we’re adding genomics, proteomics, and metabolomics to ECGs now? Will I need a PhD in bioinformatics just to understand my next check-up, or will there be an app for that?
That’s a great question! While a PhD might not be required, user-friendly apps and tools are definitely part of the vision. Our goal is to make this advanced analysis accessible and understandable for everyone, bridging the gap between complex data and actionable health insights.
Editor: MedTechNews.Uk
Thank you to our Sponsor Esdebe
The discussion around integrating ECG data with multi-omics is fascinating, especially the potential for identifying novel biomarkers. Exploring standardized data formats will be crucial for effective analysis and for fostering collaboration across different research groups.