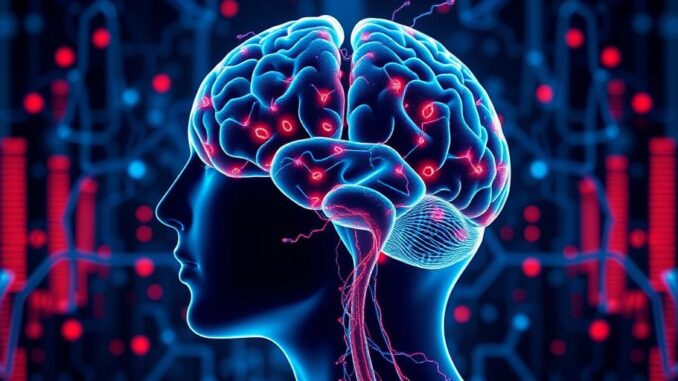
Abstract
The identification and validation of biomarkers hold immense promise for revolutionizing the diagnosis, prognosis, and treatment of mental health disorders. This review critically examines the current landscape of biomarker research in psychiatry, encompassing diverse methodologies from genomics and proteomics to neuroimaging and electrophysiology. We assess the progress made in identifying biomarkers for a range of conditions, including mood disorders, schizophrenia, anxiety disorders, and neurodevelopmental disorders. Special attention is given to the challenges associated with biomarker discovery and translation, such as heterogeneity of mental disorders, limitations in sample size and study design, the influence of environmental factors, and ethical considerations. We evaluate the reliability and validity of current findings, discussing the need for rigorous validation studies and the integration of multi-omic approaches. Furthermore, we explore the potential of biomarkers to personalize treatment strategies, predict treatment response, and facilitate early intervention. Finally, we outline future directions for biomarker research, emphasizing the importance of collaborative efforts, data sharing, and the development of novel technologies to accelerate progress in this field.
Many thanks to our sponsor Esdebe who helped us prepare this research report.
1. Introduction
Mental health disorders represent a significant global health burden, affecting millions of individuals worldwide and contributing substantially to disability, premature mortality, and economic costs (WHO, 2022). Despite advances in understanding the neurobiological underpinnings of these disorders, diagnosis and treatment remain largely based on clinical criteria and subjective assessments. This reliance on symptomatic classification often leads to diagnostic heterogeneity, delayed diagnoses, and suboptimal treatment outcomes. The limitations of current diagnostic and therapeutic approaches underscore the urgent need for objective and reliable biomarkers that can improve the precision and personalization of mental healthcare.
Biomarkers, defined as measurable indicators of biological state or condition, offer the potential to overcome these limitations by providing objective measures of disease risk, diagnosis, progression, and treatment response (Biomarkers Definitions Working Group, 2001). In the context of mental health, biomarkers encompass a wide range of molecular, genetic, neuroimaging, and physiological parameters that can be used to characterize the underlying pathophysiology of these disorders. The identification and validation of biomarkers can pave the way for earlier and more accurate diagnoses, the development of targeted therapies, and the prediction of treatment outcomes, ultimately leading to improved patient care.
This review aims to provide a comprehensive overview of the current status of biomarker research in mental health, highlighting the key advances, challenges, and future directions in this rapidly evolving field. We will discuss the various types of biomarkers being investigated, the methodologies used for their identification and measurement, the challenges associated with their validation and translation into clinical practice, and the ethical considerations surrounding their use. By critically evaluating the existing literature, we hope to provide insights into the potential of biomarkers to transform mental healthcare and accelerate the development of more effective and personalized treatments.
Many thanks to our sponsor Esdebe who helped us prepare this research report.
2. Types of Biomarkers in Mental Health Research
Biomarker research in mental health encompasses a wide array of approaches, investigating biomarkers at multiple levels of biological organization. These can be broadly categorized into:
-
Genetic Biomarkers: These include single nucleotide polymorphisms (SNPs), copy number variations (CNVs), and epigenetic modifications that influence gene expression and increase the risk of developing mental health disorders. Genome-wide association studies (GWAS) have identified numerous genetic variants associated with various disorders, although the effect sizes of individual SNPs are often small, suggesting that complex interactions between multiple genes and environmental factors contribute to disease risk. Epigenetic modifications, such as DNA methylation and histone modifications, can also play a role in regulating gene expression and influencing disease susceptibility.
-
Transcriptomic Biomarkers: These reflect the expression levels of genes and RNA molecules, providing insights into the biological pathways and processes that are dysregulated in mental health disorders. Microarray and RNA sequencing technologies allow for the comprehensive profiling of gene expression patterns in different brain regions and peripheral tissues. Differential gene expression analysis can identify genes that are upregulated or downregulated in individuals with mental health disorders compared to healthy controls, providing potential targets for therapeutic intervention.
-
Proteomic Biomarkers: These encompass the levels and modifications of proteins in biological fluids, such as blood, cerebrospinal fluid (CSF), and brain tissue. Proteomic approaches, such as mass spectrometry, can be used to identify and quantify proteins that are differentially expressed or modified in individuals with mental health disorders. Protein biomarkers can provide insights into the molecular mechanisms underlying disease pathogenesis and can be used to monitor treatment response.
-
Metabolomic Biomarkers: These reflect the levels of small molecules (metabolites) in biological fluids, providing information about metabolic pathways and processes that are altered in mental health disorders. Metabolomic approaches, such as gas chromatography-mass spectrometry (GC-MS) and liquid chromatography-mass spectrometry (LC-MS), can be used to identify and quantify metabolites that are differentially abundant in individuals with mental health disorders. Metabolomic biomarkers can provide insights into the metabolic consequences of disease and can be used to identify potential therapeutic targets.
-
Neuroimaging Biomarkers: These include structural and functional brain imaging measures, such as magnetic resonance imaging (MRI), positron emission tomography (PET), and electroencephalography (EEG). Structural MRI can reveal changes in brain volume, cortical thickness, and white matter integrity, while functional MRI can assess brain activity during cognitive tasks or at rest. PET imaging can measure the levels of neurotransmitters and receptors in the brain, providing insights into the neurochemical abnormalities associated with mental health disorders. EEG can measure brain electrical activity, providing information about brain oscillations and connectivity.
-
Electrophysiological Biomarkers: Similar to EEG, Magnetoencephalography (MEG) provides a non-invasive measure of brain activity by detecting magnetic fields produced by electrical currents in the brain. It has superior spatial resolution to EEG. Event-related potentials (ERPs), derived from EEG or MEG data, are time-locked neural responses to specific sensory, motor, or cognitive events. Alterations in ERP components, such as latency, amplitude, and morphology, can reflect deficits in information processing associated with various mental disorders. Transcranial magnetic stimulation (TMS) induces changes in cortical excitability. Its impact on neural circuits can serve as a biomarker for treatment response.
-
Peripheral Biomarkers: These include measures of hormones, cytokines, and other molecules in blood or saliva. The hypothalamic-pituitary-adrenal (HPA) axis, which regulates the stress response, is often dysregulated in mental health disorders, and measures of cortisol levels can be used as a biomarker of stress. Cytokines, which are signaling molecules involved in inflammation, are also often elevated in individuals with mental health disorders, suggesting a role for inflammation in disease pathogenesis. Furthermore, gut microbiome composition and function are increasingly recognized as potential biomarkers, as alterations in the gut microbiome have been linked to various mental health disorders through the gut-brain axis. These are very difficult to accurately measure and understand though, and should be assessed using a robust and repeatable approach.
Many thanks to our sponsor Esdebe who helped us prepare this research report.
3. Methodologies for Biomarker Identification and Measurement
The identification and measurement of biomarkers in mental health research require a diverse range of methodologies, each with its own strengths and limitations.
-
Omics Technologies: Genomics, transcriptomics, proteomics, and metabolomics technologies are used to comprehensively profile the molecular landscape of biological samples. These technologies can generate large datasets that require sophisticated bioinformatics and statistical analysis to identify potential biomarkers. Mass spectrometry, in particular, has emerged as a powerful tool for proteomic and metabolomic profiling, allowing for the identification and quantification of thousands of proteins and metabolites in a single sample. These are most effective when integrated. Multi-omic approaches that combine data from different omic platforms can provide a more comprehensive understanding of the molecular mechanisms underlying mental health disorders.
-
Neuroimaging Techniques: MRI, PET, and EEG are used to assess brain structure, function, and activity. These techniques provide non-invasive measures of brain activity that can be used to identify biomarkers of disease. For example, fMRI can be used to measure brain activity during cognitive tasks or at rest, revealing differences in brain activity patterns between individuals with mental health disorders and healthy controls. PET imaging can be used to measure the levels of neurotransmitters and receptors in the brain, providing insights into the neurochemical abnormalities associated with mental health disorders. There are various methods for pre-processing and extracting meaningful information from neuroimaging data. The choice of method is critical and can impact reproducibility.
-
Clinical and Cognitive Assessments: Standardized clinical interviews and cognitive tests are used to assess symptoms and cognitive function in individuals with mental health disorders. These assessments can provide valuable information about the clinical phenotype of the disorder and can be used to correlate with biomarker data. For example, scores on depression scales can be correlated with levels of cortisol or inflammatory markers in blood. Furthermore, advances in digital phenotyping, using data from smartphones and wearable sensors, offer new avenues for passively monitoring behavior and identifying digital biomarkers of mental health.
-
Statistical and Machine Learning Approaches: Sophisticated statistical and machine learning techniques are used to analyze biomarker data and identify patterns that can distinguish between individuals with mental health disorders and healthy controls. Machine learning algorithms can be trained to predict disease risk, diagnosis, or treatment response based on biomarker profiles. These approaches are particularly useful for analyzing high-dimensional data, such as those generated by omics technologies. However, it is important to validate machine learning models on independent datasets to ensure their generalizability and avoid overfitting. Causal inference methods are increasingly being used to establish causal relationships between biomarkers and disease outcomes. It’s worth remembering though that correlation doesn’t imply causation, and that these methods can be sensitive to the parameters used.
Many thanks to our sponsor Esdebe who helped us prepare this research report.
4. Challenges in Biomarker Research in Mental Health
Despite the significant progress made in biomarker research in mental health, several challenges remain that hinder the identification, validation, and translation of biomarkers into clinical practice.
-
Heterogeneity of Mental Disorders: Mental health disorders are highly heterogeneous, both in terms of their clinical presentation and their underlying pathophysiology. This heterogeneity makes it difficult to identify biomarkers that are consistently associated with specific disorders. Furthermore, diagnostic boundaries between different mental health disorders can be blurred, making it challenging to define clear diagnostic groups for biomarker studies. Transdiagnostic approaches, which focus on identifying biomarkers that cut across diagnostic boundaries, may be a more promising strategy for biomarker discovery in mental health.
-
Small Sample Sizes and Study Design Limitations: Many biomarker studies in mental health are limited by small sample sizes and study design limitations. Small sample sizes can lead to false positive findings and can limit the power to detect true associations between biomarkers and disease. Study design limitations, such as cross-sectional designs, can make it difficult to establish causal relationships between biomarkers and disease outcomes. Longitudinal studies, which follow individuals over time, are needed to assess the predictive validity of biomarkers and to understand how biomarkers change over the course of the disease. This requires large cohorts to ensure statistical power and to reduce the impact of confounding factors. Furthermore, the complexity of mental health disorders demands carefully controlled studies to minimize bias and ensure the reliability of findings.
-
Influence of Environmental Factors: Environmental factors, such as stress, diet, and exposure to toxins, can influence the expression of biomarkers and can confound the results of biomarker studies. It is important to carefully control for environmental factors in biomarker studies and to consider the interactions between genes and environment in disease pathogenesis. Furthermore, socioeconomic factors, access to healthcare, and cultural influences can also impact mental health outcomes and should be considered in biomarker research.
-
Lack of Reproducibility and Validation: A major challenge in biomarker research is the lack of reproducibility of findings across different studies. This can be due to differences in study populations, methodologies, and data analysis techniques. It is essential to validate biomarker findings in independent datasets to ensure their generalizability and to avoid overfitting. Furthermore, standardization of protocols for sample collection, processing, and analysis is needed to improve the reproducibility of biomarker studies. This includes rigorous quality control measures and the use of standardized assays.
-
Ethical Considerations: The use of biomarkers in mental health raises several ethical considerations, including privacy, confidentiality, and the potential for discrimination. It is important to protect the privacy of individuals who participate in biomarker studies and to ensure that their data is used responsibly. Furthermore, the use of biomarkers to predict disease risk or treatment response raises concerns about the potential for discrimination based on genetic or biological information. Guidelines and regulations are needed to ensure that biomarkers are used ethically and responsibly in mental health research and clinical practice. In particular, the potential for stigmatization associated with certain biomarkers requires careful attention.
Many thanks to our sponsor Esdebe who helped us prepare this research report.
5. Biomarkers for Predicting Treatment Response
One of the most promising applications of biomarkers in mental health is their potential to predict treatment response. Identifying biomarkers that can predict which individuals are most likely to respond to a particular treatment can help to personalize treatment strategies and to avoid exposing patients to ineffective or harmful treatments.
-
Pharmacogenomics: Pharmacogenomics, the study of how genes affect a person’s response to drugs, has identified several genetic variants that are associated with treatment response in mental health disorders. For example, genetic variants in genes encoding drug-metabolizing enzymes can affect the levels of drugs in the body and can influence treatment efficacy and side effects. Genetic testing can be used to identify individuals who are likely to respond to a particular drug or who are at risk for developing side effects. CYP450 enzyme variants are well known, and can be used to adjust dosages for patients on a variety of psychotropic medications.
-
Neuroimaging Biomarkers: Neuroimaging techniques can be used to identify biomarkers that predict treatment response in mental health disorders. For example, baseline brain activity patterns measured by fMRI can predict which individuals are most likely to respond to antidepressant medication or cognitive behavioral therapy. Furthermore, changes in brain activity patterns during treatment can be used to monitor treatment response and to adjust treatment strategies accordingly. Resting-state functional connectivity, particularly in regions related to the default mode network and salience network, has shown promise in predicting response to various interventions.
-
Electrophysiological Biomarkers: ERPs and EEG measures have also been investigated as potential predictors of treatment response. For instance, pre-treatment EEG measures, such as alpha power and coherence, have been linked to antidepressant response. Furthermore, changes in ERP components during treatment can reflect neural plasticity and predict long-term outcomes. Theta/beta power ratio can also be used to assess the effectiveness of treatments for ADHD.
-
Molecular Biomarkers: Molecular biomarkers, such as levels of inflammatory markers or neurotransmitters in blood or CSF, can also predict treatment response in mental health disorders. For example, elevated levels of inflammatory markers have been associated with poorer response to antidepressant medication. Furthermore, changes in levels of neurotransmitters during treatment can be used to monitor treatment response and to adjust treatment strategies accordingly. The combination of molecular and neuroimaging biomarkers may provide a more comprehensive and accurate prediction of treatment response.
Many thanks to our sponsor Esdebe who helped us prepare this research report.
6. Future Directions and Conclusion
Biomarker research in mental health holds immense promise for transforming the diagnosis, prognosis, and treatment of these disorders. However, significant challenges remain that must be addressed to realize the full potential of biomarkers in clinical practice. Future research should focus on:
-
Large-Scale Collaborative Studies: Large-scale collaborative studies are needed to increase sample sizes and to improve the statistical power of biomarker studies. These studies should involve multiple research centers and should collect data from diverse populations to ensure the generalizability of findings. Data sharing and collaboration are essential to accelerate progress in biomarker research. The creation of standardized data repositories and the development of common data elements can facilitate data sharing and collaboration.
-
Multi-Omic Approaches: Multi-omic approaches that integrate data from different omic platforms can provide a more comprehensive understanding of the molecular mechanisms underlying mental health disorders. These approaches can identify biomarkers that are not detectable by single-omic approaches and can provide insights into the complex interactions between genes, proteins, metabolites, and environmental factors.
-
Longitudinal Studies: Longitudinal studies are needed to assess the predictive validity of biomarkers and to understand how biomarkers change over the course of the disease. These studies should follow individuals over time and should collect data on clinical symptoms, cognitive function, and biomarker levels. Longitudinal data can be used to develop predictive models that can identify individuals who are at risk for developing mental health disorders or who are likely to respond to a particular treatment. This requires careful selection of time points and the development of statistical methods to account for repeated measures.
-
Development of Novel Technologies: The development of novel technologies for biomarker identification and measurement is needed to overcome the limitations of current methods. For example, new imaging techniques can provide more detailed information about brain structure and function. New biosensors can measure biomarkers in real-time and can provide continuous monitoring of physiological parameters. Advances in artificial intelligence and machine learning can be used to analyze complex biomarker data and to identify patterns that are not detectable by traditional statistical methods.
-
Translation into Clinical Practice: The ultimate goal of biomarker research is to translate biomarker findings into clinical practice. This requires the development of validated biomarker assays that can be used in routine clinical settings. Furthermore, clinical guidelines and decision support tools are needed to guide the use of biomarkers in diagnosis, prognosis, and treatment. The integration of biomarkers into electronic health records can facilitate the use of biomarkers in clinical decision-making.
In conclusion, biomarker research in mental health is a rapidly evolving field that holds great promise for improving the lives of individuals with these disorders. By addressing the challenges outlined in this review and by pursuing the future directions outlined above, we can accelerate progress in biomarker discovery and translation and can ultimately transform mental healthcare.
Many thanks to our sponsor Esdebe who helped us prepare this research report.
References
- Biomarkers Definitions Working Group. (2001). Biomarkers and surrogate endpoints: preferred definitions and conceptual framework. Clinical Pharmacology & Therapeutics, 69(3), 89-95.
- World Health Organization (WHO). (2022). Mental health. Retrieved from https://www.who.int/news-room/fact-sheets/detail/mental-health-strengthening-our-response
- Dwivedi, Y. (2021). Advances in understanding the neurobiology of depression. Dialogues in Clinical Neuroscience, 23(4), 349-360.
- Insel, T. R. (2009). Translating neuroscience to mental health. Nature, 460(7252), 194-198.
- Kapur, S., Phillips, A. G., & Insel, T. R. (2012). Why has it taken so long for biological psychiatry to develop clinical tests?. Molecular Psychiatry, 17(12), 1174-1179.
- Ramasamy, A., Lambert, D., Mathieson, I., & Whittaker, P. (2008). Genome-wide association studies: design, analysis and interpretation. Nature Reviews Genetics, 9(2), 99-110.
- Hyman, S. E. (2008). Can neuroscience be a guide to discovery of new medicines for mental disorders?. Nature Reviews Neuroscience, 9(1), 67-75.
- Miller, A. H., Raison, C. L. (2016). The role of inflammation in depression: from evolutionary imperative to modern treatment target. Nature Reviews Immunology, 16, 22-34.
- Arbabshirani, M. R., Havaki, S., Allen, J., Calhoun, V. D., Kiehl, K. A., Pearlson, G. D., … & Sui, J. (2017). Machine learning in schizophrenia: A systematic review and meta-analysis. European Archives of Psychiatry and Clinical Neuroscience, 267(8), 677-693.
- Bot, B. M., Suver, C., Neto, E. C., Kellen, M., Klein, A., Bareis, N., … & Friend, S. H. (2016). Integrating smartwatches into clinical trials: Bringing connected health to personalized medicine. PloS one, 11(5), e0154468.
So, if our gut microbiome is the new frontier for mental health biomarkers, does that mean yogurt commercials are now stealth public service announcements? Asking for a friend who may or may not be a probiotic.
That’s a hilarious thought! It’s fascinating how much the gut-brain axis is being explored. Perhaps future yogurt commercials will highlight specific strains and their mental health benefits, moving beyond just digestive health. It opens up a whole new avenue for preventative mental wellness!
Editor: MedTechNews.Uk
Thank you to our Sponsor Esdebe
If we’re chasing biomarkers to predict treatment response, does that mean my future psychiatrist will be demanding a blood sample along with my insurance card? And will they offer a frequent-flyer program for repeat venipuncture patients?
That’s a great, and funny, question! While blood samples are a possibility, the field is also exploring less invasive options like digital phenotyping using wearable sensors (mentioned in the article). Perhaps future mental health assessments will involve analyzing data from your smartwatch alongside other factors. No needles required!
Editor: MedTechNews.Uk
Thank you to our Sponsor Esdebe