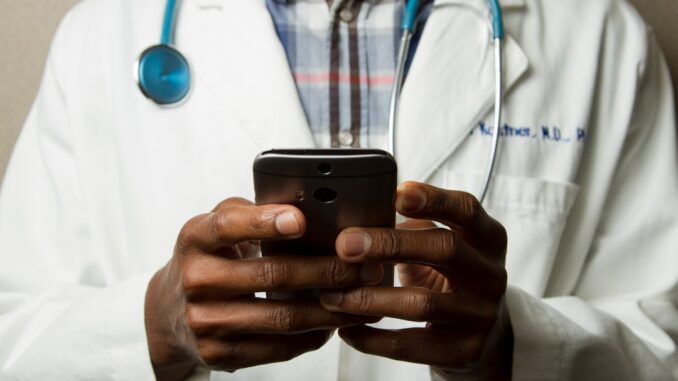
Abstract
Clinical documentation is the cornerstone of modern healthcare, serving as the primary record of patient care, a vital source for communication among healthcare providers, a foundation for billing and reimbursement, and a crucial resource for research and quality improvement. However, traditional clinical documentation processes are often time-consuming, error-prone, and contribute to physician burnout. This research report provides a comprehensive analysis of the current state of clinical documentation, exploring the multifaceted challenges and opportunities presented by technological advancements, evolving regulatory requirements, and the increasing demand for data-driven healthcare. It examines the impact of innovative technologies, such as artificial intelligence (AI), natural language processing (NLP), and speech recognition, on streamlining documentation workflows, enhancing data quality, and improving patient outcomes. Furthermore, the report addresses the critical ethical and legal considerations associated with these technologies, including privacy, security, and bias. Finally, this report proposes recommendations for optimizing clinical documentation practices, promoting interoperability, and ensuring that documentation serves as a valuable asset for improving the quality, safety, and efficiency of healthcare delivery.
Many thanks to our sponsor Esdebe who helped us prepare this research report.
1. Introduction
Clinical documentation, the comprehensive record of a patient’s medical history, diagnoses, treatments, and outcomes, is fundamental to the provision of safe, effective, and patient-centered care. High-quality clinical documentation facilitates seamless communication among healthcare professionals, ensures continuity of care, supports accurate billing and reimbursement, provides a legal record of treatment, and serves as a rich source of data for research, quality improvement, and public health initiatives. However, the current state of clinical documentation is often characterized by inefficiencies, inconsistencies, and challenges that negatively impact healthcare providers, patients, and the healthcare system as a whole.
Traditional documentation methods, which often rely on manual processes, paper-based records, and cumbersome electronic health record (EHR) systems, contribute to physician burnout, reduce face-to-face time with patients, and limit the availability of timely and accurate data. Studies have shown that physicians spend a significant portion of their workdays on documentation-related tasks, leaving less time for direct patient care and contributing to increased stress and fatigue (Verghese et al., 2018). Furthermore, inadequate or incomplete documentation can lead to medical errors, inaccurate billing, and difficulties in tracking patient outcomes.
The emergence of innovative technologies, such as AI, NLP, and speech recognition, offers the potential to transform clinical documentation processes and address many of the challenges associated with traditional methods. These technologies can automate documentation tasks, extract relevant information from unstructured data, improve the accuracy and completeness of documentation, and provide real-time decision support to healthcare providers. However, the adoption of these technologies also raises important questions about data privacy, security, bias, and the potential impact on the physician-patient relationship.
This research report aims to provide a comprehensive overview of the current state of clinical documentation, exploring the challenges, opportunities, and critical considerations associated with the integration of technology into documentation workflows. The report will examine the impact of various technologies on documentation quality, efficiency, and patient outcomes, while also addressing the ethical, legal, and regulatory aspects of using these technologies in clinical practice. By providing a nuanced and evidence-based analysis of the clinical documentation landscape, this report seeks to inform healthcare professionals, policymakers, and technology developers about the best practices for optimizing documentation processes and ensuring that documentation serves as a valuable tool for improving the quality, safety, and efficiency of healthcare delivery.
Many thanks to our sponsor Esdebe who helped us prepare this research report.
2. Current Challenges in Clinical Documentation
Despite the advancements in EHR technology, clinical documentation remains a significant challenge for healthcare providers and organizations. Several factors contribute to these challenges, including:
2.1 Time Burden and Physician Burnout:
One of the most pressing issues in clinical documentation is the significant time burden it places on healthcare providers. Studies have consistently shown that physicians spend a substantial portion of their workdays on documentation-related tasks, often at the expense of direct patient care. For instance, a study published in the Annals of Internal Medicine found that physicians spend approximately two hours on EHR tasks for every hour of direct patient care (Sinsky et al., 2016). This excessive documentation burden contributes to physician burnout, which is a growing concern in the healthcare industry. Burnout can lead to decreased job satisfaction, increased turnover rates, and compromised patient care (West et al., 2018).
The complexity of EHR systems and the need to comply with ever-changing regulatory requirements exacerbate the time burden. Physicians often find themselves navigating complex EHR interfaces, searching for specific information, and entering data into multiple fields. The pressure to document everything thoroughly to avoid potential legal or financial repercussions adds to the workload and can lead to “note bloat,” where documentation becomes excessively detailed and difficult to navigate.
2.2 Data Quality and Accuracy:
Ensuring the quality and accuracy of clinical documentation is crucial for patient safety, care coordination, and data analysis. However, several factors can compromise data quality, including:
- Incomplete or Missing Information: Incomplete or missing information can lead to misdiagnosis, inappropriate treatment, and difficulties in tracking patient outcomes. This can occur due to time constraints, inadequate training, or errors in data entry.
- Inconsistent Terminology and Coding: The use of inconsistent terminology and coding practices can hinder data analysis and make it difficult to compare data across different healthcare settings. This issue is particularly relevant when dealing with unstructured data, such as clinical notes.
- Copy-and-Paste Errors: The practice of copying and pasting information from previous notes can introduce errors and perpetuate inaccuracies. This can lead to the propagation of outdated or incorrect information, which can have serious consequences for patient care.
- Lack of Standardized Data Elements: The absence of standardized data elements makes it challenging to aggregate and analyze data across different EHR systems. This limits the ability to identify trends, track outcomes, and improve the quality of care.
2.3 Interoperability Challenges:
Interoperability, the ability of different EHR systems to exchange and use information seamlessly, is essential for effective care coordination and data sharing. However, despite efforts to promote interoperability, significant challenges remain.
- Technical Barriers: Different EHR systems often use different data formats and standards, making it difficult to exchange information without manual intervention. This can lead to delays, errors, and increased costs.
- Semantic Interoperability: Even when data can be exchanged, differences in terminology and coding practices can make it difficult to understand and use the information effectively. Achieving semantic interoperability requires the use of standardized terminologies and ontologies.
- Organizational and Policy Barriers: Organizational and policy barriers, such as concerns about data privacy and security, can also hinder interoperability. These barriers often require policy changes and collaborative efforts to overcome.
2.4 Regulatory Compliance:
Healthcare providers must comply with a complex web of regulations related to clinical documentation, including HIPAA, the HITECH Act, and various state-specific laws. These regulations aim to protect patient privacy, ensure data security, and promote the meaningful use of EHR technology. However, complying with these regulations can be challenging and time-consuming.
- HIPAA: The Health Insurance Portability and Accountability Act (HIPAA) sets standards for the privacy and security of protected health information (PHI). Healthcare providers must implement policies and procedures to protect PHI from unauthorized access, use, or disclosure. Violations of HIPAA can result in significant fines and penalties.
- HITECH Act: The Health Information Technology for Economic and Clinical Health (HITECH) Act was enacted to promote the adoption and meaningful use of EHR technology. The HITECH Act also strengthens HIPAA’s privacy and security provisions and increases penalties for violations.
- Meaningful Use/Promoting Interoperability: The Meaningful Use program, now known as Promoting Interoperability, provides incentives for healthcare providers to adopt and use EHR technology in a meaningful way. However, complying with the program’s requirements can be challenging, and failure to meet the requirements can result in reduced reimbursement rates.
These challenges highlight the need for innovative solutions to streamline clinical documentation processes, improve data quality, enhance interoperability, and reduce the burden on healthcare providers. The following sections will explore how technology can address these challenges and transform clinical documentation in the future.
Many thanks to our sponsor Esdebe who helped us prepare this research report.
3. Technology-Driven Solutions for Clinical Documentation
Advancements in technology offer promising solutions to address the challenges in clinical documentation and enhance the efficiency, accuracy, and usability of healthcare data. Key technologies impacting clinical documentation include:
3.1 Speech Recognition Technology:
Speech recognition technology, also known as voice recognition, converts spoken words into text. This technology has the potential to significantly reduce the time and effort required for documentation. Healthcare providers can dictate clinical notes, orders, and other documentation directly into the EHR system, eliminating the need for manual typing. Modern speech recognition software leverages AI and machine learning to improve accuracy and adapt to individual speech patterns and accents. This greatly reduces transcription errors and facilitates a natural documentation workflow. Some advanced systems even support real-time transcription and contextual suggestions, further enhancing efficiency.
- Benefits: Reduced documentation time, improved accuracy, increased physician satisfaction, and enhanced patient engagement.
- Challenges: Initial training and adaptation, potential for errors in noisy environments, and the need for ongoing maintenance and updates.
3.2 Natural Language Processing (NLP):
NLP is a branch of AI that focuses on enabling computers to understand and process human language. In clinical documentation, NLP can be used to extract relevant information from unstructured text, such as clinical notes, discharge summaries, and radiology reports. NLP algorithms can identify key concepts, relationships, and entities within the text, and then structure this information in a way that can be easily analyzed and used for decision support. NLP also helps in automating tasks such as coding, billing, and quality reporting.
- Benefits: Automated data extraction, improved data quality, enhanced clinical decision support, and reduced administrative burden.
- Challenges: The complexity of medical language, the need for large training datasets, and the potential for bias in NLP algorithms.
3.3 Artificial Intelligence (AI) and Machine Learning (ML):
AI and ML are transforming clinical documentation in several ways. AI-powered systems can automate documentation tasks, such as generating preliminary drafts of clinical notes, identifying potential diagnoses, and flagging inconsistencies or errors in documentation. ML algorithms can be trained on large datasets of clinical data to predict patient outcomes, identify high-risk patients, and personalize treatment plans. These technologies can also be used to improve the accuracy and completeness of documentation by identifying missing information and prompting providers to fill in the gaps. AI-powered virtual assistants can also support physicians during patient encounters, retrieving relevant information from the EHR and suggesting appropriate actions.
- Benefits: Automated documentation, improved accuracy, enhanced decision support, personalized treatment plans, and increased efficiency.
- Challenges: Data privacy and security concerns, the potential for bias in AI algorithms, and the need for explainable AI to ensure transparency and trust.
3.4 Computer-Assisted Physician Documentation (CAPD):
CAPD is a technology that provides real-time feedback and guidance to healthcare providers during the documentation process. CAPD systems analyze the information entered into the EHR and suggest relevant codes, diagnoses, and procedures. They also identify potential errors or omissions and prompt providers to correct them. This helps to improve the accuracy and completeness of documentation, reduce coding errors, and ensure compliance with regulatory requirements. CAPD systems can be integrated with speech recognition and NLP technologies to provide a comprehensive documentation solution.
- Benefits: Improved documentation accuracy, reduced coding errors, enhanced compliance, and increased efficiency.
- Challenges: Integration with existing EHR systems, the need for ongoing maintenance and updates, and the potential for alert fatigue.
3.5 Blockchain Technology:
While still in its early stages of adoption in healthcare, blockchain technology offers potential solutions for secure and transparent data sharing. In clinical documentation, blockchain can be used to create a secure and immutable record of patient information. This can improve data integrity, facilitate interoperability, and enhance patient privacy. Blockchain can also be used to track the provenance of data, ensuring that it has not been tampered with and that it comes from a trusted source. This is particularly important for sensitive data, such as genetic information and mental health records.
- Benefits: Improved data security, enhanced data integrity, increased transparency, and facilitated interoperability.
- Challenges: Scalability, regulatory uncertainty, and the need for widespread adoption to realize its full potential.
These technologies represent a significant step forward in transforming clinical documentation and addressing the challenges that have plagued the healthcare industry for years. However, successful implementation requires careful planning, adequate training, and a clear understanding of the ethical and legal considerations associated with these technologies.
Many thanks to our sponsor Esdebe who helped us prepare this research report.
4. Regulatory Landscape and Ethical Considerations
The integration of technology into clinical documentation is governed by a complex regulatory landscape, primarily shaped by HIPAA and the HITECH Act, and raises several ethical considerations that must be addressed to ensure responsible and beneficial use of these technologies.
4.1 HIPAA and Data Privacy:
The Health Insurance Portability and Accountability Act (HIPAA) is the cornerstone of data privacy in healthcare. It sets standards for the privacy and security of protected health information (PHI). The use of technology in clinical documentation must comply with HIPAA’s requirements for data encryption, access controls, and breach notification. Healthcare organizations must implement policies and procedures to protect PHI from unauthorized access, use, or disclosure. This includes ensuring that AI algorithms and NLP models are trained on de-identified data and that access to PHI is restricted to authorized personnel. Furthermore, patients must be informed about how their data is being used and given the opportunity to control their data.
4.2 HITECH Act and Data Security:
The Health Information Technology for Economic and Clinical Health (HITECH) Act strengthens HIPAA’s privacy and security provisions and increases penalties for violations. The HITECH Act also promotes the adoption and meaningful use of EHR technology. Healthcare organizations must implement robust security measures to protect patient data from cyberattacks, data breaches, and other threats. This includes using firewalls, intrusion detection systems, and other security technologies to prevent unauthorized access to data. Organizations must also conduct regular security audits and risk assessments to identify and address potential vulnerabilities. Failing to comply with the HITECH Act can result in significant fines and penalties.
4.3 GDPR and International Regulations:
For healthcare organizations that operate internationally or collect data from individuals in the European Union, the General Data Protection Regulation (GDPR) must also be considered. The GDPR sets strict requirements for the collection, processing, and storage of personal data. It gives individuals greater control over their data and requires organizations to be transparent about how they use data. Compliance with the GDPR requires healthcare organizations to implement robust data governance policies and procedures and to obtain explicit consent from individuals before collecting or processing their data.
4.4 Ethical Considerations:
Beyond regulatory compliance, the use of technology in clinical documentation raises several ethical considerations that must be addressed to ensure responsible and beneficial use.
- Bias: AI algorithms and NLP models can be biased if they are trained on biased data. This can lead to inaccurate or discriminatory outcomes. Healthcare organizations must take steps to mitigate bias in AI algorithms and to ensure that these technologies are used fairly and equitably.
- Transparency: The algorithms used in clinical documentation should be transparent and explainable. Healthcare providers must be able to understand how these algorithms work and how they arrive at their conclusions. This is essential for building trust in these technologies and for ensuring that they are used appropriately.
- Autonomy: The use of technology in clinical documentation should not undermine the autonomy of healthcare providers. Physicians should still be able to exercise their clinical judgment and make decisions based on their own knowledge and experience. Technology should be used to augment, not replace, the skills and expertise of healthcare providers.
- Privacy: The use of technology in clinical documentation must protect patient privacy. Healthcare organizations must implement policies and procedures to ensure that patient data is not disclosed without their consent. This includes using anonymization techniques to protect the privacy of individuals whose data is used to train AI algorithms.
- Accountability: Healthcare organizations must be accountable for the use of technology in clinical documentation. This includes establishing clear lines of responsibility for the development, implementation, and use of these technologies. Organizations must also have mechanisms in place to monitor the performance of these technologies and to address any problems that may arise.
Addressing these regulatory and ethical considerations is crucial for ensuring that the use of technology in clinical documentation is both safe and effective. Healthcare organizations must take a proactive approach to compliance and ethics, and they must involve stakeholders from across the organization in the development and implementation of these technologies.
Many thanks to our sponsor Esdebe who helped us prepare this research report.
5. Potential Benefits and Risks
The integration of technology into clinical documentation offers a wide range of potential benefits, but also presents certain risks that must be carefully managed. A balanced assessment of these factors is crucial for successful implementation and maximizing the positive impact on healthcare.
5.1 Potential Benefits:
- Improved Patient Care: Streamlined documentation processes and enhanced data quality can lead to better informed clinical decision-making and improved patient outcomes. Accurate and complete documentation ensures continuity of care and reduces the risk of medical errors.
- Reduced Costs: Automation of documentation tasks can reduce administrative costs and free up healthcare providers to focus on patient care. Improved coding and billing accuracy can also lead to increased revenue.
- Enhanced Data Analysis: The use of NLP and AI can unlock valuable insights from clinical data, enabling healthcare organizations to identify trends, track outcomes, and improve the quality of care. This data can be used to support research, quality improvement initiatives, and public health programs.
- Increased Efficiency: Technology can automate many of the time-consuming tasks associated with clinical documentation, such as data entry, coding, and billing. This can free up healthcare providers to focus on more important tasks, such as patient care.
- Improved Physician Satisfaction: Reducing the documentation burden can improve physician satisfaction and reduce burnout. This can lead to increased job satisfaction, reduced turnover rates, and improved patient care.
5.2 Potential Risks:
- Privacy Concerns: The use of technology in clinical documentation raises concerns about patient privacy. Healthcare organizations must implement robust security measures to protect patient data from unauthorized access, use, or disclosure.
- Data Security: Clinical data is a valuable target for cyberattacks. Healthcare organizations must implement robust security measures to protect patient data from cyberattacks and data breaches.
- Bias: AI algorithms and NLP models can be biased if they are trained on biased data. This can lead to inaccurate or discriminatory outcomes. Healthcare organizations must take steps to mitigate bias in AI algorithms and to ensure that these technologies are used fairly and equitably.
- Lack of Transparency: The algorithms used in clinical documentation should be transparent and explainable. Healthcare providers must be able to understand how these algorithms work and how they arrive at their conclusions. This is essential for building trust in these technologies and for ensuring that they are used appropriately.
- Dependence on Technology: Over-reliance on technology can lead to a loss of clinical skills and expertise. Healthcare providers must maintain their clinical judgment and skills, even when using technology to support their work.
- Alert Fatigue: The use of technology in clinical documentation can lead to alert fatigue, where healthcare providers become desensitized to alerts and warnings. This can lead to missed opportunities to prevent errors and improve patient care.
- Implementation Costs: The implementation of new technologies can be expensive. Healthcare organizations must carefully consider the costs and benefits of implementing new technologies before making a decision.
Mitigating these risks requires a proactive and comprehensive approach that includes implementing robust security measures, addressing bias in AI algorithms, ensuring transparency and explainability, and providing adequate training and support to healthcare providers. By carefully managing these risks, healthcare organizations can maximize the benefits of technology in clinical documentation and improve the quality, safety, and efficiency of healthcare delivery.
Many thanks to our sponsor Esdebe who helped us prepare this research report.
6. Recommendations and Future Directions
To optimize clinical documentation in the evolving healthcare landscape, several recommendations and future directions should be considered:
6.1 Standardization and Interoperability:
- Promote the adoption of standardized data elements and terminologies to improve data quality and facilitate interoperability.
- Support the development and implementation of open standards for data exchange to enable seamless communication between different EHR systems.
- Incentivize healthcare organizations to participate in health information exchanges (HIEs) to share patient data securely and efficiently.
6.2 Technology Adoption and Implementation:
- Provide adequate training and support to healthcare providers on the use of new technologies for clinical documentation.
- Involve healthcare providers in the design and implementation of new technologies to ensure that they meet their needs and workflows.
- Develop and implement clear policies and procedures for the use of technology in clinical documentation.
- Implement robust security measures to protect patient data from unauthorized access, use, or disclosure.
- Monitor the performance of new technologies and make adjustments as needed to ensure that they are meeting their intended goals.
6.3 Research and Innovation:
- Invest in research to develop new and innovative technologies for clinical documentation, such as AI-powered virtual assistants and blockchain-based data sharing platforms.
- Support the development of open-source tools and resources for clinical documentation to lower the cost of adoption and promote innovation.
- Encourage collaboration between researchers, healthcare providers, and technology developers to develop solutions that meet the needs of the healthcare industry.
6.4 Policy and Regulation:
- Develop clear and consistent policies and regulations for the use of technology in clinical documentation.
- Address ethical concerns related to the use of AI and other technologies in healthcare, such as bias and transparency.
- Protect patient privacy and data security by implementing robust regulatory frameworks and enforcement mechanisms.
- Promote the responsible use of technology in clinical documentation to ensure that it benefits patients and improves the quality of care.
6.5 Future Directions:
- Predictive Analytics: Utilize AI and machine learning to predict patient outcomes and identify high-risk patients, enabling proactive interventions and personalized treatment plans.
- Personalized Documentation: Tailor documentation templates and workflows to the specific needs of individual patients and healthcare providers, improving efficiency and reducing the documentation burden.
- Remote Monitoring: Integrate remote patient monitoring data into clinical documentation to provide a more complete and accurate picture of patient health.
- Patient-Generated Data: Incorporate patient-generated data, such as wearable sensor data and patient-reported outcomes, into clinical documentation to improve patient engagement and enhance care coordination.
By implementing these recommendations and pursuing these future directions, the healthcare industry can optimize clinical documentation and ensure that it serves as a valuable tool for improving the quality, safety, and efficiency of healthcare delivery.
Many thanks to our sponsor Esdebe who helped us prepare this research report.
7. Conclusion
Clinical documentation is undergoing a significant transformation driven by technological advancements, evolving regulatory requirements, and the increasing demand for data-driven healthcare. While traditional documentation processes are often inefficient, error-prone, and contribute to physician burnout, innovative technologies such as AI, NLP, and speech recognition offer the potential to streamline workflows, enhance data quality, and improve patient outcomes.
However, the adoption of these technologies also raises important ethical, legal, and regulatory considerations. Healthcare organizations must address data privacy and security concerns, mitigate bias in AI algorithms, ensure transparency and explainability, and protect patient autonomy. By carefully managing these risks and implementing best practices for technology adoption, healthcare organizations can maximize the benefits of technology in clinical documentation and improve the quality, safety, and efficiency of healthcare delivery.
The future of clinical documentation will likely involve a greater emphasis on standardization, interoperability, and the use of AI and machine learning to extract insights from clinical data and support clinical decision-making. By embracing these technologies and addressing the associated challenges, the healthcare industry can transform clinical documentation into a valuable asset for improving patient care and advancing healthcare innovation.
Many thanks to our sponsor Esdebe who helped us prepare this research report.
References
- Sinsky, C. A., Colligan, J., Li, L., Prgomet, M., Reynolds, S., Goeders, L., … & Tutty, M. (2016). Allocation of physician time in ambulatory practice: A time and motion study in 4 specialties. Annals of Internal Medicine, 165(11), 753-760.
- Verghese, A., Brady, E., Shah, N. H., Harrington, R. A. (2018). Maximizing the value of the human presence in healthcare. JAMA, 319(1), 23–24.
- West, C. P., Dyrbye, L. N., Erwin, P. J., & Shanafelt, T. D. (2016). Interventions to prevent and reduce physician burnout: a systematic review and meta-analysis. The Lancet, 388(10057), 2272-2281.
- Murdoch, T. B., & Detsky, A. S. (2013). The inevitable application of big data to health care. JAMA, 309(21), 2249-2250.
- Shortliffe, E. H., & Cimino, J. J. (Eds.). (2014). Biomedical informatics: computer applications in health care and biomedicine. Springer.
- Kern, L. M., Edwards, A. M., Isakov, A., & McCoy, A. B. (2013). Natural language processing in healthcare: a review of applications and challenges. International journal of medical informatics, 82(6), 445-454.
- Kohane, I. S., Altman, R. B., & Butte, A. J. (2011). Health-IT as a learning healthcare system. New England Journal of Medicine, 365(24), 2271.
- Price, W. N., & Cohen, I. G. (2019). Privacy in the age of medical big data. Nature medicine, 25(1), 37-43.
- Meskó, B., Dusek, P., & Knap, J. (2018). Opinion: Competing with medical chatbot apps as a physician. NPJ digital medicine, 1(1), 1-3.
AI scribes writing our notes? Sounds amazing…until they start embellishing my coffee intake as “essential for maintaining peak cognitive function.” Suddenly, my caffeine addiction is “optimized healthcare.” Who needs a doctor when you have a well-meaning robot novelist?
That’s a hilarious and insightful point! The potential for AI to reframe our habits is definitely something to consider. Perhaps future systems will need a ‘transparency toggle’ to show the unvarnished truth. Thanks for sparking this discussion!
Editor: MedTechNews.Uk
Thank you to our Sponsor Esdebe
Given the potential for predictive analytics within clinical documentation, what safeguards should be implemented to ensure equitable and unbiased applications across diverse patient populations, particularly in underserved communities?
That’s an incredibly important question! Ensuring equitable application of predictive analytics, especially in underserved communities, requires careful attention. We need diverse datasets for training AI, continuous monitoring for bias, and transparent algorithms. Community involvement in the development process can also help ensure fairness and address specific needs. Let’s continue discussing practical safeguards!
Editor: MedTechNews.Uk
Thank you to our Sponsor Esdebe
The report highlights the potential of AI and NLP to extract insights from clinical data. What are the best practices for validating the accuracy and reliability of these AI-driven insights to ensure they translate into improved clinical decision-making and patient outcomes?