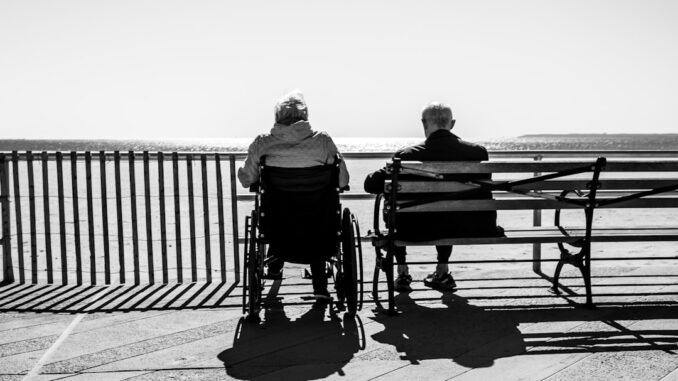
Abstract
Falls are a leading cause of morbidity and mortality in older adults, contributing significantly to healthcare costs and diminished quality of life. While exercise and home modifications are established interventions, a comprehensive approach to fall prevention necessitates a deeper understanding of the multi-system pathophysiology underlying falls, refined risk stratification methodologies, and the exploration of advanced interventional strategies. This research report synthesizes current literature to provide an in-depth analysis of these critical areas. We examine the complex interplay of neurological, musculoskeletal, cardiovascular, and sensory systems in fall etiology. We further scrutinize the limitations of existing risk assessment tools and propose advancements incorporating biomechanical and physiological biomarkers. Finally, we explore emerging interventional strategies, including targeted pharmacological interventions, neurorehabilitation techniques, and technology-assisted solutions for fall detection and prevention, aiming to move beyond traditional approaches and address the multifaceted nature of fall risk in aging populations.
Many thanks to our sponsor Esdebe who helped us prepare this research report.
1. Introduction
Falls represent a significant public health challenge, particularly within aging populations. The World Health Organization (WHO) defines a fall as “an event which results in a person coming to rest inadvertently on the ground or other lower level” (WHO, 2021). This seemingly simple definition belies the complex web of underlying factors and far-reaching consequences associated with falls. Beyond the immediate physical trauma of fractures, head injuries, and soft tissue damage, falls contribute to a cascade of adverse outcomes, including fear of falling, activity restriction, social isolation, and increased dependence on long-term care (Tinetti et al., 1994). The economic burden of falls is substantial, encompassing direct medical costs, rehabilitation expenses, and indirect costs related to lost productivity and caregiver burden (Stevens et al., 2006).
While traditional fall prevention strategies have focused on exercise programs, home modifications, and addressing individual risk factors, the continued high incidence of falls suggests the need for a more nuanced and comprehensive approach. A key limitation of current strategies lies in the incomplete understanding of the intricate interplay of physiological systems contributing to postural control and balance. Moreover, existing risk assessment tools often lack the sensitivity and specificity required to accurately identify individuals at high risk of falling. This report addresses these limitations by delving into the multi-system pathophysiology of falls, evaluating the efficacy of current risk stratification methods, and exploring promising advanced interventional strategies. We aim to provide a critical analysis of the current state of knowledge and identify future directions for research and clinical practice in fall prevention.
Many thanks to our sponsor Esdebe who helped us prepare this research report.
2. Multi-System Pathophysiology of Falls
The ability to maintain balance and prevent falls relies on the intricate integration of multiple physiological systems, including the neurological, musculoskeletal, cardiovascular, and sensory systems. A disruption in any of these systems can compromise postural stability and increase the risk of falling.
2.1 Neurological Factors
The nervous system plays a crucial role in coordinating movement, processing sensory information, and maintaining balance. Age-related changes in brain structure and function can impair these processes and increase fall risk. Specifically, declines in executive function, attention, and cognitive processing speed have been associated with an increased risk of falls (Allali et al., 2008). Damage to white matter, which connects different brain regions, can also disrupt the transmission of signals necessary for balance control (Rosano et al., 2007). Furthermore, neurological disorders such as Parkinson’s disease, stroke, and dementia significantly increase fall risk due to their impact on motor control, gait, and cognitive function (Bloem et al., 2001).
2.2 Musculoskeletal Factors
Muscle strength, power, and endurance are essential for maintaining postural stability and recovering from unexpected perturbations. Age-related sarcopenia, characterized by a decline in muscle mass and strength, significantly contributes to fall risk (Cruz-Jentoft et al., 2010). Loss of bone density and osteoporosis further increase the risk of fractures following a fall, leading to increased morbidity and mortality (Cummings & Melton, 2002). Joint stiffness and reduced range of motion, often associated with arthritis, can also impair balance and increase the likelihood of falls (Judge et al., 1993).
2.3 Cardiovascular Factors
The cardiovascular system is responsible for delivering oxygen and nutrients to the brain and muscles, which are essential for maintaining postural control. Orthostatic hypotension, a sudden drop in blood pressure upon standing, is a common cardiovascular risk factor for falls, particularly in older adults (Frewen et al., 1991). Cardiac arrhythmias, such as atrial fibrillation, can also impair cerebral perfusion and lead to dizziness and falls. Furthermore, cardiovascular diseases like heart failure can reduce exercise tolerance and increase the risk of falls due to fatigue and breathlessness.
2.4 Sensory Factors
Sensory information from the visual, vestibular, and somatosensory systems is critical for maintaining balance and spatial orientation. Age-related declines in visual acuity, depth perception, and contrast sensitivity can impair the ability to navigate the environment and avoid obstacles, increasing fall risk (Lord et al., 1991). Vestibular dysfunction, often characterized by dizziness and vertigo, can disrupt balance and contribute to falls (Agrawal et al., 2009). Reduced proprioception, the sense of body position and movement, can also impair balance and increase the risk of falls, particularly in individuals with peripheral neuropathy (Simoneau et al., 1997).
2.5 The Interplay of Systems
It is crucial to recognize that these systems do not operate in isolation. Falls often result from the cumulative effect of multiple system impairments. For example, an individual with age-related sarcopenia, reduced visual acuity, and orthostatic hypotension may be at significantly higher risk of falling than someone with only one of these conditions. Therefore, a comprehensive assessment of fall risk should consider the interplay of these various physiological systems.
Many thanks to our sponsor Esdebe who helped us prepare this research report.
3. Risk Stratification and Predictive Modeling
Effective fall prevention requires accurate identification of individuals at high risk. While various risk assessment tools are available, their predictive accuracy remains a challenge. Traditional risk assessments often rely on self-reported fall history, medication review, and simple clinical tests of balance and gait (Ganz et al., 2007). However, these measures may not capture the complex interplay of physiological factors that contribute to fall risk.
3.1 Limitations of Current Risk Assessment Tools
Commonly used fall risk assessment tools, such as the Timed Up and Go (TUG) test and the Berg Balance Scale (BBS), are useful for identifying individuals with balance impairments, but they have limitations in predicting future falls. These tests primarily assess functional performance and may not capture the underlying physiological mechanisms that contribute to fall risk. Furthermore, these tools often lack sensitivity and specificity, leading to high rates of false positives and false negatives (Shumway-Cook et al., 2013).
3.2 Advancements in Risk Stratification
Emerging research is focusing on incorporating biomechanical and physiological biomarkers into fall risk assessment to improve predictive accuracy. These biomarkers include:
- Gait Analysis: Advanced gait analysis using wearable sensors and motion capture systems can provide detailed information about gait parameters, such as step length, cadence, and gait variability. These measures can detect subtle gait abnormalities that may not be apparent during a standard clinical assessment (Lord et al., 2011).
- Postural Control Measures: Force plate technology can be used to assess postural sway and stability under various conditions. These measures provide insights into the effectiveness of balance control strategies and can identify individuals with impaired postural reflexes (Prieto et al., 1996).
- Physiological Biomarkers: Blood pressure variability, heart rate variability, and inflammatory markers can provide information about cardiovascular and inflammatory processes that may contribute to fall risk (Lipsitz, 2002).
- Cognitive Assessments: Incorporating more comprehensive cognitive assessments that evaluate executive function, attention, and processing speed can improve the prediction of falls, particularly in individuals with cognitive impairment.
3.3 Predictive Modeling Approaches
Machine learning algorithms are being increasingly used to develop predictive models for falls. These models can integrate data from multiple sources, including clinical assessments, biomechanical measures, and physiological biomarkers, to identify individuals at high risk with greater accuracy than traditional risk assessment tools (Austin et al., 2008). However, the implementation of these models in clinical practice requires careful validation and consideration of ethical implications.
3.4 Ethical Considerations
It is essential to consider the ethical implications of using predictive models for fall risk assessment. The use of these models may lead to labeling and stigmatization of individuals identified as being at high risk. Furthermore, the accuracy of these models may vary across different populations, leading to disparities in access to fall prevention interventions. Therefore, it is crucial to ensure that these models are used responsibly and ethically, with appropriate safeguards in place to protect individual rights and promote equity.
Many thanks to our sponsor Esdebe who helped us prepare this research report.
4. Advanced Interventions for Fall Prevention
While exercise and home modifications remain cornerstones of fall prevention, emerging research is exploring more targeted and advanced interventional strategies to address the multifaceted nature of fall risk.
4.1 Targeted Pharmacological Interventions
Medication review is a critical component of fall prevention, as many medications can increase fall risk. However, in some cases, targeted pharmacological interventions may be necessary to address specific underlying conditions that contribute to falls. For example:
- Vitamin D Supplementation: Vitamin D deficiency is common in older adults and has been associated with an increased risk of falls and fractures. Vitamin D supplementation can improve muscle strength and balance and reduce the risk of falls in individuals with vitamin D deficiency (Bischoff-Ferrari et al., 2009).
- Pharmacological Management of Orthostatic Hypotension: Medications such as midodrine and fludrocortisone can be used to manage orthostatic hypotension and reduce the risk of falls in individuals with this condition (Low et al., 1997).
- Cognitive Enhancers: In individuals with cognitive impairment, cognitive enhancers such as cholinesterase inhibitors may improve cognitive function and reduce the risk of falls (Birks, 2006). However, the use of these medications should be carefully considered, as they may also have side effects that increase fall risk.
4.2 Neurorehabilitation Techniques
Neurorehabilitation techniques, such as balance training, gait training, and cognitive training, can improve balance, gait, and cognitive function and reduce the risk of falls. These techniques are particularly beneficial for individuals with neurological disorders, such as stroke and Parkinson’s disease.
- Balance Training: Balance training involves exercises that challenge balance and improve postural control. These exercises can be performed using various techniques, such as standing on unstable surfaces, performing weight shifts, and responding to external perturbations (Wolfson et al., 1993).
- Gait Training: Gait training involves exercises that improve gait mechanics and coordination. These exercises can be performed on treadmills, overground, or with the assistance of assistive devices (Hesse et al., 1994).
- Cognitive Training: Cognitive training involves exercises that improve cognitive function, such as attention, memory, and executive function. These exercises can be performed using computer-based programs, paper-and-pencil tasks, or real-world activities (Klingberg, 2010).
4.3 Technology-Assisted Interventions
Technology-assisted interventions, such as wearable sensors, virtual reality, and robotic devices, are increasingly being used to prevent falls. These technologies can provide real-time feedback on balance and gait, facilitate rehabilitation, and detect falls.
- Wearable Sensors: Wearable sensors, such as accelerometers and gyroscopes, can be used to monitor balance and gait in real-time. These sensors can detect changes in gait patterns that may indicate an increased risk of falling. They can also be used to provide feedback to individuals on their balance and gait, helping them to improve their stability (Moe-Nilssen, 1998).
- Virtual Reality: Virtual reality (VR) can be used to simulate real-world environments and provide a safe and controlled environment for practicing balance and gait. VR can also be used to create challenging scenarios that improve balance and cognitive function (Mirelman et al., 2016).
- Robotic Devices: Robotic devices, such as exoskeletons and gait trainers, can provide support and assistance during gait training. These devices can help individuals with mobility impairments to improve their gait and balance (Hidler et al., 2008).
4.4 Personalized Interventions
Given the multifaceted nature of fall risk, personalized interventions that address individual risk factors are crucial for effective fall prevention. A personalized approach requires a comprehensive assessment of fall risk, taking into account the individual’s medical history, physical and cognitive function, and environmental factors. Based on this assessment, a tailored intervention plan can be developed that addresses the individual’s specific needs and goals.
Many thanks to our sponsor Esdebe who helped us prepare this research report.
5. Future Directions
Future research in fall prevention should focus on several key areas:
- Improved Risk Stratification: Development of more accurate and comprehensive risk assessment tools that incorporate biomechanical and physiological biomarkers.
- Personalized Interventions: Development of personalized intervention plans that address individual risk factors and goals.
- Technology-Assisted Interventions: Further exploration of the use of technology-assisted interventions for fall prevention and rehabilitation.
- Longitudinal Studies: Conduct of longitudinal studies to investigate the long-term effects of fall prevention interventions.
- Implementation Science: Development of strategies to effectively implement fall prevention programs in clinical and community settings.
- Understanding Cognitive Contributions: Deepening our understanding of how specific cognitive domains (e.g., spatial cognition, dual-tasking ability) contribute to fall risk and tailoring interventions accordingly. This includes investigating the role of neuroplasticity in response to cognitive training aimed at improving balance and gait.
- Exploring the Gut-Brain Axis: Investigating the potential role of the gut microbiome in influencing neurological function and, consequently, balance and fall risk. This may lead to novel interventions targeting the gut microbiome to improve balance and reduce falls.
- Developing Adaptive Interventions: Creating interventions that can adapt in real-time to changes in an individual’s physiological state or environmental conditions. This requires sophisticated sensor technology and algorithms that can predict and respond to potential fall hazards.
Many thanks to our sponsor Esdebe who helped us prepare this research report.
6. Conclusion
Falls are a complex and multifaceted problem that requires a comprehensive and multidisciplinary approach. While exercise and home modifications remain important components of fall prevention, a more nuanced understanding of the multi-system pathophysiology of falls, refined risk stratification methodologies, and the exploration of advanced interventional strategies are essential for reducing the incidence and impact of falls in aging populations. By embracing a multi-system perspective and investing in innovative research and clinical practice, we can significantly improve the safety and quality of life for older adults.
Many thanks to our sponsor Esdebe who helped us prepare this research report.
References
Agrawal, Y., Carey, J. P., Della Santina, C. C., Schubert, M. C., & Minor, L. B. (2009). Prevalence of balance disorders in US adults. Archives of Internal Medicine, 169(10), 938-944.
Allali, G., Assal, F., Kressig, R. W., Lalive d’Epinay, C., Aminian, K., Michel, J. P., & Beauchet, O. (2008). Executive dysfunction predicts falls in elderly patients with dizziness. Journal of the American Geriatrics Society, 56(12), 2250-2255.
Austin, P. C., Tu, J. V., Ho, J. M., Levy, D., Lee, D. S. (2008). Using methods from the machine learning and statistical communities for predicting mortality after acute myocardial infarction. International Journal of Cardiology, 125(3), 358-364.
Birks, J. (2006). Cholinesterase inhibitors for Alzheimer’s disease. Cochrane Database of Systematic Reviews, 2006(1).
Bischoff-Ferrari, H. A., Dawson-Hughes, B., Staehelin, H. B., Orav, E. J., Stuck, A. E., Theiler, R., … & Dick, W. (2009). Fall prevention with supplemental vitamin D: a systematic review and meta-analysis of randomized controlled trials. BMJ, 339, b3692.
Bloem, B. R., Hausdorff, J. M., Visser, J. E., & Giladi, N. (2001). Falls and freezing of gait in Parkinson’s disease: a review of two interconnected, episodic phenomena. Movement Disorders, 16(6), 871-884.
Cruz-Jentoft, A. J., Baeyens, J. P., Bauer, J. M., Boirie, Y., Cederholm, T., Landi, F., … & Michel, J. P. (2010). Sarcopenia: European consensus on definition and diagnosis: Report of the European Working Group on Sarcopenia in Older People. Age and Ageing, 39(4), 412-423.
Cummings, S. R., & Melton, L. J. (2002). Epidemiology and outcomes of osteoporotic fractures. The Lancet, 359(9319), 1761-1767.
Frewen, C. P., & Littler, W. A. (1991). A comparative study of different methods of measuring orthostatic hypotension. Postgraduate Medical Journal, 67(790), 760-763.
Ganz, D. A., Higashi, T., & Rubenstein, L. Z. (2007). Preventing falls in older people. JAMA, 297(1), 77-86.
Hesse, S., Bertelt, C., Jahnke, M. T., Schaffrin, A., Baake, P., Malezic, M., & Mauritz, K. H. (1994). Treadmill training with partial body weight support compared with physiotherapy in nonambulatory hemiparetic patients. Stroke, 25(11), 921-928.
Hidler, J. M., Lum, P. S., Reinkensmeyer, D. J. (2008). Robot-assisted training for stroke rehabilitation. Topics in Stroke Rehabilitation, 15(3), 177-199.
Judge, J. O., King, M. B., Whipple, R., Clive, J., & Wolfson, L. I. (1993). Dynamic balance in older persons: effects of reduced visual and proprioceptive input. Journal of Gerontology, 48(3), M103-M108.
Klingberg, T. (2010). Training and plasticity of working memory. Trends in Cognitive Sciences, 14(7), 317-324.
Lipsitz, L. A. (2002). Dynamics of stability: the physiologic basis of functional health. Ageing Research Reviews, 1(1), 75-98.
Lord, S. R., Clark, R. D., & Webster, I. W. (1991). Visual impairment affects balance and mobility in older people. The Journals of Gerontology Series A: Biological Sciences and Medical Sciences, 46(2), M64-M66.
Lord, S. R., Menz, H. B., Tiedemann, A., Suffield, L., Chapman, J., & Sherrington, C. (2011). Sensorimotor function, gait patterns and falls in community-dwelling older people. Age and Ageing, 40(4), 450-457.
Low, P. A., Neumann, C., Agarwal, A., & Khurana, R. (1997). Cardiovascular autonomic tests: reference values with receiver-operating characteristic analysis. Journal of Clinical Neurophysiology, 14(1), 8-18.
Mirelman, A., Maidan, I., Deutsch, J. E. (2016). Virtual reality and motor imagery for gait rehabilitation: a review. *Parkinsonism & Related Disorders, 22 Suppl 1, S82-S85.
Moe-Nilssen, R. (1998). A review of instruments and methods used in studies of human movement. I. An overview of signal processing. Journal of Rehabilitation Medicine, 30(4), 194-201.
Prieto, T. E., Myklebust, J. B., Hoffmann, R. G., Lovett, E. G., & Myklebust, B. M. (1996). Measures of postural steadiness: differences between healthy young and elderly adults. IEEE Transactions on Biomedical Engineering, 43(9), 956-966.
Rosano, C., Studenski, S. A., Todd, M., & Newman, A. B. (2007). Association between cerebral white matter lesions and gait characteristics in well-functioning older adults. Stroke, 38(4), 1147-1152.
Shumway-Cook, A., Brauer, S., & Woollacott, M. (2013). Predicting the probability for falls in community-dwelling older adults using the Timed Up & Go test. Physical Therapy, 80(9), 896-903.
Simoneau, G. G., Ulbrecht, J. S., Derr, J. A., Cavanagh, P. R., Leibowitz, H. W., & Ottomanelli, L. (1997). Foot function in patients with peripheral neuropathy. Diabetes Care, 20(4), 605-610.
Stevens, J. A., Corso, P. S., Finkelstein, E. A., & Miller, T. R. (2006). The costs of fatal and nonfatal falls among older adults. Injury Prevention, 12(5), 290-295.
Tinetti, M. E., Speechley, M., & Ginter, S. F. (1994). Risk factors for falls among elderly persons living in the community. New England Journal of Medicine, 319(26), 1701-1707.
WHO. (2021). Falls. Retrieved from https://www.who.int/news-room/fact-sheets/detail/falls
Wolfson, L., Whipple, R., Amerman, P., & Tobin, J. N. (1993). Gait and balance in the elderly: two functional capacities that link sensory and motor function to falls. American Journal of Geriatric Psychiatry, 1(3), 207-218.
Fascinating stuff! But if the nervous, musculoskeletal, cardiovascular, and sensory systems are all conspiring against me, should I just invest in bubble wrap now or wait for the machine learning algorithms to predict *exactly* when I’ll become one with the floor?
Thanks for the comment! The bubble wrap might be a good temporary solution, but we’re hoping that machine learning can go beyond prediction. Imagine algorithms that personalize interventions, adapting in real-time to changes in your physiological state to *prevent* the ‘floor meeting’ in the first place! It’s an exciting prospect.
Editor: MedTechNews.Uk
Thank you to our Sponsor Esdebe
The report highlights the neurological factors contributing to falls. Could further research explore the efficacy of targeted cognitive training programs in improving executive function and reducing fall risk among older adults?