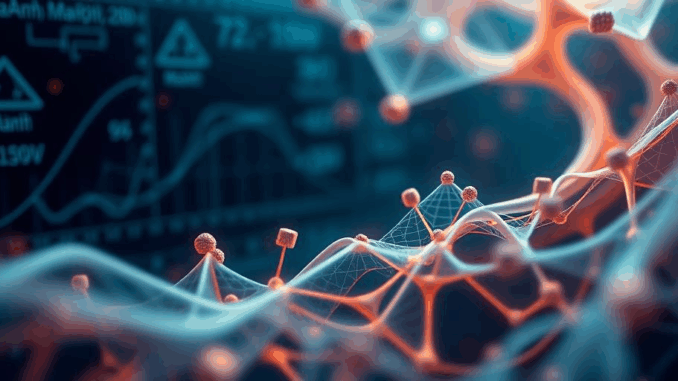
Mathematical Modeling of Disease: A Comprehensive Overview of Approaches, Challenges, and Future Directions
Many thanks to our sponsor Esdebe who helped us prepare this research report.
Abstract
Mathematical modeling has emerged as a powerful tool for understanding, predicting, and controlling the spread and impact of disease. This research report provides a comprehensive overview of the diverse mathematical modeling approaches employed in disease research, encompassing both infectious and chronic diseases. We explore the fundamental principles underpinning these models, including compartmental models, agent-based models, and network models. Furthermore, we discuss the crucial role of data in model development, validation, and refinement, highlighting the challenges associated with data acquisition, quality, and interpretation. We delve into the applications of mathematical models in informing public health interventions, treatment strategies, and drug development. Finally, we address the limitations of current models and outline promising avenues for future research, including the integration of multi-scale data, the development of personalized models, and the incorporation of behavioral and social factors into disease modeling frameworks.
Many thanks to our sponsor Esdebe who helped us prepare this research report.
1. Introduction
Disease, a deviation from the normal structural or functional state of an organism, has been a persistent challenge throughout human history. Understanding the complex dynamics of disease, whether infectious or chronic, is crucial for developing effective strategies for prevention, treatment, and control. Mathematical modeling offers a powerful framework for representing and analyzing these dynamics, providing insights that can inform public health policy, clinical decision-making, and drug development.
The application of mathematical models to disease research dates back to the early 20th century, with the seminal work of Kermack and McKendrick (1927) on compartmental models for infectious disease transmission. Since then, the field has witnessed significant advancements, driven by increased computational power, the availability of larger and more diverse datasets, and the development of novel modeling techniques. Today, mathematical models are used to study a wide range of diseases, from highly infectious outbreaks like Ebola and COVID-19 to chronic conditions such as cancer and cardiovascular disease.
This report provides a comprehensive overview of the application of mathematical modeling to disease. We will explore the different types of models used, the data required to build and validate them, and the challenges and opportunities in this rapidly evolving field. We aim to provide a resource for researchers and practitioners interested in using mathematical modeling to better understand and manage disease.
Many thanks to our sponsor Esdebe who helped us prepare this research report.
2. Types of Mathematical Models for Disease
A variety of mathematical models are employed to study disease, each with its own strengths and weaknesses. The choice of model depends on the specific disease, the research question, and the available data.
2.1 Compartmental Models
Compartmental models are perhaps the most widely used type of mathematical model for infectious disease. These models divide the population into compartments based on their disease status (e.g., susceptible, infected, recovered) and use differential equations to describe the flow of individuals between these compartments. The simplest compartmental model is the SIR model, which divides the population into three compartments: Susceptible (S), Infected (I), and Recovered (R). The model assumes that individuals move from the S compartment to the I compartment upon infection, and then from the I compartment to the R compartment upon recovery. More complex compartmental models can incorporate additional compartments, such as exposed (E), vaccinated (V), or deceased (D), to account for more nuanced aspects of disease transmission. These are commonly referred to as SEIR, SEIV or SEIRD models.
The SIR model and its variations are useful for understanding the basic dynamics of infectious disease outbreaks, such as the peak of the epidemic and the proportion of the population that will be infected. However, they have limitations. Compartmental models typically assume that the population is homogeneously mixed, meaning that everyone has an equal chance of interacting with everyone else. This assumption is often unrealistic, as individuals tend to interact more frequently with people in their own social circles or geographic locations. Furthermore, compartmental models typically do not account for individual-level heterogeneity in susceptibility, infectivity, or recovery rates.
2.2 Agent-Based Models
Agent-based models (ABMs) are a more sophisticated approach that explicitly represents individual agents and their interactions. Each agent has its own set of attributes (e.g., age, sex, location, health status) and follows a set of rules that govern its behavior. ABMs can simulate the spread of disease through a population by tracking the interactions between individual agents. This allows for the incorporation of heterogeneity in susceptibility, infectivity, and contact patterns. For example, an ABM could be used to simulate the spread of influenza in a city, taking into account the different commuting patterns of different individuals, the types of contacts they make in different settings (e.g., home, work, school), and their individual vaccination status.
ABMs offer several advantages over compartmental models. They can capture the effects of heterogeneity and spatial structure on disease transmission, and they can be used to simulate the impact of interventions that target specific individuals or groups. However, ABMs are computationally more demanding than compartmental models, and they require more detailed data on individual behavior and interactions. Furthermore, the calibration and validation of ABMs can be challenging, as it is difficult to directly observe the behavior of all agents in the model.
2.3 Network Models
Network models provide a way to represent the social structure of a population and how it influences disease transmission. In a network model, individuals are represented as nodes, and their contacts are represented as edges. The structure of the network can have a significant impact on the spread of disease. For example, diseases tend to spread more rapidly in networks with high levels of connectivity, where individuals have many contacts.
Network models can be used to study the impact of different network structures on disease transmission, and to identify individuals or groups that are particularly important for spreading or containing disease. For example, network models have been used to study the spread of HIV/AIDS among intravenous drug users, and to identify strategies for targeting interventions to individuals with high levels of social connectivity.
2.4 Statistical Models
Statistical models play a crucial role in analyzing epidemiological data and understanding disease patterns. These models can be used to identify risk factors for disease, estimate the incidence and prevalence of disease, and assess the effectiveness of interventions. Regression models, such as logistic regression and Cox proportional hazards regression, are commonly used to analyze the relationship between risk factors and disease outcomes. Time series models can be used to analyze trends in disease incidence over time. Spatial statistical models can be used to analyze the geographic distribution of disease.
2.5 Models for Chronic Diseases
The models described above are often used for infectious diseases but are applicable with modification to chronic disease. Chronic diseases, such as cancer, diabetes, and cardiovascular disease, pose different modeling challenges compared to infectious diseases. Unlike infectious diseases, chronic diseases typically develop over long periods of time and are influenced by a complex interplay of genetic, environmental, and lifestyle factors. Consequently, mathematical models for chronic diseases often focus on understanding the pathogenesis of the disease, identifying risk factors, and evaluating the effectiveness of interventions aimed at preventing or delaying disease progression.
Systems biology models are often used to study the molecular mechanisms underlying chronic diseases. These models integrate data from genomics, proteomics, and metabolomics to create comprehensive representations of cellular processes and pathways. Systems biology models can be used to identify potential drug targets and to predict the response of patients to different treatments.
Many thanks to our sponsor Esdebe who helped us prepare this research report.
3. Data Requirements and Challenges
The development and validation of mathematical models require a variety of data sources. The specific data requirements depend on the type of model being used and the research question being addressed. Generally, the following types of data are needed:
- Epidemiological data: This includes data on the incidence and prevalence of disease, the age and sex distribution of cases, and the geographic distribution of cases. This data is essential for calibrating and validating models of disease transmission.
- Demographic data: This includes data on population size, age structure, and migration patterns. This data is needed to accurately represent the population in the model.
- Behavioral data: This includes data on human behavior that can influence disease transmission, such as contact patterns, hygiene practices, and vaccination rates. This data is often difficult to obtain, but it is crucial for understanding the impact of human behavior on disease dynamics.
- Clinical data: This includes data on disease progression, treatment outcomes, and drug resistance. This data is needed to develop and validate models of disease progression and treatment response.
- Biological data: This includes data on the pathogen, the immune response, and the host genetics. This data is needed to develop and validate models of disease pathogenesis and immunity.
Obtaining and interpreting data for disease modeling can be challenging for several reasons. Firstly, data may be incomplete or inaccurate. For example, disease surveillance systems may not capture all cases of disease, or individuals may be reluctant to report their symptoms. Secondly, data may be collected in different ways in different settings, making it difficult to compare data across populations or time periods. Thirdly, data may be subject to bias. For example, individuals who are more likely to seek medical care may be overrepresented in disease surveillance data. Finally, there may be privacy concerns associated with collecting and sharing data on individuals’ health status. The European GDPR rules can severely restrict use of some datasets for example.
Many thanks to our sponsor Esdebe who helped us prepare this research report.
4. Applications of Mathematical Models in Disease Research
Mathematical models have a wide range of applications in disease research. These include:
- Understanding disease dynamics: Mathematical models can be used to understand the underlying mechanisms that drive disease transmission and progression. This can help researchers to identify key factors that influence the spread of disease and to develop more effective interventions.
- Predicting disease outbreaks: Mathematical models can be used to forecast the timing and magnitude of disease outbreaks. This can help public health officials to prepare for outbreaks and to implement timely interventions.
- Evaluating interventions: Mathematical models can be used to evaluate the impact of different interventions on disease transmission and progression. This can help policymakers to make informed decisions about which interventions to implement.
- Optimizing treatment strategies: Mathematical models can be used to optimize treatment strategies for individual patients. This can help clinicians to personalize treatment plans and to improve patient outcomes.
- Drug discovery and development: Mathematical models can be used to identify potential drug targets and to predict the response of patients to different drugs. This can help pharmaceutical companies to develop more effective drugs.
Examples of successful applications of mathematical models in disease research include:
- The use of compartmental models to predict the spread of HIV/AIDS and to evaluate the impact of different prevention strategies.
- The use of agent-based models to simulate the spread of influenza and to optimize vaccination campaigns.
- The use of network models to study the spread of sexually transmitted infections and to identify individuals at high risk of infection.
- The use of systems biology models to study the pathogenesis of cancer and to identify potential drug targets.
Many thanks to our sponsor Esdebe who helped us prepare this research report.
5. Limitations and Future Directions
While mathematical models are a powerful tool for studying disease, they have several limitations. Some of the most important limitations include:
- Model complexity: Mathematical models are simplifications of reality. They cannot capture all of the complexity of biological systems or human behavior. This means that model predictions may not always be accurate.
- Data availability: Mathematical models require data to be calibrated and validated. However, data may not always be available or accurate, particularly for emerging infectious diseases or for diseases that are difficult to diagnose.
- Computational limitations: Some mathematical models, such as agent-based models, can be computationally demanding. This can limit the size and complexity of the models that can be developed.
- Uncertainty: There is always uncertainty associated with model predictions. This is because models are based on assumptions and data that may not be completely accurate.
Despite these limitations, mathematical models are becoming increasingly sophisticated and powerful. Several promising avenues for future research include:
- Multi-scale modeling: Integrating models that operate at different scales, from the molecular level to the population level, to create more comprehensive representations of disease dynamics.
- Personalized modeling: Developing models that are tailored to individual patients, based on their genetic makeup, lifestyle, and medical history. This could lead to more personalized treatment strategies.
- Incorporating behavioral and social factors: Incorporating behavioral and social factors into disease models to better understand the impact of human behavior on disease transmission and progression. This could lead to more effective interventions that promote healthy behaviors.
- Improving data collection and sharing: Developing new methods for collecting and sharing data on disease, including the use of mobile technologies and electronic health records. This could improve the accuracy and completeness of data used for disease modeling.
- Addressing model uncertainty: Developing methods for quantifying and communicating the uncertainty associated with model predictions. This could help policymakers to make more informed decisions about how to use model results.
Many thanks to our sponsor Esdebe who helped us prepare this research report.
6. Conclusion
Mathematical modeling plays a crucial role in understanding, predicting, and controlling disease. The field encompasses a diverse range of approaches, from simple compartmental models to complex agent-based and network models. The accuracy and reliability of these models depend heavily on the availability and quality of data. As computational power increases and data collection methods improve, mathematical models are becoming increasingly sophisticated and are playing an increasingly important role in public health decision-making, clinical practice, and drug development. Future research should focus on integrating multi-scale data, developing personalized models, incorporating behavioral and social factors, and addressing model uncertainty to further enhance the utility of mathematical modeling in combating disease. The evolution of computational techniques and model design is only going to increase the importance of the role that mathematical models play in the fight against disease.
Many thanks to our sponsor Esdebe who helped us prepare this research report.
References
- Anderson, R. M., & May, R. M. (1991). Infectious diseases of humans: dynamics and control. Oxford university press.
- Brauer, F., Castillo-Chavez, C., & Kirschner, D. (2019). Mathematical models in epidemiology. Springer.
- Diekmann, O., & Heesterbeek, J. A. P. (2000). Mathematical epidemiology of infectious diseases: model building, analysis and interpretation. John Wiley & Sons.
- Keeling, M. J., & Rohani, P. (2008). Modeling infectious diseases in humans and animals. Princeton University Press.
- Kermack, W. O., & McKendrick, A. G. (1927). A contribution to the mathematical theory of epidemics. Proceedings of the Royal Society of London. Series A, Containing Papers of a Mathematical or Physical Character, 115(772), 700-721.
- Murray, J. D. (2007). Mathematical biology I: An introduction. Springer Science & Business Media.
- Ozguler, M. U. (2022). Modeling infectious diseases. Frontiers in public health, 10, 913558.
- Riley, S. (2007). Mathematical modelling of infectious disease dynamics. The BMJ, 335(7630), 1216-1221.
- Vespignani, A. (2012). Modelling dynamical processes in complex socio-technical systems. Nature Physics, 8(1), 32-39.
Interesting report. The call for incorporating behavioral and social factors into disease modeling is critical. How can we best integrate qualitative research methods with quantitative models to capture nuanced human responses to public health interventions?
Thanks for your insightful comment! Integrating qualitative research is key. Perhaps incorporating ethnographic studies to understand community-specific beliefs and practices can inform agent-based models, creating more realistic simulations of intervention impact. This mixed-methods approach could significantly enhance model accuracy and relevance.
Editor: MedTechNews.Uk
Thank you to our Sponsor Esdebe