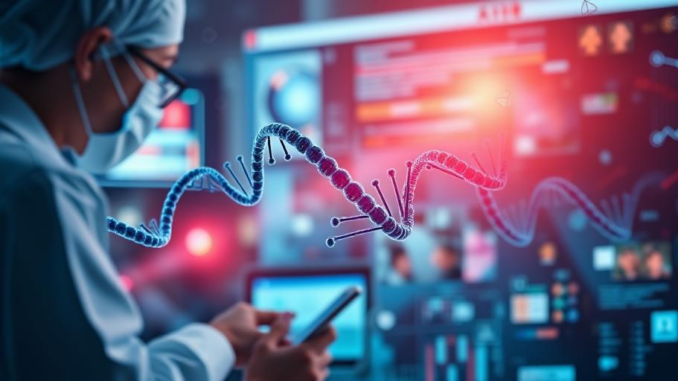
Personalized Medicine: A Comprehensive Overview of Applications, Challenges, and Future Directions
Abstract
Personalized medicine, also known as precision medicine, represents a paradigm shift in healthcare, moving away from a ‘one-size-fits-all’ approach towards tailored interventions based on an individual’s unique genetic, environmental, and lifestyle factors. This report provides a comprehensive overview of personalized medicine, encompassing its foundational principles, diverse applications across various medical fields, the technological advancements driving its progress, and the multifaceted challenges hindering its widespread implementation. We delve into the ethical, legal, and social implications (ELSI) of personalized medicine, particularly concerning data privacy, genetic discrimination, and equitable access. Furthermore, we explore future directions in personalized medicine, including the integration of multi-omics data, the development of sophisticated predictive models using artificial intelligence, and the evolving role of patient-generated health data. The report aims to provide a nuanced understanding of the potential and limitations of personalized medicine, highlighting the need for collaborative efforts among researchers, clinicians, policymakers, and patients to realize its full promise and address its associated complexities.
1. Introduction
The traditional approach to medicine often relies on standardized treatments based on population averages. While effective for many, this method inevitably leads to suboptimal outcomes for a significant proportion of patients, owing to individual variations in disease etiology, drug response, and overall health profile. Personalized medicine aims to overcome these limitations by leveraging an individual’s unique characteristics to guide diagnosis, treatment selection, and prevention strategies. This approach encompasses a wide range of factors, including genetic makeup, environmental exposures, lifestyle choices, and clinical history, to create a more tailored and effective healthcare experience.
The concept of personalized medicine has evolved significantly over the past few decades, fueled by advancements in genomics, proteomics, metabolomics, and other high-throughput technologies. The completion of the Human Genome Project in 2003 marked a pivotal moment, providing a comprehensive map of human genes and paving the way for large-scale genetic studies. Subsequently, the development of next-generation sequencing (NGS) technologies has revolutionized the field, enabling rapid and cost-effective analysis of individual genomes. These technological advancements have facilitated the identification of genetic variants associated with disease risk, drug response, and other clinically relevant traits.
Beyond genomics, personalized medicine also incorporates other ‘omics’ disciplines, such as proteomics (the study of proteins), metabolomics (the study of metabolites), and transcriptomics (the study of RNA). These ‘omics’ technologies provide complementary information about an individual’s biological state, offering a more holistic view of disease mechanisms and treatment targets. The integration of multi-omics data, along with clinical and lifestyle information, holds immense promise for developing highly personalized and effective interventions.
2. Applications of Personalized Medicine
Personalized medicine has diverse applications across various medical fields, including but not limited to oncology, cardiology, infectious diseases, and pharmacogenomics. Some of the most prominent applications are detailed below:
2.1 Oncology:
Cancer, characterized by uncontrolled cell growth and genetic instability, is a prime target for personalized medicine. Genomic profiling of tumors can identify specific mutations that drive cancer growth, allowing for the selection of targeted therapies that specifically inhibit these mutations. For instance, in non-small cell lung cancer (NSCLC), the identification of mutations in the epidermal growth factor receptor (EGFR) gene has led to the development of EGFR tyrosine kinase inhibitors (TKIs), which have significantly improved outcomes for patients with these mutations. Similarly, the identification of mutations in the BRAF gene in melanoma has led to the development of BRAF inhibitors, which have demonstrated remarkable efficacy in treating patients with BRAF-mutated melanoma [1].
Liquid biopsies, which involve analyzing circulating tumor cells (CTCs) or circulating tumor DNA (ctDNA) in blood samples, offer a non-invasive method for monitoring treatment response and detecting resistance mechanisms. Liquid biopsies can also be used to identify actionable mutations in patients for whom a tumor biopsy is not feasible or available [2].
Immunotherapy, which harnesses the power of the immune system to fight cancer, has also benefited from personalized medicine approaches. Biomarkers such as PD-L1 expression and microsatellite instability (MSI) can be used to predict which patients are most likely to respond to immune checkpoint inhibitors [3].
2.2 Cardiology:
Personalized medicine has the potential to revolutionize the prevention, diagnosis, and treatment of cardiovascular diseases, which remain a leading cause of mortality worldwide. Genetic testing can identify individuals at increased risk of developing cardiovascular diseases, such as familial hypercholesterolemia, hypertrophic cardiomyopathy, and long QT syndrome. Early identification of these individuals allows for the implementation of preventive measures, such as lifestyle modifications and medications, to reduce their risk of developing these conditions.
Pharmacogenomics plays a crucial role in optimizing drug therapy for cardiovascular diseases. For example, genetic variants in the CYP2C19 gene influence the metabolism of clopidogrel, an antiplatelet drug commonly used to prevent blood clots after a heart attack or stroke. Patients with certain CYP2C19 variants may have reduced clopidogrel efficacy, increasing their risk of adverse cardiovascular events. Genetic testing for CYP2C19 variants can help clinicians select the most appropriate antiplatelet therapy for individual patients [4].
2.3 Infectious Diseases:
Personalized medicine can be applied to infectious diseases in several ways, including identifying individuals at increased risk of infection, predicting disease severity, and optimizing treatment strategies. For example, genetic variants in the CCR5 gene influence susceptibility to HIV infection. Individuals with a specific CCR5 variant (CCR5-Δ32) are resistant to HIV infection, as this variant prevents the virus from entering immune cells [5].
Pharmacogenomics can also play a role in optimizing antiviral therapy. For example, genetic variants in the IL28B gene influence the response to interferon-based therapy for hepatitis C virus (HCV) infection. Patients with certain IL28B variants are more likely to achieve sustained virologic response (SVR) to interferon-based therapy [6].
2.4 Pharmacogenomics:
Pharmacogenomics is the study of how genes affect a person’s response to drugs. The goal of pharmacogenomics is to develop personalized drug therapies that are tailored to an individual’s genetic makeup. As described in the cardiology and infectious disease sections, genetic variations can significantly impact drug metabolism, efficacy, and toxicity. By identifying these variations, clinicians can select the most appropriate drug and dosage for each patient, minimizing adverse effects and maximizing therapeutic benefit. This is particularly relevant for drugs with narrow therapeutic indices, where even small variations in drug concentration can have significant consequences.
3. Technological Advancements Driving Personalized Medicine
Several technological advancements have been instrumental in driving the progress of personalized medicine:
3.1 Next-Generation Sequencing (NGS):
NGS technologies have revolutionized genomic research and clinical diagnostics, enabling rapid and cost-effective sequencing of entire genomes or targeted gene panels. NGS has facilitated the identification of genetic variants associated with disease risk, drug response, and other clinically relevant traits. The decreasing cost of NGS has made it increasingly accessible for clinical use, paving the way for widespread implementation of personalized medicine approaches [7].
3.2 Bioinformatics and Data Analytics:
The vast amount of data generated by NGS and other ‘omics’ technologies requires sophisticated bioinformatics tools and data analytics techniques for processing, analysis, and interpretation. Bioinformatics algorithms are used to align sequencing reads, identify genetic variants, and annotate genes. Data analytics techniques, such as machine learning and statistical modeling, are used to identify patterns and correlations in large datasets, enabling the development of predictive models for disease risk and treatment response [8].
3.3 Artificial Intelligence (AI) and Machine Learning (ML):
AI and ML are playing an increasingly important role in personalized medicine, enabling the development of sophisticated predictive models for disease risk, diagnosis, and treatment response. AI algorithms can analyze large datasets of clinical, genomic, and other ‘omics’ data to identify patterns that are not readily apparent to human researchers. ML models can be trained to predict which patients are most likely to respond to a particular treatment, allowing for the selection of the most effective therapy for each individual [9].
3.4 Electronic Health Records (EHRs):
EHRs are digital repositories of patient health information, including clinical history, laboratory results, medications, and imaging data. EHRs are an essential component of personalized medicine, providing a comprehensive source of information that can be integrated with genomic and other ‘omics’ data to guide clinical decision-making. The interoperability of EHRs is crucial for facilitating data sharing and collaboration among healthcare providers and researchers [10].
4. Challenges in Implementing Personalized Medicine
Despite its immense potential, personalized medicine faces several challenges that hinder its widespread implementation:
4.1 Data Privacy and Security:
The collection and storage of sensitive genetic and clinical data raise significant concerns about data privacy and security. Robust security measures are needed to protect patient data from unauthorized access, use, or disclosure. Regulations such as the Health Insurance Portability and Accountability Act (HIPAA) in the United States and the General Data Protection Regulation (GDPR) in Europe provide a framework for protecting patient data privacy, but ongoing vigilance and adaptation are necessary to address evolving cybersecurity threats [11].
4.2 Genetic Discrimination:
There is a risk that genetic information could be used to discriminate against individuals in areas such as employment, insurance, and education. The Genetic Information Nondiscrimination Act (GINA) in the United States prohibits genetic discrimination in employment and health insurance, but similar protections are needed in other countries and in other areas, such as life insurance and disability insurance [12].
4.3 Ethical, Legal, and Social Implications (ELSI):
Personalized medicine raises a number of complex ELSI issues, including informed consent, data ownership, and equitable access. It is crucial to engage in open and transparent discussions about these issues to ensure that personalized medicine is implemented in a responsible and ethical manner. Ensuring informed consent for genetic testing and data sharing is particularly challenging, as individuals may not fully understand the implications of these procedures. Furthermore, the potential for exacerbating health disparities due to differential access to personalized medicine technologies must be carefully addressed [13].
4.4 Cost and Reimbursement:
The cost of genomic sequencing and other ‘omics’ technologies can be a barrier to widespread adoption of personalized medicine. Developing cost-effective strategies for implementing personalized medicine approaches is essential. Furthermore, clear reimbursement policies are needed to ensure that patients have access to these technologies. Currently, reimbursement for personalized medicine services varies widely across different healthcare systems and countries [14].
4.5 Lack of Clinical Utility:
While many genetic variants have been associated with disease risk and drug response, the clinical utility of this information is not always clear. More research is needed to determine which genetic variants are most clinically relevant and how this information can be used to improve patient outcomes. Establishing clinical utility requires rigorous validation studies and the development of clear guidelines for clinical implementation [15].
4.6 Education and Training:
Healthcare professionals need to be educated and trained in the principles and applications of personalized medicine. This includes understanding genomics, proteomics, metabolomics, and other ‘omics’ technologies, as well as the ethical and legal implications of personalized medicine. Integrating personalized medicine into medical school curricula and providing continuing education opportunities for practicing physicians are essential steps in addressing this challenge [16].
4.7 Data Integration and Interoperability:
The integration of genomic, clinical, and lifestyle data is essential for personalized medicine, but this requires overcoming challenges related to data standardization, interoperability, and sharing. Developing common data standards and promoting data sharing among healthcare providers and researchers are crucial for realizing the full potential of personalized medicine. Furthermore, ensuring the security and privacy of data during sharing is paramount [17].
5. Future Directions
Personalized medicine is a rapidly evolving field, and several exciting developments are on the horizon:
5.1 Integration of Multi-Omics Data:
The integration of genomic, proteomic, metabolomic, and transcriptomic data holds immense promise for developing a more holistic understanding of disease mechanisms and treatment targets. Multi-omics data can provide a more complete picture of an individual’s biological state, allowing for the development of highly personalized interventions. Sophisticated bioinformatics tools and data analytics techniques are needed to integrate and analyze these complex datasets [18].
5.2 Development of Sophisticated Predictive Models Using AI:
AI and ML are poised to play an increasingly important role in personalized medicine, enabling the development of sophisticated predictive models for disease risk, diagnosis, and treatment response. AI algorithms can analyze large datasets of clinical, genomic, and other ‘omics’ data to identify patterns that are not readily apparent to human researchers. These models can be used to personalize treatment decisions, predict disease progression, and identify individuals at high risk of developing specific diseases [19].
5.3 Evolving Role of Patient-Generated Health Data:
Patient-generated health data (PGHD), such as data from wearable devices and mobile apps, can provide valuable insights into an individual’s health status and lifestyle. Integrating PGHD with clinical and genomic data can provide a more comprehensive picture of an individual’s health profile, allowing for the development of more personalized interventions. However, ensuring the accuracy and reliability of PGHD and addressing concerns about data privacy are important considerations [20].
5.4 CRISPR-Cas9 Gene Editing:
The CRISPR-Cas9 gene editing technology has the potential to revolutionize the treatment of genetic diseases. CRISPR-Cas9 allows for precise editing of DNA sequences, enabling the correction of disease-causing mutations. While CRISPR-Cas9 is still in its early stages of development, it holds immense promise for treating a wide range of genetic disorders. Ethical considerations surrounding the use of CRISPR-Cas9, particularly in germline editing, need to be carefully addressed [21].
5.5 Personalized Vaccines:
Personalized vaccines are being developed for cancer and infectious diseases. These vaccines are designed to target specific mutations or antigens that are unique to an individual’s tumor or pathogen. Personalized cancer vaccines have shown promising results in clinical trials, demonstrating the potential to stimulate an immune response against cancer cells. Personalized vaccines for infectious diseases could be used to protect against emerging pathogens and to overcome vaccine resistance [22].
6. Conclusion
Personalized medicine represents a transformative approach to healthcare, offering the potential to tailor interventions based on an individual’s unique characteristics. Technological advancements, particularly in genomics and data analytics, have fueled the progress of personalized medicine, leading to diverse applications across various medical fields. However, significant challenges remain, including data privacy, genetic discrimination, ethical considerations, cost, and the need for clinical utility. Addressing these challenges requires collaborative efforts among researchers, clinicians, policymakers, and patients.
The future of personalized medicine is bright, with exciting developments on the horizon, such as the integration of multi-omics data, the development of sophisticated predictive models using AI, and the evolving role of patient-generated health data. By embracing these advancements and addressing the associated challenges, we can unlock the full potential of personalized medicine to improve patient outcomes and transform healthcare.
References
[1] Dunn, S. E., & Heemels, A. (2011). The biology of EGFR and its relevance to cancer treatment. Oncogene, 30(3), 289-298.
[2] Diaz Jr, L. A., & Bardelli, A. (2014). Liquid biopsies: genotyping circulating tumor DNA. Journal of Clinical Oncology, 32(6), 579.
[3] Topalian, S. L., Hodi, F. S., Brahmer, J. R., Gettinger, S. N., Smith, D. C., McDermott, D. F., … & Pardoll, D. M. (2012). Safety, activity, and immune correlates of anti-PD-1 antibody in cancer. New England Journal of Medicine, 366(26), 2443-2454.
[4] Mega, J. L., Simon, T., Collet, J. P., Anderson, J. L., Antman, E. M., Bliden, K. P., … & Sabatine, M. S. (2009). Reduced-function CYP2C19 genotype and risk of adverse clinical outcomes among patients treated with clopidogrel for acute coronary syndromes. Jama, 301(21), 2227-2236.
[5] Dean, M., Carrington, M., Winkler, C., Huttley, G. A., Smith, M. W., Allikmets, R., … & O’brien, S. J. (1996). Genetic restriction of HIV-1 infection by the CCR5 Δ32/Δ32 homozygo us. Science, 273(5283), 1856-1862.
[6] Ge, D., Fellay, J., Thompson, A. J., Simon, J. S., Shianna, K. V., Urban, T. J., … & Goldstein, D. B. (2009). Genetic variation in IL28B predicts hepatitis C treatment-induced viral clearance. Nature, 461(7262), 399-401.
[7] Goodwin, S., McPherson, J. D., McCombie, W. R. (2016). Coming of age: ten years of next-generation sequencing technologies. Nature Reviews Genetics, 17, 333-351.
[8] Luscombe, N. M., Greenbaum, D., Gerstein, M. (2001). What is bioinformatics? A proposed definition and overview of the field. Methods of Information in Medicine, 40(4), 346-358.
[9] Obermeyer, Z., & Emanuel, E. J. (2016). Predicting the future—big data, machine learning, and clinical medicine. New England Journal of Medicine, 375(13), 1216-1219.
[10] Murff, H. J., & FitzHenry, F. (2011). Electronic health record (EHR) utility. Annual review of public health, 32, 255-276.
[11] Hyman, S. E. (2016). Data sharing: Promoting better science. Neuron, 92(4), 723-725.
[12] Hudson, K. L., Holohan, M. K., & Collins, F. S. (2008). Keeping pace with the times—the Genetic Information Nondiscrimination Act of 2008. New England Journal of Medicine, 358(25), 2661-2663.
[13] Caulfield, T., & Rachul, C. (2016). Precision medicine: a critical perspective. Genome medicine, 8(1), 8.
[14] Phillips, K. A., Annas, G. J., & Kahn, J. P. (2015). Delivering personalized medicine: the challenges and opportunities for translating genomics into healthcare. Health affairs, 34(9), 1412-1419.
[15] Khoury, M. J., Gwinn, M., Ioannidis, J. P. A., & Dowling, N. F. (2007). The emergence of translational epidemiology: from genetic association to population impact. American journal of epidemiology, 166(1), 1-5.
[16] Ginsburg, G. S., & Willard, H. F. (2009). Genomic and personalized medicine: foundations and applications. Translation Research, 154(1), 1-6.
[17] Bellazzi, R., & Zupan, B. (2008). Data mining and data fusion for data integration in systems biology. Journal of biomedical informatics, 41(6), 853-861.
[18] Hasin, Y., Seldin, M., & Lusis, A. (2017). Multi-omics approaches to disease. Genome medicine, 9(1), 39.
[19] Esteva, A., Kuprel, B., Novoa, R. A., Ko, J., Swani, S. M., Blau, H. M., … & Threlfall, C. J. (2017). Dermatologist-level classification of skin cancer with deep neural networks. Nature, 542(7639), 115-118.
[20] Lupton, D. (2013). Quantified self. Sociology Compass, 7(8), 666-678.
[21] Doudna, J. A., & Charpentier, E. (2014). The new frontier of genome engineering with CRISPR-Cas9. Science, 346(6213), 1258096.
[22] Sahin, U., & Türeci, Ö. (2018). Personalized cancer vaccines. Cancer immunology research, 6(2), 138-147.
So, beyond the ‘omics’ alphabet soup, what’s the plan for those of us whose lifestyles already involve questionable choices and environmental exposures? Do I get a personalized kale smoothie prescription based on my DNA, or just more unsolicited advice from my doctor?
That’s a fantastic point! While personalized medicine looks at the ‘omics,’ integrating lifestyle and environmental data is key. Imagine AI providing tailored recommendations based on *your* choices, working *with* your doctor to fine-tune, instead of replace, their expertise. A personalized approach to health, even if it includes the occasional less-than-perfect choice!
Editor: MedTechNews.Uk
Thank you to our Sponsor Esdebe
So, if my Fitbit knows I only walked to the fridge and back, will my “personalized” health plan just involve moving the fridge closer to the couch? Asking for a friend… obviously.
That’s a hilarious, but relevant, point! While moving the fridge might seem like a quick fix, the real power lies in integrating that data with other lifestyle factors. Think of it as the first step in a personalized nudge system, encouraging healthier choices over time. Perhaps a personalized workout routine is in order!
Editor: MedTechNews.Uk
Thank you to our Sponsor Esdebe