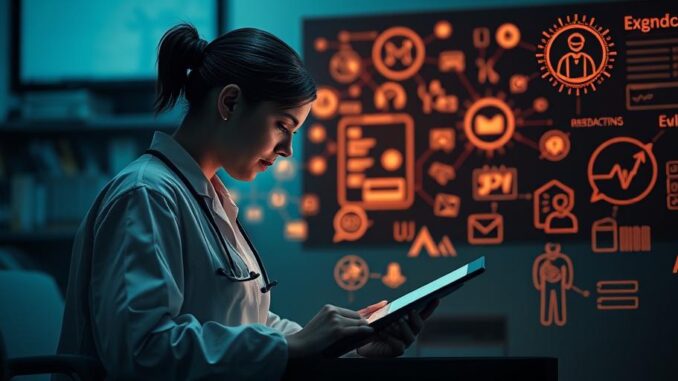
Reimagining Medical Education: A Comprehensive Exploration of Curriculum Innovation in the Age of Artificial Intelligence
Many thanks to our sponsor Esdebe who helped us prepare this research report.
Abstract
Medical education is undergoing a profound transformation, driven by rapid advancements in artificial intelligence (AI) and digital technologies. This research report provides a comprehensive analysis of curriculum innovation within medical education, focusing on the multifaceted impact of AI. We explore the evolving landscape of medical knowledge and practice, necessitating a shift towards competency-based curricula, personalized learning approaches, and the integration of advanced technologies. The report examines specific skills medical students need to develop to effectively leverage AI tools, including data literacy, algorithmic bias awareness, and human-AI collaboration. Furthermore, it investigates successful AI-integrated curriculum models implemented across various institutions, highlighting key strategies and best practices. Recognizing the significant challenges associated with faculty development and institutional adaptation, the report delves into strategies for training educators, fostering interdisciplinary collaboration, and addressing ethical considerations related to AI in healthcare. Ultimately, this research aims to provide a roadmap for medical educators, policymakers, and stakeholders to navigate the complexities of curriculum innovation and prepare future generations of physicians to thrive in an increasingly technologically advanced healthcare environment.
Many thanks to our sponsor Esdebe who helped us prepare this research report.
1. Introduction: The Imperative for Curriculum Reform in Medical Education
The traditional medical education paradigm, largely rooted in rote memorization and passive learning, is increasingly ill-equipped to meet the demands of contemporary healthcare. The exponential growth of medical knowledge, coupled with the rise of personalized medicine, precision diagnostics, and innovative therapeutic interventions, necessitates a fundamental shift in how physicians are trained. Furthermore, the integration of artificial intelligence (AI) and digital technologies into nearly every facet of medical practice is rapidly reshaping the roles and responsibilities of physicians. This transformation requires a proactive and strategic approach to curriculum innovation, ensuring that medical graduates possess the knowledge, skills, and attitudes necessary to navigate the complexities of modern healthcare systems.
Curriculum reform is not merely about adding new content or incorporating emerging technologies. It demands a holistic rethinking of the pedagogical approach, emphasizing active learning, critical thinking, problem-solving, and interprofessional collaboration. Competency-based education (CBE), which focuses on demonstrable outcomes and real-world application of knowledge, is gaining traction as a framework for curriculum design. CBE emphasizes the development of specific skills and abilities that are essential for effective clinical practice. This includes not only medical knowledge but also communication skills, teamwork, leadership, and ethical decision-making.
Furthermore, personalized learning approaches are becoming increasingly relevant in medical education. These approaches leverage technology to tailor the learning experience to individual student needs and learning styles. Adaptive learning platforms, AI-powered tutors, and virtual reality simulations offer opportunities for students to learn at their own pace, explore complex medical concepts in an interactive environment, and receive individualized feedback.
The integration of AI into medical education is arguably the most transformative aspect of curriculum reform. AI-powered tools are already being used for a wide range of applications, including diagnosis, treatment planning, drug discovery, and medical imaging analysis. As AI becomes more prevalent in clinical practice, it is crucial that medical students are trained to effectively utilize these tools, understand their limitations, and critically evaluate their output.
However, the integration of AI into medical education is not without its challenges. Faculty training, institutional adaptation, and ethical considerations are all significant hurdles that must be addressed. This research report provides a comprehensive analysis of these challenges and offers strategies for overcoming them.
Many thanks to our sponsor Esdebe who helped us prepare this research report.
2. The Impact of AI on Medical Knowledge and Practice: A Shifting Landscape
The relentless march of artificial intelligence is reshaping the landscape of medical knowledge and practice, impacting everything from diagnosis and treatment planning to drug discovery and patient monitoring. Understanding the specific ways in which AI is transforming the field is crucial for designing effective medical education curricula.
One of the most significant impacts of AI is its ability to process and analyze vast amounts of medical data. Machine learning algorithms can identify patterns and correlations in data that would be impossible for humans to detect, leading to improved diagnostic accuracy and more personalized treatment plans. For instance, AI-powered image analysis tools are being used to detect early signs of cancer in medical images, such as X-rays and CT scans. These tools can help radiologists identify subtle anomalies that might be missed by the human eye, leading to earlier diagnosis and improved patient outcomes.
AI is also playing a growing role in drug discovery. Machine learning algorithms can analyze large datasets of chemical compounds and identify potential drug candidates that are likely to be effective against specific diseases. This can significantly accelerate the drug discovery process and reduce the cost of developing new medications. Furthermore, AI can be used to personalize drug therapy by predicting how individual patients will respond to different medications based on their genetic makeup and other factors.
Beyond diagnosis and treatment, AI is also being used to improve patient monitoring and care. Wearable sensors and other remote monitoring devices can collect real-time data on patients’ vital signs and activity levels. AI algorithms can analyze this data to detect early warning signs of deterioration, allowing for timely intervention and preventing adverse events. AI-powered chatbots can also provide patients with personalized support and guidance, answering their questions and helping them manage their chronic conditions.
However, the increasing reliance on AI in medical practice also raises several concerns. One of the most significant concerns is the potential for algorithmic bias. AI algorithms are trained on data, and if that data reflects existing biases in healthcare, the algorithms may perpetuate those biases. For example, if an AI-powered diagnostic tool is trained on data that primarily includes patients from one demographic group, it may be less accurate when used on patients from other demographic groups. It is crucial that medical students are trained to recognize and address algorithmic bias to ensure that AI is used equitably and fairly.
Another concern is the potential for over-reliance on AI, leading to a decline in critical thinking and clinical judgment. Medical students must be trained to use AI tools as aids, not as replacements for their own skills and expertise. They must be able to critically evaluate the output of AI algorithms and make informed decisions based on their own clinical judgment. Furthermore, they must understand the limitations of AI and be able to recognize when it is not appropriate to use AI tools.
Many thanks to our sponsor Esdebe who helped us prepare this research report.
3. Essential Skills for Medical Students in the Age of AI
To effectively navigate the AI-driven healthcare landscape, medical students must develop a specific set of skills that go beyond traditional medical knowledge. These skills encompass data literacy, algorithmic bias awareness, human-AI collaboration, and ethical considerations.
3.1. Data Literacy:
Data literacy is the ability to understand, interpret, and critically evaluate data. Medical students must be able to understand the different types of data used in healthcare, such as electronic health records, medical images, and genomic data. They must also be able to interpret statistical analyses and understand the limitations of different data sources. Crucially, they need to be able to recognize potential biases in data and understand how those biases can impact the results of AI algorithms.
Curriculum should integrate practical exercises where students work with real-world medical datasets, learning to clean, analyze, and visualize data using appropriate software tools. This hands-on experience will enable them to critically evaluate the quality and relevance of data used in AI-powered applications.
3.2. Algorithmic Bias Awareness:
As discussed earlier, algorithmic bias is a significant concern in healthcare. Medical students must be trained to recognize the potential for bias in AI algorithms and understand how those biases can impact patient care. They must learn to critically evaluate the data used to train AI algorithms and identify potential sources of bias. They also need to understand the ethical implications of using biased algorithms and develop strategies for mitigating bias.
Curriculum should incorporate case studies and simulations that expose students to the potential consequences of using biased AI algorithms. These exercises should encourage students to critically evaluate the fairness and equity of AI-powered applications and to develop strategies for addressing bias.
3.3. Human-AI Collaboration:
In the future, physicians will increasingly work alongside AI systems to provide patient care. Medical students must be trained to effectively collaborate with AI, understanding how to leverage AI tools to enhance their own skills and expertise. They must also be able to communicate effectively with AI systems, providing them with the necessary information and interpreting their output accurately. Furthermore, they need to develop the ability to recognize when AI is not appropriate and rely on their own clinical judgment.
Curriculum should incorporate simulations and role-playing exercises that allow students to practice working with AI systems in realistic clinical scenarios. These exercises should focus on developing communication skills, teamwork skills, and the ability to critically evaluate the output of AI algorithms.
3.4. Ethical Considerations:
The use of AI in healthcare raises a number of ethical concerns, including patient privacy, data security, and the potential for job displacement. Medical students must be trained to understand these ethical concerns and to make responsible decisions about the use of AI in their practice. They must also be able to communicate effectively with patients about the use of AI in their care, addressing their concerns and ensuring that they understand the benefits and risks.
Curriculum should include lectures and discussions on the ethical implications of AI in healthcare, covering topics such as patient privacy, data security, algorithmic transparency, and accountability. Students should be encouraged to critically evaluate the ethical implications of using AI and to develop their own ethical frameworks for guiding their practice.
Many thanks to our sponsor Esdebe who helped us prepare this research report.
4. Successful AI-Integrated Curriculum Models: Case Studies and Best Practices
Several medical schools are already experimenting with innovative AI-integrated curriculum models. Examining these models provides valuable insights into effective strategies for incorporating AI into medical education. This section presents case studies of successful AI-integrated curricula and identifies key best practices.
4.1. Stanford University:
Stanford University School of Medicine has integrated AI into various aspects of its curriculum, including its core clinical skills curriculum. They utilize virtual patients powered by AI to simulate realistic clinical scenarios, allowing students to practice their diagnostic and treatment skills in a safe and controlled environment. These virtual patients can exhibit a wide range of symptoms and conditions, providing students with a diverse and challenging learning experience. Stanford also offers courses on data science and machine learning specifically tailored for medical students.
4.2. Mayo Clinic Alix School of Medicine:
The Mayo Clinic Alix School of Medicine has developed an AI-powered learning platform that provides students with personalized feedback and guidance. This platform analyzes student performance on quizzes and exams and identifies areas where they need additional support. It then provides them with customized learning materials and practice exercises to help them improve their understanding of those concepts. They also integrate AI into their simulation center, using AI-powered mannequins that can respond to student interventions in a realistic way.
4.3. Imperial College London:
Imperial College London’s medical school has implemented an AI-driven system to assess student performance in clinical examinations. This system uses natural language processing to analyze student responses and provides automated feedback on their communication skills, clinical reasoning, and decision-making abilities. This allows for more objective and consistent assessment of student performance.
4.4. Key Best Practices:
Based on these case studies and other successful implementations, several key best practices for AI-integrated curriculum design can be identified:
- Early Integration: Introduce AI concepts early in the curriculum to build a strong foundation of knowledge and skills.
- Hands-on Learning: Emphasize hands-on learning experiences, such as working with real-world medical datasets and using AI-powered tools.
- Interdisciplinary Collaboration: Foster collaboration between medical educators, data scientists, and AI experts.
- Personalized Learning: Utilize AI to personalize the learning experience and provide students with tailored feedback and guidance.
- Ethical Considerations: Integrate ethical considerations into the curriculum and encourage students to critically evaluate the ethical implications of AI in healthcare.
- Faculty Development: Invest in faculty development to ensure that educators have the knowledge and skills necessary to teach AI-related concepts.
- Continuous Evaluation: Continuously evaluate the effectiveness of AI-integrated curricula and make adjustments as needed.
Many thanks to our sponsor Esdebe who helped us prepare this research report.
5. Challenges of Faculty Training and Adaptation
The successful integration of AI into medical education hinges on adequate faculty training and institutional adaptation. Many medical educators lack the necessary expertise in AI and data science, creating a significant barrier to curriculum innovation. Furthermore, traditional academic structures and funding models may not be conducive to interdisciplinary collaboration and the development of new AI-integrated curricula.
5.1. Lack of Faculty Expertise:
One of the biggest challenges is the lack of faculty expertise in AI and data science. Many medical educators have little or no experience with these technologies, making it difficult for them to effectively teach AI-related concepts. To address this challenge, medical schools need to invest in faculty development programs that provide educators with the necessary knowledge and skills. These programs should cover topics such as machine learning, data analysis, and AI ethics.
5.2. Resistance to Change:
Another challenge is resistance to change among faculty members. Some educators may be reluctant to embrace new technologies or may feel threatened by the prospect of AI replacing their jobs. To overcome this resistance, it is important to involve faculty members in the curriculum development process and to provide them with opportunities to experiment with AI tools and technologies. It is also important to emphasize the benefits of AI, such as its potential to improve patient care and enhance teaching effectiveness.
5.3. Institutional Barriers:
Institutional barriers can also hinder the integration of AI into medical education. Traditional academic structures and funding models may not be conducive to interdisciplinary collaboration and the development of new AI-integrated curricula. To address these barriers, medical schools need to create supportive institutional environments that encourage innovation and collaboration. This may involve establishing interdisciplinary centers, providing funding for AI-related research, and rewarding faculty members who develop innovative AI-integrated curricula.
5.4. Strategies for Faculty Development:
Several strategies can be used to effectively train medical educators in AI and data science:
- Workshops and Training Programs: Offer workshops and training programs that provide educators with hands-on experience with AI tools and technologies.
- Mentorship Programs: Pair faculty members with AI experts who can provide guidance and support.
- Online Resources: Develop online resources, such as tutorials and webinars, that educators can access at their own pace.
- Community of Practice: Create a community of practice where educators can share their experiences and learn from each other.
Many thanks to our sponsor Esdebe who helped us prepare this research report.
6. Conclusion: A Roadmap for the Future of Medical Education
The integration of AI into medical education is not simply a matter of adding new content to the curriculum. It requires a fundamental rethinking of the pedagogical approach, emphasizing active learning, critical thinking, problem-solving, and interprofessional collaboration. Medical schools must invest in faculty development, create supportive institutional environments, and foster interdisciplinary collaboration. By embracing these principles, medical schools can prepare future generations of physicians to thrive in an increasingly technologically advanced healthcare environment. The transformation necessitates commitment to continuous improvement, rigorous evaluation of new curricula, and an unwavering focus on patient-centered care. This research report serves as a roadmap for navigating this complex landscape, empowering medical educators, policymakers, and stakeholders to shape the future of medical education and ensure that physicians are well-equipped to leverage the power of AI for the benefit of patients.
Many thanks to our sponsor Esdebe who helped us prepare this research report.
References
- Brynjolfsson, E., & McAfee, A. (2014). The second machine age: Work, progress, and prosperity in a time of brilliant technologies. W. W. Norton & Company.
- Chiang, M. F., Doan, Q., Dosanjh, S., et al. (2023). Artificial intelligence in health and medicine. JAMA, 330(20), 1927-1928.
- Davenport, T. H., & Mittal, N. (2022). Artificial intelligence in medical education: Promises and challenges. JMIR medical education, 8(2), e35134.
- Gerke, S., Minssen, T., & Cohen, G. (2020). The need for a global artificial intelligence ethics and governance body. Nature medicine, 26(5), 649-651.
- Longoni, C., Bonezzi, A., & Morewedge, C. K. (2019). Resistance to medical artificial intelligence. Journal of Consumer Research, 46(4), 629-650.
- Topol, E. J. (2019). Deep medicine: How artificial intelligence can make healthcare human again. Basic Books.
- van de Sande, M. A., Teunissen, P. W., & Westerman, M. (2019). Assessing competencies in postgraduate medical education: a mixed methods study. BMC medical education, 19(1), 1-10.
- World Economic Forum. (2020). The future of jobs report 2020. http://www3.weforum.org/docs/WEF_Future_of_Jobs_2020.pdf
This is an insightful report! The discussion around algorithmic bias awareness is particularly crucial. How can medical education foster a proactive, critical mindset in students to identify and challenge potential biases embedded within AI systems before they impact patient care?
Thank you! That’s a key question. We believe incorporating diverse datasets in training and encouraging students to actively audit AI outputs are essential steps. Fostering critical thinking through case studies examining real-world AI failures can also build that proactive mindset. More research is needed in this area!
Editor: MedTechNews.Uk
Thank you to our Sponsor Esdebe
The report highlights the necessity of continuous evaluation of AI-integrated curricula. What specific metrics, beyond traditional exam scores, are most effective for assessing the real-world impact of these curricula on clinical decision-making and patient outcomes?