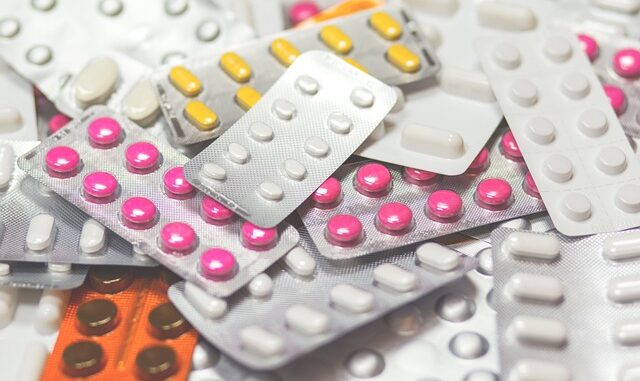
Abstract
Drug development is a notoriously lengthy, costly, and high-risk endeavor. The traditional process, involving target identification, lead discovery, preclinical studies, clinical trials, and regulatory approval, can span over a decade and cost billions of dollars. The integration of Artificial Intelligence (AI) and Machine Learning (ML) is rapidly transforming this landscape, offering the potential to accelerate timelines, reduce costs, and improve the probability of success. This research report provides a comprehensive analysis of AI’s multifaceted role in modern drug development. We delve into specific AI techniques employed across various stages, from early target identification and drug design to clinical trial optimization and post-market surveillance. Furthermore, we critically examine the impact of AI on drug development timelines and costs, exploring both the realized benefits and the remaining challenges. Special attention is paid to the crucial aspects of validating AI-discovered drug candidates, addressing the reproducibility crisis, and establishing robust regulatory frameworks. Finally, we navigate the complex ethical considerations surrounding the use of AI in drug development, including data privacy, algorithmic bias, and the potential for unintended consequences. Through a synthesis of existing literature, case studies, and expert opinions, this report aims to provide a nuanced perspective on the transformative potential and inherent complexities of AI-driven drug development, offering valuable insights for researchers, industry professionals, and policymakers alike.
Many thanks to our sponsor Esdebe who helped us prepare this research report.
1. Introduction
The pharmaceutical industry faces increasing pressure to deliver novel therapies efficiently and effectively. The rising costs of research and development (R&D), coupled with the declining success rates of traditional drug discovery methods, necessitate innovative approaches. According to a study by Deloitte, the average cost of bringing a new drug to market has nearly doubled in the last decade, while the return on investment in R&D has significantly decreased [1]. This inefficiency stems from several factors, including the inherent complexity of biological systems, the limitations of current experimental techniques, and the difficulty in predicting drug efficacy and toxicity in humans. The advent of high-throughput screening, genomics, proteomics, and other omics technologies has generated vast amounts of data, creating both opportunities and challenges for drug discovery. The sheer volume and complexity of this data exceed the capabilities of traditional analytical methods, thus highlighting the need for advanced computational approaches.
Artificial Intelligence (AI), encompassing Machine Learning (ML) and Deep Learning (DL), has emerged as a promising solution to address these challenges. AI offers the ability to analyze complex datasets, identify patterns, and make predictions with unprecedented accuracy and speed. AI-powered tools can accelerate various stages of drug development, including target identification and validation, drug design and optimization, preclinical studies, clinical trials, and post-market surveillance [2]. Moreover, AI can facilitate the development of personalized medicine by tailoring treatments to individual patients based on their genetic profiles and other relevant factors. However, the integration of AI into drug development is not without its challenges. These include the need for high-quality data, the lack of interpretability of some AI models, the difficulty in validating AI-discovered drug candidates, and the ethical considerations surrounding the use of AI in healthcare. This report aims to provide a comprehensive overview of the current state of AI in drug development, addressing both its transformative potential and its inherent complexities.
Many thanks to our sponsor Esdebe who helped us prepare this research report.
2. AI Techniques in Drug Discovery
AI is being deployed across a wide range of drug discovery activities, leveraging various techniques to enhance efficiency and effectiveness. The following subsections detail key applications and relevant AI methodologies.
2.1 Target Identification and Validation
Identifying the right drug target is a crucial first step in drug discovery. Traditionally, target identification relied on serendipitous discoveries or hypothesis-driven research. AI offers a more systematic and data-driven approach. Network analysis, a key technique in systems biology, uses graph theory to model the complex interactions between genes, proteins, and other biomolecules [3]. By analyzing these networks, AI can identify key nodes or pathways that are dysregulated in disease, thus suggesting potential drug targets. For example, algorithms can identify genes whose expression is significantly altered in disease tissues compared to healthy tissues. These genes, and the proteins they encode, can then be prioritized as potential drug targets. Furthermore, AI can integrate data from multiple sources, such as genomics, proteomics, and metabolomics, to provide a more holistic view of disease biology. Machine learning models, such as support vector machines (SVMs) and random forests, can be trained to predict drug targets based on their characteristics, such as protein structure, binding affinity, and gene expression patterns. Target validation, the process of confirming that a target is indeed relevant to the disease, can also be accelerated by AI. For example, AI can be used to predict the effects of target knockdown or knockout on cellular phenotypes, thus guiding experimental validation efforts. Natural Language Processing (NLP) is also proving invaluable, capable of sifting through scientific literature and databases to identify potential drug targets and associated pathways, significantly speeding up the process of hypothesis generation [4].
2.2 Drug Design and Optimization
Once a drug target has been identified, the next step is to design or identify molecules that can bind to the target and modulate its activity. This process, known as lead discovery, is traditionally performed through high-throughput screening (HTS) or rational drug design. AI can significantly enhance both of these approaches. Virtual screening, a computational technique that uses AI to screen large libraries of chemical compounds against a target protein, has become a powerful tool for lead discovery [5]. Molecular docking simulations, which predict the binding affinity and binding mode of a compound to a target protein, are often used in virtual screening. AI can improve the accuracy and speed of these simulations by learning from experimental data and incorporating complex physical and chemical principles. Generative models, such as variational autoencoders (VAEs) and generative adversarial networks (GANs), can be used to design novel molecules with desired properties. These models are trained on large datasets of chemical structures and biological activities, and can then generate new molecules that are similar to known drugs but with improved potency, selectivity, or bioavailability [6]. Furthermore, AI can be used to optimize the properties of existing drug candidates. Machine learning models can be trained to predict the pharmacokinetic (PK) and pharmacodynamic (PD) properties of a molecule based on its chemical structure. This allows researchers to optimize the molecule’s structure to improve its absorption, distribution, metabolism, excretion, and toxicity (ADMET) profile. Deep learning techniques, particularly convolutional neural networks (CNNs), are increasingly used to analyze molecular structures and predict their interactions with biological targets, significantly enhancing the precision of drug design.
2.3 Preclinical Studies
Preclinical studies are conducted to evaluate the safety and efficacy of drug candidates in vitro and in vivo. AI can improve the efficiency and accuracy of these studies by predicting the outcomes of experiments and identifying potential safety issues early on. For example, AI can be used to predict the toxicity of a drug candidate based on its chemical structure and biological activity. This can help researchers prioritize drug candidates for further development and avoid costly failures later on. Machine learning models can also be trained to predict the efficacy of a drug candidate in animal models. This can help researchers optimize the design of preclinical studies and select the most promising drug candidates for clinical trials. Furthermore, AI can be used to analyze preclinical data and identify biomarkers that can be used to predict drug response in humans. This can help researchers design more targeted clinical trials and improve the likelihood of success [7]. Sophisticated image analysis algorithms, often based on deep learning, are used to analyze high-content screening data and histological images, enabling more precise and efficient assessment of drug effects in preclinical models. The use of AI in predicting drug-drug interactions is also growing, contributing to improved safety profiles in preclinical testing.
2.4 Clinical Trials
Clinical trials are the most expensive and time-consuming part of drug development. AI can help to optimize clinical trials by improving patient selection, predicting treatment response, and monitoring patient safety. For example, AI can be used to identify patients who are most likely to respond to a particular treatment based on their genetic profile, medical history, and other relevant factors [8]. This can help to improve the efficiency of clinical trials by enrolling patients who are more likely to benefit from the treatment. Machine learning models can also be trained to predict treatment response based on clinical trial data. This can help researchers identify biomarkers that can be used to predict drug response in future patients. Furthermore, AI can be used to monitor patient safety during clinical trials. By analyzing patient data in real-time, AI can identify potential safety issues early on and take corrective action. AI-powered tools are also being used to automate data collection and analysis in clinical trials, reducing the risk of human error and improving the accuracy of results. Adaptive trial designs, enabled by AI, allow for adjustments during the trial based on interim results, leading to more efficient and ethical trial conduct.
2.5 Post-Market Surveillance
Even after a drug has been approved and marketed, it is important to continue monitoring its safety and efficacy. This is known as post-market surveillance. AI can be used to analyze real-world data, such as electronic health records and social media data, to identify potential safety signals and monitor drug efficacy [9]. For example, AI can be used to identify patients who are experiencing adverse events after taking a particular drug. This can help to identify rare but serious side effects that were not detected during clinical trials. Natural Language Processing (NLP) techniques can be employed to analyze patient reviews and social media posts to identify potential safety signals and assess patient satisfaction with a drug. AI can also be used to monitor drug efficacy in real-world settings. By analyzing data from electronic health records, AI can track the effectiveness of a drug over time and identify factors that may influence its efficacy. This can help to optimize drug dosing and identify patients who may benefit from alternative treatments. Predictive models can be developed to forecast potential drug shortages and ensure adequate supply chains.
Many thanks to our sponsor Esdebe who helped us prepare this research report.
3. Impact on Drug Development Timelines and Costs
The potential of AI to accelerate drug development timelines and reduce costs is a major driving force behind its adoption in the pharmaceutical industry. The traditional drug development process can take 10-15 years and cost billions of dollars. AI has the potential to significantly reduce both of these factors. By accelerating target identification and validation, AI can shorten the early stages of drug development. Virtual screening and generative models can speed up lead discovery and optimization. AI can improve the efficiency of preclinical studies by predicting the outcomes of experiments and identifying potential safety issues early on. AI can optimize clinical trials by improving patient selection, predicting treatment response, and monitoring patient safety. Furthermore, AI can reduce the risk of failure in clinical trials by identifying and mitigating potential safety issues and efficacy problems [10]. A report by McKinsey estimated that AI could reduce drug discovery timelines by up to 25% and lower R&D costs by 10-15% [11]. However, it is important to note that these are just estimates, and the actual impact of AI on drug development timelines and costs will depend on a number of factors, including the specific AI techniques used, the quality of the data, and the expertise of the researchers. While the initial investment in AI infrastructure and expertise can be substantial, the long-term cost savings and efficiency gains are expected to outweigh these costs significantly. The ability to identify and prioritize promising drug candidates earlier in the development process can prevent costly late-stage failures, further contributing to overall cost reduction.
Many thanks to our sponsor Esdebe who helped us prepare this research report.
4. Challenges in Validating AI-Discovered Drug Candidates
Despite the promise of AI in drug development, there are significant challenges in validating AI-discovered drug candidates. One of the biggest challenges is the lack of interpretability of some AI models, particularly deep learning models. These models, often referred to as “black boxes,” can make accurate predictions but provide little insight into why they made those predictions. This lack of interpretability makes it difficult to validate the predictions and understand the underlying mechanisms of action. The “black box” nature of certain AI algorithms makes it difficult to build trust and confidence in their predictions, especially when dealing with complex biological systems. Ensuring data quality and reproducibility is crucial for validating AI-discovered drug candidates. AI models are only as good as the data they are trained on. If the data is biased, incomplete, or inaccurate, the models will produce biased, incomplete, or inaccurate predictions [12]. This can lead to the identification of false-positive drug candidates and the failure of clinical trials. Furthermore, it is important to ensure that the results of AI-powered experiments are reproducible. This requires careful attention to detail in the design and execution of experiments, as well as the use of standardized protocols and data formats. The inherent complexity of biological systems also poses a challenge to validating AI-discovered drug candidates. Biological systems are highly complex and interconnected, and it can be difficult to predict how a drug will interact with the system as a whole. AI models may be able to predict the effect of a drug on a specific target, but they may not be able to predict the off-target effects or the effects on other biological pathways. Addressing these challenges requires a multi-faceted approach, including the development of more interpretable AI models, the use of high-quality data, the adoption of standardized protocols, and a thorough understanding of biological systems. Moreover, close collaboration between AI experts and domain experts (e.g., biologists, chemists, clinicians) is essential to ensure that AI is used effectively and responsibly.
Many thanks to our sponsor Esdebe who helped us prepare this research report.
5. Ethical Considerations of Using AI in Drug Development
The use of AI in drug development raises several ethical considerations. One of the most important ethical considerations is data privacy. AI models require large amounts of data to be trained effectively, and this data may contain sensitive information about patients. It is essential to protect the privacy of patients and ensure that their data is used responsibly [13]. This requires the implementation of robust data security measures, as well as the establishment of clear guidelines for data sharing and access. Algorithmic bias is another important ethical consideration. AI models can inherit biases from the data they are trained on, which can lead to unfair or discriminatory outcomes. For example, if an AI model is trained on data that is primarily from one ethnic group, it may not perform well on patients from other ethnic groups. It is essential to identify and mitigate algorithmic bias to ensure that AI is used fairly and equitably. The potential for unintended consequences is also an important ethical consideration. AI models are complex and can have unexpected effects. It is important to carefully consider the potential consequences of using AI in drug development and to take steps to mitigate any risks. Furthermore, it is important to ensure that AI is used in a way that is consistent with human values and ethical principles. Transparency and explainability are also crucial for ethical AI deployment. Understanding how AI algorithms arrive at their conclusions allows for better scrutiny and accountability. A lack of transparency can erode trust and make it difficult to identify and correct errors or biases. Robust regulatory frameworks are needed to address these ethical considerations and ensure that AI is used responsibly in drug development. These frameworks should address issues such as data privacy, algorithmic bias, transparency, and accountability. Furthermore, it is important to involve stakeholders from diverse backgrounds in the development of these frameworks to ensure that they reflect a broad range of perspectives and values. Establishing clear ethical guidelines and promoting responsible AI practices are essential to realize the full potential of AI in drug development while minimizing the risks.
Many thanks to our sponsor Esdebe who helped us prepare this research report.
6. Future Directions and Conclusion
The field of AI in drug development is rapidly evolving, with new techniques and applications emerging constantly. Future research will likely focus on developing more interpretable AI models, improving the quality and availability of data, and integrating AI with other advanced technologies, such as CRISPR gene editing and nanotechnology. The development of more explainable AI (XAI) techniques will be crucial for building trust and confidence in AI-driven drug discovery. These techniques aim to provide insights into the decision-making processes of AI models, allowing researchers to understand why a particular drug candidate was selected or why a certain prediction was made. The integration of multi-omics data (e.g., genomics, proteomics, metabolomics) with AI will enable a more comprehensive understanding of disease biology and drug mechanisms of action. This will lead to the identification of more effective and personalized therapies. Furthermore, the use of AI in personalized medicine will become increasingly important. By tailoring treatments to individual patients based on their genetic profiles and other relevant factors, AI can improve the efficacy and safety of drugs. The development of AI-powered diagnostic tools will also play a key role in personalized medicine, enabling early detection of disease and more targeted treatment strategies. The application of federated learning, where models are trained across multiple decentralized devices or servers holding local data samples without exchanging them, will address data privacy concerns and facilitate collaborative research efforts [14]. In conclusion, AI has the potential to revolutionize drug development by accelerating timelines, reducing costs, and improving the probability of success. However, it is important to address the challenges in validating AI-discovered drug candidates and the ethical considerations surrounding the use of AI in this field. By developing more interpretable AI models, ensuring data quality and reproducibility, and establishing robust regulatory frameworks, we can harness the full potential of AI to develop new and more effective treatments for diseases.
Many thanks to our sponsor Esdebe who helped us prepare this research report.
References
[1] Deloitte. (2020). Measuring the return from pharmaceutical innovation 2020: Confronting the pharma paradox. https://www2.deloitte.com/content/dam/Deloitte/uk/Documents/life-sciences-health-care/deloitte-uk-measuring-the-return-from-pharmaceutical-innovation-2020.pdf
[2] Paul, D., Sanap, G., Shenoy, S., Kalyane, D., Kalia, K., & Tekade, R. K. (2021). Artificial intelligence in drug discovery and development. Drug discovery today, 26(1), 80-93.
[3] Barabási, A. L., Gulbahce, N., & Loscalzo, J. (2011). Network medicine: a network-based approach to human disease. Nature Reviews Genetics, 12(1), 56-68.
[4] Chen, H., Engkvist, O., Wang, Y., Olivecrona, M., Blaschke, T., & Bajorath, J. (2016). The Rise of Deep Learning in Drug Discovery. Drug Discovery Today, 23(7), 1241-1250.
[5] Shoichet, B. K. (2004). Virtual screening of chemical libraries. Nature, 432(7019), 862-868.
[6] Gómez-Bombarelli, R., Wei, J. N., Duvenaud, D., Hernández-Lobato, J. M., Sánchez-Lengeling, B., Sheberla, D., … & Aspuru-Guzik, A. (2018). Automatic chemical design using a data-driven continuous representation of molecules. ACS central science, 4(2), 268-276.
[7] Mamoshina, P., Singh, R., Novoselov, A., Zhavoronkov, A., & Cantor, C. (2016). Applications of deep learning in biomedicine. Molecular pharmaceutics, 13(5), 1445-1454.
[8] Jiang, F., Jiang, Y., Zhi, H., Dong, Y., Li, H., Ma, S., … & Wang, Y. (2017). Artificial intelligence in healthcare: past, present and future. Stroke and vascular neurology, 2(4), 230-243.
[9] Beam, A. L., & Kohane, I. S. (2018). Big Data and Machine Learning in Health Care. JAMA, 319(13), 1317–1318.
[10] Schneider, G. (2018). Artificial intelligence in drug discovery. Nature Reviews Drug Discovery, 17(3), 97-115.
[11] McKinsey & Company. (2020). Notes from the AI frontier: Modeling the impact of AI on the world economy. https://www.mckinsey.com/featured-insights/artificial-intelligence/notes-from-the-ai-frontier-modeling-the-impact-of-ai-on-the-world-economy
[12] Wachter, S., Mittelstadt, B., & Russell, C. (2017). Transparent, explainable, and accountable AI for robotics. Science Robotics, 2(6), eaam9774.
[13] Price, W. N., Gerke, S., Luger, T., & O’Rourke, O. (2019). What is precision medicine? Science Translational Medicine, 11(483), eaaw7584.
[14] Rieke, N., Hancox, J., Li, W., Milletarì, F., Roth, H. R., Albarqouni, S., Bakas, S., Galtier, M. N., Landman, B. A., Maier-Hein, L., & Ourselin, S. (2020). The future of digital health with federated learning. NPJ digital medicine, 3(1), 119.
The report mentions using AI for post-market surveillance to identify potential safety signals. How might AI be used proactively to simulate the effects of a drug on diverse patient populations before launch, accounting for genetic variations and lifestyle factors to predict potential adverse reactions more accurately?
That’s a great point! Expanding on AI’s proactive use, sophisticated simulations could indeed revolutionize pre-launch testing. By modeling drug interactions with diverse genetic profiles and lifestyle factors, we could predict adverse reactions with greater accuracy, ultimately leading to safer and more effective treatments for everyone. Thanks for sparking this important discussion!
Editor: MedTechNews.Uk
Thank you to our Sponsor Esdebe