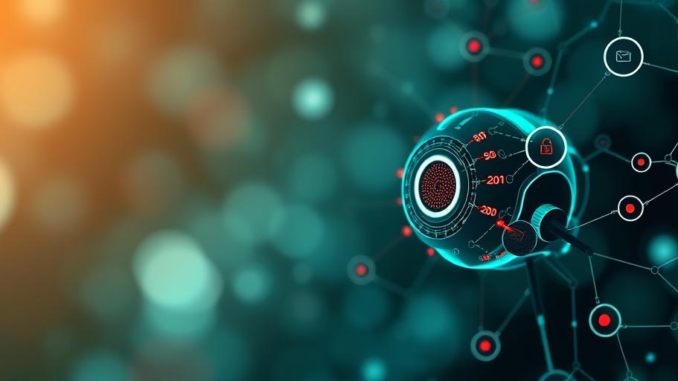
Revolutionizing Prostate Cancer Detection and Management: A Comprehensive Overview of AI Integration, Current Landscape, and Future Directions
Many thanks to our sponsor Esdebe who helped us prepare this research report.
Abstract
Prostate cancer (PCa) remains a significant global health challenge, characterized by a variable clinical course ranging from indolent disease to aggressive, metastatic forms. This research report provides a comprehensive overview of PCa, encompassing its epidemiology, current diagnostic and treatment paradigms, the economic burden, and emerging avenues for future research. A central focus is the integration of artificial intelligence (AI) into PCa management, particularly in early detection and risk stratification. While AI offers transformative potential, this report also critically examines the ethical considerations surrounding its deployment, including bias mitigation, data privacy, and the potential impact on the patient-physician relationship. The report aims to provide experts with a nuanced understanding of the current state of PCa management and the role of AI in shaping its future.
Many thanks to our sponsor Esdebe who helped us prepare this research report.
1. Introduction
Prostate cancer (PCa) is the second most frequently diagnosed cancer in men worldwide and a leading cause of cancer-related mortality. The incidence rates vary significantly across different geographical regions, with higher rates observed in developed countries. PCa presents a unique clinical challenge due to its heterogeneous nature, ranging from slow-growing, clinically insignificant tumors to aggressive, rapidly metastasizing cancers. This heterogeneity complicates diagnosis, risk stratification, and treatment decisions. The current standard of care relies on a combination of prostate-specific antigen (PSA) screening, digital rectal examination (DRE), and transrectal ultrasound-guided biopsy (TRUS-Bx). However, these methods have limitations, including the potential for overdiagnosis and overtreatment of indolent disease, as well as underdetection of aggressive tumors. The advent of artificial intelligence (AI) and machine learning (ML) technologies holds promise for improving PCa detection, risk stratification, and treatment planning. This report aims to provide a comprehensive overview of PCa, focusing on the integration of AI into various aspects of disease management, along with a critical appraisal of the ethical considerations involved.
Many thanks to our sponsor Esdebe who helped us prepare this research report.
2. Epidemiology of Prostate Cancer
The global burden of PCa is substantial, with an estimated 1.4 million new cases diagnosed annually. Incidence rates vary considerably, with the highest rates observed in North America, Europe, and Australia, and lower rates in Asia and Africa. These differences are attributed to a combination of factors, including variations in screening practices, genetic predisposition, and environmental exposures. Age is a major risk factor, with the incidence increasing significantly after the age of 50. Other established risk factors include race/ethnicity (African American men have a higher incidence and mortality rate), family history of PCa, and certain genetic mutations (e.g., BRCA1/2, HOXB13). Lifestyle factors, such as diet and obesity, have also been implicated in PCa development and progression, although the evidence remains inconclusive. The increasing use of PSA screening has contributed to the rise in PCa incidence, particularly in developed countries. However, this has also led to concerns about overdiagnosis and overtreatment of indolent tumors. In recent years, there has been a growing trend towards risk-adapted screening strategies, aiming to optimize the balance between early detection and avoiding unnecessary interventions.
Many thanks to our sponsor Esdebe who helped us prepare this research report.
3. Current Diagnostic Methods and Their Limitations
The diagnosis of PCa typically involves a combination of the following methods:
- Prostate-Specific Antigen (PSA) Screening: PSA is a glycoprotein produced by prostate cells. Elevated PSA levels can indicate the presence of PCa, but can also be elevated due to benign prostatic hyperplasia (BPH), prostatitis, or other non-cancerous conditions. The sensitivity and specificity of PSA screening are therefore limited, leading to a high false-positive rate. The optimal PSA threshold for biopsy referral remains a subject of debate, and age-specific PSA reference ranges are often used to improve accuracy. PSA density (PSA divided by prostate volume) and PSA velocity (rate of change in PSA over time) can also provide additional information. Furthermore, the use of PSA isoforms, such as free PSA and proPSA, can improve the specificity of PSA screening.
- Digital Rectal Examination (DRE): DRE involves a physical examination of the prostate gland through the rectum. It can detect palpable nodules or irregularities that may indicate the presence of PCa. However, DRE has low sensitivity and specificity, and many PCa tumors are not palpable. It is typically used in conjunction with PSA screening.
- Transrectal Ultrasound-Guided Biopsy (TRUS-Bx): TRUS-Bx is the standard method for confirming the diagnosis of PCa. It involves inserting a needle through the rectum and into the prostate gland to obtain tissue samples. The biopsy samples are then examined under a microscope by a pathologist to determine the presence of cancer and its Gleason score, which is a measure of tumor aggressiveness. TRUS-Bx has limitations, including the risk of complications such as infection, bleeding, and pain. Furthermore, it can miss aggressive tumors, particularly those located in the anterior part of the prostate gland. Systematic TRUS-Bx, which involves taking multiple samples from different regions of the prostate, has been shown to improve detection rates, but can also lead to overdiagnosis of clinically insignificant tumors.
- Multiparametric Magnetic Resonance Imaging (mpMRI): mpMRI is an imaging technique that uses magnetic fields and radio waves to create detailed images of the prostate gland. It can detect suspicious lesions and guide targeted biopsies to improve diagnostic accuracy. mpMRI typically includes T2-weighted imaging, diffusion-weighted imaging (DWI), and dynamic contrast-enhanced (DCE) imaging. The Prostate Imaging Reporting and Data System (PI-RADS) is a standardized scoring system used to interpret mpMRI findings. mpMRI has been shown to improve the detection of clinically significant PCa and reduce the number of unnecessary biopsies. However, it is not perfect, and false-positive and false-negative results can occur. Access to mpMRI may also be limited in some settings.
Many thanks to our sponsor Esdebe who helped us prepare this research report.
4. Treatment Options for Prostate Cancer
The treatment of PCa depends on several factors, including the stage and grade of the cancer, the patient’s age and overall health, and their preferences. Treatment options include:
- Active Surveillance: Active surveillance involves monitoring the cancer closely with regular PSA testing, DREs, and biopsies. It is typically recommended for men with low-risk PCa who are unlikely to benefit from immediate treatment. The goal of active surveillance is to avoid or delay treatment and its associated side effects. Treatment is initiated if there is evidence of disease progression. Recent research indicates that some men can remain on active surveillance for many years without requiring treatment.
- Radical Prostatectomy: Radical prostatectomy involves the surgical removal of the entire prostate gland and surrounding tissues. It can be performed through an open incision or laparoscopically, often with robotic assistance. Radical prostatectomy is a curative treatment option for localized PCa. However, it can have side effects, including urinary incontinence, erectile dysfunction, and bowel problems.
- Radiation Therapy: Radiation therapy uses high-energy rays to kill cancer cells. It can be delivered externally (external beam radiation therapy) or internally (brachytherapy). External beam radiation therapy involves directing radiation beams at the prostate gland from outside the body. Brachytherapy involves implanting radioactive seeds directly into the prostate gland. Radiation therapy is a curative treatment option for localized PCa. However, it can have side effects, including urinary, bowel, and sexual dysfunction.
- Hormone Therapy (Androgen Deprivation Therapy): Hormone therapy aims to reduce the levels of androgens (male hormones) in the body, which can slow the growth of PCa cells. It is typically used for men with advanced PCa or for men with high-risk localized PCa who are receiving radiation therapy. Hormone therapy can have significant side effects, including hot flashes, fatigue, loss of libido, erectile dysfunction, and osteoporosis.
- Chemotherapy: Chemotherapy uses drugs to kill cancer cells. It is typically used for men with metastatic PCa who are no longer responding to hormone therapy. Chemotherapy can have significant side effects, including nausea, vomiting, fatigue, and hair loss.
- Immunotherapy: Immunotherapy uses the body’s own immune system to fight cancer cells. It is a newer treatment option for metastatic PCa and has shown promising results in some patients. Sipuleucel-T is an example of an immunotherapy drug approved for PCa.
- Targeted Therapies: Targeted therapies are drugs that specifically target certain molecules or pathways involved in cancer cell growth. Examples include PARP inhibitors for men with certain genetic mutations and androgen receptor signaling inhibitors.
Many thanks to our sponsor Esdebe who helped us prepare this research report.
5. The Economic Burden of Prostate Cancer
The economic burden of PCa is substantial, encompassing direct medical costs (e.g., diagnosis, treatment, follow-up care) and indirect costs (e.g., lost productivity due to illness and premature death). The direct medical costs of PCa are estimated to be billions of dollars annually in the United States alone. These costs are driven by the high prevalence of the disease, the use of expensive diagnostic and treatment modalities, and the long-term follow-up care required for many patients. The indirect costs of PCa are also significant, particularly for men with advanced disease who are unable to work. Furthermore, the costs associated with managing treatment-related side effects, such as urinary incontinence and erectile dysfunction, can be substantial. The development of more cost-effective diagnostic and treatment strategies is crucial to reducing the economic burden of PCa. This includes optimizing screening practices to avoid overdiagnosis and overtreatment, promoting the use of less invasive and less expensive diagnostic methods, and developing more effective and less toxic treatment options. AI-driven tools hold promise for improving the efficiency and cost-effectiveness of PCa management.
Many thanks to our sponsor Esdebe who helped us prepare this research report.
6. Future Research Directions
Future research directions in PCa include:
- Improving Risk Stratification: Developing more accurate and reliable methods for identifying men with aggressive PCa who are most likely to benefit from treatment, while avoiding overtreatment of men with indolent disease. This includes the development of new biomarkers, genomic tests, and imaging techniques. Further research into the molecular subtypes of PCa is also crucial.
- Developing Novel Therapies: Developing new and more effective therapies for PCa, particularly for men with advanced disease who are no longer responding to standard treatments. This includes the development of new targeted therapies, immunotherapies, and combination therapies. Research into the tumor microenvironment and its role in PCa progression is also important.
- Optimizing Treatment Strategies: Optimizing the use of existing treatments for PCa to improve outcomes and reduce side effects. This includes the development of personalized treatment approaches based on individual patient characteristics and tumor biology. Research into the optimal sequencing of treatments is also needed.
- Prevention: Identifying modifiable risk factors for PCa and developing strategies to prevent the disease. This includes research into the role of diet, lifestyle, and environmental exposures in PCa development. Further research into chemoprevention strategies is also warranted.
- Artificial Intelligence (AI) and Machine Learning (ML): Integrating AI and ML into various aspects of PCa management, including early detection, risk stratification, treatment planning, and drug discovery. This includes the development of AI-powered diagnostic tools, predictive models, and personalized treatment recommendations. The ability of AI to analyze complex datasets and identify patterns that are not readily apparent to humans holds great promise for improving PCa outcomes.
Many thanks to our sponsor Esdebe who helped us prepare this research report.
7. The Role of Artificial Intelligence in Prostate Cancer Management
AI is rapidly transforming various aspects of healthcare, and PCa is no exception. AI and ML algorithms can analyze vast amounts of data, including imaging data, genomic data, and clinical data, to identify patterns and make predictions that can improve diagnostic accuracy, risk stratification, and treatment planning.
- AI in Early Detection: AI can be used to analyze mpMRI images of the prostate gland to detect suspicious lesions and guide targeted biopsies. Several studies have shown that AI-powered diagnostic tools can improve the accuracy of mpMRI interpretation and reduce the number of unnecessary biopsies. AI can also be used to analyze PSA data to identify men at high risk of developing PCa and to personalize screening recommendations. For example, AI algorithms can be trained to predict the likelihood of a positive biopsy based on PSA levels, DRE findings, and other clinical factors.
- AI in Risk Stratification: AI can be used to predict the risk of PCa progression and metastasis based on clinical, pathological, and genomic data. This can help clinicians to make more informed treatment decisions and to tailor treatment to individual patient risk profiles. For instance, AI models can predict the likelihood of biochemical recurrence after radical prostatectomy or radiation therapy based on Gleason score, PSA levels, and other pathological features.
- AI in Treatment Planning: AI can be used to optimize treatment planning for radiation therapy by identifying the optimal radiation dose and delivery technique to maximize tumor control while minimizing side effects. AI can also be used to predict the response to different treatments and to identify patients who are most likely to benefit from a particular therapy. This is particularly relevant in the context of targeted therapies and immunotherapies, where predictive biomarkers are often lacking.
- AI in Drug Discovery: AI can be used to accelerate the drug discovery process by identifying potential drug targets and predicting the efficacy of new drug candidates. AI algorithms can analyze vast amounts of genomic and proteomic data to identify genes and proteins that are involved in PCa development and progression. They can also be used to screen large libraries of compounds to identify those that are most likely to inhibit these targets.
Many thanks to our sponsor Esdebe who helped us prepare this research report.
8. Ethical Considerations of AI in Prostate Cancer Diagnosis
While AI offers tremendous potential for improving PCa management, it also raises several ethical considerations that must be addressed to ensure its responsible and equitable deployment. These considerations include:
- Bias Mitigation: AI algorithms are trained on data, and if the data is biased, the algorithm will also be biased. This can lead to disparities in diagnostic accuracy and treatment outcomes for different patient populations. For example, if an AI-powered diagnostic tool is trained primarily on data from Caucasian men, it may perform poorly on African American men, who have a higher incidence and mortality rate of PCa. It is therefore crucial to ensure that AI algorithms are trained on diverse and representative datasets and that bias is actively mitigated during the development and validation process. Techniques such as adversarial training and data augmentation can be used to reduce bias.
- Data Privacy and Security: AI algorithms require access to large amounts of patient data, which raises concerns about data privacy and security. It is essential to protect patient data from unauthorized access and disclosure. This requires the implementation of robust data security measures, such as encryption, access controls, and data anonymization techniques. Compliance with data privacy regulations, such as HIPAA and GDPR, is also crucial. Furthermore, patients should be informed about how their data will be used and should have the right to control access to their data.
- Transparency and Explainability: AI algorithms can be complex and opaque, making it difficult to understand how they arrive at their conclusions. This lack of transparency can erode trust in AI-powered diagnostic tools and make it difficult for clinicians to interpret and act on their recommendations. It is therefore important to develop AI algorithms that are transparent and explainable. This can be achieved through the use of techniques such as explainable AI (XAI), which aims to provide insights into the decision-making process of AI algorithms. However, achieving a balance between transparency and accuracy can be challenging.
- Impact on the Patient-Physician Relationship: The increasing use of AI in healthcare may alter the patient-physician relationship. Patients may feel that they are being treated by an algorithm rather than a human being, which can erode trust and satisfaction. It is therefore important to ensure that AI is used as a tool to augment, rather than replace, the role of the physician. Clinicians should be trained to effectively communicate with patients about the use of AI in their care and to address their concerns. The focus should always remain on providing patient-centered care.
- Accountability and Responsibility: It is important to establish clear lines of accountability and responsibility for the decisions made by AI algorithms. If an AI-powered diagnostic tool makes an error, who is responsible? The developer of the algorithm? The clinician who uses the tool? The hospital or healthcare system that deploys the tool? These are complex legal and ethical questions that need to be addressed. A framework for assessing and mitigating the risks associated with AI-powered diagnostic tools is also needed.
- Over-Reliance on AI: It is important to avoid over-reliance on AI and to maintain critical thinking skills. Clinicians should not blindly accept the recommendations of AI algorithms without carefully considering the individual patient’s circumstances. AI should be used as a tool to inform decision-making, but not to replace human judgment. A healthy skepticism is warranted.
Many thanks to our sponsor Esdebe who helped us prepare this research report.
9. Conclusion
Prostate cancer remains a significant global health challenge, but advancements in diagnostic and therapeutic strategies are continuously improving patient outcomes. The integration of AI into PCa management holds immense promise for revolutionizing early detection, risk stratification, treatment planning, and drug discovery. However, the ethical considerations surrounding AI deployment must be carefully addressed to ensure that these technologies are used responsibly and equitably. Future research should focus on developing AI algorithms that are accurate, unbiased, transparent, and explainable. Clinicians should be trained to effectively use AI tools and to communicate with patients about their role in care. By addressing these challenges, we can harness the power of AI to improve the lives of men with prostate cancer.
Many thanks to our sponsor Esdebe who helped us prepare this research report.
References
- American Cancer Society. (2023). Key Statistics for Prostate Cancer. Retrieved from https://www.cancer.org/cancer/prostate-cancer/about/key-statistics.html
- National Cancer Institute. (n.d.). Prostate Cancer Treatment (PDQ®)–Health Professional Version. Retrieved from https://www.cancer.gov/types/prostate/hp/prostate-treatment-pdq
- Rawla, P. (2019). Epidemiology of Prostate Cancer. World journal of oncology, 10(2), 63–89. https://doi.org/10.14740/wjon1191
- Litjens, G., et al. (2017). A survey on deep learning in medical image analysis. Medical Image Analysis, 42, 60–88.
- Obermeyer, Z., Powers, B. J., Vogeli, C., & Mullainathan, S. (2019). Dissecting racial bias in an algorithm used to manage the health of populations. Science, 366(6464), 447–453.
- European Association of Urology (EAU) Guidelines on Prostate Cancer 2023.
- Bansal, P., et al. (2022). Artificial intelligence for prostate cancer diagnosis and management. Nature Reviews Urology, 19(1), 19-34.
- Hosny, A., Parmar, C., Quackenbush, J., Schwartz, L. H., & Aerts, H. J. W. L. (2018). Artificial intelligence in radiology. Nature Reviews Cancer, 18(8), 500–510.
- Esteva, A., Kuprel, B., Novoa, R. A., Ko, J., Swani, S. M., Blau, H. M., Thrun, S., & Dean, J. (2017). Dermatologist-level classification of skin cancer with deep neural networks. Nature, 542(7639), 115–118.
- Spek, A., et al. (2021). Artificial intelligence in prostate cancer diagnostics: a systematic review. European Urology Open Science, 32, 37-50.
- IBM Watson Health. (n.d.). (Example citation – can be replaced if using IBM Watson Health explicitly).
The discussion on ethical considerations is crucial, especially regarding data privacy. As AI models analyze more patient data, what innovative approaches can be used to ensure robust data security and patient control over their health information?
Great point about data privacy! Beyond encryption, exploring federated learning could be a game-changer. It allows AI models to train on decentralized datasets without direct access, enhancing security. Giving patients more granular control over their data usage through blockchain tech could be a major step too.
Editor: MedTechNews.Uk
Thank you to our Sponsor Esdebe
The report highlights AI’s potential in early detection using mpMRI. Considering the variability in image quality and interpretation across different healthcare facilities, how can AI algorithms be standardized and validated to ensure consistent and reliable diagnostic outcomes in diverse clinical settings?
That’s a great question! Standardization across facilities is definitely key. Perhaps incorporating transfer learning techniques, where models are pre-trained on large, diverse datasets and then fine-tuned on local data, could help bridge the gap in image quality and interpretation. What are your thoughts on using synthetic data for augmentation?
Editor: MedTechNews.Uk
Thank you to our Sponsor Esdebe
AI optimizing radiation therapy? Let’s hope those algorithms are better at avoiding side effects than my last dating app experience. Anyone working on AI-driven solutions for *that* problem?
That’s a funny analogy! You raise a critical point. Minimizing side effects is paramount. AI can potentially personalize radiation plans to target the tumor more precisely and spare healthy tissue. We’re seeing research in this area focusing on adaptive planning that adjusts in real-time based on the patient’s response.
Editor: MedTechNews.Uk
Thank you to our Sponsor Esdebe
The discussion on AI’s role in early detection is compelling. Beyond mpMRI analysis, could AI algorithms be integrated with liquid biopsies to analyze circulating tumor cells or DNA, potentially offering a less invasive and more frequent monitoring method for high-risk individuals?
That’s a fantastic point! Exploring AI’s role in analyzing liquid biopsies is definitely an exciting avenue. Imagine the possibilities of real-time, less invasive monitoring. I believe this could be especially impactful for high-risk individuals and in tracking treatment response more dynamically. Thanks for bringing this up!
Editor: MedTechNews.Uk
Thank you to our Sponsor Esdebe