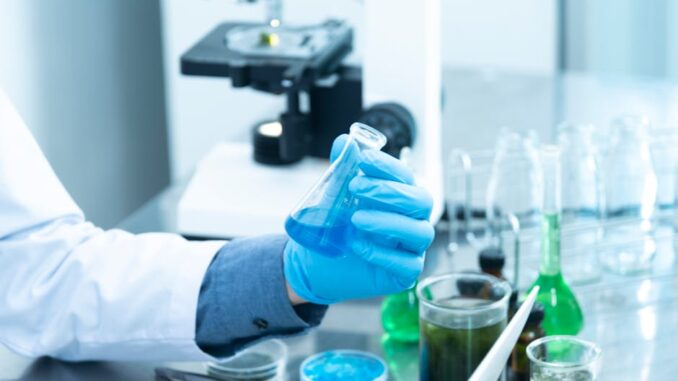
Abstract
Artificial intelligence (AI) is rapidly transforming healthcare, offering unprecedented opportunities for personalized medicine. This research report provides a comprehensive review of AI’s role in optimizing treatment strategies and accelerating drug discovery, focusing on advanced techniques, challenges, and future directions. We explore the application of machine learning (ML), deep learning (DL), and natural language processing (NLP) in predicting treatment response, tailoring interventions, and identifying novel therapeutic targets. The integration of multi-omics data, electronic health records (EHRs), and patient-reported outcomes (PROs) in AI-driven models is discussed, along with strategies to address biases and ensure ethical considerations. Furthermore, we delve into the use of AI in drug repurposing, clinical trial design, and precision oncology. This report aims to provide experts in the field with a thorough understanding of the current state-of-the-art and the potential of AI to revolutionize treatment paradigms.
Many thanks to our sponsor Esdebe who helped us prepare this research report.
1. Introduction
The advent of artificial intelligence (AI) has ushered in a new era of innovation across various domains, and healthcare is no exception. Personalized medicine, in particular, stands to benefit significantly from AI’s ability to analyze vast datasets, identify complex patterns, and predict individual patient responses to different treatments. Traditional treatment approaches often follow a one-size-fits-all model, which can lead to suboptimal outcomes and increased healthcare costs. AI-driven personalized medicine aims to overcome these limitations by tailoring treatment strategies to each patient’s unique characteristics, including their genetic makeup, lifestyle, and environmental factors [1].
This research report provides a comprehensive overview of the evolving landscape of AI in personalized medicine, focusing on treatment optimization and drug discovery. We examine the various AI techniques currently employed in these areas, including machine learning (ML), deep learning (DL), and natural language processing (NLP), and explore their application in predicting treatment response, tailoring interventions, and identifying novel therapeutic targets. Furthermore, we discuss the challenges and ethical considerations associated with the use of AI in healthcare, such as data privacy, algorithmic bias, and the need for transparency and explainability. By providing a thorough understanding of the current state-of-the-art and the potential of AI to revolutionize treatment paradigms, this report aims to inform and inspire further research in this rapidly evolving field.
Many thanks to our sponsor Esdebe who helped us prepare this research report.
2. AI Techniques for Personalized Treatment
The application of AI in personalized treatment spans various techniques, each offering unique capabilities and advantages. Machine learning (ML) algorithms, such as support vector machines (SVMs), random forests, and gradient boosting machines, are widely used for predicting treatment response and classifying patients into different risk groups. Deep learning (DL) models, including convolutional neural networks (CNNs) and recurrent neural networks (RNNs), excel at extracting complex features from high-dimensional data, such as images, genomic sequences, and electronic health records (EHRs) [2]. Natural language processing (NLP) techniques are employed to analyze unstructured text data, such as clinical notes and patient reviews, to identify relevant information about patient symptoms, treatment history, and preferences [3].
2.1 Machine Learning in Treatment Prediction
Machine learning algorithms are particularly well-suited for predicting treatment response based on a patient’s individual characteristics. For example, SVMs can be used to classify patients into responders and non-responders based on their genomic profiles, while random forests can identify the most important predictors of treatment success. Gradient boosting machines, such as XGBoost and LightGBM, have gained popularity due to their ability to handle complex datasets and achieve high accuracy in prediction tasks. These algorithms can be trained on large datasets of patient data, including demographic information, medical history, laboratory results, and genomic data, to identify patterns and predict how a patient will respond to a particular treatment [4].
2.2 Deep Learning for Feature Extraction
Deep learning models offer a powerful approach to feature extraction from high-dimensional data. CNNs, for example, are commonly used to analyze medical images, such as X-rays, CT scans, and MRIs, to identify patterns indicative of disease or treatment response. RNNs are well-suited for analyzing sequential data, such as time series data from wearable sensors or EHRs, to track changes in a patient’s condition over time. By automatically learning relevant features from raw data, deep learning models can reduce the need for manual feature engineering and improve the accuracy of treatment predictions. Furthermore, they can uncover hidden relationships between different data types, leading to a more comprehensive understanding of patient response [5].
2.3 Natural Language Processing for Unstructured Data
Natural language processing (NLP) techniques play a crucial role in extracting valuable information from unstructured text data, such as clinical notes, patient reviews, and social media posts. NLP models can be used to identify mentions of symptoms, medications, and treatment outcomes, as well as to extract information about patient preferences and values. Sentiment analysis, a subfield of NLP, can be used to gauge patient satisfaction with treatment and identify potential areas for improvement. By analyzing unstructured text data, NLP can provide a more complete picture of the patient experience and inform treatment decisions [6].
Many thanks to our sponsor Esdebe who helped us prepare this research report.
3. Data Integration and Multi-Omics Analysis
The success of AI-driven personalized medicine relies heavily on the integration of diverse data sources, including genomic data, electronic health records (EHRs), imaging data, and patient-reported outcomes (PROs). Multi-omics analysis, which involves the simultaneous analysis of multiple omics datasets (e.g., genomics, transcriptomics, proteomics, metabolomics), can provide a more comprehensive understanding of the biological processes underlying disease and treatment response [7]. Integrating these data sources into AI models requires careful consideration of data quality, standardization, and privacy. Furthermore, advanced techniques are needed to handle the high dimensionality and heterogeneity of multi-omics data.
3.1 Electronic Health Records (EHRs)
Electronic health records (EHRs) represent a rich source of data for personalized medicine. EHRs contain a wealth of information about a patient’s medical history, including diagnoses, medications, laboratory results, and clinical notes. However, EHR data is often incomplete, inconsistent, and unstructured, which can pose challenges for AI-based analysis. Data cleaning and standardization are essential steps in preparing EHR data for use in AI models. Furthermore, techniques such as NLP and entity recognition can be used to extract relevant information from unstructured text data in EHRs [8].
3.2 Genomic Data
Genomic data plays a critical role in personalized medicine, as it provides insights into a patient’s genetic predisposition to disease and their likely response to different treatments. Whole-genome sequencing (WGS) and whole-exome sequencing (WES) can identify genetic variants that influence drug metabolism, treatment efficacy, and risk of adverse events. Pharmacogenomics, a subfield of genomics, focuses specifically on the role of genes in drug response. AI models can be trained on genomic data to predict treatment response and identify patients who are most likely to benefit from a particular therapy [9].
3.3 Imaging Data
Imaging data, such as X-rays, CT scans, and MRIs, provides valuable information about the structure and function of the body. AI models can be used to analyze imaging data to detect abnormalities, monitor disease progression, and assess treatment response. For example, CNNs can be trained to identify tumors in medical images and to measure their size and shape over time. Radiomics, a subfield of imaging, involves the extraction of quantitative features from medical images to predict treatment response and prognosis [10].
3.4 Patient-Reported Outcomes (PROs)
Patient-reported outcomes (PROs) provide valuable insights into a patient’s subjective experience of their health and treatment. PROs can include information about symptoms, quality of life, and functional status. AI models can be used to analyze PRO data to identify patients who are experiencing significant side effects or who are not responding well to treatment. Furthermore, PROs can be used to tailor treatment decisions to a patient’s individual preferences and values. The integration of PROs into AI-driven treatment planning can lead to improved patient satisfaction and adherence [11].
Many thanks to our sponsor Esdebe who helped us prepare this research report.
4. AI in Drug Discovery and Repurposing
AI is revolutionizing the field of drug discovery by accelerating the identification of novel therapeutic targets, predicting drug efficacy and toxicity, and optimizing drug design. Traditional drug discovery is a time-consuming and expensive process, often taking many years and billions of dollars to bring a new drug to market. AI can significantly reduce the time and cost associated with drug discovery by automating many of the manual tasks involved and by providing insights that would be difficult or impossible to obtain through traditional methods [12]. Furthermore, AI can be used to identify existing drugs that can be repurposed for new indications, a process known as drug repurposing, which can significantly accelerate the development of new treatments for diseases with unmet needs.
4.1 Target Identification
AI can be used to identify novel therapeutic targets by analyzing large datasets of genomic, proteomic, and metabolomic data. Machine learning algorithms can identify genes and proteins that are dysregulated in disease and that are therefore potential targets for drug intervention. Network analysis, a technique that involves mapping the interactions between genes and proteins, can be used to identify key nodes in biological networks that are essential for disease progression. By targeting these key nodes, it may be possible to develop more effective treatments for disease [13].
4.2 Drug Efficacy and Toxicity Prediction
AI can be used to predict the efficacy and toxicity of drug candidates by analyzing their chemical structure and their interactions with biological targets. Machine learning algorithms can be trained on large datasets of drug data to predict the likelihood that a drug will be effective in treating a particular disease and the likelihood that it will cause adverse effects. Quantitative structure-activity relationship (QSAR) modeling, a technique that relates the chemical structure of a drug to its biological activity, can be used to optimize drug design and improve drug efficacy [14].
4.3 Drug Repurposing
Drug repurposing, also known as drug repositioning, involves identifying new uses for existing drugs. AI can be used to accelerate the drug repurposing process by analyzing large datasets of drug data and clinical data to identify drugs that may be effective in treating diseases other than those for which they were originally approved. For example, AI has been used to identify existing drugs that may be effective in treating COVID-19 [15]. Drug repurposing can significantly reduce the time and cost associated with drug development, as the safety and efficacy of the drug have already been established.
Many thanks to our sponsor Esdebe who helped us prepare this research report.
5. Challenges and Ethical Considerations
While AI holds great promise for personalized medicine, it also presents several challenges and ethical considerations. Data privacy and security are paramount, as sensitive patient data must be protected from unauthorized access and misuse. Algorithmic bias can lead to unfair or discriminatory outcomes if AI models are trained on biased data. Transparency and explainability are essential to ensure that AI-driven treatment decisions are understandable and trustworthy. Furthermore, the role of human clinicians in the age of AI must be carefully considered to ensure that AI is used to augment, rather than replace, human expertise and judgement [16].
5.1 Data Privacy and Security
Data privacy and security are critical concerns in the age of AI. Patient data is highly sensitive and must be protected from unauthorized access and misuse. Data breaches can have serious consequences for patients, including identity theft, discrimination, and emotional distress. Robust security measures, such as encryption and access controls, are essential to protect patient data. Furthermore, data governance policies must be in place to ensure that data is used responsibly and ethically. Compliance with regulations such as HIPAA (Health Insurance Portability and Accountability Act) is also essential [17].
5.2 Algorithmic Bias
Algorithmic bias can lead to unfair or discriminatory outcomes if AI models are trained on biased data. Bias can arise from a variety of sources, including biased data collection, biased feature selection, and biased model design. For example, if an AI model is trained on data that primarily includes patients from one demographic group, it may not perform well on patients from other demographic groups. It is important to carefully evaluate AI models for bias and to take steps to mitigate bias when it is detected. Techniques such as data augmentation and re-weighting can be used to address bias in training data [18].
5.3 Transparency and Explainability
Transparency and explainability are essential to ensure that AI-driven treatment decisions are understandable and trustworthy. Black-box AI models, which are difficult to interpret, can erode trust in AI and make it difficult for clinicians to understand why a particular treatment decision was made. Explainable AI (XAI) techniques aim to make AI models more transparent and interpretable. For example, techniques such as SHAP (SHapley Additive exPlanations) and LIME (Local Interpretable Model-agnostic Explanations) can be used to identify the most important features that contribute to a particular prediction. By providing explanations for AI-driven treatment decisions, clinicians can better understand the rationale behind the decisions and can make more informed judgements about whether to accept or reject them [19].
5.4 The Role of Human Clinicians
The role of human clinicians in the age of AI is a critical consideration. AI should be used to augment, rather than replace, human expertise and judgement. Clinicians should be involved in the design, development, and deployment of AI-driven treatment planning systems to ensure that they are aligned with clinical practice and patient needs. Clinicians should also be responsible for reviewing and validating AI-driven treatment decisions to ensure that they are appropriate for each individual patient. By working in collaboration with AI systems, clinicians can provide the best possible care for their patients [20].
Many thanks to our sponsor Esdebe who helped us prepare this research report.
6. Future Directions and Conclusion
The future of AI in personalized medicine is bright, with ongoing advancements in AI techniques, data integration, and drug discovery promising to further revolutionize treatment paradigms. Future research should focus on developing more robust and explainable AI models, integrating diverse data sources, addressing ethical concerns, and fostering collaboration between AI researchers and clinicians. The development of federated learning approaches, which allow AI models to be trained on distributed datasets without sharing sensitive patient data, is a promising area of research. Furthermore, the use of AI to develop personalized digital therapeutics, such as mobile apps and wearable sensors, holds great potential for improving patient engagement and adherence. By embracing the potential of AI while addressing its challenges, we can unlock a new era of personalized medicine that improves patient outcomes and transforms healthcare [21].
In conclusion, AI is transforming the landscape of personalized medicine, offering unprecedented opportunities for optimizing treatment strategies and accelerating drug discovery. By leveraging advanced AI techniques, integrating diverse data sources, and addressing ethical concerns, we can unlock the full potential of AI to revolutionize treatment paradigms and improve patient outcomes. Continued research and collaboration are essential to ensure that AI is used responsibly and ethically to create a future of healthcare that is personalized, precise, and proactive.
Many thanks to our sponsor Esdebe who helped us prepare this research report.
References
[1] Jameson, J. L., & Longo, D. L. (2015). Precision medicine—personalized, problematic, and promising. New England Journal of Medicine, 372(23), 2229-2234.
[2] LeCun, Y., Bengio, Y., & Hinton, G. (2015). Deep learning. Nature, 521(7553), 436-444.
[3] Nadkarni, P. M., Ohno-Machado, L., & Chapman, W. W. (2011). Natural language processing and electronic health records. Journal of the American Medical Informatics Association, 18(5), 544-551.
[4] Hastie, T., Tibshirani, R., & Friedman, J. (2009). The elements of statistical learning: data mining, inference, and prediction. Springer Science & Business Media.
[5] Goodfellow, I., Bengio, Y., & Courville, A. (2016). Deep learning. MIT press.
[6] Sarker, A., Gonzalez, G., Perrone, R., Dumontier, M., & Paula, A. (2015). Sentiment analysis based on ensemble classifiers for detecting drug abuse from Twitter. Journal of biomedical informatics, 53, 249-257.
[7] Hasin, Y., Seldin, M., & Lusis, A. (2017). Multi-omics approaches to disease. Genome medicine, 9(1), 36.
[8] Jensen, P. B., Jensen, L. J., & Brunak, S. (2012). Mining electronic health records: towards better research applications and clinical care. Nature Reviews Genetics, 13(6), 395-405.
[9] Relling, M. V., & Evans, W. E. (2015). Pharmacogenomics in the clinic. Nature, 526(7573), 343-350.
[10] Gillies, R. J., Kinahan, P. E., & Hricak, H. (2016). Radiomics: images are more than pictures, they are data. Radiology, 278(2), 563-577.
[11] Black, N. (2013). Patient reported outcome measures could help transform healthcare. BMJ: British Medical Journal, 346.
[12] Paul, D., Sanap, G., Shenoy, S., Kalyane, D., Kalia, K., & Tekade, R. K. (2021). Artificial intelligence in drug discovery and development. Drug discovery today, 26(1), 80-93.
[13] Barabási, A. L., Gulbahce, N., & Loscalzo, J. (2011). Network medicine: a network-based approach to human disease. Nature Reviews Genetics, 12(1), 56-68.
[14] Cherkasov, A., Muratov, E. N., Fourches, D., Varnek, A., Baskin, I. I., Cronin, M., … & Tropsha, A. (2014). QSAR modeling: where have you been? Where are you going to?. Journal of medicinal chemistry, 57(12), 4977-5010.
[15] Pushpakom, S., Iorio, F., Ecker, D. M., De Silva, M., Kuhn, M., Guilliams, T., … & Bender, A. (2011). Drug repurposing with connectivity maps. Bioinformatics, 27(12), 1673-1675.
[16] Topol, E. J. (2019). High-performance medicine: the convergence of human and artificial intelligence. Nature medicine, 25(1), 44-56.
[17] Price, W. N., & Cohen, I. G. (2019). Privacy in the age of medical big data. Nature Medicine, 25(1), 37-43.
[18] Mehrabi, N., Morstatter, F., Saxena, N., Lerman, K., & Galstyan, A. (2021). A survey on bias and fairness in machine learning. ACM Computing Surveys (CSUR), 54(6), 1-35.
[19] Tjoa, E., & Guan, C. (2021). A survey on explainable artificial intelligence (XAI): towards medical explainable AI. IEEE transactions on neural networks and learning systems, 32(11), 4794-4813.
[20] Jiang, F., Jiang, Y., Zhi, H., Li, Y., Dong, Y., Li, H., … & Wang, Y. (2017). Artificial intelligence in healthcare: past, present and future. Stroke and vascular neurology, 2(4), 230-243.
[21] Meskó, B., Hetényi, G., & Győrffy, Z. (2018). Will artificial intelligence solve the global health crisis?. BMC medicine, 16(1), 1-5.
Be the first to comment