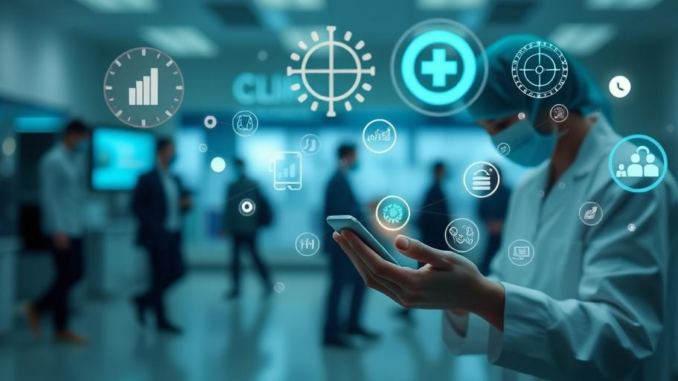
The Evolving Landscape of Clinical Trials: Innovation, Challenges, and the Future of Therapeutic Development
Abstract
Clinical trials are the cornerstone of evidence-based medicine, serving as the critical pathway for evaluating the safety and efficacy of new therapeutic interventions. This research report provides a comprehensive overview of the evolving landscape of clinical trials, examining the intricate phases involved, the complexities of trial design and execution, ethical considerations, and the pivotal role these trials play in translating scientific discoveries into tangible improvements in patient care. Beyond a descriptive overview, this report delves into the challenges inherent in conducting rigorous clinical trials, including patient recruitment and retention hurdles, the escalating demands of data management and analysis, and the ever-evolving regulatory environment. Furthermore, it explores innovative approaches and emerging trends that are poised to reshape the future of clinical trials, such as adaptive trial designs, decentralized trials, and the integration of real-world data, offering insights into how these advancements can enhance efficiency, reduce costs, and ultimately accelerate the development of novel therapies.
1. Introduction
The development of new therapies hinges on the successful completion of clinical trials, the rigorous scientific investigations designed to evaluate the safety and effectiveness of interventions in human subjects. These trials are not merely procedural hurdles but are integral to ensuring that medical advancements are both beneficial and safe for patients. The history of clinical trials is replete with examples of both groundbreaking successes and cautionary tales, highlighting the importance of robust methodologies and stringent ethical oversight. From the early observational studies of scurvy by James Lind to the complex, multi-center, randomized controlled trials (RCTs) that characterize modern drug development, the field has evolved significantly, driven by advancements in statistical methods, data science, and regulatory frameworks.
The impetus for change in clinical trials is multifaceted. Rising development costs, lengthy timelines, increasing regulatory complexity, and the need for more personalized and targeted therapies are all driving innovation in trial design and execution. Moreover, the digital revolution and the increasing availability of electronic health records, wearable sensors, and mobile technologies are opening up new avenues for data collection and remote patient monitoring. This report aims to provide an in-depth exploration of the current state of clinical trials, the challenges they face, and the innovative solutions that are shaping their future, offering insights relevant to experts in the pharmaceutical industry, regulatory agencies, and academic research institutions.
2. Phases of Clinical Trials
Clinical trials are typically conducted in a series of phases, each designed to answer specific research questions and build upon the knowledge gained in previous stages. These phases, while sequential, often overlap and can be adapted based on the specific nature of the intervention and the target patient population.
- Phase 0: Often termed ‘first-in-human’ studies, Phase 0 trials involve a very limited number of participants (typically 10-15) and are primarily focused on pharmacokinetics (PK) and pharmacodynamics (PD) to determine how the drug is processed in the body and what its initial effects are. The doses used are subtherapeutic, meaning they are not intended to produce a therapeutic effect, but rather to gather preliminary data on the drug’s behavior. Phase 0 trials are becoming increasingly relevant in oncology drug development, especially for targeted therapies.
- Phase 1: These trials assess the safety and tolerability of the intervention in a small group of healthy volunteers (typically 20-80 participants). The primary goal is to determine the maximum tolerated dose (MTD) and identify any potential side effects. While efficacy is not the main focus, some initial evidence of biological activity may be observed. Phase 1 trials are crucial for establishing the safety profile of a new therapy.
- Phase 2: Phase 2 trials are conducted in a larger group of patients (typically 100-300 participants) who have the condition or disease that the intervention is intended to treat. The focus shifts to evaluating the efficacy of the intervention and further assessing its safety profile. Phase 2 trials are often randomized and may include a placebo control group. These trials are critical for determining whether the intervention has the potential to be effective and for identifying the optimal dose and regimen for further study.
- Phase 3: Phase 3 trials are large, randomized, controlled trials (RCTs) conducted in hundreds or even thousands of patients. They are designed to definitively demonstrate the efficacy of the intervention in a real-world setting and to monitor for any adverse effects that may not have been detected in earlier phases. Phase 3 trials often involve multiple clinical sites and are critical for obtaining regulatory approval. These trials are generally the most expensive and time-consuming phase of clinical development.
- Phase 4: Also known as post-marketing surveillance, Phase 4 trials are conducted after the intervention has been approved and is available to the public. These trials are designed to monitor the long-term safety and efficacy of the intervention in a larger and more diverse patient population. They can also be used to identify rare or unexpected side effects and to explore new uses for the intervention. Phase 4 trials are essential for continuously monitoring the safety and effectiveness of medical products throughout their lifecycle.
3. Clinical Trial Design and Execution
The design of a clinical trial is paramount to its success. A well-designed trial ensures that the research question is addressed effectively, the data collected are reliable, and the conclusions drawn are valid. Several key elements contribute to a robust trial design:
- Study Population: Defining the target population is crucial. Inclusion and exclusion criteria must be carefully considered to ensure that the participants are representative of the population who will ultimately benefit from the intervention. Criteria must also minimize the risk of bias and confounding factors. The increasing emphasis on precision medicine necessitates more refined inclusion/exclusion criteria, targeting specific genetic profiles or biomarkers.
- Intervention and Control: The intervention being tested must be clearly defined, including the dose, route of administration, and duration of treatment. The control group may receive a placebo, a standard treatment, or no treatment at all. The choice of control group depends on the ethical considerations and the existing standard of care.
- Randomization: Random assignment of participants to the intervention or control group is essential to minimize bias and ensure that the groups are comparable at baseline. Various randomization techniques exist, including simple randomization, stratified randomization, and block randomization, each with its own advantages and disadvantages. Adaptive randomization methods, which adjust the probability of assignment based on accumulating data, are gaining traction.
- Blinding: Blinding (or masking) is a technique used to prevent bias by concealing the treatment assignment from the participants, the investigators, or both. In single-blind studies, the participants are unaware of their treatment assignment. In double-blind studies, both the participants and the investigators are unaware of the treatment assignment. Triple-blind studies extend the blinding to the data analysts. Blinding helps to minimize the influence of subjective factors on the outcome assessment.
- Outcome Measures: The primary and secondary outcome measures must be clearly defined and measurable. These measures should be relevant to the research question and clinically meaningful. The use of validated outcome measures is essential to ensure the reliability and validity of the data. Composite endpoints, which combine multiple outcome measures into a single endpoint, are often used to increase the statistical power of the study. However, their interpretation can be complex.
- Statistical Analysis: The statistical analysis plan should be developed before the study begins and should specify the statistical methods that will be used to analyze the data. The sample size must be calculated to ensure that the study has sufficient statistical power to detect a clinically meaningful difference between the intervention and control groups. Appropriate statistical methods must be used to account for potential confounding factors and to address missing data. The growing sophistication of statistical modeling, including Bayesian methods, offers improved analysis of complex clinical trial data.
The execution of a clinical trial involves a complex interplay of logistics, data management, and regulatory compliance. Patient recruitment and retention are often major challenges, requiring creative strategies to engage potential participants and maintain their commitment throughout the study. Data management involves the collection, cleaning, and storage of vast amounts of data, requiring robust systems and processes to ensure data integrity and security. Regulatory compliance involves adherence to a complex set of regulations and guidelines, including those issued by regulatory agencies such as the FDA and EMA. Any deviation from these regulations can have serious consequences, including delays in approval or even rejection of the application.
4. Ethical Considerations
Clinical trials raise significant ethical considerations that must be carefully addressed to protect the rights and well-being of participants. These considerations are guided by ethical principles such as respect for persons, beneficence, and justice.
- Informed Consent: Informed consent is the cornerstone of ethical clinical research. Participants must be fully informed about the purpose of the study, the procedures involved, the potential risks and benefits, and their right to withdraw from the study at any time without penalty. The informed consent process must be documented and must be conducted in a language that the participant understands. Special considerations are required for vulnerable populations, such as children, the elderly, and individuals with cognitive impairments.
- Equipoise: Equipoise refers to a state of genuine uncertainty among experts about the relative merits of the interventions being compared in a clinical trial. This principle requires that researchers only conduct trials when there is a legitimate scientific rationale for comparing the interventions and when there is no clear evidence that one intervention is superior to another. The concept of equipoise is often debated, particularly in the context of trials that compare a new intervention to a placebo when a standard treatment is available.
- Justice: The principle of justice requires that clinical trials be conducted fairly and equitably, ensuring that the benefits and burdens of research are distributed fairly across different populations. This includes ensuring that vulnerable populations are not disproportionately burdened by research and that they have equal access to the benefits of research. Special efforts are needed to ensure that clinical trials are representative of the populations who will ultimately benefit from the interventions being tested.
- Data Safety Monitoring: Data Safety Monitoring Boards (DSMBs) are independent groups of experts who are responsible for monitoring the safety and efficacy of clinical trials. DSMBs review the data on an ongoing basis and can recommend that the trial be stopped if there are concerns about safety or if there is clear evidence that one intervention is superior to another. DSMBs play a critical role in protecting the safety and well-being of participants in clinical trials.
- Privacy and Confidentiality: The privacy and confidentiality of participants must be protected throughout the clinical trial process. Data must be stored securely and accessed only by authorized personnel. Participants must be informed about how their data will be used and protected. The use of electronic health records and other digital technologies raises new challenges for protecting privacy and confidentiality.
Ethical review boards (IRBs) play a crucial role in ensuring that clinical trials are conducted ethically. IRBs are responsible for reviewing and approving research protocols to ensure that they meet ethical standards and that the rights and welfare of participants are adequately protected. IRBs are composed of individuals with diverse backgrounds and expertise, including physicians, scientists, ethicists, and community members.
5. Challenges in Clinical Trials
Despite the progress made in clinical trial design and execution, numerous challenges persist, hindering the efficiency and effectiveness of therapeutic development.
- Patient Recruitment and Retention: Recruiting and retaining patients in clinical trials is a major challenge. Many trials fail to meet their enrollment targets or experience high dropout rates. Factors contributing to this challenge include lack of awareness of clinical trials, distrust of the medical system, complex study protocols, geographic barriers, and financial constraints. Innovative recruitment strategies, such as the use of social media and mobile technologies, are needed to reach potential participants and improve enrollment rates. Patient-centered approaches, such as providing transportation assistance and flexible scheduling, can improve retention rates.
- Data Management and Analysis: Clinical trials generate vast amounts of data, requiring sophisticated data management and analysis systems. Ensuring data quality, integrity, and security is essential. The increasing use of electronic data capture (EDC) systems has improved data management efficiency, but challenges remain in integrating data from multiple sources and ensuring data interoperability. Advanced statistical methods, such as machine learning and artificial intelligence, are being used to analyze clinical trial data and identify patterns that may not be apparent using traditional methods.
- Regulatory Compliance: The regulatory environment for clinical trials is complex and constantly evolving. Navigating the regulatory landscape requires specialized expertise and resources. Compliance with regulations such as Good Clinical Practice (GCP) is essential to ensure the integrity and validity of clinical trial data. The use of risk-based monitoring approaches, which focus on the most critical aspects of the trial, can improve efficiency and reduce the burden of regulatory compliance.
- Cost and Time: Clinical trials are expensive and time-consuming. The cost of developing a new drug can reach billions of dollars, and the process can take many years. Streamlining clinical trial processes, such as using adaptive trial designs and decentralized trials, can reduce costs and accelerate the development of new therapies. Public-private partnerships can also help to share the costs and risks of clinical development.
- Reproducibility and Transparency: Concerns about the reproducibility and transparency of clinical trial data have increased in recent years. Selective reporting of results and lack of access to raw data can undermine the credibility of clinical research. Promoting data sharing and transparency is essential to improve the reproducibility of clinical trial findings and build public trust in clinical research. Initiatives such as the AllTrials campaign are advocating for greater transparency in clinical trials.
6. Emerging Trends and Innovations
Several emerging trends and innovations are poised to reshape the future of clinical trials, offering the potential to improve efficiency, reduce costs, and accelerate the development of new therapies.
- Adaptive Trial Designs: Adaptive trial designs allow for modifications to the study protocol based on accumulating data. These modifications can include changes to the sample size, the treatment arms, or the outcome measures. Adaptive designs can improve the efficiency of clinical trials by allowing for faster decision-making and by reducing the number of patients needed to achieve statistical significance. However, adaptive designs require careful planning and statistical expertise to ensure that the integrity of the study is maintained.
- Decentralized Clinical Trials (DCTs): Decentralized clinical trials (DCTs), also known as remote or virtual trials, are conducted using digital technologies to reduce the need for patients to travel to clinical sites. DCTs can improve patient recruitment and retention by making it easier for patients to participate in trials. DCTs can also reduce costs by eliminating the need for expensive clinical facilities. However, DCTs require careful planning to ensure that data quality and patient safety are maintained.
- Real-World Data (RWD) and Real-World Evidence (RWE): Real-world data (RWD) are data collected outside of traditional clinical trials, such as data from electronic health records, claims databases, and wearable sensors. Real-world evidence (RWE) is the evidence generated from the analysis of RWD. RWD and RWE can be used to supplement clinical trial data and to provide a more comprehensive understanding of the safety and effectiveness of medical products. RWD and RWE can also be used to support regulatory decision-making and to inform clinical practice guidelines. The use of RWD and RWE raises new challenges for data privacy and security, as well as for ensuring the quality and reliability of the data.
- Artificial Intelligence (AI) and Machine Learning (ML): Artificial intelligence (AI) and machine learning (ML) are being used to improve various aspects of clinical trials, including patient recruitment, data management, and outcome prediction. AI and ML can be used to identify potential participants for clinical trials, to automate data entry and cleaning, and to predict which patients are most likely to respond to a particular treatment. However, the use of AI and ML in clinical trials raises new challenges for transparency and interpretability, as well as for ensuring that the algorithms are not biased.
- Patient-Centric Trials: Patient-centric trials are designed to meet the needs and preferences of patients. These trials involve patients in the design and execution of the study and prioritize outcomes that are meaningful to patients. Patient-centric trials can improve patient recruitment and retention and can lead to more relevant and impactful research findings. Patient advocacy groups are playing an increasingly important role in advocating for patient-centric trials.
7. Conclusion
Clinical trials are essential for advancing medical knowledge and improving patient care. While significant progress has been made in clinical trial design and execution, numerous challenges remain. These challenges include patient recruitment and retention, data management, regulatory compliance, and cost. Emerging trends and innovations, such as adaptive trial designs, decentralized trials, real-world data, and artificial intelligence, offer the potential to address these challenges and to accelerate the development of new therapies. However, the successful implementation of these innovations requires careful planning, collaboration, and a commitment to ethical principles.
The future of clinical trials will likely be characterized by greater efficiency, flexibility, and patient-centricity. As technology continues to evolve and as our understanding of disease biology deepens, clinical trials will become increasingly sophisticated and personalized. By embracing innovation and by addressing the challenges that lie ahead, we can ensure that clinical trials continue to play a vital role in improving the health and well-being of patients around the world.
References
- ICH. (2016). E6(R2) Good Clinical Practice: Integrated Addendum to ICH E6(R1). Retrieved from https://database.ich.org/sites/default/files/E6_R2_Addendum.pdf
- FDA. (2019). Framework for FDA’s Real-World Evidence Program. Retrieved from https://www.fda.gov/media/120060/download
- EMA. (2021). Guideline on computerised systems and electronic data in clinical trials. Retrieved from https://www.ema.europa.eu/en/documents/scientific-guideline/guideline-computerised-systems-electronic-data-clinical-trials_en.pdf
- Getz, K. A., Stergachis, A., & Schulman, K. A. (2016). The costs of drug development: A systematic review. PharmacoEconomics, 34(9), 907-918.
- Kent, D. M., Hayward, R. A., Griffith, J., Veenstra, D. L., Beshansky, J. R., Lau, J., & Chalmers, T. C. (2001). Reporting of subgroup analyses in clinical trials. Annals of Internal Medicine, 135(6), 423-427.
- Byrne, A. J. (2019). Clinical trials: A review. JAMA, 321(11), 1105-1106.
- Chalmers, I., Glasziou, P., & Godlee, F. (2016). All Trials registered: the next steps. BMJ, 352, i907.
- European Medicines Agency. (2023). Clinical Trials. Retrieved from https://www.ema.europa.eu/en/human-regulatory/research-development/clinical-trials
- US Food and Drug Administration. (2023). Clinical Trials and Human Subject Protection. Retrieved from https://www.fda.gov/science-research/science-and-research-special-topics/clinical-trials-and-human-subject-protection
Decentralized trials sound promising, but how do we ensure my grandma doesn’t accidentally skew the data while trying to Facetime her cat during her remote blood pressure check? Asking for science!
That’s a fantastic question! We are exploring user-friendly tech and thorough training for participants. Imagine visual cues or voice prompts guiding the process! Regular check-ins and data validation will also help us catch any “cat-astrophic” interference. Your grandma’s tech skills are valuable for testing these trials!
Editor: MedTechNews.Uk
Thank you to our Sponsor Esdebe
Fascinating! With AI predicting patient responses, will we soon see trials where the control group gets prescribed placebos…chosen by algorithms for their *lack* of effect? Is the future of “sugar pills” personalized too?
That’s a brilliant point! The idea of AI tailoring placebos to minimize perceived effects is a truly innovative concept. It raises interesting questions about the future of control groups and how we might refine our understanding of the placebo effect itself. Perhaps personalized medicine extends beyond active treatments!
Editor: MedTechNews.Uk
Thank you to our Sponsor Esdebe
Decentralized trials sound great until my Roomba starts collecting real-world data on my mobility, and suddenly my “healthy” walking pace is being judged by an algorithm.
That’s a hilarious and insightful concern! The ethical implications of ambient data collection are definitely something we need to address proactively. Imagine the possibilities (and pitfalls) if our smart homes become unwitting participants in clinical research. Clear guidelines and user control are essential!
Editor: MedTechNews.Uk
Thank you to our Sponsor Esdebe