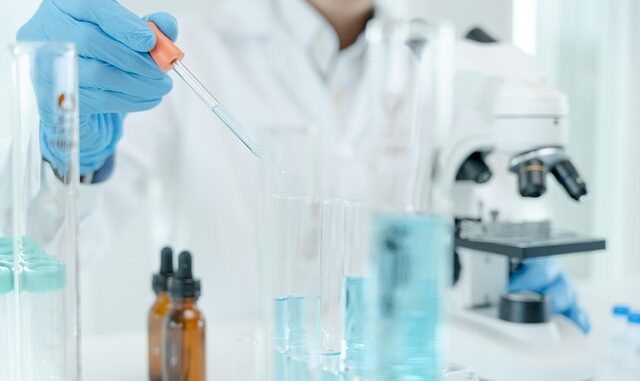
Abstract
Genomic research has undergone a profound transformation in recent decades, driven by advancements in sequencing technologies, computational power, and data analysis methodologies. This report provides a comprehensive overview of the current state of genome research, examining key areas from data generation and analysis to ethical considerations and translational applications. We delve into the intricacies of diverse genomic datasets, including whole-genome sequencing, transcriptomics, epigenomics, and proteomics, and explore the integration of these datasets to gain a holistic understanding of biological processes. The report also addresses the computational challenges associated with handling and analyzing vast genomic datasets, emphasizing the need for innovative algorithms and infrastructure. Furthermore, we critically evaluate the ethical, legal, and social implications (ELSI) of genomic research, focusing on issues such as data privacy, informed consent, and equitable access to genomic technologies. Finally, we highlight the potential of genomics to revolutionize medicine, agriculture, and other fields, while also acknowledging the challenges and limitations that must be addressed to realize its full potential. This report offers expert insights into the dynamic and ever-evolving field of genome research, providing a roadmap for future investigations and applications.
Many thanks to our sponsor Esdebe who helped us prepare this research report.
1. Introduction
The advent of high-throughput sequencing technologies has ushered in a new era in biology, characterized by the ability to generate and analyze genomic data on an unprecedented scale. What started with the Human Genome Project – a monumental effort to sequence the entire human genome – has evolved into a dynamic and multifaceted field encompassing diverse areas such as personalized medicine, disease diagnostics, drug discovery, and evolutionary biology. The initial promise of genomics was to understand the underlying causes of human diseases and to develop targeted therapies based on an individual’s genetic makeup. While this vision is still unfolding, significant progress has been made in identifying disease-associated genes, understanding gene regulation, and elucidating the complex interplay between genes and the environment.
The scope of genomic research extends far beyond the human genome. Comparative genomics, for instance, allows us to study the evolutionary relationships between different species and to identify conserved genomic regions that are essential for life. Metagenomics, on the other hand, enables the study of microbial communities in various environments, from the human gut to the deep sea, providing insights into microbial diversity and their roles in ecosystem function. Plant genomics is crucial for improving crop yields, developing disease-resistant varieties, and adapting crops to changing climatic conditions.
This report aims to provide a comprehensive overview of the current state of genome research, highlighting the key advances, challenges, and future directions in the field. We will discuss the various types of genomic data, the methods used to generate and analyze them, the ethical considerations surrounding genomic research, and the potential applications of genomic information in medicine, agriculture, and other fields. We will also address the computational challenges associated with data storage and processing, as well as the ongoing efforts to create more comprehensive and diverse genomic databases.
Many thanks to our sponsor Esdebe who helped us prepare this research report.
2. Genomic Data Types and Generation
Genomic research relies on a variety of data types, each providing unique insights into the structure, function, and regulation of the genome. These data types can be broadly classified into the following categories:
- Whole-Genome Sequencing (WGS): WGS involves determining the complete DNA sequence of an organism’s genome. This provides a comprehensive view of the genetic makeup of an individual or species, allowing for the identification of genetic variations, such as single nucleotide polymorphisms (SNPs), insertions, deletions, and structural variants. WGS is typically performed using high-throughput sequencing platforms, such as Illumina, PacBio, and Oxford Nanopore.
- Exome Sequencing: Exome sequencing focuses on sequencing the protein-coding regions of the genome, which constitute only about 1-2% of the total genome. This is a more cost-effective approach than WGS and is often used to identify disease-causing mutations in Mendelian disorders. Target enrichment methods are used to selectively capture the exome before sequencing.
- Transcriptomics (RNA-Seq): Transcriptomics involves studying the expression levels of genes by sequencing RNA molecules. RNA-Seq provides a snapshot of the transcriptome, revealing which genes are actively transcribed in a particular cell type or tissue at a specific time. This information is crucial for understanding gene regulation, cellular differentiation, and disease pathogenesis.
- Epigenomics: Epigenomics investigates the chemical modifications of DNA and histones that influence gene expression without altering the DNA sequence itself. These modifications, such as DNA methylation and histone acetylation, can affect chromatin structure and accessibility, thereby regulating gene transcription. Epigenomic data is generated using techniques such as chromatin immunoprecipitation sequencing (ChIP-Seq) and bisulfite sequencing.
- Metagenomics: Metagenomics involves studying the genetic material recovered directly from environmental samples. This approach allows for the characterization of microbial communities without the need for culturing individual organisms. Metagenomic data can provide insights into microbial diversity, metabolic pathways, and their roles in ecosystem function. 16S rRNA gene sequencing and shotgun metagenomic sequencing are common methods used in metagenomics.
- Proteomics: Proteomics studies the entire set of proteins expressed by an organism or cell. Mass spectrometry is the primary technique used in proteomics to identify and quantify proteins. Proteomic data can provide insights into protein-protein interactions, post-translational modifications, and cellular signaling pathways.
The generation of genomic data typically involves several steps, including sample preparation, DNA or RNA extraction, library preparation, sequencing, and data processing. Each step requires careful optimization and quality control to ensure the accuracy and reliability of the data. The choice of sequencing platform and sequencing depth depends on the specific research question and the type of genomic data being generated.
Many thanks to our sponsor Esdebe who helped us prepare this research report.
3. Genomic Data Analysis and Interpretation
Analyzing genomic data is a complex and computationally intensive task that requires specialized tools and expertise. The analysis pipeline typically involves several steps, including:
- Read Alignment: The first step in analyzing sequencing data is to align the reads to a reference genome. This involves identifying the location in the reference genome where each read originated. Alignment algorithms, such as BWA and Bowtie, are used to perform this task.
- Variant Calling: After read alignment, the next step is to identify genetic variations, such as SNPs, insertions, deletions, and structural variants. Variant calling algorithms, such as GATK and FreeBayes, are used to detect these variations.
- Gene Expression Quantification: In transcriptomics, the goal is to quantify the expression levels of genes. This involves counting the number of reads that map to each gene and normalizing these counts to account for differences in library size and gene length. Tools like RSEM and Salmon are used for gene expression quantification.
- Differential Expression Analysis: Differential expression analysis aims to identify genes that are differentially expressed between different experimental conditions. Statistical methods, such as DESeq2 and edgeR, are used to perform this analysis.
- Pathway Analysis: Pathway analysis involves identifying biological pathways that are enriched in a set of differentially expressed genes or proteins. This can provide insights into the biological processes that are affected by the experimental conditions. Tools like DAVID and KEGG are used for pathway analysis.
- Genome-Wide Association Studies (GWAS): GWAS are used to identify genetic variants that are associated with a particular trait or disease. This involves analyzing the genomes of a large number of individuals with and without the trait or disease and identifying SNPs that are significantly more common in one group than the other. Statistical methods, such as logistic regression, are used to perform GWAS.
- Machine Learning and Artificial Intelligence: Machine learning algorithms are increasingly being used in genomic research to predict disease risk, identify drug targets, and personalize treatment strategies. These algorithms can analyze vast amounts of genomic data and identify complex patterns that are not readily apparent to humans. Deep learning, a type of machine learning, has shown promising results in various genomic applications.
The interpretation of genomic data requires careful consideration of the biological context and the limitations of the data. It is important to validate findings using independent datasets and experimental approaches. Moreover, the integration of genomic data with other types of data, such as clinical data, environmental data, and lifestyle data, can provide a more comprehensive understanding of biological processes and disease etiology.
Many thanks to our sponsor Esdebe who helped us prepare this research report.
4. Ethical, Legal, and Social Implications (ELSI) of Genomic Research
The rapid advances in genomic research have raised a number of ethical, legal, and social implications (ELSI) that must be carefully considered. These include:
- Data Privacy and Security: Genomic data is highly sensitive and personal, and its privacy and security must be protected. There is a risk that genomic data could be used to discriminate against individuals or groups, or to identify individuals without their consent. Robust data security measures, such as encryption and access controls, are necessary to protect genomic data from unauthorized access.
- Informed Consent: Individuals who participate in genomic research must provide informed consent, which means that they must understand the purpose of the research, the risks and benefits of participation, and their rights to withdraw from the study at any time. It is challenging to obtain truly informed consent in genomic research, as the potential uses of genomic data are often unknown at the time of collection. Broad consent models, which allow for the use of genomic data for future research purposes, are becoming increasingly common, but they raise concerns about autonomy and control.
- Data Ownership and Access: The ownership and access rights to genomic data are complex and contested issues. Who owns the genomic data generated from an individual’s sample? Should individuals have the right to access their own genomic data? Should researchers have the right to share genomic data with other researchers? These questions have no easy answers and require careful consideration of the interests of all stakeholders.
- Genetic Discrimination: Genetic discrimination occurs when individuals are treated differently based on their genetic information. This can occur in a variety of contexts, such as employment, insurance, and healthcare. Laws prohibiting genetic discrimination have been enacted in some countries, but these laws may not provide complete protection.
- Equitable Access to Genomic Technologies: Genomic technologies are not equally accessible to all populations. There is a risk that genomic research could exacerbate existing health disparities if it is primarily focused on affluent populations. Efforts must be made to ensure that genomic technologies are accessible to all populations, regardless of their socioeconomic status or geographic location.
- Misinterpretation and Misuse of Genomic Information: Genomic information can be complex and difficult to interpret, and there is a risk that it could be misinterpreted or misused. For example, a genetic variant that is associated with a particular disease may not necessarily cause the disease. It is important to communicate genomic information to the public in a clear and accurate manner, and to avoid making claims that are not supported by scientific evidence.
Addressing these ELSI requires a multi-faceted approach involving researchers, ethicists, policymakers, and the public. It is essential to develop ethical guidelines and regulations that protect the rights and interests of individuals and communities, while also promoting the responsible conduct of genomic research.
Many thanks to our sponsor Esdebe who helped us prepare this research report.
5. Applications of Genomic Information
Genomic information has a wide range of potential applications in medicine, agriculture, and other fields. Some of the most promising applications include:
- Personalized Medicine: Personalized medicine aims to tailor medical treatments to an individual’s genetic makeup. This involves using genomic information to predict an individual’s risk of developing a particular disease, to select the most effective drug for that individual, and to monitor the individual’s response to treatment. Pharmacogenomics, the study of how genes affect a person’s response to drugs, is a key component of personalized medicine.
- Disease Diagnostics: Genomic information can be used to diagnose diseases, particularly genetic disorders. Genetic testing can be used to identify disease-causing mutations in individuals with suspected genetic disorders, or to screen individuals for genetic predispositions to certain diseases. Non-invasive prenatal testing (NIPT) uses genomic sequencing of fetal DNA in maternal blood to screen for chromosomal abnormalities in the fetus.
- Drug Discovery: Genomic information can be used to identify new drug targets and to develop more effective drugs. By understanding the genetic basis of disease, researchers can identify genes and proteins that are involved in the disease process and develop drugs that target these molecules. CRISPR-Cas9 gene editing technology is being used to develop new therapies for genetic diseases.
- Agriculture: Genomic information can be used to improve crop yields, develop disease-resistant varieties, and adapt crops to changing climatic conditions. Marker-assisted selection (MAS) uses genetic markers to select plants with desirable traits, such as high yield or disease resistance. Genetically modified (GM) crops have been developed that are resistant to pests, herbicides, or drought.
- Forensic Science: Genomic information can be used to identify individuals in forensic investigations. DNA fingerprinting, which involves analyzing highly variable regions of the genome, is used to match DNA samples found at crime scenes to suspects. DNA phenotyping, which involves predicting an individual’s physical appearance from their DNA, is also being used in forensic investigations.
- Evolutionary Biology: Genomic information can be used to study the evolutionary relationships between different species and to understand the genetic basis of adaptation. Comparative genomics, which involves comparing the genomes of different species, can provide insights into the evolution of genes and genomes. Ancient DNA analysis, which involves sequencing DNA extracted from ancient remains, can provide insights into the history of human populations.
The translation of genomic research into practical applications requires significant investment in infrastructure, technology, and training. It also requires close collaboration between researchers, clinicians, industry, and policymakers. While the potential benefits of genomic information are enormous, it is important to proceed cautiously and to address the ethical, legal, and social implications of genomic research.
Many thanks to our sponsor Esdebe who helped us prepare this research report.
6. Challenges and Future Directions
Despite the remarkable progress in genomic research, a number of challenges remain. These include:
- Data Storage and Processing: The vast amounts of genomic data generated by high-throughput sequencing technologies pose a significant challenge for data storage and processing. Cloud computing and distributed computing are being used to address this challenge. Novel data compression algorithms are also needed to reduce the storage requirements for genomic data.
- Data Integration: Integrating genomic data with other types of data, such as clinical data, environmental data, and lifestyle data, is essential for gaining a comprehensive understanding of biological processes and disease etiology. However, data integration can be challenging due to differences in data formats, data quality, and data access policies. Standardized data formats and data sharing policies are needed to facilitate data integration.
- Data Interpretation: Interpreting genomic data is a complex task that requires specialized expertise. Many genetic variants are of unknown function, and it is difficult to predict their effects on phenotype. Improved computational methods and experimental approaches are needed to improve our ability to interpret genomic data.
- Reproducibility: The reproducibility of genomic research is a growing concern. Many genomic studies are not reproducible due to methodological flaws, statistical errors, or publication bias. Efforts are being made to improve the reproducibility of genomic research by developing standardized protocols, promoting data sharing, and encouraging replication studies.
- Diversity: Genomic databases are currently biased towards individuals of European ancestry. This limits the generalizability of genomic findings and hinders the development of personalized medicine for individuals of other ancestries. Efforts are being made to increase the diversity of genomic databases by recruiting participants from underrepresented populations.
The future of genomic research is bright. Advances in sequencing technologies, computational methods, and data analysis techniques are paving the way for new discoveries and applications. Some of the key future directions in genomic research include:
- Long-Read Sequencing: Long-read sequencing technologies, such as PacBio and Oxford Nanopore, are enabling the sequencing of long DNA fragments, which can improve the accuracy of genome assembly and variant calling. Long-read sequencing is particularly useful for studying structural variants, which are often difficult to detect using short-read sequencing.
- Single-Cell Genomics: Single-cell genomics is a rapidly growing field that involves studying the genomes, transcriptomes, and epigenomes of individual cells. This can provide insights into cellular heterogeneity, cell differentiation, and disease pathogenesis.
- Spatial Transcriptomics: Spatial transcriptomics is a new technology that allows for the measurement of gene expression in tissue sections while preserving spatial information. This can provide insights into the organization of tissues and the interactions between cells.
- Artificial Intelligence and Deep Learning: Artificial intelligence and deep learning are being used to develop new methods for analyzing genomic data, predicting disease risk, and personalizing treatment strategies. These technologies have the potential to revolutionize genomic research and medicine.
By addressing the challenges and pursuing these future directions, genomic research will continue to advance our understanding of biology and improve human health.
Many thanks to our sponsor Esdebe who helped us prepare this research report.
7. Conclusion
Genome research has emerged as a cornerstone of modern biological and medical science. From its origins in mapping the human genome to its current applications in personalized medicine, drug discovery, and agriculture, the field continues to evolve at an accelerating pace. This report has highlighted the diverse types of genomic data, the complex methods for their analysis, and the crucial ethical considerations that must guide their use. The integration of large, diverse datasets, coupled with advances in computational power and innovative algorithms, holds immense promise for unlocking the secrets of life and improving human health. However, challenges remain in data storage, processing, interpretation, and ensuring equitable access to genomic technologies. By addressing these challenges and fostering collaboration among researchers, clinicians, policymakers, and the public, we can harness the full potential of genomics to transform medicine and other fields for the benefit of all.
Many thanks to our sponsor Esdebe who helped us prepare this research report.
References
- International HapMap Consortium. (2003). The International HapMap Project. Nature, 426(6968), 789-796.
- Lander, E. S., Linton, L. M., Birren, B., et al. (2001). Initial sequencing and analysis of the human genome. Nature, 409(6822), 860-921.
- Venter, J. C., Adams, M. D., Myers, E. W., et al. (2001). The sequence of the human genome. Science, 291(5507), 1304-1351.
- Goodwin, S., McPherson, J. D., & McCombie, W. R. (2016). Coming of age: ten years of next-generation sequencing technologies. Nature Reviews Genetics, 17(6), 333-351.
- Consortium, T. E. P. (2012). An integrated encyclopedia of DNA elements in the human genome. Nature, 489(7414), 57-74.
- Koboldt, D. C., Zhang, Q., Larson, D. E., et al. (2012). VarScan 2: somatic mutation and copy number alteration discovery in cancer by exome sequencing. Genome Biology, 13(9), R77.
- Anders, S., Pyl, P. T., & Huber, W. (2015). HTSeq–a Python framework to work with high-throughput sequencing data. Bioinformatics, 31(2), 166-169.
- Love, M. I., Huber, W., & Anders, S. (2014). Moderated estimation of fold change and dispersion for RNA-seq data with DESeq2. Genome Biology, 15(12), 550.
- Huang da, W., Sherman, B. T., & Lempicki, R. A. (2009). Systematic and integrative analysis of large gene lists using DAVID bioinformatics resources. Nature Protocols, 4(1), 44-57.
- Pearson, T. A., Manolio, T. A. (2008). How to interpret a genome-wide association study. JAMA, 299(11), 1335-1344.
- Telenti, A., Schwab, M. E., & Panese, G. (2015). Data sharing: what makes it ethical?. BMC Medical Ethics, 16(1), 56.
- Caulfield, T., McGuire, A. L., Cho, M., Buchanan, J. A., Burgess, M. M., Danilczyk, U., … & Wilson, B. J. (2008). Research ethics recommendations for whole-genome research: consensus statement. PLoS Biology, 6(3), e73.
- Ashley, E. A. (2015). Towards precision medicine. The Lancet, 385(9962), 39-45.
- Collins, F. S., & Varmus, H. (2015). A new initiative on precision medicine. New England Journal of Medicine, 372(9), 793-795.
- Jinek, M., Chylinski, K., Fonfara, I., Hauer, M., Doudna, J. A., & Charpentier, E. (2012). A programmable dual-RNA-guided DNA endonuclease in adaptive bacterial immunity. Science, 337(6096), 816-821.
- Varshney, R. K., Graner, A., & Sorrells, M. E. (2005). Genomic approaches for crop improvement. Trends in Plant Science, 10(12), 621-630.
- Butler, J. M. (2015). DNA typing: current trends and future directions. Nature Reviews Genetics, 6(7), 559-571.
- Allentoft, M. E., Sikora, M., Sjögren, K. G., Rasmussen, S., Rasmussen, M., Stenderup, M., … & Willerslev, E. (2015). Population genomics of Bronze Age Eurasia. Nature, 522(7555), 167-172.
- Marx, V. (2013). Biology: The big challenges of big data. Nature, 498(7453), 255-260.
- Erlich, Y., & Narayanan, A. (2014). Routes for breaching and protecting genetic privacy. Nature Reviews Genetics, 15(6), 409-421.
- Durbin, R. (2010). Biological sequence analysis in the era of high-throughput genomics. Nature Genetics, 42(1), 18-27.
So, basically, we’re all just walking around with incredibly complex, personalized instruction manuals that we’re only just beginning to understand? Suddenly, user manuals for my IKEA furniture seem a lot less daunting.
That’s a fantastic analogy! It really puts the complexity into perspective. And you’re right, deciphering the genome is a much bigger puzzle than assembling flat-pack furniture. Maybe one day we’ll have AI assistants to help us navigate our own instruction manuals!
Editor: MedTechNews.Uk
Thank you to our Sponsor Esdebe
So, after all that effort to sequence and analyze everything, are we *really* any closer to knowing which genes are responsible for my questionable taste in music? Inquiring minds want to know!
That’s a fun question! While we might not pinpoint specific ‘music taste genes’ just yet, understanding gene networks could reveal predispositions to certain preferences or emotional responses to music. The complexity is fascinating!
Editor: MedTechNews.Uk
Thank you to our Sponsor Esdebe
So, are we going to need bigger hard drives or smaller humans to cope with all this genomic data? Asking for a friend (who may or may not be a server farm).