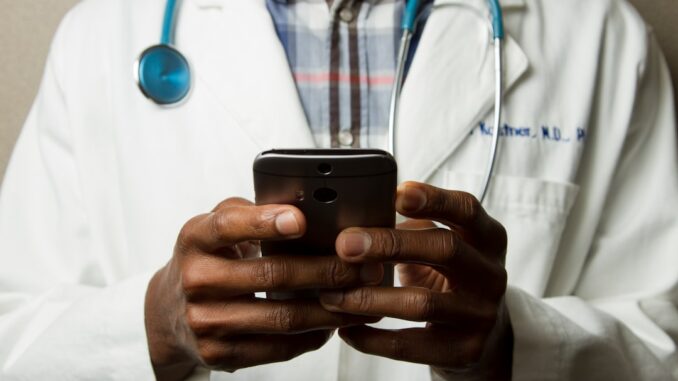
Abstract
Patient-Reported Outcome Measures (PROMs) have become increasingly integral to healthcare, offering a direct assessment of patients’ perspectives on their health status, functional capacity, and overall well-being. This research report provides a comprehensive overview of the evolving landscape of PROMs, moving beyond a simple description of their types and applications. We delve into advanced methodological considerations, explore persistent implementation challenges, and critically analyze future directions for the field. We examine the advancements in psychometric validation techniques, including item response theory (IRT) and network analysis, and discuss the complexities of adapting PROMs for diverse populations and cultural contexts. We also investigate the impact of technological advancements, such as electronic PROMs (ePROMs) and machine learning, on data collection, analysis, and personalized healthcare delivery. Furthermore, the report addresses ethical considerations surrounding the use of PROMs, particularly in vulnerable populations, and highlights the need for patient-centered approaches in PROM development and implementation. Finally, we propose future research directions to address existing gaps and enhance the utility of PROMs in improving patient outcomes and healthcare quality.
Many thanks to our sponsor Esdebe who helped us prepare this research report.
1. Introduction
The patient-centered care paradigm has placed increasing emphasis on incorporating the patient’s voice into clinical decision-making and healthcare evaluation. Patient-Reported Outcome Measures (PROMs) serve as a crucial tool in this endeavor, providing a standardized and quantifiable method for capturing patients’ subjective experiences of their health (Greenhalgh, 2009). Unlike traditional clinical measures that focus on physiological or objective indicators, PROMs directly assess patients’ perceptions of their symptoms, functional limitations, and overall quality of life (QoL). This information is invaluable for understanding the impact of disease and treatment from the patient’s perspective, thereby facilitating more informed and personalized care.
The field of PROMs has witnessed significant advancements in recent years, with the development of sophisticated methodologies for instrument design, validation, and implementation. These advancements have broadened the applicability of PROMs across diverse healthcare settings, including clinical trials, routine clinical practice, and population health surveillance (Calvert et al., 2013). However, challenges remain in ensuring the validity, reliability, and responsiveness of PROMs across different patient populations and cultural contexts. Furthermore, the effective integration of PROMs into clinical workflows requires careful consideration of logistical, technological, and ethical issues.
This research report aims to provide a comprehensive overview of the current state of PROMs research, focusing on methodological advancements, implementation challenges, and future directions. We will critically examine the strengths and limitations of existing PROMs, explore innovative approaches to PROM development and validation, and discuss strategies for overcoming barriers to PROM implementation. By synthesizing the existing literature and highlighting key areas for future research, this report seeks to contribute to the advancement of PROMs as a valuable tool for improving patient outcomes and healthcare quality.
Many thanks to our sponsor Esdebe who helped us prepare this research report.
2. Methodological Advancements in PROM Development and Validation
2.1. Item Response Theory (IRT)
Classical Test Theory (CTT) has historically been the dominant framework for PROM development and validation. However, CTT-based approaches have limitations, including sample dependency and inability to compare scores across different instruments (DeVellis, 2016). Item Response Theory (IRT) offers a more sophisticated and nuanced approach to PROM analysis, providing item-level information and allowing for the development of computer-adaptive testing (CAT) (Embretson & Reise, 2000). IRT models estimate the probability of a respondent endorsing a particular item as a function of their underlying trait level (e.g., pain intensity, functional ability). This allows for the creation of item banks that can be tailored to individual patients, reducing respondent burden and improving measurement precision.
Furthermore, IRT enables differential item functioning (DIF) analysis, which assesses whether items function differently for different subgroups of respondents (e.g., based on age, gender, or ethnicity). Identifying and addressing DIF is crucial for ensuring the fairness and validity of PROMs across diverse populations (Millsap, 2011). Although IRT offers significant advantages over CTT, it requires larger sample sizes and more specialized statistical expertise, which can be a barrier to its widespread adoption.
2.2. Network Analysis
Traditional PROM validation methods often assume that items are measuring a single, unidimensional construct. However, many PROMs assess complex and multidimensional concepts, such as quality of life or mental health. Network analysis provides a novel approach to understanding the relationships between PROM items and the underlying constructs they represent (Epskamp et al., 2018). Network analysis treats PROM items as nodes in a network, with edges representing the statistical associations between items. This allows for the identification of central items that are strongly connected to other items and may represent key indicators of the underlying construct. Network analysis can also reveal clusters of items that form distinct subdomains within the PROM, providing insights into the multidimensionality of the construct.
Furthermore, network analysis can be used to examine the dynamic relationships between PROM items over time, providing valuable information about the evolution of symptoms and the impact of interventions (Bringmann et al., 2013). While network analysis is a promising approach for PROM validation, it is still a relatively new technique and requires further research to establish its validity and reliability in different contexts.
2.3. Qualitative Methods
Quantitative methods are crucial for establishing the psychometric properties of PROMs, but they often fail to capture the richness and complexity of patients’ lived experiences. Qualitative methods, such as interviews and focus groups, can provide valuable insights into the relevance, comprehensibility, and acceptability of PROMs from the patient’s perspective (Doyle et al., 2012). Qualitative research can be used to identify important concepts that may not be adequately captured by existing PROMs and to inform the development of new items that are more relevant and meaningful to patients.
Furthermore, qualitative methods can be used to explore the cognitive processes that patients use when responding to PROM items, helping to identify potential sources of response bias and improve the clarity of item wording (Willis, 2015). Integrating qualitative and quantitative methods in PROM development and validation can lead to more robust and patient-centered instruments.
Many thanks to our sponsor Esdebe who helped us prepare this research report.
3. Implementation Challenges and Strategies
3.1. Ensuring Age-Appropriateness and Cultural Sensitivity
A significant challenge in PROM implementation is ensuring that instruments are age-appropriate and culturally sensitive for the target population. Children and adolescents may have difficulty understanding the language and concepts used in adult PROMs, requiring the development of specialized instruments that are tailored to their developmental stage (Eiser & Morse, 2001). Similarly, cultural differences in values, beliefs, and communication styles can influence how patients interpret and respond to PROM items, necessitating the adaptation and validation of PROMs for different cultural contexts (Herdman et al., 2011).
Translation and linguistic validation are essential steps in adapting PROMs for different languages, but they are not sufficient to ensure cultural equivalence. Cognitive interviewing and focus groups with members of the target culture can help to identify potential cultural biases and ensure that the PROM is measuring the intended construct in a culturally meaningful way (Beaton et al., 2000).
3.2. Minimizing Response Bias
Response bias can significantly affect the validity and reliability of PROM data. Common types of response bias include social desirability bias (the tendency to respond in a way that is seen as favorable by others), acquiescence bias (the tendency to agree with statements regardless of their content), and extreme response bias (the tendency to choose extreme response options) (Paulhus, 1991).
Several strategies can be used to minimize response bias, including ensuring anonymity and confidentiality, using neutral language in item wording, and providing clear and concise instructions. Furthermore, forced-choice response formats (e.g., choosing between two equally desirable or undesirable options) can reduce social desirability bias (Tourangeau et al., 2000). Statistical techniques, such as the use of response style scales and the application of latent variable modeling, can also be used to detect and adjust for response bias in PROM data (Meade & Craig, 2012).
3.3. Integrating PROMs into Clinical Workflows
The successful implementation of PROMs in clinical practice requires careful consideration of logistical and technological factors. Integrating PROMs into existing clinical workflows can be challenging, particularly in busy healthcare settings with limited resources. Electronic PROMs (ePROMs) offer a promising solution to streamline data collection and reduce administrative burden. ePROMs can be administered via computers, tablets, or smartphones, allowing patients to complete questionnaires at their convenience, either in the clinic or at home (Basch et al., 2013).
However, ensuring equitable access to ePROMs for all patients is crucial, particularly for those who lack access to technology or have limited digital literacy. Providing alternative modes of administration, such as paper-based questionnaires or telephone interviews, can help to overcome this barrier (Irvine et al., 2013). Furthermore, training healthcare providers on how to interpret and utilize PROM data is essential for integrating PROMs into clinical decision-making (Marshall et al., 2015).
Many thanks to our sponsor Esdebe who helped us prepare this research report.
4. Technological Applications of PROMs
4.1. Electronic PROMs (ePROMs) and Mobile Health (mHealth)
The advent of electronic PROMs (ePROMs) has revolutionized data collection, offering increased efficiency, reduced administrative burden, and improved data quality compared to traditional paper-based methods. ePROMs can be administered through various platforms, including web-based portals, mobile apps, and tablet devices, allowing patients to complete questionnaires at their convenience and in a setting that is comfortable for them (Weldring & Smith, 2013). Mobile health (mHealth) technologies, such as smartphones and wearable sensors, further enhance the potential of ePROMs by enabling real-time monitoring of patient symptoms and functional status. This continuous data collection can provide valuable insights into the dynamic nature of disease and treatment response, facilitating personalized care and proactive intervention (Kumar et al., 2013).
However, the successful implementation of ePROMs and mHealth technologies requires careful consideration of usability, accessibility, and data security. Ensuring that ePROMs are user-friendly and accessible to patients with diverse technological skills and physical limitations is crucial. Furthermore, protecting the privacy and security of patient data is paramount, requiring the implementation of robust security measures and adherence to ethical guidelines (Huckvale et al., 2015).
4.2. Machine Learning and Personalized Healthcare
Machine learning (ML) algorithms have the potential to unlock new insights from PROM data and facilitate personalized healthcare delivery. ML can be used to identify patterns and predictors of patient outcomes, enabling the development of predictive models that can identify patients at risk of adverse events or treatment failure (Obermeyer & Emanuel, 2016). Furthermore, ML can be used to personalize treatment recommendations based on individual patient characteristics and preferences, as captured by PROMs. For example, ML algorithms can analyze PROM data to identify patients who are most likely to benefit from a particular intervention or who are at risk of experiencing specific side effects (Austin, 2012).
However, the use of ML in healthcare raises several ethical concerns, including the potential for bias and discrimination. ML algorithms are trained on historical data, which may reflect existing biases in healthcare delivery. If these biases are not addressed, ML algorithms can perpetuate and even amplify them, leading to inequitable outcomes for certain patient populations. Ensuring fairness, transparency, and accountability in the development and deployment of ML algorithms is crucial for realizing the full potential of ML in personalized healthcare (Angus et al., 2020).
4.3. Natural Language Processing (NLP) for Analyzing Unstructured Patient Data
While PROMs provide structured, quantifiable data on patient experiences, much valuable information remains trapped in unstructured formats, such as clinical notes, patient narratives, and social media posts. Natural language processing (NLP) techniques can be used to extract meaningful information from these unstructured data sources, complementing and enriching PROM data. NLP can automatically identify and extract key concepts, such as symptoms, emotions, and concerns, from patient text, providing a more comprehensive understanding of the patient’s perspective (Sarker et al., 2018).
Furthermore, NLP can be used to analyze patient narratives to identify unmet needs and areas for improvement in healthcare delivery. By analyzing patient feedback, healthcare providers can gain valuable insights into the patient experience and tailor their services to better meet patient needs. However, the use of NLP in healthcare requires careful consideration of data privacy and ethical concerns. Ensuring that patient data is anonymized and used responsibly is crucial for maintaining patient trust and protecting patient rights (Uzuner et al., 2011).
Many thanks to our sponsor Esdebe who helped us prepare this research report.
5. Ethical Considerations
5.1. Informed Consent and Data Privacy
The ethical use of PROMs requires careful attention to informed consent and data privacy. Patients should be fully informed about the purpose of PROMs, how their data will be used, and their right to refuse to participate. The informed consent process should be tailored to the patient’s level of understanding, particularly for vulnerable populations such as children and individuals with cognitive impairments (Appelbaum et al., 2007). Protecting the privacy and security of patient data is paramount, requiring the implementation of robust data security measures and adherence to relevant regulations, such as the Health Insurance Portability and Accountability Act (HIPAA) in the United States (HIPAA, 1996).
5.2. Cultural Sensitivity and Avoiding Bias
PROMs should be developed and implemented in a culturally sensitive manner to avoid perpetuating existing biases and inequalities. Cultural differences in values, beliefs, and communication styles can influence how patients interpret and respond to PROM items. Ensuring that PROMs are adapted and validated for different cultural contexts is crucial for ensuring their validity and relevance (Herdman et al., 2011). Furthermore, healthcare providers should be aware of their own biases and assumptions and strive to provide equitable care to all patients, regardless of their cultural background.
5.3. Use of PROMs in Vulnerable Populations
The use of PROMs in vulnerable populations, such as children, individuals with cognitive impairments, and individuals from marginalized communities, requires special consideration. These populations may be more susceptible to coercion and undue influence, and their ability to provide informed consent may be compromised. Healthcare providers should take extra precautions to ensure that these patients are protected and that their rights are respected. Furthermore, specialized PROMs may be needed to assess the unique needs and experiences of these populations (Eiser & Morse, 2001).
Many thanks to our sponsor Esdebe who helped us prepare this research report.
6. Future Directions
6.1. Developing More Patient-Centered PROMs
Future research should focus on developing PROMs that are more patient-centered and responsive to the needs and preferences of individual patients. This includes incorporating patient input into the design and development of PROMs, using qualitative methods to understand patient experiences, and developing PROMs that are tailored to specific patient populations and conditions. Furthermore, PROMs should be designed to be easy to use and understand, minimizing respondent burden and maximizing patient engagement.
6.2. Integrating PROMs with Other Data Sources
Future research should explore the integration of PROMs with other data sources, such as clinical data, genomic data, and social determinants of health data, to provide a more comprehensive understanding of patient health and well-being. This integrated approach can facilitate personalized care and improve the effectiveness of interventions. Furthermore, integrating PROMs with electronic health records (EHRs) can streamline data collection and improve the accessibility of PROM data for healthcare providers.
6.3. Developing and Evaluating Novel Interventions Based on PROM Data
Future research should focus on developing and evaluating novel interventions that are based on PROM data. This includes using PROM data to identify patients at risk of adverse events or treatment failure, developing personalized interventions to address specific patient needs, and evaluating the effectiveness of these interventions in improving patient outcomes. Furthermore, research should explore the use of PROM data to inform shared decision-making between patients and healthcare providers, empowering patients to take an active role in their care.
Many thanks to our sponsor Esdebe who helped us prepare this research report.
7. Conclusion
Patient-Reported Outcome Measures (PROMs) represent a vital tool for capturing the patient’s voice in healthcare, offering insights into their subjective experiences of health, functionality, and well-being. This report has highlighted the significant advancements in PROM methodologies, particularly in the areas of IRT, network analysis, and the integration of qualitative methods, leading to more robust and nuanced instruments. However, the successful implementation of PROMs is not without its challenges. Ensuring age-appropriateness, cultural sensitivity, minimizing response bias, and seamlessly integrating PROMs into clinical workflows remain critical considerations.
The technological landscape offers exciting possibilities for enhancing PROM data collection and analysis, with ePROMs, mHealth, machine learning, and NLP playing increasingly important roles. However, these advancements must be approached with careful consideration of ethical implications, including data privacy, algorithmic bias, and the potential for misuse in vulnerable populations.
Looking forward, the field of PROMs needs to prioritize patient-centered approaches, integrating patient input into every stage of PROM development and implementation. Further research is needed to explore the integration of PROMs with other data sources, such as clinical, genomic, and social determinants of health data, to provide a more holistic understanding of patient health. Ultimately, the goal is to leverage PROM data to develop and evaluate novel interventions that are tailored to individual patient needs and preferences, leading to improved outcomes and a more patient-centric healthcare system.
Many thanks to our sponsor Esdebe who helped us prepare this research report.
References
- Angus, D. C., Carlet, J., & da Cruz, A. M. (2020). Ethical issues in using big data and artificial intelligence to transform healthcare. Critical Care, 24(1), 1-7.
- Appelbaum, P. S., Lidz, C. W., & Klitzman, R. (2007). Informed consent for research involving human subjects. Archives of General Psychiatry, 64(5), 545-554.
- Austin, P. C. (2012). An introduction to propensity score methods for reducing the effects of confounding in observational studies. Multivariate Behavioral Research, 46(3), 399-424.
- Basch, E., Abernethy, A. P., Reeve, B. B., Mitchell, S. A., & McDonagh, M. (2013). Patient-reported outcomes in comparative effectiveness research: opportunities and challenges. Medical Care, 51(8 Suppl 3), S69-S75.
- Beaton, D. E., Bombardier, C., Guillemin, F., & Ferraz, M. B. (2000). Guidelines for the process of cross-cultural adaptation of self-report measures. Spine, 25(24), 3186-3191.
- Bringmann, L. F., Vissers, N., Wichers, M., Geschwind, N., Kuppens, P., Peeters, F., … & Borsboom, D. (2013). A network approach to psychopathology: new insights into clinical longitudinal data. PloS one, 8(4), e60188.
- Calvert, M., Brundage, M., Jacobsen, P. B., Schünemann, H. J., & Efficace, F. (2013). The CONSORT PRO extension checklist: report of the ISPOR Task Force on Patient-Reported Outcome (PRO) endpoints. Value in Health, 16(8), 966-974.
- DeVellis, R. F. (2016). Scale development: Theory and applications. Sage publications.
- Doyle, J., Crowe, S., & Lambert, K. (2012). Qualitative research in health: An introduction. Journal of the Royal College of Physicians of Edinburgh, 42(1), 67-72.
- Eiser, C., & Morse, R. (2001). A review of measures of quality of life for children with chronic illness. Archives of Disease in Childhood, 84(3), 205-211.
- Embretson, S. E., & Reise, S. P. (2000). Item response theory for psychologists. Psychology press.
- Epskamp, S., Cramer, A. O., Waldorp, L. J., Schmittmann, V. D., & Borsboom, D. (2012). qgraph: Network visualizations of relationships in psychometric data. Journal of Statistical Software, 48(4), 1-24.
- Greenhalgh, T. (2009). Patient and public involvement in research: beyond tokenism. BMJ, 338, a867.
- Herdman, M., Fox-Rushby, J., Badia, X., Ayuso-Mateos, J. L., Drukker, M., Ferrer, M., … & Rajmil, L. (2011). A model of equivalence in the cultural adaptation of HRQoL instruments: the universalist approach. Quality of Life Research, 20(5), 661-679.
- HIPAA. (1996). Health Insurance Portability and Accountability Act of 1996. Public Law 104-191, 104th Congress.
- Huckvale, K., Car, J., & Gunn, L. H. (2015). Framework for the impact assessment of healthcare apps. BMC medicine, 13(1), 1-11.
- Irvine, D., Baker, A. H., Smith, K., Jandhyala, R., Gillies, K., Campbell, D., … & Williams, B. (2013). Development and initial evaluation of a postal questionnaire for measuring patient reported outcomes following elective hip replacement: the HOOS, JR. BMC musculoskeletal disorders, 14(1), 1-11.
- Kumar, S., Nilsen, W. J., Abernethy, A., Atienza, A., Patrick, K., Pavel, M., … & Swendeman, D. (2013). Mobile health technology for better health. Journal of the American Medical Informatics Association, 20(e2), e228-e232.
- Marshall, S., Haywood, K., & Fitzpatrick, R. (2015). Impact of patient-reported outcome measures on routine clinical practice: a structured review. BMJ, 350, g1102.
- Meade, A. W., & Craig, S. B. (2012). Identifying careless responses in survey data. Psychological Methods, 17(3), 437.
- Millsap, R. E. (2011). Statistical approaches to measurement invariance. Psychology Press.
- Obermeyer, Z., & Emanuel, E. J. (2016). Predicting the future—big data, machine learning, and clinical medicine. New England Journal of Medicine, 375(13), 1216-1219.
- Paulhus, D. L. (1991). Measurement and control of response bias. In J. P. Robinson, P. R. Shaver, & L. S. Wrightsman (Eds.), Measures of personality and social psychological attitudes (pp. 17-59). Academic Press.
- Sarker, A., Gonzalez, G., Perrone, J., & Uzuner, Ö. (2018). Sentiment analysis of suicide-related posts in online forums. JAMIA open, 1(1), 26-31.
- Tourangeau, R., Rips, L. J., & Rasinski, K. (2000). The psychology of survey response. Cambridge University Press.
- Uzuner, Ö., South, B. R., Shen, S., & Denny, J. C. (2011). 2010 i2b2/VA challenge on concepts, assertions, and relations in clinical text. Journal of the American Medical Informatics Association, 18(5), 552-556.
- Weldring, T., & Smith, S. M. (2013). Patient-reported outcomes (PROs). BMJ, 347, f4012.
- Willis, G. B. (2015). Cognitive interviewing: A tool for improving questionnaire design. Sage publications.
The emphasis on integrating qualitative methods alongside quantitative approaches could greatly enhance PROMs. Capturing nuanced patient experiences and perspectives seems essential for developing truly patient-centered and effective measures. I wonder how this integration could be standardized across different healthcare settings?