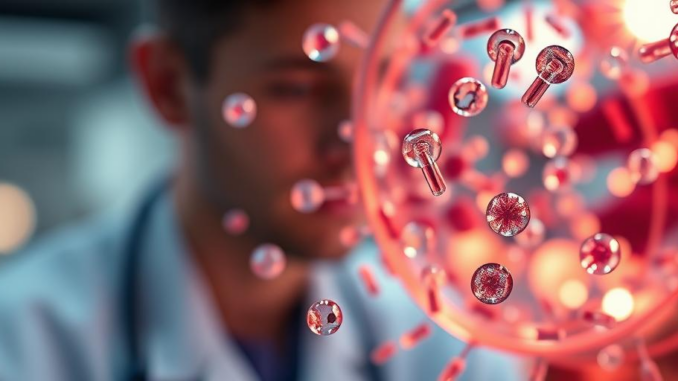
Abstract
Biomarkers, measurable indicators of biological state or condition, are rapidly transforming healthcare. While historically used primarily for disease monitoring, the field is evolving towards early disease detection, prediction of treatment response, and personalized therapeutic strategies. This review provides an in-depth examination of the latest advancements in biomarker research, encompassing discovery techniques, validation methodologies, and diverse clinical applications. We explore various biomarker types, including genomics, proteomics, metabolomics, and imaging biomarkers, highlighting their strengths and limitations. Furthermore, we address the critical challenges of translating biomarker research into clinical practice, emphasizing the need for rigorous validation, standardization, and the integration of multi-omics data for a holistic understanding of disease. Finally, we discuss future directions in biomarker research, focusing on the potential of artificial intelligence, liquid biopsies, and point-of-care diagnostics to revolutionize disease management and usher in an era of precision medicine.
Many thanks to our sponsor Esdebe who helped us prepare this research report.
1. Introduction
The quest for improved disease diagnosis and treatment has driven relentless innovation in biomedical research. A central pillar of this progress is the identification and utilization of biomarkers – objective measures that reflect a specific biological or pathological process. The National Institutes of Health (NIH) defines a biomarker as “a characteristic that is objectively measured and evaluated as an indicator of normal biological processes, pathogenic processes, or pharmacologic responses to a therapeutic intervention.” This broad definition encompasses a vast range of molecules, cellular components, and imaging modalities that can provide valuable insights into an individual’s health status.
The impact of biomarkers is felt across the entire spectrum of healthcare, from early disease detection and risk stratification to personalized therapy and monitoring of treatment efficacy. Classic examples include the use of prostate-specific antigen (PSA) for prostate cancer screening and cholesterol levels for assessing cardiovascular risk. However, recent advancements in high-throughput technologies and data analysis have propelled biomarker research into a new era, enabling the identification of increasingly complex and sophisticated biomarkers with improved sensitivity and specificity.
This review aims to provide a comprehensive overview of the current state of biomarker research, focusing on the latest advancements in discovery, validation, and clinical application. We will delve into the diverse types of biomarkers, discuss the challenges associated with their development, and explore future directions that hold the promise of transforming healthcare through personalized medicine.
Many thanks to our sponsor Esdebe who helped us prepare this research report.
2. Biomarker Discovery: Unveiling the Molecular Signatures of Disease
The initial step in biomarker development is the discovery phase, where potential biomarkers are identified and characterized. This process typically involves comparing biological samples (e.g., blood, tissue, urine) from diseased and healthy individuals to identify molecules or patterns that are differentially expressed or modified. A variety of techniques are employed in biomarker discovery, each with its strengths and limitations.
2.1. Genomics
Genomic biomarkers include DNA sequence variations (single nucleotide polymorphisms, copy number variations), gene expression patterns (mRNA levels), and epigenetic modifications (DNA methylation, histone acetylation). Genome-wide association studies (GWAS) have been instrumental in identifying genetic variants associated with various diseases, although the effect size of individual variants is often small. Microarray technology and RNA sequencing (RNA-seq) allow for the simultaneous measurement of the expression levels of thousands of genes, providing a comprehensive snapshot of the cellular transcriptome. Epigenome-wide association studies (EWAS) are emerging as a powerful tool for identifying epigenetic modifications associated with disease risk and progression. A key challenge in genomic biomarker discovery is the high degree of inter-individual variability and the influence of environmental factors on gene expression and epigenetic marks.
2.2. Proteomics
Proteomics focuses on the study of the entire protein complement of a cell, tissue, or organism. Mass spectrometry (MS) is the workhorse of proteomics research, enabling the identification and quantification of thousands of proteins in a complex biological sample. Various MS-based approaches are used in biomarker discovery, including label-free quantification, isotope labeling, and targeted proteomics. Protein microarrays, another proteomic technology, allow for the high-throughput detection of specific proteins using antibodies. Post-translational modifications (PTMs) play a crucial role in regulating protein function and are often dysregulated in disease. Therefore, the identification and characterization of PTMs are an important aspect of proteomic biomarker discovery. Limitations of proteomics include the complexity of the proteome, the dynamic range of protein abundance, and the challenges of identifying low-abundance proteins.
2.3. Metabolomics
Metabolomics involves the comprehensive analysis of small molecules (metabolites) in a biological sample. These metabolites are the end products of cellular metabolism and can provide valuable insights into the physiological state of an organism. Nuclear magnetic resonance (NMR) spectroscopy and mass spectrometry (MS) are the two primary analytical techniques used in metabolomics. Metabolomics is particularly well-suited for identifying biomarkers of metabolic disorders, such as diabetes and obesity, as well as biomarkers of drug response. The relatively small size and structural diversity of metabolites present analytical challenges, requiring sophisticated separation and detection methods.
2.4. Lipidomics
Lipidomics is a specialized branch of metabolomics that focuses on the comprehensive analysis of lipids, a diverse class of molecules involved in various biological processes, including energy storage, cell signaling, and membrane structure. Mass spectrometry-based techniques, often coupled with liquid chromatography, are the primary tools for lipidomics analysis. Lipidomics has proven particularly valuable in identifying biomarkers for cardiovascular disease, metabolic syndrome, and neurological disorders. The structural complexity and isomeric diversity of lipids present significant analytical challenges.
2.5. Imaging Biomarkers
Imaging biomarkers utilize non-invasive imaging techniques, such as magnetic resonance imaging (MRI), computed tomography (CT), positron emission tomography (PET), and ultrasound, to visualize and quantify biological processes in vivo. These biomarkers can provide valuable information about disease progression, treatment response, and prognosis. For example, tumor size and volume, as measured by MRI or CT, are commonly used as biomarkers in cancer clinical trials. PET imaging, using radioactive tracers that bind to specific targets, can provide information about metabolic activity and receptor expression. The development of new imaging agents and improved image analysis techniques is expanding the scope of imaging biomarkers.
2.6. Multi-omics Integration
Increasingly, biomarker discovery efforts are incorporating a multi-omics approach, integrating data from genomics, proteomics, metabolomics, and other omics platforms. This holistic approach can provide a more comprehensive understanding of disease mechanisms and lead to the identification of more robust and informative biomarkers. However, the integration of multi-omics data presents significant analytical and computational challenges, requiring sophisticated bioinformatics tools and statistical methods. Machine learning algorithms are increasingly being used to identify patterns and relationships within multi-omics datasets.
Many thanks to our sponsor Esdebe who helped us prepare this research report.
3. Biomarker Validation: Ensuring Reliability and Accuracy
Once potential biomarkers have been identified, it is crucial to validate their reliability and accuracy before they can be used in clinical practice. Biomarker validation involves a series of rigorous experiments designed to assess the sensitivity, specificity, reproducibility, and clinical utility of the biomarker.
3.1. Analytical Validation
Analytical validation focuses on the performance characteristics of the assay used to measure the biomarker. This includes assessing the assay’s accuracy, precision, sensitivity, specificity, linearity, and stability. Standardized protocols and quality control measures are essential for ensuring the reliability of biomarker measurements. Reference materials and proficiency testing programs can help to ensure the accuracy and reproducibility of biomarker assays across different laboratories.
3.2. Clinical Validation
Clinical validation assesses the ability of the biomarker to accurately predict a clinical outcome, such as disease risk, diagnosis, prognosis, or treatment response. This typically involves conducting prospective clinical studies in well-defined patient populations. The sensitivity and specificity of the biomarker are assessed, as well as its positive and negative predictive values. Receiver operating characteristic (ROC) curve analysis is commonly used to evaluate the diagnostic performance of a biomarker. It is critical to consider potential confounding factors that may influence the biomarker’s performance, such as age, sex, ethnicity, and co-morbidities.
3.3. Qualification
Biomarker qualification is the process of providing evidence that a biomarker is suitable for a specific purpose in drug development or clinical practice. This typically involves demonstrating that the biomarker is associated with a clinically relevant outcome and that it can be reliably measured in a defined population. Regulatory agencies, such as the FDA and EMA, play a crucial role in establishing standards and guidelines for biomarker qualification.
3.4. Challenges in Biomarker Validation
Biomarker validation is a complex and challenging process. One of the main challenges is the lack of standardized protocols and quality control measures for many biomarker assays. Another challenge is the high cost and time required to conduct prospective clinical studies. The heterogeneity of patient populations and the influence of environmental factors can also complicate biomarker validation. Furthermore, the lack of access to well-characterized biobanks with high-quality samples can hinder biomarker research. It’s worth expressing an opinion here – current processes for validation are far too slow and expensive, slowing the clinical use of biomarkers. Investment in accelerating this process is critical.
Many thanks to our sponsor Esdebe who helped us prepare this research report.
4. Clinical Applications of Biomarkers: Transforming Disease Management
Biomarkers are playing an increasingly important role in all aspects of disease management, from early detection and risk stratification to personalized therapy and monitoring of treatment efficacy.
4.1. Early Disease Detection and Risk Stratification
Biomarkers can be used to detect diseases at an early stage, before symptoms appear, when treatment is more likely to be effective. For example, liquid biopsies, which involve the analysis of circulating tumor cells (CTCs) and cell-free DNA (cfDNA) in blood, are being developed as a non-invasive approach for early cancer detection. Biomarkers can also be used to stratify individuals based on their risk of developing a particular disease. For example, genetic risk scores, based on multiple genetic variants, can be used to assess an individual’s risk of developing cardiovascular disease or type 2 diabetes. This is an area of huge potential, but also potential ethical and social pitfalls which must be navigated carefully.
4.2. Diagnosis and Prognosis
Biomarkers are routinely used in the diagnosis of many diseases. For example, cardiac troponins are used to diagnose myocardial infarction, and liver enzymes are used to assess liver function. Biomarkers can also provide valuable prognostic information, helping to predict the likely course of a disease and identify patients who are at high risk of adverse outcomes. For example, the Gleason score, based on the microscopic appearance of prostate cancer cells, is used to predict the risk of disease progression.
4.3. Personalized Therapy
Biomarkers are increasingly being used to guide treatment decisions, tailoring therapy to the individual characteristics of each patient. This is particularly important in cancer, where biomarkers can be used to predict response to specific therapies and identify patients who are most likely to benefit from a particular treatment. For example, EGFR mutations in lung cancer can predict response to EGFR inhibitors, and HER2 overexpression in breast cancer can predict response to trastuzumab. Pharmacogenomics, the study of how genes affect a person’s response to drugs, is another important aspect of personalized therapy.
4.4. Monitoring Treatment Efficacy
Biomarkers can be used to monitor the effectiveness of treatment and detect early signs of disease recurrence. For example, PSA levels are monitored in patients with prostate cancer to detect disease recurrence after surgery or radiation therapy. Circulating tumor DNA (ctDNA) can also be used to monitor treatment response and detect minimal residual disease in cancer patients. The use of biomarkers for monitoring treatment efficacy can help to optimize treatment regimens and improve patient outcomes. Again, liquid biopsies offer a less invasive method of monitoring than traditional methods.
Many thanks to our sponsor Esdebe who helped us prepare this research report.
5. Future Directions: The Biomarker Revolution Continues
The field of biomarker research is rapidly evolving, driven by technological advancements and a growing understanding of disease mechanisms. Several promising areas of research hold the potential to revolutionize disease management in the years to come.
5.1. Artificial Intelligence and Machine Learning
Artificial intelligence (AI) and machine learning (ML) are transforming biomarker research by enabling the analysis of large and complex datasets, identifying patterns and relationships that would be impossible to detect using traditional statistical methods. AI and ML algorithms are being used to predict disease risk, diagnose diseases, predict treatment response, and identify new biomarker candidates. These technologies are particularly well-suited for integrating multi-omics data and developing personalized treatment strategies. The need for large, well-annotated datasets to train AI and ML models remains a major challenge.
5.2. Liquid Biopsies
Liquid biopsies, which involve the analysis of circulating biomarkers in blood or other bodily fluids, are gaining increasing attention as a non-invasive approach for disease diagnosis, monitoring, and treatment selection. Liquid biopsies can provide a real-time snapshot of the disease state and can be used to detect early signs of disease recurrence or treatment resistance. Various types of circulating biomarkers are being investigated, including circulating tumor cells (CTCs), cell-free DNA (cfDNA), exosomes, and microRNAs. Standardization of sample collection and analysis methods is critical for the widespread adoption of liquid biopsies.
5.3. Point-of-Care Diagnostics
Point-of-care (POC) diagnostics, which allow for rapid and convenient biomarker testing at the patient’s bedside or in the physician’s office, are poised to revolutionize disease management. POC diagnostics can provide immediate results, enabling timely treatment decisions and improved patient outcomes. Various POC technologies are being developed, including microfluidic devices, biosensors, and lateral flow assays. POC diagnostics are particularly valuable in resource-limited settings and for managing acute conditions. Cost-effectiveness and regulatory approval are important considerations for the widespread implementation of POC diagnostics.
5.4. Focus on Prevention
While much of the research focuses on detecting existing disease, future work will likely concentrate more on preventive biomarkers that can determine a patient’s risk of developing a condition in the future. This will include looking at the influence of lifestyle factors, environmental factors and genetic markers to ascertain a patient’s risk profile.
Many thanks to our sponsor Esdebe who helped us prepare this research report.
6. Conclusion
Biomarkers are at the forefront of personalized medicine, offering the potential to transform healthcare by improving disease diagnosis, prognosis, treatment selection, and monitoring. The field is rapidly evolving, driven by technological advancements and a growing understanding of disease mechanisms. While significant progress has been made, challenges remain in translating biomarker research into clinical practice. Rigorous validation, standardization, and the integration of multi-omics data are essential for ensuring the reliability and clinical utility of biomarkers. The future of biomarker research is bright, with promising new technologies and approaches on the horizon that hold the potential to revolutionize disease management and usher in an era of precision medicine.
Many thanks to our sponsor Esdebe who helped us prepare this research report.
References
- Atkinson, A. J., Colburn, W. A., DeGruttola, V. G., DeMets, D. L., Downing, G. J., Hoth, D. F., … & Zeger, S. L. (2001). Biomarkers and surrogate endpoints: preferred definitions and conceptual framework. Clinical Pharmacology & Therapeutics, 69(3), 89-95.
- Califf, R. M. (2018). Biomarker definitions and their applications. Clinical Pharmacology & Therapeutics, 103(6), 939-941.
- Duffy, M. J. (2006). Clinical uses of tumor markers: a critical review. Critical Reviews in Clinical Laboratory Sciences, 43(3), 225-262.
- Frank, R., & Hargreaves, R. (2003). Clinical biomarkers in drug discovery and development. Nature Reviews Drug Discovery, 2(7), 566-580.
- Goodsaid, F. M., Mendrick, D. L., & Mirkin, S. (2009). Advancing translational medicine through biomarker qualification. Nature Biotechnology, 27(10), 895-901.
- Mayeux, R. (2004). Biomarkers: potential uses and limitations. NeuroRx, 1(2), 182-188.
- Mishra, A. (2011). Circulating tumor cells: an overview. Journal of Cellular and Molecular Medicine, 15(4), 749-759.
- Nicholson, J. K., Holmes, E., Lindon, J. C., & Wilson, I. D. (2002). Metabolomics: new strategies for metabolic phenotyping in human health and disease. Trends in Biochemical Sciences, 27(8), 441-449.
- Shah, K., & Merchant, K. (2018). Clinical applications of liquid biopsies. Trends in Genetics, 34(12), 897-907.
- Sokoll, L. J., & Chan, D. W. (2009). Biomarkers: from discovery to clinical application. Molecular Diagnosis & Therapy, 13(1), 1-13.
- Speicher, M. R., & Pantel, K. (2014). Tumor heterogeneity: a major challenge in cancer diagnosis and therapy. EMBO Molecular Medicine, 6(4), 475-482.
- Venkatesh, D., & Ellison, Iii, G. L. (2008). Cardiac troponin. American Journal of Medicine, 121(12), 1033-1038.
- Wong, D. T. (2012). Point-of-care diagnostics. Chest, 142(5), 1315-1324.
This is a comprehensive overview of biomarker research! The discussion on multi-omics integration highlights the power of combining different data types for a more holistic understanding of disease. The analytical and computational challenges involved are significant, but the potential benefits for personalized medicine are enormous.
Thanks for your comment! I agree, the promise of personalized medicine through multi-omics is incredible. The analytical challenges are definitely a hurdle, especially in managing the sheer volume of data and ensuring data quality across different platforms. It’s a really exciting area to watch develop!
Editor: MedTechNews.Uk
Thank you to our Sponsor Esdebe
So, we’re pinning all our hopes on AI to sort through this biomarker haystack? How long until algorithms replace actual scientists, or at least, tell them what to do?