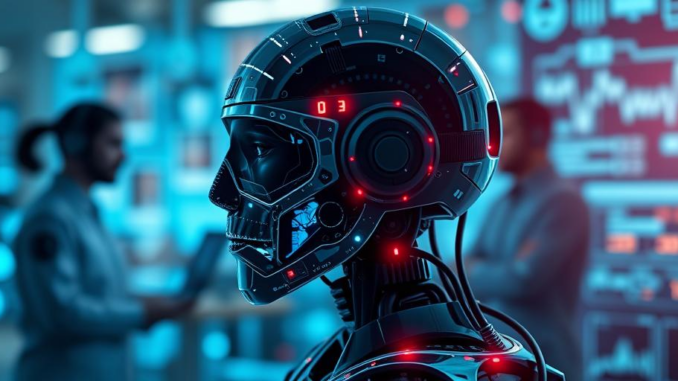
Abstract
Artificial Intelligence (AI) is rapidly reshaping the healthcare landscape, and payers – including insurance companies and government healthcare programs – are at the forefront of this transformation. This research report delves into the multifaceted impact of AI on payer organizations, extending beyond the common narratives of claims processing and fraud detection. We explore the evolving business models that AI is enabling, the strategic investment patterns observed within the payer ecosystem, the intricate web of regulatory and legal challenges associated with AI implementation, and the critical ethical considerations that must be addressed to ensure equitable and responsible AI utilization. Furthermore, this report investigates the shifting dynamics in payer-provider relationships driven by AI-powered tools and data-driven insights, analyzes the potential for both increased efficiency and cost savings, and examines emerging trends in personalized healthcare, predictive analytics, and proactive care management. The report also identifies key challenges and opportunities for payers as they navigate this complex technological shift, providing a comprehensive overview of the current state and future trajectory of AI in healthcare payer operations.
Many thanks to our sponsor Esdebe who helped us prepare this research report.
1. Introduction
The healthcare industry is facing unprecedented challenges, including rising costs, an aging population, increasing prevalence of chronic diseases, and a growing demand for personalized and accessible care. Artificial Intelligence (AI) is emerging as a potentially transformative technology capable of addressing these challenges by automating tasks, improving diagnostic accuracy, personalizing treatment plans, and enhancing operational efficiency. While much of the focus has been on AI’s impact on providers (e.g., hospitals, clinics, and physicians), the role of payers – the organizations that finance and manage healthcare services – is equally critical. Payers are increasingly leveraging AI to optimize their operations, improve patient outcomes, and manage costs. This report provides a comprehensive overview of the evolving role of AI in the payer landscape, exploring the multifaceted dimensions of its impact on business models, investment strategies, regulatory compliance, ethical considerations, payer-provider relationships, and overall efficiency.
The traditional role of payers has been primarily focused on claims processing, utilization management, and risk assessment. However, the advent of AI is empowering payers to move beyond these traditional functions and embrace a more proactive and strategic role in healthcare delivery. AI-powered tools are enabling payers to gain deeper insights into patient populations, identify high-risk individuals, personalize care pathways, and proactively manage chronic conditions. This shift towards value-based care and population health management is fundamentally altering the business models of payers and creating new opportunities for innovation.
This report aims to provide a nuanced understanding of the current state and future trajectory of AI in healthcare payer operations. It is intended for a diverse audience, including healthcare executives, policymakers, researchers, and technology innovators who are interested in the transformative potential of AI in healthcare.
Many thanks to our sponsor Esdebe who helped us prepare this research report.
2. Evolving Business Models of Payers in the Age of AI
The traditional business model of payers, largely centered on fee-for-service reimbursement and reactive claims processing, is undergoing a profound transformation driven by AI. The rise of value-based care (VBC) models, where payers reimburse providers based on patient outcomes and quality of care, has created a significant impetus for payers to adopt AI-powered solutions. This transition towards VBC necessitates a deeper understanding of patient populations, identification of risk factors, and proactive management of chronic conditions – all of which are areas where AI can provide significant value.
2.1. From Claims Processing to Proactive Care Management:
AI is enabling payers to move beyond reactive claims processing and embrace a more proactive approach to care management. AI-powered tools can analyze vast amounts of data from various sources, including electronic health records (EHRs), claims data, wearable devices, and social determinants of health, to identify individuals at high risk of developing chronic conditions or experiencing adverse health events. This allows payers to intervene early and provide targeted interventions, such as personalized coaching, remote monitoring, and medication adherence programs, to prevent costly hospitalizations and improve patient outcomes. For example, AI algorithms can predict the likelihood of hospital readmissions based on a patient’s medical history, demographics, and social factors, allowing payers to proactively address the underlying causes of readmissions and improve care coordination.
2.2. Personalized Healthcare and Tailored Benefit Designs:
AI is also facilitating the development of personalized healthcare solutions tailored to individual patient needs and preferences. Payers can leverage AI to analyze patient data and identify patterns that can inform the design of more effective and engaging benefit programs. For example, AI can be used to personalize health risk assessments, recommend personalized wellness programs, and provide tailored recommendations for preventive care services. This personalized approach can increase patient engagement, improve adherence to treatment plans, and ultimately lead to better health outcomes. Some payers are even exploring the use of AI to dynamically adjust insurance premiums based on individual health behaviors and risk profiles, although this raises significant ethical and privacy concerns.
2.3. New Revenue Streams and Partnership Opportunities:
The adoption of AI is also creating new revenue streams and partnership opportunities for payers. Payers can leverage their data analytics capabilities and AI-powered insights to offer value-added services to providers, such as population health management tools, clinical decision support systems, and predictive analytics dashboards. These services can help providers improve their clinical performance, reduce costs, and better manage their patient populations. Payers can also partner with technology companies to develop and deploy innovative AI-powered solutions for healthcare. For instance, payers can partner with AI startups to develop AI-powered chatbots that provide patients with 24/7 access to healthcare information and support. Some forward-thinking payers are even investing in AI-driven healthcare startups, fostering innovation and gaining early access to cutting-edge technologies.
2.4. Challenges in Business Model Transformation:
While the potential benefits of AI-driven business model transformation are significant, payers also face several challenges. These include the need to invest in new infrastructure and talent, the complexity of integrating AI solutions into existing systems, the challenge of ensuring data privacy and security, and the need to address ethical concerns related to the use of AI in healthcare. Furthermore, the lack of clear regulatory guidelines for AI in healthcare creates uncertainty and can hinder adoption. Payers need to carefully navigate these challenges to successfully transition to AI-driven business models.
Many thanks to our sponsor Esdebe who helped us prepare this research report.
3. Investment Strategies in AI by Healthcare Payers
Healthcare payers are increasingly recognizing the strategic importance of AI and are making significant investments in this technology. These investments span a wide range of areas, including data infrastructure, AI platforms, AI-powered applications, and talent acquisition. Understanding these investment patterns is crucial for assessing the adoption and impact of AI in the payer landscape.
3.1. Data Infrastructure and Data Management:
The foundation for any successful AI initiative is high-quality data. Payers are investing heavily in building robust data infrastructure to collect, store, and manage the vast amounts of data generated from various sources. This includes investments in cloud computing, data lakes, data warehouses, and data governance tools. Payers are also investing in data quality initiatives to ensure that the data used for AI algorithms is accurate, complete, and consistent. Furthermore, investments are being made in interoperability solutions to enable seamless data exchange between payers, providers, and other stakeholders.
3.2. AI Platforms and Machine Learning Tools:
Payers are also investing in AI platforms and machine learning tools that enable them to develop and deploy AI-powered applications. These platforms provide the infrastructure, tools, and services needed to build, train, and deploy machine learning models at scale. Payers are increasingly adopting cloud-based AI platforms that offer a wide range of pre-built machine learning algorithms and tools, as well as the ability to customize algorithms to meet specific needs. Open-source AI frameworks, such as TensorFlow and PyTorch, are also gaining traction among payers.
3.3. AI-Powered Applications and Solutions:
The most visible area of investment is in AI-powered applications and solutions that address specific business challenges. These applications span a wide range of areas, including claims processing, fraud detection, utilization management, risk assessment, care management, and customer service. Payers are investing in both off-the-shelf AI solutions and custom-built applications tailored to their specific needs. Some examples of AI-powered applications include:
- Claims Processing Automation: AI is being used to automate the processing of claims, reducing manual effort and improving accuracy.
- Fraud Detection: AI algorithms can identify suspicious claims patterns and detect fraudulent activity.
- Utilization Management: AI can be used to predict the utilization of healthcare services and identify opportunities to optimize care delivery.
- Risk Assessment: AI can assess patient risk and identify individuals who are likely to require more intensive care.
- Care Management: AI-powered care management platforms can personalize care plans and provide tailored support to patients.
- Customer Service: AI-powered chatbots can provide 24/7 customer support and answer common questions.
3.4. Talent Acquisition and Training:
Implementing AI successfully requires a skilled workforce. Payers are investing in talent acquisition and training programs to build a team of data scientists, machine learning engineers, and AI experts. This includes hiring experienced AI professionals and providing training to existing employees to upskill their knowledge of AI. Payers are also partnering with universities and research institutions to develop AI training programs and attract top talent.
3.5. Challenges in AI Investment:
While the potential benefits of AI investment are significant, payers face several challenges in this area. These include the high cost of AI implementation, the difficulty of measuring the return on investment (ROI) of AI projects, the shortage of skilled AI professionals, and the challenge of integrating AI into existing systems. Payers need to carefully evaluate the risks and benefits of AI investments and develop a clear strategy for implementing AI successfully.
Many thanks to our sponsor Esdebe who helped us prepare this research report.
4. Regulatory Hurdles and Legal Challenges
The implementation of AI in healthcare payer operations is subject to a complex web of regulatory and legal requirements. Navigating these challenges is crucial for ensuring compliance and mitigating legal risks. Key regulatory and legal considerations include data privacy, security, algorithmic bias, and liability.
4.1. Data Privacy and Security (HIPAA and Beyond):
The Health Insurance Portability and Accountability Act (HIPAA) establishes strict rules for the protection of protected health information (PHI). Payers must ensure that their AI systems comply with HIPAA regulations, including those related to data security, access control, and data use limitations. This includes implementing appropriate safeguards to protect PHI from unauthorized access, use, or disclosure. Furthermore, payers must comply with other data privacy regulations, such as the California Consumer Privacy Act (CCPA) and the General Data Protection Regulation (GDPR), which provide individuals with greater control over their personal data. These laws often mandate transparency in data processing practices and require organizations to obtain consent before using personal data for AI applications. The use of federated learning can provide privacy benefits by training AI models on decentralized data sources without directly accessing or sharing sensitive patient information.
4.2. Algorithmic Bias and Fairness:
AI algorithms can perpetuate and amplify existing biases in data, leading to unfair or discriminatory outcomes. For example, AI algorithms used to predict patient risk may be biased against certain demographic groups, resulting in unequal access to care. Payers must carefully evaluate their AI algorithms for bias and take steps to mitigate any biases that are identified. This includes using diverse and representative datasets, developing fairness-aware algorithms, and regularly monitoring AI systems for bias. Algorithmic auditing and explainable AI (XAI) are becoming increasingly important tools for identifying and addressing bias in AI systems.
4.3. Liability and Accountability:
The use of AI in healthcare raises complex questions about liability and accountability. Who is responsible when an AI algorithm makes a mistake that leads to patient harm? Is it the payer, the provider, the AI developer, or the patient? The legal framework for addressing these questions is still evolving. Payers need to develop clear policies and procedures for addressing AI-related errors and ensuring that appropriate accountability measures are in place. Considerations include establishing clear lines of responsibility, implementing robust error detection and correction mechanisms, and providing adequate training to healthcare professionals on the use of AI systems. The concept of ‘human-in-the-loop’ AI, where human oversight is maintained, is often advocated to ensure that critical decisions are not solely based on AI algorithms.
4.4. Regulatory Clarity and Guidance:
The lack of clear regulatory guidance for AI in healthcare creates uncertainty and can hinder adoption. Regulators, such as the Food and Drug Administration (FDA) and the Centers for Medicare & Medicaid Services (CMS), are working to develop regulatory frameworks for AI-based medical devices and healthcare applications. However, more clarity is needed to provide payers with a clear understanding of their legal and regulatory obligations. Payers should actively engage with regulators and policymakers to help shape the future of AI regulation in healthcare.
Many thanks to our sponsor Esdebe who helped us prepare this research report.
5. Ethical Considerations in AI for Care Delivery and Claims Processing
The use of AI in healthcare raises significant ethical considerations that must be addressed to ensure equitable and responsible AI utilization. These considerations include transparency, fairness, privacy, and patient autonomy.
5.1. Transparency and Explainability:
AI algorithms are often complex and opaque, making it difficult to understand how they arrive at their decisions. This lack of transparency can erode trust in AI systems and make it difficult to identify and address biases. Payers should strive to use AI algorithms that are transparent and explainable. This includes providing clear explanations of how AI algorithms work and how they are used to make decisions. Explainable AI (XAI) techniques are becoming increasingly important for understanding the reasoning behind AI decisions and ensuring that they are fair and justified. It is vital that both clinicians and patients can understand the rationale behind AI-driven recommendations to foster trust and ensure appropriate use.
5.2. Fairness and Equity:
AI algorithms can perpetuate and amplify existing biases in data, leading to unfair or discriminatory outcomes. Payers must ensure that their AI algorithms are fair and equitable and do not discriminate against certain demographic groups. This includes using diverse and representative datasets, developing fairness-aware algorithms, and regularly monitoring AI systems for bias. It is also crucial to consider the social determinants of health when designing and deploying AI systems to ensure that they do not exacerbate existing health disparities. Fairness metrics and bias detection techniques should be integrated into the AI development lifecycle.
5.3. Privacy and Data Security:
The use of AI in healthcare requires access to large amounts of sensitive patient data. Payers must ensure that patient data is protected from unauthorized access, use, or disclosure. This includes implementing robust data security measures and complying with data privacy regulations, such as HIPAA and GDPR. It is also important to obtain informed consent from patients before using their data for AI applications. Differential privacy techniques can be employed to protect individual privacy while still allowing AI models to learn from aggregate data. Furthermore, anonymization and pseudonymization techniques can be used to reduce the risk of re-identification.
5.4. Patient Autonomy and Informed Consent:
Patients should have the right to make informed decisions about their healthcare, including the use of AI-powered tools. Payers should provide patients with clear and understandable information about how AI is being used to make decisions about their care. Patients should also have the right to opt out of the use of AI and to request a human review of AI-generated decisions. It is crucial to ensure that AI is used to augment, not replace, human judgment and that patients retain control over their healthcare decisions. Ethical frameworks should guide the development and deployment of AI systems to ensure that patient autonomy is respected.
Many thanks to our sponsor Esdebe who helped us prepare this research report.
6. Impact of AI on Payer-Provider Relationships
AI is transforming the relationship between payers and providers, creating new opportunities for collaboration and data sharing. This can lead to improved care coordination, reduced administrative costs, and better patient outcomes.
6.1. Data Sharing and Interoperability:
AI relies on access to large amounts of data, and payers and providers are increasingly collaborating to share data and improve interoperability. This can enable payers to gain a more comprehensive view of patient health and improve their ability to identify high-risk individuals and personalize care pathways. Providers can benefit from access to payer data, such as claims data and utilization patterns, which can help them improve their clinical performance and reduce costs. Secure data sharing platforms and standardized data formats are essential for facilitating this collaboration. Blockchain technology can potentially be used to create a secure and transparent platform for data sharing between payers and providers.
6.2. Collaborative Care Management:
AI-powered care management platforms can facilitate collaboration between payers and providers in managing chronic conditions and improving patient outcomes. These platforms can provide a shared view of patient data, enabling payers and providers to coordinate care and track progress. They can also provide tools for communication and collaboration, such as secure messaging and video conferencing. This collaborative approach can lead to more effective care management and better patient engagement.
6.3. Value-Based Care Partnerships:
AI is playing a key role in the shift towards value-based care, which rewards providers for delivering high-quality care at a lower cost. Payers and providers are increasingly entering into value-based care arrangements, such as accountable care organizations (ACOs) and bundled payment models. AI can help both payers and providers track performance, identify areas for improvement, and optimize care delivery. Data analytics and predictive modeling can be used to identify high-risk patients, personalize care pathways, and reduce costs. AI can also be used to monitor patient outcomes and track progress towards quality goals.
6.4. Challenges in Payer-Provider Collaboration:
Despite the potential benefits of AI-driven collaboration, payers and providers face several challenges. These include concerns about data privacy and security, the lack of trust between payers and providers, and the difficulty of aligning incentives. Addressing these challenges requires a commitment to transparency, collaboration, and data governance. Establishing clear data sharing agreements, building trust through open communication, and aligning financial incentives are crucial for successful payer-provider collaboration.
Many thanks to our sponsor Esdebe who helped us prepare this research report.
7. Potential for Increased Efficiency and Cost Savings
AI has the potential to significantly increase efficiency and reduce costs in healthcare payer operations. This can lead to lower premiums for consumers and improved financial sustainability for payers.
7.1. Automation of Administrative Tasks:
AI can automate many of the administrative tasks that currently consume significant resources in payer operations. This includes claims processing, eligibility verification, prior authorization, and customer service. Automating these tasks can reduce manual effort, improve accuracy, and free up staff to focus on more strategic activities. Robotic process automation (RPA) and natural language processing (NLP) are key technologies for automating administrative tasks.
7.2. Fraud Detection and Prevention:
Healthcare fraud is a significant problem, costing payers billions of dollars each year. AI can be used to detect and prevent fraud by analyzing claims data and identifying suspicious patterns. AI algorithms can identify fraudulent claims more quickly and accurately than human reviewers, reducing fraud losses and improving financial performance. Machine learning techniques can be used to identify anomalies and predict fraudulent behavior.
7.3. Improved Utilization Management:
AI can be used to improve utilization management by identifying opportunities to optimize care delivery and reduce unnecessary costs. This includes identifying high-risk patients, personalizing care pathways, and promoting preventive care. AI can also be used to predict the utilization of healthcare services and identify opportunities to negotiate better rates with providers. Predictive analytics can be used to forecast future healthcare utilization and identify potential cost savings opportunities.
7.4. Reduction in Medical Errors:
Medical errors are a significant cause of morbidity and mortality, and they also contribute to healthcare costs. AI can be used to reduce medical errors by providing clinical decision support, flagging potential drug interactions, and improving diagnostic accuracy. AI-powered diagnostic tools can assist clinicians in making more accurate and timely diagnoses, reducing the risk of medical errors. This can lead to better patient outcomes and reduced healthcare costs.
7.5. Challenges in Achieving Efficiency and Cost Savings:
While the potential for efficiency and cost savings is significant, payers face several challenges in achieving these benefits. These include the high cost of AI implementation, the difficulty of measuring the ROI of AI projects, and the need to address ethical concerns. Payers need to carefully evaluate the risks and benefits of AI investments and develop a clear strategy for realizing the potential benefits of AI.
Many thanks to our sponsor Esdebe who helped us prepare this research report.
8. Future Trends and Conclusion
The use of AI in healthcare payer operations is still in its early stages, but it is poised for significant growth in the coming years. Several key trends are shaping the future of AI in the payer landscape.
8.1. Increasing Adoption of AI-Powered Solutions:
The adoption of AI-powered solutions is expected to accelerate as payers become more comfortable with the technology and as more vendors develop innovative AI applications. This will lead to increased efficiency, reduced costs, and improved patient outcomes.
8.2. Focus on Personalized Healthcare:
Payers will increasingly focus on using AI to personalize healthcare and tailor benefit programs to individual patient needs. This will lead to more effective care management and better patient engagement.
8.3. Greater Emphasis on Data Interoperability:
Data interoperability will become increasingly important as payers and providers collaborate to share data and improve care coordination. This will require the adoption of standardized data formats and the development of secure data sharing platforms.
8.4. Growing Regulatory Scrutiny:
The use of AI in healthcare will be subject to increasing regulatory scrutiny as regulators grapple with the ethical and legal implications of this technology. Payers will need to comply with evolving regulations and ensure that their AI systems are fair, transparent, and accountable.
8.5. Emergence of New Business Models:
AI is enabling the emergence of new business models for payers, such as risk-based contracting and value-based care. These models will reward payers for delivering high-quality care at a lower cost.
Conclusion:
Artificial Intelligence is poised to revolutionize the healthcare payer landscape. By embracing AI-powered solutions, payers can transform their business models, improve efficiency, reduce costs, and enhance patient outcomes. However, it is crucial to address the ethical and regulatory challenges associated with AI implementation to ensure that this technology is used responsibly and equitably. By carefully navigating these challenges and embracing innovation, payers can unlock the full potential of AI and shape the future of healthcare.
Many thanks to our sponsor Esdebe who helped us prepare this research report.
References
- Jiang, F., Jiang, Y., Zhi, H., Dong, Y., Li, H., Ma, S., … & Wang, Y. (2017). Artificial intelligence in healthcare: past, present and future. Stroke and vascular neurology, 2(4), 230-243.
- Topol, E. J. (2019). High-performance medicine: the convergence of human and artificial intelligence. Nature medicine, 25(1), 44-56.
- Mesko, B., & Topol, E. J. (2017). Artificial intelligence in healthcare: a roadmap. The BMJ, 357, j2624.
- Holzinger, A., Biemann, C., Pattichis, C. S., & Kell, D. B. (2017). What do we need to build explainable AI for healthcare?. Artificial intelligence in medicine, 84, 1-5.
- Obermeyer, Z., Powers, B., Vogeli, C., & Mullainathan, S. (2019). Dissecting racial bias in an algorithm used to manage the health of populations. Science, 366(6464), 447-453.
- US Department of Health and Human Services. (n.d.). HIPAA. Retrieved from https://www.hhs.gov/hipaa/index.html
- Goodman, B., & Flaxman, S. (2017). European Union regulations on algorithmic decision-making and a “right to explanation”. AI Magazine, 38(3), 50-57.
- Price, W. N., Gerke, S., & Cohen, I. G. (2021). Potential liability for physicians using artificial intelligence. Jama, 326(7), 613-614.
- Rad, P., Daugherty, P., & Carriere, J. (2021). Winning in healthcare’s new AI battlegrounds. Accenture. Retrieved from https://www.accenture.com/us-en/insights/health/winning-healthcare-ai-battlegrounds
- European Parliament. (2016). Regulation (EU) 2016/679 (General Data Protection Regulation). Official Journal of the European Union, L 119(1).
AI predicting hospital readmissions? Finally, a use for algorithms I can understand! My own readmission rate to the couch after a large pizza is shockingly predictable, but I hadn’t considered AI could help manage it. Maybe they can develop an algorithm for that next.
That’s a great point! While we’re focusing on hospital readmissions, the underlying technology could definitely be adapted for more lighthearted applications, like predicting post-pizza couch readmission rates. It highlights the versatility of AI and predictive modeling beyond just healthcare. Imagine the insights we could gain!
Editor: MedTechNews.Uk
Thank you to our Sponsor Esdebe
So, AI is diving headfirst into healthcare payments? Guess I’ll start being extra polite to my insurance company’s chatbot; wouldn’t want to accidentally trigger a premium hike because I complained too much about deductibles. Now, will these algorithms ever tackle the mystery of why medical bills are so cryptic?
That’s a hilarious and insightful point! AI’s potential impact on explaining those cryptic medical bills is huge. Imagine algorithms that translate the jargon and break down costs into understandable components. That could really empower patients and foster transparency in the billing process. #AIinHealthcare #HealthcareInnovation
Editor: MedTechNews.Uk
Thank you to our Sponsor Esdebe
So AI *might* reduce costs for payers? Will that saving magically appear on my premium, or will it vanish faster than a free donut in a staff meeting? Inquiring minds want to know where the savings actually *go*.