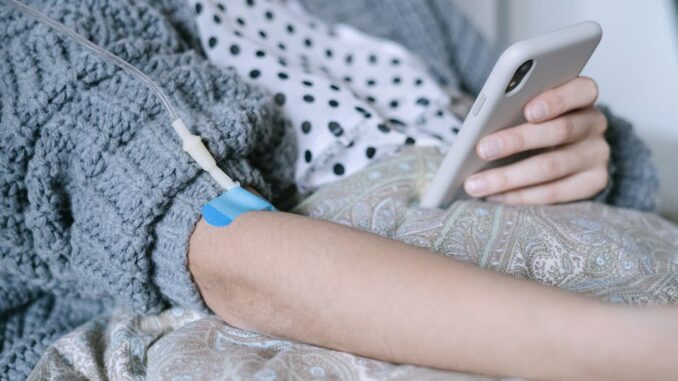
Abstract
Biomarkers have emerged as indispensable tools in modern medicine, playing pivotal roles in disease diagnosis, prognosis, prediction of treatment response, and monitoring of disease progression. This research report provides a comprehensive overview of the diverse landscape of biomarkers, encompassing their various types, applications across different disease areas, and the challenges associated with their development and validation. We delve into the latest advancements in biomarker research, including the discovery of novel biomarkers through high-throughput technologies, the application of multi-omics approaches for biomarker identification, and the integration of artificial intelligence (AI) for enhanced biomarker analysis and clinical decision-making. We examine the specific role of biomarkers in oncology, cardiovascular disease, neurological disorders, and infectious diseases, highlighting their clinical utility and limitations. The report also addresses the critical aspects of biomarker validation, standardization, and regulatory considerations, emphasizing the need for rigorous methodologies to ensure their reliability and reproducibility. Finally, we explore the future directions of biomarker research, including the development of personalized biomarker panels, the application of liquid biopsies for non-invasive disease monitoring, and the integration of biomarkers with other clinical data to improve patient outcomes. This review aims to provide a comprehensive understanding of the current state of biomarker research and its potential to revolutionize healthcare.
Many thanks to our sponsor Esdebe who helped us prepare this research report.
1. Introduction
The term “biomarker” encompasses a broad range of measurable indicators that can provide valuable insights into physiological and pathological processes within an organism. According to the National Institutes of Health (NIH), a biomarker is defined as “a characteristic that is objectively measured and evaluated as an indicator of normal biological processes, pathogenic processes, or pharmacologic responses to a therapeutic intervention” [1]. Biomarkers can be molecules (e.g., proteins, nucleic acids, metabolites), cells, or even imaging characteristics, and they can be measured in various biological samples, including blood, urine, tissue, and cerebrospinal fluid. The allure of biomarkers stems from their potential to provide objective and quantitative information about an individual’s health status, enabling early disease detection, accurate diagnosis, personalized treatment strategies, and effective monitoring of therapeutic interventions. The development and validation of biomarkers is a complex and multidisciplinary process, requiring expertise in fields such as molecular biology, biochemistry, immunology, clinical chemistry, and bioinformatics. Furthermore, the integration of biomarkers into clinical practice requires careful consideration of regulatory guidelines, ethical considerations, and cost-effectiveness. This research report aims to provide a comprehensive overview of the current state of biomarker research, highlighting their diverse applications, challenges, and future directions. It will explore the different types of biomarkers, their role in various disease areas, the latest advancements in biomarker discovery and validation, and the integration of artificial intelligence for improved biomarker analysis and clinical decision-making.
Many thanks to our sponsor Esdebe who helped us prepare this research report.
2. Types of Biomarkers
Biomarkers can be classified based on various criteria, including their origin, function, and the type of biological information they provide.
2.1. Molecular Biomarkers
Molecular biomarkers represent the largest and most diverse category of biomarkers. They encompass a wide range of molecules, including proteins, nucleic acids (DNA, RNA, microRNA), lipids, and metabolites.
-
Proteomic Biomarkers: Proteins are arguably the most widely studied type of molecular biomarker. They play crucial roles in virtually all biological processes, and their expression levels, modifications, and interactions can provide valuable information about disease status. Proteomic biomarkers can be detected using a variety of techniques, including enzyme-linked immunosorbent assays (ELISAs), mass spectrometry, and protein arrays [2]. Examples of clinically relevant proteomic biomarkers include prostate-specific antigen (PSA) for prostate cancer screening, troponin for myocardial infarction diagnosis, and CA-125 for ovarian cancer monitoring. However, it is important to note that many proteomic biomarkers lack sufficient specificity and sensitivity for reliable clinical use, highlighting the need for improved biomarker discovery and validation strategies.
-
Genomic Biomarkers: DNA-based biomarkers are increasingly used in disease diagnosis and prognosis, particularly in the field of oncology. Genomic biomarkers can provide information about genetic mutations, chromosomal abnormalities, and gene expression patterns that are associated with disease risk, progression, and treatment response. Examples of genomic biomarkers include BRCA1/2 mutations for breast cancer risk assessment, EGFR mutations for targeted therapy selection in lung cancer, and KRAS mutations for predicting resistance to EGFR inhibitors in colorectal cancer [3]. Next-generation sequencing (NGS) technologies have revolutionized genomic biomarker research, enabling the rapid and cost-effective analysis of entire genomes or specific gene panels.
-
Transcriptomic Biomarkers: RNA-based biomarkers, including messenger RNA (mRNA) and microRNA (miRNA), reflect the dynamic changes in gene expression that occur in response to disease or therapeutic interventions. mRNA biomarkers can be used to identify differentially expressed genes that are associated with disease pathogenesis, while miRNA biomarkers can act as regulators of gene expression and play important roles in various biological processes. Techniques such as quantitative reverse transcription PCR (qRT-PCR) and RNA sequencing are commonly used to detect and quantify transcriptomic biomarkers [4].
-
Metabolomic Biomarkers: Metabolites are small molecules that are involved in metabolic pathways, and their levels can be influenced by various factors, including genetics, diet, and environmental exposures. Metabolomic biomarkers can provide a snapshot of an individual’s metabolic state and can be used to identify metabolic signatures that are associated with disease risk, progression, and treatment response. Techniques such as gas chromatography-mass spectrometry (GC-MS) and liquid chromatography-mass spectrometry (LC-MS) are used to analyze metabolomic biomarkers [5].
2.2. Cellular Biomarkers
Cellular biomarkers are cells or cellular components that can be used to assess disease status or predict treatment response. Examples of cellular biomarkers include circulating tumor cells (CTCs) for cancer monitoring, immune cells for assessing immune function, and stem cells for regenerative medicine applications.
-
Circulating Tumor Cells (CTCs): CTCs are cancer cells that have detached from the primary tumor and entered the bloodstream. CTCs can be used to monitor disease progression, predict treatment response, and identify potential therapeutic targets in patients with metastatic cancer. Techniques such as CellSearch and Parsortix are used to isolate and enumerate CTCs from blood samples [6].
-
Immune Cells: Immune cells, such as T cells, B cells, and natural killer (NK) cells, play critical roles in the immune response to disease. The number, type, and activation state of immune cells can be used to assess immune function and predict treatment response in patients with cancer, autoimmune diseases, and infectious diseases. Flow cytometry and immunohistochemistry are commonly used to analyze immune cell biomarkers [7].
2.3. Imaging Biomarkers
Imaging biomarkers are anatomical, physiological, or molecular features that can be detected using medical imaging techniques, such as magnetic resonance imaging (MRI), computed tomography (CT), positron emission tomography (PET), and ultrasound. Imaging biomarkers can provide non-invasive assessment of disease status and treatment response [8].
-
Anatomical Imaging Biomarkers: Anatomical imaging biomarkers, such as tumor size, shape, and location, can be used to assess disease progression and treatment response in patients with cancer. MRI and CT are commonly used to detect anatomical imaging biomarkers.
-
Physiological Imaging Biomarkers: Physiological imaging biomarkers, such as blood flow, metabolism, and oxygenation, can provide information about the functional status of tissues and organs. PET and functional MRI are commonly used to detect physiological imaging biomarkers.
-
Molecular Imaging Biomarkers: Molecular imaging biomarkers are molecules that are labeled with radioactive isotopes or other detectable tags and used to visualize specific molecular targets in vivo. PET and single-photon emission computed tomography (SPECT) are commonly used to detect molecular imaging biomarkers.
Many thanks to our sponsor Esdebe who helped us prepare this research report.
3. Applications of Biomarkers in Different Disease Areas
Biomarkers have a wide range of applications in different disease areas, including oncology, cardiovascular disease, neurological disorders, and infectious diseases.
3.1. Oncology
Biomarkers play a crucial role in all aspects of cancer management, from screening and diagnosis to prognosis and treatment selection.
-
Cancer Screening: Biomarkers such as PSA for prostate cancer, CA-125 for ovarian cancer, and AFP for hepatocellular carcinoma are used for cancer screening. However, the use of these biomarkers for screening is often controversial due to their limited sensitivity and specificity, leading to false positives and false negatives [9].
-
Cancer Diagnosis: Biomarkers can be used to confirm a cancer diagnosis and to differentiate between different types of cancer. For example, immunohistochemistry markers are used to classify breast cancer subtypes based on the expression of estrogen receptor (ER), progesterone receptor (PR), and HER2.
-
Cancer Prognosis: Biomarkers can be used to predict the likelihood of disease recurrence and survival in patients with cancer. For example, gene expression signatures such as Oncotype DX and MammaPrint are used to predict the risk of recurrence in patients with early-stage breast cancer [10].
-
Prediction of Treatment Response: Biomarkers can be used to predict which patients are most likely to benefit from a particular treatment. For example, EGFR mutations are used to select patients with lung cancer who are likely to respond to EGFR inhibitors, and KRAS mutations are used to predict resistance to EGFR inhibitors in patients with colorectal cancer.
-
Monitoring of Treatment Response: Biomarkers can be used to monitor the response of cancer to treatment. For example, CEA and CA 19-9 levels are used to monitor the response of colorectal cancer to chemotherapy, and circulating tumor DNA (ctDNA) is used to monitor the response of various cancers to targeted therapy and immunotherapy.
3.2. Cardiovascular Disease
Biomarkers are used to assess cardiovascular risk, diagnose acute cardiac events, and monitor the response to cardiovascular therapies.
-
Risk Assessment: Biomarkers such as cholesterol, triglycerides, and high-sensitivity C-reactive protein (hs-CRP) are used to assess cardiovascular risk. Novel biomarkers such as lipoprotein(a) and trimethylamine N-oxide (TMAO) are also being investigated for their potential to improve risk stratification [11].
-
Diagnosis of Acute Cardiac Events: Biomarkers such as troponin, creatine kinase-MB (CK-MB), and myoglobin are used to diagnose acute myocardial infarction (AMI). Troponin is the preferred biomarker for AMI diagnosis due to its high sensitivity and specificity [12].
-
Monitoring of Heart Failure: Biomarkers such as B-type natriuretic peptide (BNP) and N-terminal pro-B-type natriuretic peptide (NT-proBNP) are used to monitor the severity of heart failure and to guide treatment decisions.
3.3. Neurological Disorders
Biomarkers are increasingly used in the diagnosis, prognosis, and monitoring of neurological disorders, such as Alzheimer’s disease, Parkinson’s disease, and multiple sclerosis.
-
Alzheimer’s Disease: Biomarkers such as amyloid-beta (Aβ) and tau proteins in cerebrospinal fluid (CSF) and amyloid PET imaging are used to diagnose Alzheimer’s disease and to predict the risk of developing Alzheimer’s disease in individuals with mild cognitive impairment (MCI) [13].
-
Parkinson’s Disease: Biomarkers such as α-synuclein in CSF and dopamine transporter (DaT) SPECT imaging are being investigated for their potential to diagnose Parkinson’s disease and to monitor disease progression.
-
Multiple Sclerosis: Biomarkers such as oligoclonal bands in CSF and MRI lesions are used to diagnose multiple sclerosis and to monitor disease activity.
3.4. Infectious Diseases
Biomarkers are used to diagnose infectious diseases, to monitor disease progression, and to predict treatment response.
-
Viral Infections: Biomarkers such as viral load and antibody titers are used to diagnose and monitor viral infections such as HIV, hepatitis B, and hepatitis C.
-
Bacterial Infections: Biomarkers such as procalcitonin (PCT) and C-reactive protein (CRP) are used to diagnose bacterial infections and to guide antibiotic therapy.
-
Sepsis: Biomarkers such as PCT, CRP, and interleukin-6 (IL-6) are used to diagnose sepsis and to predict mortality risk.
Many thanks to our sponsor Esdebe who helped us prepare this research report.
4. Latest Advancements in Biomarker Research
Biomarker research is a rapidly evolving field, with numerous advancements being made in biomarker discovery, validation, and application.
4.1. High-Throughput Technologies for Biomarker Discovery
High-throughput technologies, such as genomics, proteomics, and metabolomics, are being used to identify novel biomarkers for various diseases. These technologies allow for the simultaneous analysis of thousands of molecules, providing a comprehensive overview of the molecular changes that occur in disease [14].
4.2. Multi-Omics Approaches for Biomarker Identification
Multi-omics approaches, which integrate data from multiple omics platforms, such as genomics, proteomics, and metabolomics, are being used to identify complex biomarker signatures that are more informative than single biomarkers. These approaches can provide a more holistic understanding of disease pathogenesis and can lead to the discovery of novel therapeutic targets [15].
4.3. Liquid Biopsies for Non-Invasive Disease Monitoring
Liquid biopsies, which involve the analysis of biological fluids such as blood, urine, and saliva, are being used for non-invasive disease monitoring. Liquid biopsies can provide valuable information about disease progression, treatment response, and the emergence of drug resistance [16].
4.4. Role of Artificial Intelligence (AI) in Biomarker Research
Artificial intelligence (AI) is being used to analyze large and complex biomarker datasets, to identify novel biomarker patterns, and to predict disease outcomes. AI algorithms can be trained to recognize subtle patterns in biomarker data that are not readily apparent to humans [17].
Many thanks to our sponsor Esdebe who helped us prepare this research report.
5. The Role of Artificial Intelligence (AI) in Biomarker Analysis and Clinical Decision-Making
AI is revolutionizing biomarker research and clinical decision-making by enabling the analysis of large and complex datasets, the identification of novel biomarker patterns, and the prediction of disease outcomes.
5.1. AI-Powered Biomarker Discovery
AI algorithms can be used to analyze high-throughput omics data and to identify novel biomarkers that are associated with disease. Machine learning techniques, such as support vector machines (SVMs) and random forests, can be trained to classify patients based on their biomarker profiles and to predict disease risk, progression, and treatment response [18].
5.2. AI-Enhanced Biomarker Analysis
AI algorithms can be used to improve the accuracy and reliability of biomarker measurements. For example, AI can be used to correct for batch effects in omics data and to remove noise from imaging data. Deep learning algorithms can be used to automate the analysis of medical images and to extract quantitative features that are associated with disease [19].
5.3. AI-Driven Clinical Decision-Making
AI algorithms can be used to integrate biomarker data with other clinical information, such as patient demographics, medical history, and imaging results, to make personalized treatment recommendations. AI-powered decision support systems can help clinicians to select the most appropriate treatment for each patient based on their individual risk factors and biomarker profiles [20].
Many thanks to our sponsor Esdebe who helped us prepare this research report.
6. Challenges in Validating and Standardizing Biomarkers for Clinical Use
The validation and standardization of biomarkers for clinical use is a complex and challenging process. Several factors can contribute to the variability of biomarker measurements, including pre-analytical factors (e.g., sample collection, processing, and storage), analytical factors (e.g., assay performance, reagent quality, and instrument calibration), and post-analytical factors (e.g., data analysis and interpretation).
6.1. Pre-Analytical Variability
Pre-analytical variability can significantly affect the accuracy and reliability of biomarker measurements. It is crucial to standardize sample collection, processing, and storage procedures to minimize pre-analytical variability. The use of standardized operating procedures (SOPs) and quality control measures can help to ensure the consistency of biomarker measurements across different laboratories and studies [21].
6.2. Analytical Variability
Analytical variability can arise from various sources, including assay performance, reagent quality, and instrument calibration. It is essential to use validated assays with known performance characteristics and to ensure that reagents are of high quality and properly stored. Regular instrument calibration and quality control measures can help to minimize analytical variability [22].
6.3. Lack of Standardization
The lack of standardization of biomarker assays and data analysis methods can make it difficult to compare results across different laboratories and studies. Standardization efforts are needed to develop consensus guidelines for biomarker measurement and data analysis [23].
6.4. Regulatory Considerations
The use of biomarkers in clinical practice is subject to regulatory oversight by agencies such as the FDA and the EMA. Biomarkers used for diagnostic or prognostic purposes must be validated and approved by these agencies before they can be used in clinical practice [24].
Many thanks to our sponsor Esdebe who helped us prepare this research report.
7. Future Directions
The field of biomarker research is poised for significant advancements in the coming years. Some of the key future directions include:
-
Development of Personalized Biomarker Panels: The development of personalized biomarker panels that are tailored to an individual’s specific disease and risk factors will improve diagnostic accuracy and personalize treatment strategies.
-
Application of Liquid Biopsies for Non-Invasive Disease Monitoring: Liquid biopsies will become increasingly important for non-invasive disease monitoring, allowing for earlier detection of disease recurrence and the monitoring of treatment response in real-time.
-
Integration of Biomarkers with Other Clinical Data: The integration of biomarkers with other clinical data, such as imaging results, patient demographics, and medical history, will improve clinical decision-making and lead to better patient outcomes.
-
Increased Use of AI and Machine Learning: AI and machine learning will play an increasingly important role in biomarker discovery, validation, and application, enabling the analysis of large and complex datasets and the identification of novel biomarker patterns.
Many thanks to our sponsor Esdebe who helped us prepare this research report.
8. Conclusion
Biomarkers are essential tools in modern medicine, playing critical roles in disease diagnosis, prognosis, and treatment selection. The development and validation of biomarkers is a complex and multidisciplinary process, requiring expertise in various fields. Recent advancements in high-throughput technologies, multi-omics approaches, and liquid biopsies have led to the discovery of novel biomarkers for various diseases. The integration of artificial intelligence (AI) is further revolutionizing biomarker research by enabling the analysis of large and complex datasets and the identification of novel biomarker patterns. Despite the significant progress made in biomarker research, several challenges remain, including the need for improved validation and standardization of biomarkers for clinical use. Addressing these challenges will require collaborative efforts from researchers, clinicians, and regulatory agencies. By continuing to invest in biomarker research and development, we can unlock the full potential of biomarkers to improve patient outcomes and transform healthcare.
Many thanks to our sponsor Esdebe who helped us prepare this research report.
References
[1] Biomarkers Definitions Working Group. Biomarkers and surrogate endpoints: preferred definitions and conceptual framework. Clin Pharmacol Ther. 2001;69(3):89-95.
[2] Anderson L, Seibert C. Quantitative mass spectrometry as a tool for biomarker discovery. J Proteome Res. 2008;7(9):3361-77.
[3] Dienstmann R, Tabernero J, Siena S, et al. Equivalent efficacy of mFOLFOX6 plus cetuximab beyond disease progression in patients with KRAS wild-type metastatic colorectal cancer: a phase III randomized trial (FIRE-3). Ann Oncol. 2015;26(12):2477-84.
[4] Livak KJ, Schmittgen TD. Analysis of relative gene expression data using real-time quantitative PCR and the 2(-Delta Delta C(T)) method. Methods. 2001;25(4):402-8.
[5] Nicholson JK, Lindon JC, Holmes E. ‘Metabonomics’: understanding the metabolic responses of living systems to pathophysiological stimuli via multivariate statistical analysis of biological NMR spectroscopic data. Xenobiotica. 1999;29(11):1181-9.
[6] Cristofanilli M, Budd GT, Ellis MJ, et al. Circulating tumor cells, disease progression, and survival in metastatic breast cancer. N Engl J Med. 2004;351(8):781-91.
[7] Maecker HT, McCoy JP Jr, Nussenblatt R. Standardizing immunophenotyping for clinical trials. Nat Rev Immunol. 2012;12(3):191-200.
[8] Lambin P, Rios-Velazquez E, Leijenaar RT, et al. Radiomics: extracting more information from medical images using advanced feature analysis. Eur J Cancer. 2012;48(4):441-6.
[9] Etzioni R, Gulati R, Tsodikov A, et al. Overdiagnosis due to prostate-specific antigen screening: lessons from U.S. trends. J Natl Cancer Inst. 2002;94(13):981-90.
[10] Paik S, Shak S, Tang G, et al. A multigene assay to predict recurrence of tamoxifen-treated, node-negative breast cancer. N Engl J Med. 2004;351(27):2817-26.
[11] Tsimikas S, Karwatowska-Prokopczuk E, Viney NJ, et al. Lipoprotein(a) oxidation, antioxidants, and established cardiovascular risk factors: assessment with an isoform-independent assay. J Am Coll Cardiol. 2003;41(8):1467-75.
[12] Thygesen K, Alpert JS, Jaffe AS, et al. Third universal definition of myocardial infarction. Circulation. 2012;126(16):2020-35.
[13] Jack CR Jr, Knopman DS, Jagust WJ, et al. Tracking pathophysiological processes in Alzheimer’s disease: an updated hypothetical model of dynamic biomarkers. Lancet Neurol. 2013;12(2):207-16.
[14] Hood L, Perlmutter RM. The impact of systems approaches on biological problems. Science. 2004;306(5695):640-3.
[15] Subramanian I, Verma S, Kumar S, et al. Multi-omics data integration, interpretation, and its application. Bioinform Biol Insights. 2020;14:1177932220906614.
[16] Pantel K, Alix-Panabières C. Circulating tumour cells in cancer patients: challenges and perspectives. Trends Mol Med. 2010;16(9):398-406.
[17] Esteva A, Kuprel B, Novoa RA, et al. Dermatologist-level classification of skin cancer with deep neural networks. Nature. 2017;542(7639):115-8.
[18] Hastie T, Tibshirani R, Friedman J. The Elements of Statistical Learning. 2nd ed. New York, NY: Springer; 2009.
[19] LeCun Y, Bengio Y, Hinton G. Deep learning. Nature. 2015;521(7553):436-44.
[20] Obermeyer Z, Emanuel EJ. Predicting the Future – Big Data, Machine Learning, and Clinical Medicine. N Engl J Med. 2016;375(13):1216-9.
[21] Betsou F, Giepmans L, Stolker D, et al. Preanalytical quality control in clinical proteomics. Proteomics. 2012;12(13):2097-117.
[22] Wood AJ, Weed DL. On the scientific basis of standards and regulations for biomarkers. J Natl Cancer Inst. 2006;98(10):651-8.
[23] Vesper HW, Miller WG, Myers GL. Reference materials and laboratory standardization for clinical enzymology. Clin Chim Acta. 2008;390(1-2):33-9.
[24] Food and Drug Administration. Guidance for Industry: Pharmacogenomic Data Submission. Rockville, MD: Food and Drug Administration; 2005.
This report effectively highlights the potential of liquid biopsies for non-invasive disease monitoring. How might these technologies be further refined to improve sensitivity and specificity, particularly for early-stage disease detection where biomarker concentrations may be very low?
Thanks for your insightful question! Refining enrichment strategies is key. Exploring methods like microfluidic devices and nanomaterials to capture rare biomarkers could significantly boost sensitivity. Combining these with advanced signal amplification techniques may also help detect those elusive early-stage indicators. What novel approaches do you find most promising?
Editor: MedTechNews.Uk
Thank you to our Sponsor Esdebe
So, if AI is sifting through biomarker data, does that mean my Fitbit is about to become my new primary care physician? Asking for a friend who *might* be avoiding their annual check-up…