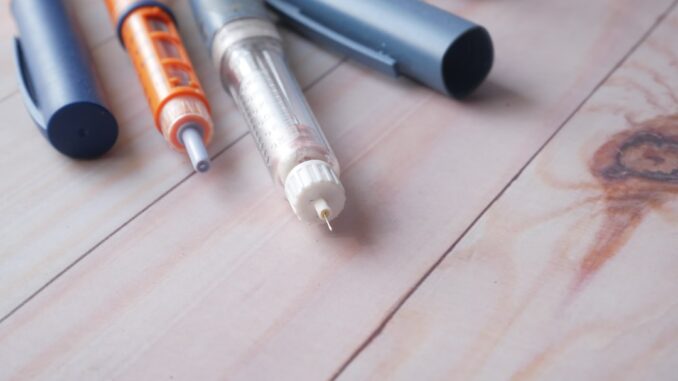
Abstract
Diabetes mellitus, a global health crisis, encompasses a spectrum of metabolic disorders characterized by hyperglycemia. While historically classified primarily as Type 1 and Type 2 diabetes, mounting evidence reveals a profound heterogeneity within these broad categories. This review delves into the emerging landscape of diabetes subtyping, exploring the underlying biological mechanisms that distinguish these subtypes. We examine the current understanding of insulin resistance, beta-cell dysfunction, incretin effects, and other pathophysiological factors contributing to subtype-specific disease progression. Furthermore, we discuss the potential of personalized therapeutic strategies tailored to individual subtypes, highlighting both promising advancements and existing challenges in translating this knowledge into clinical practice. This report aims to provide a comprehensive overview of the complex landscape of diabetes subtypes, fostering a deeper understanding of the disease and paving the way for more effective and targeted interventions.
Many thanks to our sponsor Esdebe who helped us prepare this research report.
1. Introduction
Diabetes mellitus (DM) represents a significant public health challenge worldwide, with a rapidly increasing prevalence and associated morbidity and mortality [1]. Traditionally, DM has been classified into Type 1 diabetes (T1D), an autoimmune-mediated destruction of pancreatic beta-cells leading to absolute insulin deficiency, and Type 2 diabetes (T2D), characterized by insulin resistance and progressive beta-cell dysfunction [2]. Gestational diabetes (GDM) and other specific types of diabetes are also recognized, but the primary focus has remained on distinguishing between T1D and T2D. However, this binary classification fails to capture the substantial clinical and pathophysiological heterogeneity observed within each type, particularly T2D [3].
The recognition of this heterogeneity has spurred intense research efforts to identify distinct diabetes subtypes, driven by advancements in omics technologies (genomics, transcriptomics, proteomics, metabolomics) and sophisticated analytical methods, including machine learning [4]. These approaches have revealed that T2D, in particular, is not a single disease entity but rather a collection of distinct disorders with varying degrees of insulin resistance, beta-cell dysfunction, incretin deficiency, and other contributing factors. The identification of these subtypes holds the promise of personalized medicine, allowing for the development of targeted therapies that address the specific underlying mechanisms driving disease progression in each subtype [5].
This review provides a comprehensive overview of the current understanding of diabetes subtypes, focusing on the biological characteristics, diagnostic challenges, therapeutic implications, and future directions in this rapidly evolving field. We will explore the different subtyping approaches, discuss the identified subtypes and their underlying mechanisms, examine the potential of personalized therapeutic strategies, and highlight the challenges and opportunities in translating subtype-specific treatments into clinical practice.
Many thanks to our sponsor Esdebe who helped us prepare this research report.
2. Methods for Diabetes Subtyping
Several methodologies have been employed to identify and characterize diabetes subtypes, each with its strengths and limitations. These approaches can be broadly categorized into:
- Clinical Phenotyping: This involves clustering individuals based on readily available clinical characteristics, such as age at diagnosis, body mass index (BMI), HbA1c levels, insulin secretion, insulin resistance, and the presence of diabetes-related complications [6]. While relatively simple and cost-effective, clinical phenotyping may be limited by the subjectivity in data collection and the potential for overlapping phenotypes.
- Omics-Based Subtyping: This approach leverages high-throughput omics technologies to identify molecular signatures associated with different diabetes subtypes. Genomics studies aim to identify genetic variants that predispose individuals to specific subtypes [7]. Transcriptomics analysis examines gene expression patterns in relevant tissues (e.g., pancreatic islets, adipose tissue, muscle) to identify subtype-specific molecular pathways [8]. Proteomics and metabolomics approaches analyze protein and metabolite profiles, respectively, providing further insights into the biochemical processes underlying subtype-specific disease mechanisms [9, 10].
- Machine Learning: Machine learning (ML) algorithms, particularly unsupervised learning methods such as cluster analysis, have emerged as powerful tools for identifying diabetes subtypes. ML algorithms can analyze large datasets of clinical and omics data to identify distinct clusters of individuals with similar characteristics [11]. These algorithms can also be used to predict subtype membership based on individual patient characteristics, facilitating personalized treatment decisions.
- Integrated Approaches: The most promising approach involves integrating clinical and omics data to provide a more comprehensive understanding of diabetes subtypes. By combining clinical phenotypes with molecular signatures, researchers can identify subtypes that are both clinically relevant and biologically distinct. This integrated approach allows for a more nuanced understanding of the disease and facilitates the development of targeted therapeutic strategies.
Each of these methods has limitations. Clinical phenotyping is limited by available data and the potential for subjectivity. Omics-based subtyping can be expensive and complex, requiring sophisticated data analysis techniques. Machine learning algorithms can be sensitive to the quality and completeness of the input data. Therefore, the most robust approach involves integrating multiple methodologies to validate subtype identification and gain a more comprehensive understanding of the underlying mechanisms.
Many thanks to our sponsor Esdebe who helped us prepare this research report.
3. Emerging Diabetes Subtypes
Several studies have identified potential diabetes subtypes using different methodologies. While the specific number and characteristics of subtypes vary across studies, some common themes have emerged. A landmark study from the All New Diabetics in Scania (ANDIS) consortium identified five replicable clusters of T2D based on six variables: age at diagnosis, BMI, HbA1c, glutamic acid decarboxylase antibodies (GADA), beta-cell function and insulin resistance [12]. These clusters were:
- Severe Autoimmune Diabetes (SAID): This subtype is characterized by the presence of GADA antibodies and a clinical presentation similar to T1D, suggesting a slowly progressing form of autoimmune diabetes in adults. Often misdiagnosed initially as T2D.
- Severe Insulin-Deficient Diabetes (SIDD): Individuals in this subtype exhibit impaired insulin secretion and poor glycemic control, often requiring insulin therapy early in the disease course. Lower BMI compared to other T2D subtypes.
- Severe Insulin-Resistant Diabetes (SIRD): This subtype is characterized by marked insulin resistance, obesity, and a high risk of non-alcoholic fatty liver disease (NAFLD). Respond relatively well to insulin-sensitizing medications.
- Mild Obesity-Related Diabetes (MOD): This subtype is characterized by moderate insulin resistance and obesity but generally good glycemic control. Relatively younger age of onset.
- Mild Age-Related Diabetes (MARD): This subtype is characterized by older age at diagnosis and relatively mild metabolic abnormalities.
These clusters are supported by independent replication studies and have shown differential risk for specific diabetes complications.
Other research has focused on identifying subtypes based on specific molecular pathways. For example, some studies have identified subtypes characterized by increased inflammation, oxidative stress, or endoplasmic reticulum stress [13]. These molecular subtypes may be more amenable to targeted therapies that address the underlying pathological mechanisms.
It’s crucial to note that the identified subtypes represent a spectrum of disease states rather than discrete categories. Individuals may exhibit characteristics of multiple subtypes, and their subtype membership may change over time as the disease progresses. Furthermore, the identified subtypes may vary across different populations due to genetic and environmental factors. Therefore, further research is needed to refine subtype definitions and validate their clinical utility.
Many thanks to our sponsor Esdebe who helped us prepare this research report.
4. Biological Mechanisms Underlying Diabetes Subtypes
The heterogeneity of diabetes subtypes reflects the complex interplay of multiple pathophysiological mechanisms. Understanding these mechanisms is crucial for developing targeted therapies that address the specific underlying causes of disease progression in each subtype. Key factors contributing to diabetes heterogeneity include:
- Insulin Resistance: Insulin resistance, a hallmark of T2D, varies significantly across subtypes. Some subtypes, such as SIRD, are characterized by severe insulin resistance, while others, such as SIDD, exhibit relatively normal insulin sensitivity [14]. The degree of insulin resistance can be influenced by genetic factors, obesity, inflammation, and other environmental factors. At the molecular level, insulin resistance involves impaired insulin signaling in target tissues, such as muscle, liver, and adipose tissue, leading to reduced glucose uptake and utilization.
- Beta-Cell Dysfunction: Beta-cell dysfunction, characterized by impaired insulin secretion, is another key contributor to diabetes heterogeneity. Some subtypes, such as SIDD, exhibit severe beta-cell dysfunction early in the disease course, while others maintain relatively normal insulin secretion for many years [15]. Beta-cell dysfunction can be caused by genetic factors, glucotoxicity, lipotoxicity, inflammation, and other factors. At the cellular level, beta-cell dysfunction involves impaired glucose sensing, reduced insulin biosynthesis, and increased beta-cell apoptosis.
- Incretin Deficiency: Incretins, such as glucagon-like peptide-1 (GLP-1) and glucose-dependent insulinotropic polypeptide (GIP), are gut hormones that stimulate insulin secretion and suppress glucagon secretion in response to glucose ingestion. Incretin deficiency or resistance can contribute to diabetes heterogeneity by impairing glucose-stimulated insulin secretion and increasing postprandial hyperglycemia [16]. Some studies have shown that individuals with certain subtypes of diabetes exhibit reduced incretin secretion or impaired incretin sensitivity.
- Inflammation: Chronic low-grade inflammation plays a critical role in the pathogenesis of both insulin resistance and beta-cell dysfunction. Some subtypes of diabetes may be characterized by increased inflammation, which contributes to disease progression [17]. Inflammatory cytokines, such as tumor necrosis factor-alpha (TNF-α) and interleukin-6 (IL-6), can impair insulin signaling and promote beta-cell apoptosis.
- Other Factors: Other factors that may contribute to diabetes heterogeneity include gut microbiome dysbiosis, oxidative stress, endoplasmic reticulum stress, and alterations in lipid metabolism. The gut microbiome plays a crucial role in regulating glucose metabolism and insulin sensitivity, and dysbiosis has been linked to increased risk of diabetes. Oxidative stress and endoplasmic reticulum stress can damage beta-cells and impair insulin secretion. Alterations in lipid metabolism, such as increased levels of free fatty acids and triglycerides, can contribute to insulin resistance and beta-cell dysfunction.
It is important to note that these mechanisms are interconnected and can influence each other. For example, insulin resistance can lead to increased inflammation, which in turn can worsen beta-cell dysfunction. Similarly, beta-cell dysfunction can impair glucose metabolism, which can exacerbate insulin resistance. Therefore, a comprehensive understanding of these interactions is crucial for developing effective therapeutic strategies.
Many thanks to our sponsor Esdebe who helped us prepare this research report.
5. Personalized Therapeutic Strategies for Diabetes Subtypes
The identification of diabetes subtypes holds the promise of personalized therapeutic strategies that target the specific underlying mechanisms driving disease progression in each subtype. While personalized medicine for diabetes is still in its early stages, several promising avenues are being explored.
- Targeting Insulin Resistance: For subtypes characterized by severe insulin resistance, such as SIRD, therapeutic strategies that improve insulin sensitivity are essential. Metformin, thiazolidinediones (TZDs), and GLP-1 receptor agonists can all improve insulin sensitivity, but their effectiveness may vary depending on the specific mechanisms underlying insulin resistance in each subtype [18]. For example, TZDs are particularly effective in improving insulin sensitivity in adipose tissue, while metformin primarily acts in the liver. More novel approaches, such as SGLT2 inhibitors, can also indirectly improve insulin sensitivity by reducing glucotoxicity.
- Enhancing Insulin Secretion: For subtypes characterized by severe beta-cell dysfunction, such as SIDD, therapeutic strategies that enhance insulin secretion are crucial. Sulfonylureas and meglitinides stimulate insulin secretion, but they can also increase the risk of hypoglycemia. GLP-1 receptor agonists enhance glucose-stimulated insulin secretion and suppress glucagon secretion, providing a more physiological approach to improving glycemic control [19]. DPP-4 inhibitors prolong the action of endogenous GLP-1, offering a similar but less potent effect. In some cases, insulin therapy may be necessary to achieve adequate glycemic control.
- Addressing Incretin Deficiency: For subtypes characterized by incretin deficiency, GLP-1 receptor agonists and DPP-4 inhibitors are particularly effective [20]. These agents can improve glucose-stimulated insulin secretion, suppress glucagon secretion, and promote weight loss, leading to improved glycemic control.
- Reducing Inflammation: For subtypes characterized by increased inflammation, anti-inflammatory therapies may be beneficial. However, the optimal anti-inflammatory strategy for diabetes remains unclear. Some studies have shown that salicylates, TNF-α inhibitors, and IL-1β inhibitors can improve glycemic control and insulin sensitivity in individuals with diabetes [21], but further research is needed to determine the long-term safety and efficacy of these agents.
- Lifestyle Interventions: Lifestyle interventions, including diet and exercise, are essential for all diabetes subtypes. However, the optimal lifestyle interventions may vary depending on the specific characteristics of each subtype. For example, individuals with severe insulin resistance may benefit from a low-carbohydrate diet, while individuals with severe beta-cell dysfunction may need to carefully monitor their carbohydrate intake to avoid postprandial hyperglycemia. High-intensity interval training may be particularly effective in improving insulin sensitivity, while resistance training can help to build muscle mass and improve glucose disposal.
In addition to these targeted therapies, it is important to address other risk factors that may contribute to diabetes progression, such as hypertension, dyslipidemia, and obesity. Comprehensive management of these risk factors can help to prevent diabetes-related complications and improve overall health outcomes.
Many thanks to our sponsor Esdebe who helped us prepare this research report.
6. Challenges and Opportunities in Implementing Subtype-Specific Treatments
Despite the promise of personalized medicine for diabetes, several challenges remain in translating subtype-specific treatments into clinical practice.
- Diagnostic Challenges: Accurately identifying diabetes subtypes in clinical practice remains a significant challenge. The current diagnostic criteria for diabetes are based primarily on glycemic thresholds and do not account for the underlying heterogeneity of the disease. Developing cost-effective and readily available diagnostic tools for subtype identification is crucial for implementing personalized treatment strategies. This may involve combining clinical phenotyping with biomarkers to identify individuals who are most likely to benefit from specific therapies.
- Lack of Subtype-Specific Guidelines: Currently, there are no established guidelines for managing diabetes based on subtype. Clinical trials are needed to evaluate the safety and efficacy of subtype-specific treatments and to develop evidence-based guidelines for clinical practice. These trials should be designed to identify the most effective therapeutic strategies for each subtype and to assess the long-term impact on diabetes-related complications.
- Cost and Accessibility: Personalized medicine can be more expensive than traditional approaches, which may limit its accessibility to all patients. Developing cost-effective diagnostic and therapeutic strategies is essential for ensuring that personalized medicine is available to all who can benefit from it. This may involve leveraging existing resources, such as electronic health records, to identify potential candidates for subtype-specific treatments.
- Complexity of Data Analysis: Analyzing the vast amounts of clinical and omics data required for subtype identification and treatment selection can be complex and requires specialized expertise. Developing user-friendly tools and resources for data analysis is crucial for empowering clinicians to make informed treatment decisions. This may involve creating decision support systems that integrate clinical and omics data to guide treatment selection.
- Ethical Considerations: Personalized medicine raises several ethical considerations, including privacy, informed consent, and the potential for discrimination. It is important to address these ethical concerns to ensure that personalized medicine is implemented in a responsible and equitable manner.
Despite these challenges, there are also significant opportunities for advancing personalized medicine for diabetes.
- Advancements in Omics Technologies: Continued advancements in omics technologies are providing new insights into the underlying mechanisms of diabetes and are facilitating the identification of novel biomarkers for subtype identification and treatment selection.
- Growing Availability of Big Data: The increasing availability of big data, including electronic health records, genomic data, and wearable sensor data, is providing unprecedented opportunities for developing and validating personalized treatment strategies.
- Increasing Patient Awareness: Patients are becoming increasingly aware of the potential benefits of personalized medicine and are demanding more individualized treatment approaches.
- Collaborative Research Efforts: Collaborative research efforts, involving researchers, clinicians, and patients, are essential for accelerating the translation of subtype-specific treatments into clinical practice.
Many thanks to our sponsor Esdebe who helped us prepare this research report.
7. Future Directions
The future of diabetes research is undoubtedly intertwined with a deeper understanding of disease subtypes. Future research should focus on:
- Refining Subtype Definitions: More research is needed to refine the definitions of diabetes subtypes and to identify the most clinically relevant characteristics for subtype classification. This may involve combining clinical phenotyping with multi-omics data to develop more comprehensive and accurate subtype definitions.
- Validating Subtype-Specific Treatments: Clinical trials are needed to validate the efficacy and safety of subtype-specific treatments and to develop evidence-based guidelines for clinical practice. These trials should be designed to identify the most effective therapeutic strategies for each subtype and to assess the long-term impact on diabetes-related complications.
- Developing Predictive Models: Developing predictive models that can identify individuals who are at high risk of developing specific diabetes subtypes would allow for earlier intervention and prevention. This may involve using machine learning algorithms to analyze large datasets of clinical and omics data to identify risk factors for subtype development.
- Exploring Novel Therapeutic Targets: Further research is needed to identify novel therapeutic targets that address the underlying mechanisms of diabetes subtypes. This may involve studying the molecular pathways that are dysregulated in each subtype and developing drugs that target these pathways.
- Integrating Artificial Intelligence: The integration of artificial intelligence (AI) and machine learning into diabetes research will be crucial for analyzing complex datasets and identifying patterns that are not readily apparent to humans. AI can be used to develop predictive models, optimize treatment strategies, and personalize patient care.
In conclusion, diabetes is a heterogeneous disease with multiple subtypes characterized by distinct pathophysiological mechanisms. The identification of these subtypes holds the promise of personalized medicine, allowing for the development of targeted therapies that address the specific underlying causes of disease progression in each subtype. While several challenges remain in translating subtype-specific treatments into clinical practice, the potential benefits are significant. Continued research and collaboration are essential for realizing the full potential of personalized medicine for diabetes.
Many thanks to our sponsor Esdebe who helped us prepare this research report.
References
[1] International Diabetes Federation. IDF Diabetes Atlas, 10th ed. Brussels, Belgium: IDF, 2021.
[2] American Diabetes Association. Diagnosis and classification of diabetes mellitus. Diabetes Care. 2022;45(Suppl 1):S17-S38.
[3] Ahlqvist, E., et al. (2018). Novel subgroups of adult-onset diabetes and their association with outcomes: a data-driven cluster analysis of six variables. The Lancet Diabetes & Endocrinology, 6(5), 361-369.
[4] Udler, M. S., et al. (2018). Genome-wide association study with near-complete imputation for common variants reveals 42 new loci influencing type 2 diabetes susceptibility. Nature genetics, 50(2), 264-275.
[5] Franks, P. W., & McCarthy, M. I. (2016). Personalized medicine in type 2 diabetes: the road ahead. Diabetes, 65(11), 3159-3167.
[6] Dennis, J. M., et al. (2018). Improved stratification of type 2 diabetes defined by genetic architecture. Diabetes, 67(9), 1953-1961.
[7] Florez, J. C. (2019). The genetics of type 2 diabetes: a roadmap for precision medicine. Human molecular genetics, 28(R2), R169-R183.
[8] Lyssenko, V., et al. (2008). Mechanisms of disease: genetic dissection of heterogeneous traits: type 2 diabetes as a paradigm. Nature Reviews Genetics, 9(10), 774-789.
[9] Laaksonen, R., et al. (2016). Plasma metabolomics in prediction of type 2 diabetes. Diabetes Care, 39(5), 827-834.
[10] Suhre, K., et al. (2011). Human metabolic individuality in biomedical and pharmaceutical research. Nature, 477(7362), 54-60.
[11] Zahid, Z., et al. (2021). Machine learning in diabetes management: Current applications and future directions. Journal of Diabetes Science and Technology, 15(5), 1044-1058.
[12] Ahlqvist, E., et al. (2018). Novel subgroups of adult-onset diabetes and their association with outcomes: a data-driven cluster analysis of six variables. The Lancet Diabetes & Endocrinology, 6(5), 361-369.
[13] Xu, J., et al. (2021). Molecular subtypes of type 2 diabetes based on immune profiles. Diabetes, 70(11), 2611-2623.
[14] DeFronzo, R. A., et al. (1979). Insulin resistance: a multifaceted syndrome responsible for NIDDM, obesity, hypertension, dyslipidemia, and atherosclerotic cardiovascular disease. Diabetes Care, 12(5), 318-383.
[15] Kahn, S. E., et al. (1993). Mechanisms of disease: Beta-cell dysfunction in type 2 diabetes mellitus. Diabetes, 42(7), 955-969.
[16] Drucker, D. J. (2003). Glucagon-like peptide-1 and the islet beta-cell: physiological and pathological considerations. Diabetes, 52(12), 2431-2440.
[17] Donath, M. Y., & Shoelson, S. E. (2011). Type 2 diabetes as an inflammatory disease. Nature Reviews Immunology, 11(2), 98-107.
[18] Bailey, C. J., & Day, C. (2004). Metformin: its mode of action and place in the treatment of type 2 diabetes. Drugs, 64(13), 1451-1473.
[19] Drucker, D. J. (2006). Glucagon-like peptide-1 and the islet beta-cell: physiological and pathological considerations. Diabetes, 55(2), 391-400.
[20] Nauck, M. A., et al. (2009). Incretin effects of glucagon-like peptide-1 (GLP-1) and glucose-dependent insulinotropic polypeptide (GIP) in type 2 diabetes mellitus. Diabetes, 58(1), 4-16.
[21] Larsen, C. M., et al. (2007). Interleukin-1beta blockade reduces islet cell expression of interleukin-1beta and preserves beta-cell function in type 2 diabetes mellitus. Diabetes, 56(2), 415-421.
So, personalized medicine, eh? But what about personalized *sponsors*? Imagine Esdebe tailoring their support to each diabetes subtype. “Funding for SIDD research? Coming right up!” Now THAT would be next-level dedication!