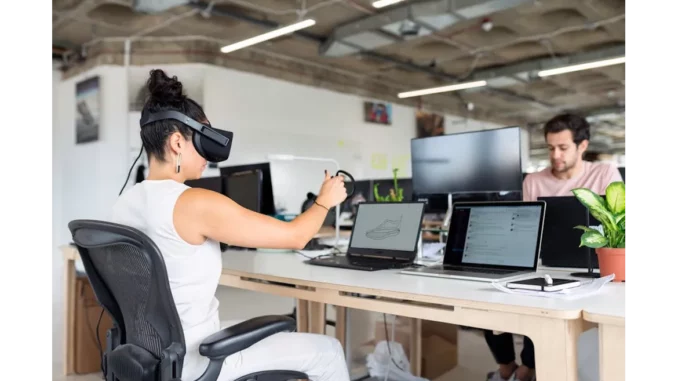
Upon meeting Dr. Sarah Bennett, a senior researcher at the Queensland University of Technology (QUT), one is immediately captivated by her palpable enthusiasm for melt electrowriting (MEW) technology. As she delves into the nuances of this burgeoning field, it becomes clear that MEW is positioned at the intriguing crossroads of advanced engineering and the next frontier of biomedical applications. Dr. Bennett, along with her colleagues, is pioneering efforts to harness machine learning to elevate 3D printing to unprecedented heights. Led by prominent figures such as Dr. Pawel Mieszczanek and Distinguished Professor Dietmar W. Hutmacher, the QUT team is setting new benchmarks in high-resolution 3D printing.
MEW distinguishes itself from other 3D printing technologies by its multifaceted potential, spanning domains such as bioengineering, biomaterials science, and soft robotics. “We’re unlocking possibilities that could redefine tissue engineering and regenerative medicine,” Dr. Bennett explained, brimming with optimism. However, the journey to this point was not without its challenges. Since its inception over a decade ago, MEW has grappled with lengthy experimentation periods, inconsistent results, sluggish printing speeds, and a heavy reliance on manual controls. “Initially, it was quite daunting,” Dr. Bennett admitted, “but we were driven by the promise of what MEW could become.”
A turning point arrived with the integration of machine learning into MEW technology. This innovation enabled the creation of a closed-loop process control system, effectively automating MEW operations. Dr. Bennett described this advancement as transformative: “With machine learning, we can monitor and analyse the fibre-flight path in real-time using continuous imaging. It’s akin to endowing the printer with cognitive abilities.” The design of this novel system employs a feedforward neural network, coupled with optimisation techniques and feedback loops, ensuring consistent reproducibility of printed components—an essential feature for applications in sensitive fields like tissue engineering.
The impact of machine learning on MEW is profound, reducing experimental times from weeks to mere hours, thereby accelerating research and edging closer to industry-ready additive manufacturing. The automation of data collection has significantly reduced human error and maximised efficiency. “It’s about augmenting human capabilities rather than replacing them,” Dr. Bennett emphasised. The potential applications of these advancements are extensive. In regenerative medicine, for instance, the production of high-resolution, consistent 3D-printed scaffolds could revolutionise tissue repair and regeneration strategies, enabling more tailored and effective patient treatments.
Dr. Bennett also highlighted the collaborative nature of this endeavour, which involves partnerships with esteemed researchers such as Distinguished Emeritus Professor Peter Corke and academics from the University of Oregon. “The diversity of our team enriches the project with a breadth of knowledge and perspectives, fostering innovation and exploration of uncharted territories,” she noted. These interdisciplinary collaborations are instrumental in pushing the boundaries of what is possible with MEW and machine learning.
Reflecting on the future of MEW and machine learning in 3D printing, Dr. Bennett’s outlook is decidedly optimistic. “We’re only beginning to tap into the potential of these technologies. As we continue to refine our methods, we anticipate a fundamental shift not just in printing, but in manufacturing as a whole,” she envisioned. This future promises to be one where machines not only assist humans but also learn and adapt alongside them, expanding the horizons of what can be achieved.
The conversation with Dr. Bennett left a lasting impression, underscoring the remarkable progress being made behind the scenes in the realm of 3D printing. The integration of machine learning into MEW signifies a major leap forward, with the potential to profoundly influence a multitude of scientific disciplines. For observers, this represents a tantalising glimpse into a technological future where our tools are intelligent partners in innovation, reshaping our capabilities in ways we are just beginning to comprehend.
Be the first to comment