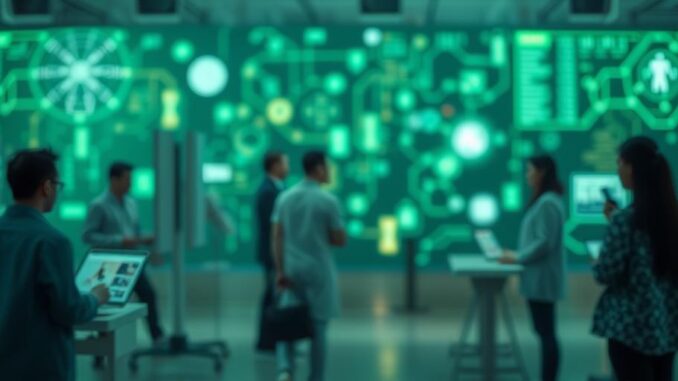
Summary
This article explores seven key challenges in integrating AI into healthcare, focusing on data fragmentation, ethical concerns, user adoption, security, workforce adaptation, maintaining accuracy, and expanding AI capabilities. It proposes practical solutions for each challenge, emphasizing the importance of a strategic approach, ongoing training, and interdisciplinary collaboration. Successfully addressing these challenges will pave the way for AI to revolutionize healthcare delivery and improve patient outcomes.
** Main Story**
AI’s poised to shake up healthcare, promising leaps forward in everything from diagnosing illnesses to tailoring treatments. But, let’s be real, slotting AI into the already complex world of healthcare isn’t exactly a walk in the park. There are hurdles to clear, and some of them are pretty significant.
So, what are the big roadblocks preventing a seamless AI takeover? I’ve broken down seven key challenges – and some potential solutions – that we need to be thinking about.
Data Fragmentation: The Information Scramble
Imagine trying to build a house with bricks scattered across different construction sites. That’s pretty much what working with healthcare data feels like. It often lives in separate systems, making it a massive pain to pull together the comprehensive datasets needed to train AI algorithms effectively. The end result? Inaccurate analysis, or worse, incomplete insights. I remember working on a project last year where we spent nearly half our time just wrangling the data into a usable format. It was a nightmare.
- Remedy: Get serious about data integration. Standardize those formats, build systems that talk to each other, and consider a data lake or warehouse to centralize everything. It’s a big investment, sure, but it’s essential.
Ethical Minefield: Navigating the Moral Maze
With great power comes great responsibility, right? AI raises some seriously knotty ethical questions. Data privacy is paramount, bias in algorithms is a real concern, and what about the potential for AI to replace human healthcare workers? We’ve got to protect patient data, tackle algorithmic bias head-on, and clearly define the roles for both AI and us humans. It’s a must for responsible AI integration.
- Remedy: We need clear ethical guidelines, plain and simple. Promote transparency in how algorithms work and how data is used. And for goodness sake, let’s have open discussions with ethicists, clinicians, and patients to get everyone on board. It’s about building trust.
User Adoption: Overcoming the Skepticism
Let’s face it; not everyone’s thrilled about the idea of AI in healthcare. Some professionals are worried about their jobs, others find AI systems too complex. And who can blame them? Nobody wants to be replaced by a machine, or spend hours troubleshooting a complicated system.
- Remedy: Education is key. We need to train healthcare pros on the benefits of AI and its practical uses. Start with AI systems that mirror current activities to demonstrate how its reliable. Open communication, addressing concerns? All important.
Security and Compliance: Guarding the Treasure Trove
Healthcare data is gold dust for hackers, so security is non-negotiable. AI systems must adhere to strict regulations like HIPAA to protect patient privacy and keep data safe. Compliance is mandatory.
- Remedy: Beef up cybersecurity, implement data encryption and access controls, and carry out regular audits. Stay updated on all those compliance regulations, so your AI systems adhere to the highest standards.
Workforce Adaptation: Preparing for the Robot Revolution
AI is changing the game, and that means job roles are going to evolve. We need to prepare our workforce for these changes through training and education. It’s about upskilling and reskilling to meet future demands. Are we doing this? Often times it seems like we are behind the curve.
- Remedy: Training programs that focus on AI literacy and the changing roles are the way to go. Offer upskilling and reskilling to meet demands, and foster a culture of continuous learning.
Maintaining Accuracy: The Reliability Factor
AI systems need to be accurate. Inaccurate diagnoses or treatment recommendations could have serious consequences. Ongoing monitoring and validation are crucial. So important.
- Remedy: Quality control is key, like continuous monitoring, regular validation with real data, and mechanisms for error correction.
Expanding AI Capabilities: Pushing the Envelope
AI is like a toddler, it’s always growing and changing, you know? Continuously exploring new capabilities and applying them in healthcare is how we get the most out of it.
- Remedy: A culture of innovation and experimentation is essential. Promote collaboration between researchers, clinicians, and tech developers. Invest in R&D to push boundaries, the further the better.
In conclusion, integrating AI into healthcare isn’t easy, but it’s definitely worth the effort. With the right approach, ongoing training, and collaboration, we can unlock AI’s potential to revolutionize healthcare and improve lives. It will take time but the end result will be worth it.
The point about data fragmentation is crucial. Federated learning approaches, which train AI models across decentralized data sources, could offer a promising solution while addressing privacy and security concerns.
Great point about federated learning! It’s definitely a promising avenue for tackling data fragmentation while respecting data privacy. I’m curious to hear about any specific use cases you’ve seen where federated learning has made a significant impact in healthcare. Has it been more successful in particular domains or with certain types of data?
Editor: MedTechNews.Uk
Thank you to our Sponsor Esdebe
Data fragmentation, the bane of every AI hopeful! Wrangling data felt like herding cats last year. Anyone found a foolproof way to standardize formats without losing crucial nuances? Asking for a friend… who may or may not be an AI.
Haha, the ‘herding cats’ analogy is spot on! We found some success using metadata tagging to maintain nuance during standardization. It’s not foolproof, but it helped preserve context. Anyone else have tricks for wrangling fragmented data without losing key details?
Editor: MedTechNews.Uk
Thank you to our Sponsor Esdebe