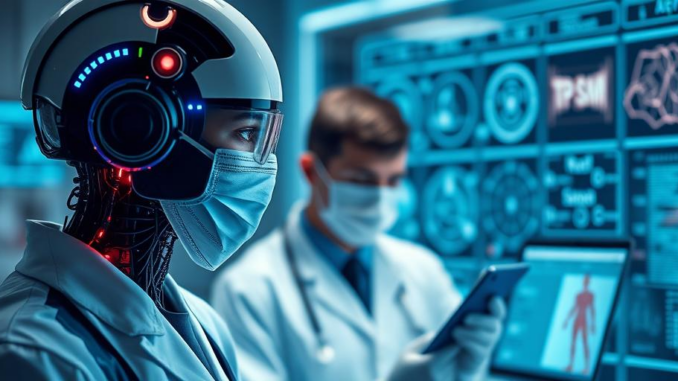
Summary
The rapid growth of AI in medicine raises concerns about adequate testing. Current benchmarks often rely on medical student exams and lack real-world patient data. This article explores the shortcomings of current testing methods and the need for more robust evaluations to ensure patient safety and effective implementation.
Healthcare data growth can be overwhelming scale effortlessly with TrueNAS by Esdebe.
** Main Story**
The Rise of AI in Medicine: A Double-Edged Scalpel
Artificial intelligence is making waves in healthcare, that’s for sure. From sifting through breast cancer screenings to even writing up clinical notes, it’s popping up everywhere. We’re even seeing AI in health insurance management and those virtual nurse apps. It’s a brave new world, right? Companies are really pushing the idea of boosting efficiency and easing the load on healthcare workers. But, and it’s a big but, are these tools really living up to the hype? Experts are scratching their heads, wondering if they’ve really been put through their paces properly. I mean, are we sure they’re ready for prime time?
The Shortcomings of Current AI Testing
So, here’s the thing: a lot of the ways we’re testing these AI tools just aren’t cutting it. It’s like, instead of seeing how they handle actual patients, they’re being graded on old medical school exams, like the MCAT. Seriously? I read this review that said only about 5% of studies looking at healthcare AI used real patient data. Can you believe that? That’s a huge problem! It makes you wonder if the results even mean anything in the real world. Makes you think about if they are really safe, and how much we can actually use them.
The Need for Real-World Evaluation
The current benchmarks? Well, they can be pretty distracting. They’re not really measuring what matters most: actual clinical skills. You know, how well can the AI diagnose a tricky case, suggest the right treatment, or even just communicate effectively with a patient? What about nurses and other medical staff, surely there input is also important? Often, these tests don’t consider the complexity of real-life situations, and they’re too rigid to evaluate different clinical tasks. Plus, since a lot of tests rely on physician knowledge, they might miss out on the valuable insights of nurses and other medical staff.
Rethinking AI Benchmarks: A Call for Task-Oriented Evaluation
Now, some experts are suggesting a different approach, which I think is a great idea. Instead of focusing on knowledge-based tests, they want task-oriented evaluations. Imagine this: instead of answering multiple-choice questions, an AI tool has to write a prescription, summarize a patient conversation, or even interact with a virtual patient. This, right here, is gold. It would give us a much clearer picture of how well it can actually perform in a clinical setting. And honestly, shouldn’t that be the goal?
Addressing Bias in AI: A Critical Consideration
And don’t even get me started on bias. If AI algorithms are trained on biased or incomplete data, they are going to give skewed results. And who suffers? Patients. That could make existing health inequalities even worse. To build trust and make sure everyone gets fair treatment, AI systems need to be transparent, explainable, and free from bias. We need to be able to understand how these systems are making decisions, and we need to make sure those decisions are fair.
Collaboration and Standardization: A Path to Responsible AI Implementation
Making AI work in healthcare? That’s going to take a village. We need healthcare providers, tech developers, and regulatory agencies all working together. Clear guidelines and standards for data collection, model training, and performance evaluation are a must. Oh, and we can’t just set it and forget it. We need continuous monitoring and adjustments to make sure AI stays reliable and effective for all patient populations. It requires a level of collaboration, that lets be honest, isn’t always there, but it needs to be!
The Future of AI in Healthcare: Balancing Innovation with Patient Safety
Look, AI has the potential to transform healthcare, no doubt about it. I remember when I first saw a demo of an AI-powered diagnostic tool – it was mind-blowing! But we’ve got to remember that potential can only be unlocked through rigorous testing and careful implementation. We have to prioritize real-world evaluation, tackle bias head-on, and build strong partnerships. That’s the only way we’ll create a future where AI actually enhances patient care while keeping safety and ethics front and center. Because at the end of the day, that’s what really matters, doesn’t it?
The call for task-oriented evaluations is crucial. Expanding this, how can we simulate the dynamic and unpredictable nature of a real clinical environment within these evaluations to better assess AI’s adaptability and decision-making under pressure?
Great point about simulating real-world unpredictability! Maybe incorporating unexpected events during AI evaluations, like equipment malfunctions or sudden changes in patient conditions, could push the AI to truly demonstrate its adaptability and critical thinking under pressure. What are your thoughts on that?
Editor: MedTechNews.Uk
Thank you to our Sponsor Esdebe
The point about biased data is critical. How can we ensure diverse datasets are used for training AI, reflecting the wide range of patient demographics and conditions, to mitigate the risk of perpetuating health inequalities?
Thanks for highlighting the importance of diverse datasets! It’s definitely a crucial area. One approach involves actively seeking out and incorporating data from underrepresented populations. This requires collaboration between healthcare providers, researchers, and community organizations to ensure we’re building truly representative datasets and mitigating potential biases.
Editor: MedTechNews.Uk
Thank you to our Sponsor Esdebe