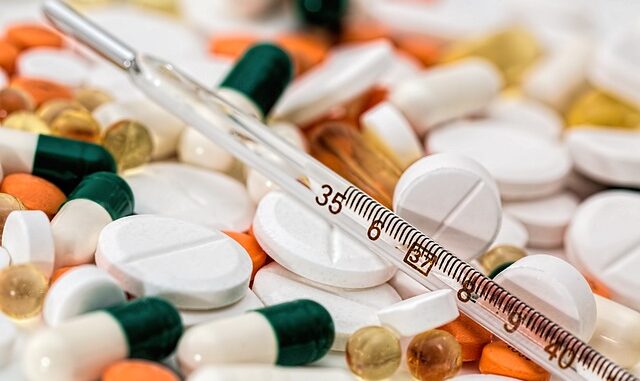
Summary
AI models now predict bacterial resistance to antibiotics with impressive accuracy. This breakthrough offers new tools for combating the growing threat of drug-resistant infections. These models leverage large genetic datasets to understand resistance development, paving the way for improved diagnostics and targeted interventions.
Healthcare data growth can be overwhelming scale effortlessly with TrueNAS by Esdebe.
** Main Story**
Okay, so, antibiotic resistance. It’s a real problem, right? I mean, common infections are becoming a nightmare to treat, and think about things like organ transplants – suddenly, they’re way riskier. But there’s some seriously cool stuff happening with AI that could be a game-changer.
Basically, researchers have built AI models that can predict which bacteria will become resistant to antibiotics, and they’re doing it with pretty amazing accuracy. This isn’t just incremental progress; it’s a potential revolution in how we handle these drug-resistant infections. How’s it working? Let’s take a look.
How AI Is Predicting Resistance
These AI models are analyzing massive amounts of genetic data from bacterial samples. Think of it like teaching a computer to spot patterns. It’s a data-driven approach, and it allows the AI to identify genetic markers that are linked to antibiotic resistance.
-
Early detection is a huge deal. AI can spot resistance before it even shows up in clinical settings. That means healthcare providers can tailor treatment, using the right antibiotics from the get-go. Which means we’re more likely to win, and less likely to accidentally breed even stronger bacteria. It’s like precision medicine, but for infectious diseases.
-
Tracking resistance trends: The models can follow how resistance genes spread. This is crucial for understanding how resistance develops and, more importantly, spreads. It helps us find the hotspots – places like wastewater treatment plants or even our own bodies – and then we can actually, you know, do something about it. I remember reading a paper on that a few months back, fascinating stuff.
-
New diagnostic tools: Imagine AI built into molecular diagnostics. We could detect new multi-resistant bacteria faster and more accurately than ever before. Quicker detection means quicker intervention, potentially saving lives. That’s the bottom line, isn’t it?
What’s Next for AI in This Fight?
It’s a big deal, this AI development. But, it’s important to say that researchers aren’t just stopping here, they’re trying to improve the models and broaden how we use them. So what’s next? A few thoughts:
-
Bigger Datasets: More data means better predictions. Training these models on larger, more diverse datasets will only make them more powerful. The more the merrier really.
-
Cross-Species Prediction: What if we could predict resistance across different types of bacteria? That would give us a way broader understanding of how resistance works. A single model would be groundbreaking.
-
Real-time Monitoring: Imagine AI integrated into systems that are constantly monitoring for resistance. We’d get early warnings of new threats, giving us time to prepare. That has got to be a good thing.
That said, AI isn’t just about antibiotics. It’s popping up everywhere in medicine: diagnostics, personalized medicine, drug discovery. The list goes on. And as the technology gets better, expect even more of this. It all points to better patient care, better treatment outcomes, and a totally new way of tackling global health challenges.
Ultimately, it all comes back to this: AI models predicting antibiotic resistance are a major step forward. By using the power of AI, we can work towards a future where we can better manage the problems antibiotic resistance is creating, protecting public health worldwide. And frankly? I think that’s pretty awesome.
The potential for early detection of antibiotic resistance using AI is truly exciting. I wonder what the implications are for proactive public health interventions, particularly in developing countries with limited access to advanced diagnostics.
That’s a great point! The implications for proactive public health in developing countries are definitely key. Thinking about how AI-driven diagnostics can be adapted for resource-limited settings is crucial for equitable access to these advancements. Perhaps mobile, AI-powered tools could bridge the gap?
Editor: MedTechNews.Uk
Thank you to our Sponsor Esdebe
Given the models’ reliance on genetic data, how might variations in data collection and sequencing technologies across different regions affect the accuracy and generalizability of these AI predictions globally?